Identification of pancreatic adenocarcinoma immune subtype associated with tumor neoantigen from aberrant alternative splicing
Highlight box
Key findings
• We performed an integrated analysis of aberrantly upregulated genes, alternatively spliced genes, genes associated with nonsense-mediated RNA decay factors, antigen presentation and overall survival in The Cancer Genome Atlas-pancreatic adenocarcinoma (PAAD). We revealed that PLEC is a promising neoantigen for PAAD-targeted therapy. We also propose a novel immune subtyping system for PAAD to indicate patient prognosis and opportunities for immunotherapy, such as immune checkpoint inhibitors.
What is known and what is new?
• PAAD is referred to as an immunologically “cold” tumor that responds poorly to immunotherapy. Some previous studies have shown that PAAD patients with long-term survival have specific neoantigen profiles, and suggested that the interaction between neoantigens and the immune system plays an important role in prognosis. Alternative splicing of pre-mRNA, which could alter the proteomic diversity of many cancers, has been reported to be involved in neoantigen production. However, related neoantigens have not been studied in PAAD.
• Our work identified a promising neoantigen via aberrant alternative splicing, and provides guidance for immunotherapy which will aid in patient selection for appropriate treatment.
What is the implication, and what should change now?
• Overall, mRNA vaccines based on neoantigens derived from PLEC may become a promising strategy to boost the immunogenicity of PAAD.
Introduction
Pancreatic adenocarcinoma (PAAD) remains one of the most malignant cancers, with dismal prognosis (1,2). In the era in which immunotherapy is a widely recognized approach to treat many cancers, scarce convincing evidence has illustrated the clinical value of immunotherapy, especially immune checkpoint (ICP) inhibitors, for PAAD patients (3). In this context, PAAD is commonly referred to as an immunologically “cold” tumor that lacks sufficient response towards immunotherapy (4).
Tumors have long been regarded as a “self” component without immunogenicity (5,6). However, with an increasing number of studies revealing that genome alterations occur in malignant cells, it seems that some tumor-derived peptides can trigger immune responses (7,8). Despite some controversies, the tumor mutation burden (TMB) has been widely reported as a factor that can predict the clinical outcomes of patients receiving immunotherapy (9,10), and a fundamental theory explaining the low immunogenicity of PAAD is the dramatically low TMB of PAAD tumors. PAAD is characterized by the mutations of four driver genes: KRAS, SMAD4, TP53 and CDKN2A (11,12). Partial mutations of these genes have been reported to show a mild association with immune infiltrates in PAAD; however, no stringent findings support promising translational value for clinical decision-making. Nonetheless, not all PAADs feature a “desert” tumor microenvironment, and abundant immune infiltrates can still be found in a portion of patients with PAAD (13,14). Notably, Bailey et al. identified a pancreatic ductal adenocarcinoma (PDAC) subtype designated as immunogenic tumors with altered immune pathways (13). Hence, tumor antigens generated by other genomic events beyond mutations with high frequencies may play an important role in immune cell recruitment in PAAD. Alternative splicing of pre-mRNA, which can alter the proteomic diversity of many cancers, has been reported to be involved in neoantigen production (15,16). Recent studies have revealed the correlation between alternative splicing and both immune cell infiltration and patients’ prognosis in multiple cancers (17-20). Aberrant alternative splicing is associated with poor prognosis of cancer patients (21-23). The interplay of alternative splicing, immune microenvironment and patients’ prognosis seemed to be promising in patients’ clinical management (24). Integrated analysis of such events and the transcriptome will contribute to the exploration of potential targets for immunotherapy.
In this study, we performed systematic bioinformatic analyses to explore alternative splicing events, novel PAAD antigens and immune subtypes. Our findings identified a subpopulation of PAADs that is associated with better prognosis and may benefit from antigen-based immunotherapy. We present this article in accordance with the MDAR reporting checklist (available at https://jgo.amegroups.com/article/view/10.21037/jgo-24-340/rc).
Methods
Biological sources
The data used for splicing analysis were downloaded from The Cancer Genome Atlas (TCGA) SpliceSeq database (https://bioinformatics.mdanderson.org/TCGASpliceSeq/singlegene.jsp) in 3rd January 2022, of which percent spliced in (PSI) with 0 or 1 was filtered out. The TCGA-PAAD expression and mutation profile data were downloaded from Xena (https://xenabrowser.net/datapages/). In addition, clinical and follow-up information was acquired from the same database. The bulk transcriptome dataset GSE62452 and single-cell dataset GSE154778 were downloaded from the Gene Expression Omnibus (GEO) database (https://www.ncbi.nlm.nih.gov/geo/). We performed pretreatment for gene chip data as follows: (I) the probe was mapped to the gene according to the annotation file; (II) the empty probe was removed; and (III) the maximum value corresponding to the same gene was selected as the expression level of the gene. The study was conducted in accordance with the Declaration of Helsinki (as revised in 2013).
Analysis of alternative splicing events
According to the grouping of samples, we used the Wilcox test to detect the difference in alternative splicing events between two groups. P<0.05 was considered significant when screening alternative splicing events. The packages “clusterProfiler” and “ReactomePA” were used for Gene Ontology (GO) and Reactome enrichment analysis.
Detection of differentially expressed genes (DEGs)
DESeq2 was performed to explore DEGs between PAAD and normal pancreas samples. The threshold to screen DEGs was set as |log fold change (FC)| >1 and P.adj <0.05. Transcriptome data of the normal pancreas were downloaded from the Genotype-Tissue Expression (GTEx) dataset (https://www.gtexportal.org/home/index.html) in 3rd January 2022.
