Hepatoid adenocarcinoma of the stomach: discrimination from conventional gastric adenocarcinoma with a computed tomography-based radiomics nomogram
Highlight box
Key findings
• Distinguishing hepatoid adenocarcinoma of the stomach (HAS) from conventional gastric adenocarcinoma (CGA) has relied on the serum alpha-fetoprotein (AFP) level and radiomics signature. The nomogram, comprised of the radiomics signature and the serum AFP level, yielded excellent accuracy for identifying HAS.
What is known and what is new?
• Radiomics employs an image analysis method to extract high-dimensional features for quantitative analysis, which can facilitate preoperative diagnosis and establishment of treatment strategies.
• The computed tomography (CT)-based radiomics nomogram, integrating clinical parameters and a radiomics signature, provides a valuable noninvasive approach to enhance the detection rate of HAS prior to surgery.
What is the implication, and what should change now?
• Patients with gastric adenocarcinoma may benefit from an additional diagnostic option offered by this CT-based radiomics nomogram. The pathological difference between HAS and CGA leading to customized treatment cannot be ignored.
Introduction
Hepatoid adenocarcinoma mainly occurs in the stomach, and is known for high malignancy and poor prognosis (1,2). Hepatoid adenocarcinoma of the stomach (HAS) is a rare subtype of gastric adenocarcinoma, exhibiting an approximate annual incidence of 0.58–0.83 cases per million individuals (3,4). Gastric adenocarcinoma mainly contains the conventional subtypes, such as tubular and papillary adenocarcinoma, and mucinous adenocarcinoma (5). The clinical manifestations and imaging features of HAS and conventional gastric adenocarcinoma (CGA) are similar. However, the incidence of vascular and neural invasion, and lymph node (LN) and liver metastases is higher in HAS compared to CGA (6,7). High serum alpha-fetoprotein (AFP) level and advanced clinical tumor, node, metastasis (TNM) stage (particularly N stage) are characteristic manifestations of HAS (1). The treatment of HAS is predominantly interventional therapy, neoadjuvant therapy, and other radical resection procedures (8,9). The preoperative identification of HAS from CGA holds significant clinical importance.
The diagnostic criteria for HAS rely on the presence of a hepatocellular differentiation area in primary gastric cancer (GC) after excision, regardless of serum AFP level (10,11). The limited number of biopsy specimens obtained from gastroscopy may result in histological findings inconsistent with those from surgical resection. Imaging findings from computed tomography (CT), magnetic resonance imaging (MRI), and positron emission tomography/CT (PET/CT) are crucial for diagnosing and treating GC (12,13). CT is the most frequently employed medical imaging modality for GC diagnosis (14). CT findings of HAS and CGA are similar. Misdiagnosis of HAS as CGA leaves the patient susceptible to imprecise treatment, recurrence, and metastasis.
Radiomics employs an image analysis method to extract high-dimensional features for quantitative analysis (15). For conventional GC, Nie et al. developed a radiomics nomogram by integrating CT images and clinical features to preoperatively differentiate Lauren’s diffuse-type GC from intestinal-type (16). Zhao et al. developed and validated a conventional CT-based radiomics model to address the limitation of gastroscopy biopsy in fully reflecting human epidermal growth factor receptor 2 (HER-2) status of GC, enabling accurate identification of HER-2 positive status in GC (17). For special types of GC, Wang et al. developed a nomogram that incorporated the radiomic signature based on the portal phase, tumor margin, and LN metastasis for distinguishing gastric neuroendocrine carcinoma from gastric adenocarcinoma, showing significant clinical implications (18). Chen et al. demonstrated that a radiomics nomogram exhibited remarkable potential in distinguishing between diffuse-type and signet ring cell GC (19).