Cox regression analysis
Univariate Cox analysis was performed using the coxph function of the “survival” R package, which analyzes the impact of the expression value of a single gene or feature gene on the overall survival (OS) or disease-free survival (DFS) of patients. The regression model was based on prognosis-related genes with P<0.05, extracting corresponding modeling parameters, and a forest plot was obtained by using the R package “forestplot”. Multivariate Cox analysis was used to construct a multiple gene-involved model based on the coxph function of the survival package.
Estimation of immune cell infiltration in PAAD
Immune scores, including cell infiltration and microenvironment scores, were downloaded from the TIMER database (http://timer.comp-genomics.org/). Among the available algorithms, we chose CIBERSORT to evaluate the immune cell distribution among PAADs. We calculated the correlation between candidate gene expression and immune cell percentage using Spearman statistics. The cytolytic activity (CYT) score was calculated with the average expression level of GZMA and PRF1 (25). For International Cancer Genome Consortium (ICGC) data, immune cell infiltration was calculated using CIBERSORT algorithms. Signature based on 28 tumor-infiltrating lymphocyte (TIL) subpopulations [effector and memory T cells and immunosuppressive cells Tregs, myeloid-derived suppressor cells (MDSCs)] was collected from a previous study (26) and used to score each sample. Pseudotime analysis was performed using the R package “monocle”, and the top two principal components correlated with immune signatures.
Consensus clustering
An immune gene set was downloaded from ImmPort, and univariate Cox regression was used to screen prognosis-related genes. The expression profile was normalized using the median value. The R package “ConsensusClusterPlus” was employed to perform consensus clustering based on the Partitioning Around Medoids (PAM) method with 200 bootstraps.
Somatic mutation, copy number variation (CNV) analysis and genome signature score
The maf file was downloaded from TCGA with the Mutect analytic version, and nonsynonymous mutations were screened among tumor samples. TMB was calculated using the R package “maftools” with visualization. The CNV calculated by Genomic Identification of Significant Targets in Cancer (GISTIC) was downloaded from the Xena database. The frequency of CNV gain and loss samples was calculated and visualized. The DNA damage repair (DDR) score was downloaded from the website https://gdc.cancer.gov/about-data/publications/PanCan-DDR-2018. The neoantigen list for PAAD was downloaded from The Cancer Immunome Atlas (TCIA) database (https://tcia.at/home). Chromosome instability genes were collected from a previously published paper (27). The chromosome instability score was calculated as the average value of the expression level of these genes; the stemness score of PAAD samples was calculated according to a previously published algorithm (28).
Single-cell analysis
Single-cell analysis was performed based on the Seurat workflow. Feature gene >1,000, mitochondrial gene <5%, and RNA count <10,000 were the criteria for cell quality control. To investigate intercellular communication pathways within our samples, we utilized the “CellChat” R package, a comprehensive framework for inferring, visualizing, and analyzing cell-cell communication networks based on single-cell RNA sequencing data. This tool identifies potential signaling interactions between cells by analyzing the expression patterns of known ligands, receptors, and corresponding cofactors.
Construction of the weighted gene co-expression network analysis (WGCNA) co-expression network
We screened the expression levels of immune genes in TCGA-PAAD using the WGCNA package, and the PAAD expression matrix was used to construct a co-expression network. The soft power was calculated from 1 to 10, and the scale-free fitting index and the average connectivity of the network varied with the soft threshold parameters. With the scale-free fitting index >0.8 as the threshold, the soft power was set to 4 to meet the scale-free network. Next, the co-expression network was constructed, and the prognostic module was identified. The minimum number of genes in the module was 30. Modules with correlation coefficients greater than 0.75 were merged. Then, we calculated the Pearson correlation between the genes in the module and the module feature genes. Hub genes were defined as |cor| >0.8, P<0.001. A risk score was calculated based on the multivariate regression of these hub genes.
Statistical analysis
GraphPad Prism 9.0 and SPSS 23.0 were applied for statistical analysis. Student’s t-test was utilized to analyse differences between two groups, and one-way analysis of variance (ANOVA) was used for multi-group comparisons. The differences in proportion were determined by the χ2 test and Fisher’s exact test. Correlations were evaluated by Pearson correlation analysis. Survival was analysed by Kaplan-Meier method, and significance was evaluated with the log-rank test. A P value of <0.05 was considered statistically significant for all tests.
Results
Identification of deregulated alternative splicing events in PAAD
Among the seven alternative splicing events in TCGA-PAAD, the frequency and number of genes involved in exon skipping were the highest, whereas the event of mutually exclusive exons had the lowest frequency (Figure 1A-1C). Using the Wilcox test, we detected 1,144 upregulated alternative splicing events and 873 downregulated alternative splicing events in the tumors compared with adjacent pancreas samples (Figure S1A). The top 100 differential alternative splicing events are shown in a heatmap in Figure S1B, and the list of detailed information on the alternative splicing events is provided in available online: https://cdn.amegroups.cn/static/public/jgo-24-340-1.xlsx.
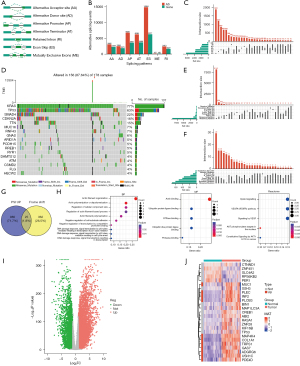
Exploration of the mutation landscape in PAAD
We displayed genes with the highest mutation frequencies in Figure 1D. Nonetheless, only five genes showed a mutation percentage over 10%, supporting the low mutation burden of PAAD. The frequency of missense mutations was much higher than that of other types of point mutations, followed by nonsense mutations (Figure 1E). Multiple types of mutations may often occur in the same individual at the same time, with missense mutations, nonsense mutations, and deletions having the highest probability of frameshift mutation (Figure 1F). Then, we visualized the constitution of mutation types for the top 10 genes with the highest mutation percentage (Figure S1C). In addition, we analyzed the proportion of each specified type of gene variation in all samples, and KRAS missense mutation was still the most predominant (Figure S1D).
Identification of candidate tumor antigens for PAAD
By intersecting genes with upregulated alternative splicing events and genes with frameshift mutations, we detected 25 potential tumor antigen genes (Figure 1G). Both biological process (BP) and molecular function (MF) analyses of GO enrichment indicated that these genes are involved in actin binding and structural interactions. Reactome analysis showed that these genes are associated with VEGF/VEGFR signaling and the AKT pathway (Figure 1H).
Differential expression analysis screening of upregulated tumor antigens
To explore whether these candidate tumor antigens are indeed upregulated in PAAD, we performed a differential expression analysis for all transcripts between tumor and normal samples. Given that the TCGA dataset includes few normal samples, we incorporated the transcriptome information of the normal pancreas in the GTEx dataset into our analysis and detected 6,268 upregulated genes in PAAD, of which 11 encode the candidate tumor antigens mentioned above (Figure 1I,1J and available online: https://cdn.amegroups.cn/static/public/jgo-24-340-2.xlsx).
Nonsense-mediated RNA decay (NMD) is associated with candidate tumor antigens and genes involved in alternative splicing events
NMD is referred to as an RNA surveillance pathway that targets aberrant mRNAs with premature termination codons (PTCs) for degradation, and understanding the relationship between NMD and candidate tumor antigen expression is important. We divided the TCGA-PAAD datasets into two subgroups based on the median expression levels of five NMD mediators, including UPF1, SMG5, SMG7, SMG9, and DHX34 (29). Then, we compared the mRNA expression of the candidate tumor antigens between the two subgroups. The results showed different expression patterns associated with NMD regulators for nine candidate tumor antigens. Overall, INF2, PLCB3, PLEC, RPS6KB2, SLC4A2, and SSH3 were overexpressed in groups with high expression of all NMD mediators. In contrast, TRPS1 and GAS7 were not expressed in PAAD samples with high expression of NMD mediators (Figure S1E). We also investigated the relationship between genes involved in alternative splicing events and NMD mediators based on splicing data. In addition to the genes encoding candidate tumor antigens mentioned above, we observed that ADGRG6, BIN1, COL1A1, CTNND1, KIF16B, MAP4K4, MUC1, PDE4D, and ZNF28 were deregulated in PAAD samples with higher or lower expression of NMD regulators (Figure S2A-S2E).
Prognostic implications of candidate tumor antigens
Next, we explored the relationship between genes for candidate tumor antigens and patient OS and DFS. Through performing Cox regression analysis, we detected 14 OS-associated genes and 10 DFS-associated genes. Among them, nine genes were simultaneously associated with both OS and DFS, which showed a significant association with survival (Figure 2A). Using the median expression level as the cutoff value, Kaplan-Meier curves for these genes revealed ADGRG6, MAP1LC3A, and TRPS1 to be the most significant survival-related genes (Figure 2B).

The relationship between candidate tumor antigens and infiltrates of antigen-presenting cells (APCs)
APCs play an important role in presenting tumor antigens to adaptive immune cells, inducing immune memory and antitumor activities. Using the TIMER algorithm, we calculated the percentage of infiltrating APCs within PAAD and correlated them with the expression of the candidate tumor antigens. The results showed that PLEC, COL1A1, and MAP4K4 were positively associated with macrophage M0 infiltration, but ADGRG6 and PLEC correlated negatively with B-naïve infiltration, which suggests that these antigens may be preferentially presented by M0 macrophages in TCGA-PAAD (Figure 2C). According to our results, PLEC is upregulated in PAAD and correlates highly with NMD mediators; it also appears to be associated with poor prognosis and low recruitment of APCs. Hence, PLEC is the most promising candidate tumor antigen in PAAD (Figure 2D). Using the TCIA database, we confirmed that many peptides of PLEC may serve as neoantigens that can be recognized by the immune system (available online: https://cdn.amegroups.cn/static/public/jgo-24-340-3.xls).
Identification of immune subtypes for PAAD
Although PAAD is widely recognized as a “cold” tumor, we attempted to perform unsupervised clustering for PAAD samples based on immunity-related genes (available online: https://cdn.amegroups.cn/static/public/jgo-24-340-4.xls). First, we conducted Cox regression for genes involved in immune activities, screening out 349 prognosis-relevant immune genes. Based on the expression levels of these genes, we identified two independent subclusters for TCGA-PAAD (C1 and C2) (Figure 3A-3E), which exhibited a significant difference in OS (Figure 3F). To explore whether such subtyping may be applied to other PAAD cohorts, we performed clustering for the PAAD samples in a GEO dataset (GSE62452) based on the same procedures (Figure 3G). Notably, the subtyping revealed a more stringent trend in terms of OS between C1 and C2, suggesting that such subtyping has predictive value for the prognostic evaluation of PAAD. Next, we compared the percentages of clinical parameters, including stage, pancreatitis, diabetes, tumor, node, metastasis (TNM) stage, and grade, between the C1 and C2 subgroups and observed a mild enrichment of PAAD patients with a history of pancreatitis (P=0.006) in the C1 subgroup and that C1 featured overall higher grade (P<0.001) (Figure 3H).