CT-based radiomics analysis is hypothesized to be a valuable approach for augmenting the detection rate of HAS. Therefore, it is imperative to develop a reliable CT-based radiomics prediction model that encompasses both clinical predictors and radiomics signatures for the identification of HAS. The aim of this study was to establish a valuable noninvasive method for identifying HAS from CGA dependent on the construction of a radiomics nomogram prior to surgery. We present this article in accordance with the TRIPOD reporting checklist (available at https://jgo.amegroups.com/article/view/10.21037/jgo-24-210/rc).
Methods
Patients
The study was conducted in accordance with the Declaration of Helsinki (as revised in 2013). The study was approved by the Institutional Review Board of Affiliated Hospital of Nanjing University of Chinese Medicine (No. 2024NL-123-02) and the requirement for individual consent for this retrospective analysis was waived. We searched for patients with pathologically confirmed HAS from the Affiliated Hospital of Nanjing University of Chinese Medicine from March 2012 to January 2023. The eligibility criteria for HAS were delineated as follows: (I) surgical pathology diagnosis of HAS; (II) no history of other tumors; (III) unenhanced and multiphase enhanced CT conducted one week before surgery; (IV) without neoadjuvant chemotherapy or radiotherapy. The exclusion criteria for HAS were as follows: (I) stomach with insufficient distension; (II) pathological biopsy before CT examination; (III) diameter of the tumor <5 mm; (IV) poor quality of CT images. Patients with CGA were systemically selected to ensure a match between HAS and CGA based on age, gender, and clinical T stage. The patients were randomly allocated in a 7:3 ratio. A total of 59 patients with HAS and 122 patients with CGA enrolled in this study were divided into the training and test cohorts, with 126 patients in the training cohort and 55 patients in the test cohort. The inclusion and exclusion criteria for HAS are outlined in Figure 1.
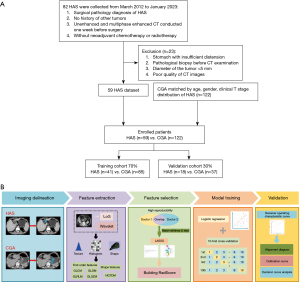
The measurement of serum biochemical biomarkers included assessing the levels of serum AFP (0–19 ng/mL: normal; ≥20 ng/mL: above normal), carcinoembryonic antigen (CEA) (0–4 ng/mL: normal; ≥5 ng/mL: above normal), carbohydrate antigen 19-9 (CA19-9) (0–36 ng/mL: normal; ≥37 ng/mL: above normal), and cancer antigen 125 (CA125) (0–34 ng/mL: normal; ≥35 ng/mL: above normal) (20).
CT image acquisition
All patients underwent both unenhanced and multiphase contrast-enhanced CT examinations. Each patient was required to fast for a duration exceeding five hours and the stomach was distended by the patient drinking 600–1,000 mL warm water before CT scanning. CT examinations were performed on two CT scanners (Light Speed Volume CT, GE Healthcare, Chicago, IL, USA; Brilliance 64, Philips Medical Systems, Amsterdam, Netherlands). The primary scanning parameters of the two CT scanners were as follows: a tube voltage of 120 kV, rotation time ranging from 0.4 to 0.5 seconds, and a tube current of 250–640 mA. Reconstruction was accomplished utilizing 1.25- and 5-mm thicknesses. In the subsequent procedure, iopromide (300 mg/mL, Schering, Berlin, Germany) was administered intravenously via an antecubital vein with the velocity of 3.0–3.5 mL/s through an 18-gauge catheter accompanied by 40 mL of normal saline solution following the unenhanced CT scan. The contrast-enhanced CT images were acquired separately during the arterial phase, portal phase, and delayed phase at 30, 60, and 120 seconds post-contrast medium injection.
CT features evaluation
CT features were independently analyzed with blind evaluation by two gastrointestinal radiologists with 7 and 12 respective years of interpretation experience in gastrointestinal radiology. Discrepancies between the two radiologists were resolved via joint consultation. The quantitative CT features were derived from the average values ascertained by both radiologists.