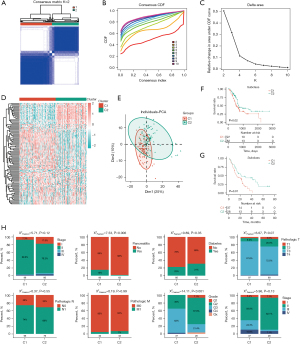
The mutation and CNV characteristics of the C1 and C2 immune subtypes
We then sought to determine whether different immune subtypes of PAAD have distinct genetic landscapes. Interestingly, the C1 subtype, with worse prognosis, showed a higher TMB and numerous mutated genes (Figure 4A,4B), and we drew a waterfall plot to show the differences in mutated genes and their types between the C1 and C2 samples (Figure 4C,4D). Globally, more mutations in driver genes were found in the C1 subcluster, including KRAS, TP53, SMAD4, and CDKN2A. Notably, for C2 samples, the mutation frequency of TTN surpassed that of SMAD4 and CDKN2A, becoming the third gene with a high mutation frequency. Fifteen percent of PAAD samples harbor TTN mutations, of which most are missense. We further assessed CNV peaks across chromosomes in TCGA-PAAD, of which integrated analysis showed that the C1 group had higher CNV frequencies (Figure 4E,4F). We also calculated the PSI for candidate tumor antigens according to the spliced-seq data and compared the PSI of these genes between the C1 and C2 subgroups using the Wilcox test. A total of 18 genes with deregulated PSI were identified, with the PSI of ADGRG6, COL1A1, GAS7, INF2, PLCB3, RASA1, RPS6KB2 and SLC4A2 being significantly increased in the C1 subgroup (Figure 4G).
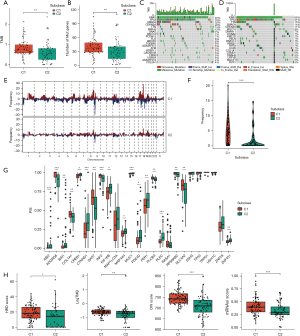
Assessment of homologous recombination deficiency (HRD), TMB, chromosome instability and the stemness index between C1 and C2 PAAD subclusters
We calculated HRD, TMB, chromosome instability and the stemness index for each PAAD sample and found these biomarkers to be tightly associated with the malignant behavior and therapeutic strategies for PAAD. The results showed that all four indexes were higher in the C1 subcluster, suggesting that the C1 samples have more obvious genome disturbance (Figure 4H).
Evaluation of immune activities between C1 and C2 PAAD subclusters
The Tracking Tumor Immunophenotype (TIP) algorithm allows researchers to evaluate the stage of immune response of tumor samples. We found that many immune procedures differed between the C1 and C2 subgroups. For example, recruitment of T cells, dendritic cells, macrophages and Th17 cells was significantly increased in C2 subgroup but that of basophils and eosinophils decreased (Figure 5A). Overall immune activity was stringently elevated in the C2 group.
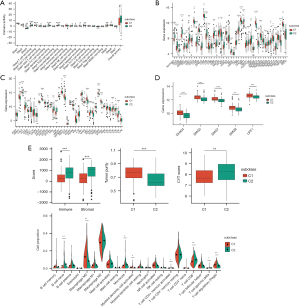
Comparison of expression levels of ICP genes, mediators of immunogenic cell death (ICD) and NMD factors between the C1 and C2 PAAD subclusters
ICP inhibitors have been widely applied in clinical practice for many cancers. In addition, our previous studies summarized the role of ICD in antitumor immunity (5,6). We acquired a gene list consisting of 47 ICP genes and 43 ICD genes from published literature (30-34) and compared the expression levels of these factors between the C1 and C2 groups in both the TCGA and GEO cohorts. A total of 35 differentially expressed ICP, 23 ICD and 5 NMD factors (Figure 5B-5D) were identified in the TCGA cohort, while 18 ICP, 17 ICD and 3 NMD factors were identified in the GSE62452 dataset (Figure S3A-S3C).
Immune subtypes feature different patterns of immune cell infiltration
Infiltrating immune cells play an important role in antitumor procedures, influencing patient prognosis. Hence, we evaluated the differences between immune subtypes. The C2 subtype had fewer tumor components and was enriched with more immune and stromal components (Figure 5E). Notably, the C2 subtype had higher cytotoxic scores than its counterparts, which suggests that the C2 subtype exhibits more sophisticated antitumor activity in the immune microenvironment (Figure S3D). Supportively, we found more CD8+ T cell infiltration in the C2 subtype, whereas enrichment of Treg cells, which are recognized as immune inhibitory cells, was increased in the C1 subtype (Figure S3D).
Immune subtypes are associated with multiple biomarkers in PAAD
Previous studies have reported multiple diagnostic and prognostic biomarkers for PAAD, including VHL, CDKN2A, KRAS, SMAD4, TP53, GNAS, RNF43, PIK3CA, and PTEN, and we compared the expression levels of these biomarkers between the C1 and C2 subtypes (35,36). In the TCGA dataset, GNAS, PTEN, and SMAD4 were significantly upregulated in the C2 subtype, but higher expression of KRAS and RNF43 was observed in the C1 subtype. Thorsson et al. reported immune subtypes at the pan-cancer level (37), and by using their classification method, this study analyzed the percentage of their six subtypes in the classifier generated. The results showed more immunoC3 and fewer immunoC1 subtypes in the C2 subgroup (Figure 6A), of which immunoC1 subtypes displayed a high proliferation rate, while ImmunoC3 displayed an activation of type I immune response and had the most favorable prognosis.
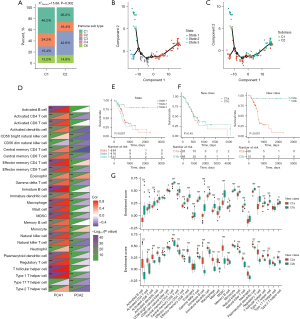
Characterization of the immune landscape for PAAD
According to the definition of 28 immune cell clusters in a previous study (26), we performed ssGSEA to quantify enrichment of immune cells in TCGA-PAAD. We conducted dimensionality reduction using DDRTree, followed by pseudotime analysis (Figure 6B,6C). Then, we calculated the correlation between principal components and 28 immune cells, with PCA1 correlating positively with activated B cells, immature B cells, and type 1 T helper cells and PCA2 correlating negatively with central memory CD4 T cells, activated dendritic cells, and natural killer T cells (Figure 6D). Principal component analysis (PCA) identified three cell states, of which PAADs in cellstate2 showed prolonged survival time (Figure 6E). Based on the same principal components, the C1 and C2 subtypes that we identified were further divided into two subtypes each. Interestingly, C2b patients showed a significantly prolonged survival time compared to C2a patients, with no differences between C1a and C2a in terms of survival time. Next, we studied the differential distribution of immune cells between the two subtypes for both C1 and C2. Although the prognoses for C1a and C1b were similar, more immune cells were infiltrated in the C1b subtype. In addition, the C2b subtype showed a prolonged survival rate compared with the C2a subtype. Nonetheless, the C2a subtype showed superior enrichment of pan-immune cells, suggesting that an overactivated inflammatory microenvironment may not predict better survival in “noncold” PAAD (Figure 6F,6G). Hence, we further investigated whether immune gene modules are able to characterize PAAD prognosis.
Identification of immune gene modules associated with the prognosis of PAAD
A co-expression network was constructed based on WGCNA using the transcriptome of TCGA-PAAD samples (Figure 7A-7C). The modules including black, brown, red, and turquoise showed higher strength in the C2 subgroup, while genes in the blue and yellow modules had lower expression levels in the C1 subgroup. The number of specific genes in the turquoise module surpassed 400, and the yellow module had the least number of specific genes (Figure 7D,7E). Next, we performed Cox regression for the eigengenes in each module to screen for prognosis-relevant modules (Figure 7F). The results showed that both the blue and green modules are associated with poor prognosis in PAAD. Based on GO analysis, the genes in the blue module are mostly relevant to cytokine-mediated signaling pathways; those in the green module are associated with positive regulation of the MAPK cascade (Figure 7G and available online: https://cdn.amegroups.cn/static/public/jgo-24-340-5.xlsx; https://cdn.amegroups.cn/static/public/jgo-24-340-6.xlsx). Next, we screened hub genes of the modules with prognostic value and constructed a predictive tool to classify TCGA-PAAD samples with different prognoses (Figure 7H,7I). According to the ROC curve analysis, the area under the curve (AUC) value of the generated predictive tool for prognosis prediction reached 0.81, suggesting that the predictive tool constructed based on hub genes of each module has high accuracy (Figure 7J).
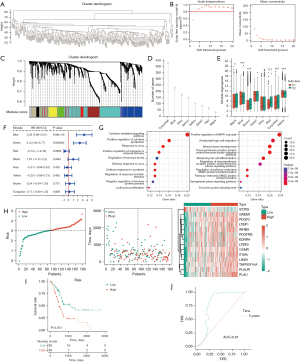
Single-cell analysis reveals the relationship between risk scores and trajectory in tumor cells
After a series of quality controls, we acquired an expression matrix of 7,530 cells from single-cell dataset GSE154778 samples (Figure 8A,8B). Then, we extracted tumor cells from these cells and labeled risk scores based on the model described above (Figure 8C). Pseudotime trajectory analysis showed that the four subclusters of tumor cells may have stringent evolutionary differences (Figure 8D). Moreover, tumor cells with lower risk scores displayed an evolutionary tendency towards tumor cells with higher risk scores (Figure 8D). We showed similar results by using t-distributed stochastic neighbor embedding (t-SNE) clustering methods (Figure 8E,8F). We divided tumor cells into high- and low-risk types according to the risk score and compared their differences in terms of cell-cell communication. Tumor cells with lower risk scores are more likely to communicate with macrophages through MIF signaling, and those with higher risk scores tend to communicate with monocytes, T cells and natural killer (NK) cells via MDK, SPP1 and GALECTIN signaling (Figure 8G).