The tumor locations were categorized into two regions: the antrum and the cardia, fundus, or body of the stomach. The maximum diameter was measured at the position of greatest cross-section of the lesion. The CT attenuation values were measured three times in 10 mm regions of interest (ROIs) on each phase, and the average value was calculated. To mitigate partial volume effects, the center of the mass was determined as the ROI. We categorized the enhancement as homogeneous or heterogeneous and defined growth patterns as elevated, ulcerative, or infiltrative types. Clinical T stage was determined based on the eighth edition of American Joint Committee on Cancer (AJCC) staging system (21). Table S1 shows the pathological manifestations and CT features of clinical T stage (22).
Segmentation and feature extraction
We used portal phase CT images in Digital Imaging and Communications in Medicine (DICOM) format for subsequent analysis (Figure 1). The ROI was delineated using 3D-Slicer application (Version 4.11.20210226; https://www.slicer.org). The volume of interest (VOI) was constructed by combining the ROIs of the same patient during the portal phase. The delineation procedure was independently performed by two gastrointestinal radiologists with 7 and 12 years of respective experience with blind evaluation. Intraluminal fluid, intragastric air, necrosis area, perigastric adipose tissue, and enlarged LNs were excluded from the contour.
CT radiomics features were extracted using 3D-Slicer software, which automatically generated 1,130 features from VOIs including 14 shape, 18 first-order histogram features, and 1,098 texture features after spatial resampling and gray value normalization.
Feature selection and radiomic signature building
The intra- and inter-observer reproducibility was investigated by randomly selecting 29 cases from the training cohort. Both radiologists performed delineation of images and extraction of radiomics features. To ensure the robustness of the extracted features, we evaluated their reproducibility and stability using intra- and inter-class correlation coefficient (ICC). Radiomics features were retained only when ICC exceeded 0.75.
The Mann-Whitney U test compared the radiomics features between HAS and CGA group in the training set. Repeated 10-fold cross-validation was conducted on the selected features using the least absolute shrinkage and selection operator (LASSO) to identify the most significant ones. The coefficients of each selected feature were determined through a generalized linear model, enabling the computation of the radiomics score (Radscore).
Radiomic nomogram construction and evaluation
The prediction model was established through multivariable logistic regression analysis, which integrated independent clinical parameters and Radscore (P<0.05). The combined model was visualized as the nomogram in the training cohort to enhance the clinical predictive value in the test cohort, which was compared with the clinical model and Radscore model.
Statistical analysis
Statistical analysis in this study was performed using the software SPSS 26.0 (IBM Corp., Armonk, NY, USA) and R software (Version 3.5.1; R Foundation for Statistical Computing, Vienna, Austria). Continuous variables were expressed as mean ± standard deviation (SD) and compared by independent t-test for normally distributed data or Mann-Whitney U test for non-normally distributed data. Chi-squared test or Fisher’s exact test was employed to compare categorical variables. Multivariable logistic regression analysis was applied to identify the independent predictors. The differences in the area under the curve (AUC) were assessed using Delong test. Statistical significance was set at P values below 0.05. The calibration curves were employed to assess the concordance between the predicted and observed probability of the nomogram. Decision curve analysis (DCA) evaluated the actual utility of the nomogram by calculating the net benefits at various threshold probabilities.
Results
Clinical, serum biochemical parameters and CT features
Based on a 7:3 ratio, 126 patients were assigned into the training cohort, whereas the test cohort consisted of 55 patients. Clinical parameters and CT features of HAS and CGA patients are displayed in Table 1. Serum AFP level, CT density on portal phase and delayed phase exhibited significant differences between them in the training cohort (P<0.001, P<0.001, P=0.02) and the test cohort (P=0.02, P=0.001, P=0.07), respectively. Serum CEA level, tumor location, and maximum tumor diameter were significantly different in the training cohort (P=0.001, P=0.001, P<0.001, respectively), yet displayed no statistical difference in the test cohort (P=0.31, P=0.13, P=0.22, respectively).