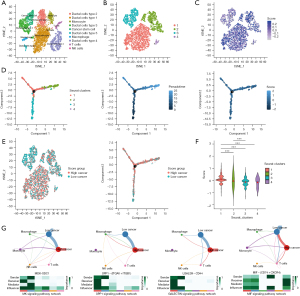
Discussion
Although immunotherapy has become a promising treatment modality for multiple cancers, it has failed to achieve satisfactory efficacy in pancreatic cancer. Many factors undermine the response of pancreatic cancer cells to immunotherapy, including a fibrotic microenvironment, low immune cell infiltration, and low immunogenicity. Low TMB has been seen as an essential element to explain why pancreatic cancer cells barely show immune induction and can easily evade immune surveillance. Unlike other solid tumors, pancreatic cancer does not have common drug targets. Most genome mutations of pancreatic cancer cannot be targeted due to undruggable molecular structures, such as KRAS G12D. Hence, finding genomic targets beyond the driver genes of pancreatic cancer is a promising way to guide immunotherapy for PAAD.
The present study systematically explored aberrantly upregulated genes, alternatively spliced genes, and genes associated with NMD factors, antigen presentation, and OS in TCGA-PAAD, revealing that PLEC is a promising neoantigen for PAAD-targeted therapy. Previous studies have shown that PAAD patients with long-term survival have specific neoantigen profiles (38,39). Evolution analysis revealed that reduced levels of high-quality neoantigens are characteristic of recurrent disease in PAAD patients with long survival, suggesting that the interaction between neoantigens and the immune system plays an important role in prognosis. Huang et al. and Rojas et al. recently described potential neoantigen and mRNA vaccine candidates in PAAD and cholangiocarcinoma based on public sequencing data (30,40). However, they did not take alternative splicing and NMD factors into consideration when screening translational targets. Wang et al. reported six prognostic splicing biomarker, including UBA1, S100A13, SH3KBP1, COPSTA, GSE1 and NISCH. The risk model based on the six genes with alternative splicing events showed broad association with the fractions of macrophages M1, resting mast cells, CD8 T cells, T cells regulatory, naive B cells and memory B cells (41). In cancer, alternative splicing is often dysregulated, leading to the production of novel protein isoforms that can serve as tumor-specific antigens. Several studies have highlighted the role of alternative splicing-derived neoantigens in eliciting immune responses against tumors. For instance, alternative splicing can create novel peptides that are presented on the surface of tumor cells by major histocompatibility complex (MHC) molecules, making them recognizable by T cells (42,43). These suggested alternative splicing could be a vital factor for immune microenvironment, which should be taken into considered in analysis. Here, we report that PLEC is a promising target with therapeutic value for PAAD. Although some published studies have recognized PLEC as a biomarker for tumorigenesis of PAAD, few studies have addressed its immunogenicity. As an immunologically “cold” tumor, PAAD shows poor immunogenicity and a low response to immunotherapy. Overall, mRNA vaccines based on neoantigens derived from PLEC may become a promising strategy to boost the immunogenicity of PAAD.
Notably, we describe a novel immune subtyping system for PAAD to indicate patient prognosis and present opportunities for immunotherapy, such as ICP inhibitors. TCGA-PAAD classified as the C2 subtype showed better prognosis and more CD8+ T-cell infiltration. In addition, mutational profiles, immune infiltrations, NMD factor expression levels and genomic parameters were distinct between the two immune subgroups. By using single-cell sequencing-based trajectory analysis, we further classified two minor subgroups each from the C1 and C2 subtypes, of which the C2b subtype had the best prognosis. We also identified prognosis-related immune modules through WGCNA, whereby blue and green modules were associated with the shortest OS duration. Enrichment analysis showed the hub genes in the blue module to be associated with cytokine-mediated signaling pathways and those in the green module to be associated with positive regulation of the MAPK cascade. Many studies have established the complicated role of cytokines in the immune microenvironment of cancers (44,45). Recently, an increasing number of studies have uncovered that MAPK activation fuels immune evasion during tumorigenesis. In contrast, the combination of MAPK inhibition and immunotherapy contributes to synergistically increased efficacy (46,47). Our results reinforce the combinational value of applying MAPK inhibition and ICP inhibitors. Moreover, based on the hub genes for each immune module, we constructed a prognostic model to predict the OS of patients with PAAD, which showed a high accuracy for the 5-year survival rate. The greatest advantage of our prognosis model is its applicability for researchers aiming to investigate the immunogenicity of PAAD samples. The biggest challenge we currently face is the lack of clinical validation, which we plan to address in future studies. Although the accuracy of the generated model is not the best in the literature, it is applicable in cases in which researchers aim to investigate the immunogenicity of PAAD samples.
Conclusions
In conclusion, the present study used a transcriptome-guided approach to screen out neoantigen candidates based on alternative splicing, NMD factors and antigen-presenting signatures. The prognostic model established in the present study can predict patient prognosis and the immune microenvironment and indicate the selection of patients for clinical trials of immunotherapy.
Acknowledgments
Funding: This study was jointly supported by
Footnote
Reporting Checklist: The authors have completed the MDAR reporting checklist. Available at https://jgo.amegroups.com/article/view/10.21037/jgo-24-340/rc
Peer Review File: Available at https://jgo.amegroups.com/article/view/10.21037/jgo-24-340/prf
Conflicts of Interest: All authors have completed the ICMJE uniform disclosure form (available at https://jgo.amegroups.com/article/view/10.21037/jgo-24-340/coif). The authors have no conflicts of interest to declare.
Ethical Statement: The authors are accountable for all aspects of the work in ensuring that questions related to the accuracy or integrity of any part of the work are appropriately investigated and resolved. The study was conducted in accordance with the Declaration of Helsinki (as revised in 2013).
Open Access Statement: This is an Open Access article distributed in accordance with the Creative Commons Attribution-NonCommercial-NoDerivs 4.0 International License (CC BY-NC-ND 4.0), which permits the non-commercial replication and distribution of the article with the strict proviso that no changes or edits are made and the original work is properly cited (including links to both the formal publication through the relevant DOI and the license). See: https://creativecommons.org/licenses/by-nc-nd/4.0/.
References
- Siegel RL, Miller KD, Wagle NS, et al. Cancer statistics, 2023. CA Cancer J Clin 2023;73:17-48. [Crossref] [PubMed]
- Ilic M, Ilic I. Epidemiology of pancreatic cancer. World J Gastroenterol 2016;22:9694-705. [Crossref] [PubMed]
- Laface C, Memeo R, Maselli FM, et al. Immunotherapy and Pancreatic Cancer: A Lost Challenge? Life (Basel) 2023;13:1482. [Crossref] [PubMed]
- Falcomatà C, Bärthel S, Schneider G, et al. Context-Specific Determinants of the Immunosuppressive Tumor Microenvironment in Pancreatic Cancer. Cancer Discov 2023;13:278-97. [Crossref] [PubMed]
- Tang R, Xu J, Zhang B, et al. Ferroptosis, necroptosis, and pyroptosis in anticancer immunity. J Hematol Oncol 2020;13:110. [Crossref] [PubMed]
- Tong X, Tang R, Xiao M, et al. Targeting cell death pathways for cancer therapy: recent developments in necroptosis, pyroptosis, ferroptosis, and cuproptosis research. J Hematol Oncol 2022;15:174. [Crossref] [PubMed]
- Awad MM, Govindan R, Balogh KN, et al. Personalized neoantigen vaccine NEO-PV-01 with chemotherapy and anti-PD-1 as first-line treatment for non-squamous non-small cell lung cancer. Cancer Cell 2022;40:1010-1026.e11. [Crossref] [PubMed]
- Xie N, Shen G, Gao W, et al. Neoantigens: promising targets for cancer therapy. Signal Transduct Target Ther 2023;8:9. [Crossref] [PubMed]
- Chan TA, Yarchoan M, Jaffee E, et al. Development of tumor mutation burden as an immunotherapy biomarker: utility for the oncology clinic. Ann Oncol 2019;30:44-56. [Crossref] [PubMed]
- Palmeri M, Mehnert J, Silk AW, et al. Real-world application of tumor mutational burden-high (TMB-high) and microsatellite instability (MSI) confirms their utility as immunotherapy biomarkers. ESMO Open 2022;7:100336. [Crossref] [PubMed]
- Luo J. KRAS mutation in pancreatic cancer. Semin Oncol 2021;48:10-8. [Crossref] [PubMed]
- Mizukami K, Iwasaki Y, Kawakami E, et al. Genetic characterization of pancreatic cancer patients and prediction of carrier status of germline pathogenic variants in cancer-predisposing genes. EBioMedicine 2020;60:103033. [Crossref] [PubMed]
- Bailey P, Chang DK, Nones K, et al. Genomic analyses identify molecular subtypes of pancreatic cancer. Nature 2016;531:47-52. [Crossref] [PubMed]
- Grünwald BT, Devisme A, Andrieux G, et al. Spatially confined sub-tumor microenvironments in pancreatic cancer. Cell 2021;184:5577-5592.e18. [Crossref] [PubMed]
- Choi S, Cho N, Kim KK. The implications of alternative pre-mRNA splicing in cell signal transduction. Exp Mol Med 2023;55:755-66. [Crossref] [PubMed]
- Lee Y, Rio DC. Mechanisms and Regulation of Alternative Pre-mRNA Splicing. Annu Rev Biochem 2015;84:291-323. [Crossref] [PubMed]
- Wang Z, Zhu L, Li K, et al. Alternative splicing events in tumor immune infiltration in renal clear cell carcinomas. Cancer Gene Ther 2022;29:1418-28. [Crossref] [PubMed]
- Shi JY, Bi YY, Yu BF, et al. Alternative Splicing Events in Tumor Immune Infiltration in Colorectal Cancer. Front Oncol 2021;11:583547. [Crossref] [PubMed]
- Lai J, Yang H, Xu T. Systemic characterization of alternative splicing related to prognosis and immune infiltration in malignant mesothelioma. BMC Cancer 2021;21:848. [Crossref] [PubMed]
- Huang T, Ye W, Lin X. Alternative Splicing Events in Immune Infiltration of Lung Adenocarcinoma. Med Sci Monit 2021;27:e934491. [Crossref] [PubMed]
- Zhang D, Zou D, Deng Y, et al. Systematic analysis of the relationship between ovarian cancer prognosis and alternative splicing. J Ovarian Res 2021;14:120. [Crossref] [PubMed]
- Wu ZH, Tang Y, Zhou Y. Alternative splicing events implicated in carcinogenesis and prognosis of thyroid gland cancer. Sci Rep 2021;11:4841. [Crossref] [PubMed]
- Wu Z, Chen H, Liang Y, et al. Alternative splicing implicated in immunity and prognosis of colon adenocarcinoma. Int Immunopharmacol 2020;89:107075. [Crossref] [PubMed]
- Ma S, Zhu J, Wang M, et al. A comprehensive characterization of alternative splicing events related to prognosis and the tumor microenvironment in lung adenocarcinoma. Ann Transl Med 2022;10:479. [Crossref] [PubMed]
- Rooney MS, Shukla SA, Wu CJ, et al. Molecular and genetic properties of tumors associated with local immune cytolytic activity. Cell 2015;160:48-61. [Crossref] [PubMed]
- Charoentong P, Finotello F, Angelova M, et al. Pan-cancer Immunogenomic Analyses Reveal Genotype-Immunophenotype Relationships and Predictors of Response to Checkpoint Blockade. Cell Rep 2017;18:248-62. [Crossref] [PubMed]
- Carter SL, Eklund AC, Kohane IS, et al. A signature of chromosomal instability inferred from gene expression profiles predicts clinical outcome in multiple human cancers. Nat Genet 2006;38:1043-8. [Crossref] [PubMed]
- Malta TM, Sokolov A, Gentles AJ, et al. Machine Learning Identifies Stemness Features Associated with Oncogenic Dedifferentiation. Cell 2018;173:338-354.e15. [Crossref] [PubMed]
- Oka M, Xu L, Suzuki T, et al. Aberrant splicing isoforms detected by full-length transcriptome sequencing as transcripts of potential neoantigens in non-small cell lung cancer. Genome Biol 2021;22:9. [Crossref] [PubMed]
- Huang X, Tang T, Zhang G, et al. Identification of tumor antigens and immune subtypes of cholangiocarcinoma for mRNA vaccine development. Mol Cancer 2021;20:50. [Crossref] [PubMed]
- Huang X, Zhang G, Tang T, et al. Identification of tumor antigens and immune subtypes of pancreatic adenocarcinoma for mRNA vaccine development. Mol Cancer 2021;20:44. [Crossref] [PubMed]
- Galluzzi L, Vitale I, Warren S, et al. Consensus guidelines for the definition, detection and interpretation of immunogenic cell death. J Immunother Cancer 2020;8:e000337. [Crossref] [PubMed]
- Fucikova J, Kepp O, Kasikova L, et al. Detection of immunogenic cell death and its relevance for cancer therapy. Cell Death Dis 2020;11:1013. [Crossref] [PubMed]
- Galluzzi L, Buqué A, Kepp O, et al. Immunogenic cell death in cancer and infectious disease. Nat Rev Immunol 2017;17:97-111. [Crossref] [PubMed]
- Neoptolemos JP, Kleeff J, Michl P, et al. Therapeutic developments in pancreatic cancer: current and future perspectives. Nat Rev Gastroenterol Hepatol 2018;15:333-48. [Crossref] [PubMed]
- Hayashi A, Hong J, Iacobuzio-Donahue CA. The pancreatic cancer genome revisited. Nat Rev Gastroenterol Hepatol 2021;18:469-81. [Crossref] [PubMed]
- Thorsson V, Gibbs DL, Brown SD, et al. The Immune Landscape of Cancer. Immunity 2018;48:812-830.e14. [Crossref] [PubMed]
- Balachandran VP, Łuksza M, Zhao JN, et al. Identification of unique neoantigen qualities in long-term survivors of pancreatic cancer. Nature 2017;551:512-6. [Crossref] [PubMed]
- Levink IJM, Brosens LAA, Rensen SS, et al. Neoantigen Quantity and Quality in Relation to Pancreatic Cancer Survival. Front Med (Lausanne) 2022;8:751110. [Crossref] [PubMed]
- Rojas LA, Sethna Z, Soares KC, et al. Personalized RNA neoantigen vaccines stimulate T cells in pancreatic cancer. Nature 2023;618:144-50. [Crossref] [PubMed]
- Wang L, Bi J, Li X, et al. Prognostic alternative splicing signature reveals the landscape of immune infiltration in Pancreatic Cancer. J Cancer 2020;11:6530-44. [Crossref] [PubMed]
- Burbage M, Rocañín-Arjó A, Baudon B, et al. Epigenetically controlled tumor antigens derived from splice junctions between exons and transposable elements. Sci Immunol 2023;8:eabm6360. [Crossref] [PubMed]
- Brochu H, Wang R, Tollison T, et al. Alternative splicing and genetic variation of mhc-e: implications for rhesus cytomegalovirus-based vaccines. Commun Biol 2022;5:1387. [Crossref] [PubMed]
- Bill R, Wirapati P, Messemaker M, et al. CXCL9:SPP1 macrophage polarity identifies a network of cellular programs that control human cancers. Science 2023;381:515-24. [Crossref] [PubMed]
- Goenka A, Khan F, Verma B, et al. Tumor microenvironment signaling and therapeutics in cancer progression. Cancer Commun (Lond) 2023;43:525-61. [Crossref] [PubMed]
- Haas L, Elewaut A, Gerard CL, et al. Acquired resistance to anti-MAPK targeted therapy confers an immune-evasive tumor microenvironment and cross-resistance to immunotherapy in melanoma. Nat Cancer 2021;2:693-708. [Crossref] [PubMed]
- Ma X, Xiao L, Liu L, et al. CD36-mediated ferroptosis dampens intratumoral CD8(+) T cell effector function and impairs their antitumor ability. Cell Metab 2021;33:1001-1012.e5. [Crossref] [PubMed]
(English Language Editor: J.Y. Yeo)