Table 1
Variables | Training cohort | Test cohort | |||||
---|---|---|---|---|---|---|---|
CGA | HAS | P value | CGA | HAS | P value | ||
Gender | 0.15 | 0.12 | |||||
Male | 58 (68.2) | 33 (80.5) | 24 (64.9) | 16 (88.9) | |||
Female | 27 (31.8) | 8 (19.5) | 13 (35.1) | 2 (11.1) | |||
Age (years) | 64.8±9.9 | 64.2±7.0 | 0.45 | 65.7±9.7 | 67.0±8.3 | 0.62 | |
AFP | <0.001 | 0.02 | |||||
≥20 ng/mL | 1 (1.2) | 21 (51.2) | 1 (2.7) | 5 (27.8) | |||
<20 ng/mL | 84 (98.8) | 20 (48.8) | 36 (97.3) | 13 (72.2) | |||
CEA | 0.001 | 0.31 | |||||
≥5 ng/mL | 16 (18.8) | 18 (43.9) | 8 (21.6) | 7 (38.9) | |||
<5 ng/mL | 69 (81.2) | 23 (56.1) | 29 (78.4) | 11 (61.1) | |||
CA19-9 | 0.19 | 0.36 | |||||
≥37 ng/mL | 16 (18.8) | 12 (29.3) | 7 (18.9) | 1 (5.6) | |||
<37 ng/mL | 69 (81.2) | 29 (70.7) | 30 (81.1) | 17 (94.4) | |||
CA125 | 0.52 | >0.99 | |||||
≥35 ng/mL | 6 (7.1) | 1 (2.4) | 1 (2.7) | 1 (5.6) | |||
<35 ng/mL | 79 (92.9) | 40 (97.6) | 36 (97.3) | 17 (94.4) | |||
Location | 0.001 | 0.13 | |||||
Antrum | 34 (40.0) | 29 (70.7) | 9 (24.3) | 8 (44.4) | |||
Cardia/fundus/body | 51 (60.0) | 12 (29.3) | 28 (75.7) | 10 (55.6) | |||
Maximum diameter (cm) | 3.9±1.6 | 5.3±2.1 | <0.001 | 4.0±1.6 | 4.6±1.6 | 0.22 | |
Growth pattern | 0.67 | 0.39 | |||||
Elevated type | 10 (11.8) | 7 (17.1) | 8 (21.6) | 3 (16.7) | |||
Ulcerative type | 68 (80.0) | 30 (73.2) | 27 (73.0) | 15 (83.3) | |||
Infiltrative type | 7 (8.2) | 4 (9.8) | 2 (5.4) | 0 (0.0) | |||
Necrosis | 0.20 | >0.99 | |||||
Absence | 80 (94.1) | 35 (85.4) | 35 (94.6) | 17 (94.4) | |||
Presence | 5 (5.9) | 6 (14.6) | 2 (5.4) | 1 (5.6) | |||
Clinical T grade | 0.66 | 0.43 | |||||
cT1 | 6 (7.1) | 1 (2.4) | 4 (10.8) | 1 (5.6) | |||
cT2 | 13 (15.3) | 8 (19.5) | 3 (8.1) | 4 (22.2) | |||
cT3 | 52 (61.2) | 26 (63.4) | 20 (54.1) | 10 (55.6) | |||
cT4 | 14 (16.5) | 6 (14.6) | 10 (27.0) | 3 (16.7) | |||
Unenhanced CT density (HU) | 39.2±3.8 | 38.3±3.0 | 0.18 | 39.0±3.6 | 38.2±3.9 | 0.46 | |
Arterial phase CT density (HU) | 67.1±8.0 | 66.2±7.4 | 0.57 | 66.7±8.3 | 64.6±8.1 | 0.37 | |
Portal phase CT density (HU) | 89.8±10.7 | 82.7±7.7 | <0.001 | 93.4±9.9 | 84.2±10.8 | 0.001 | |
Delayed phase CT density (HU) | 77.8±9.9 | 73.3±8.8 | 0.02 | 79.7±8.6 | 75.3±8.4 | 0.07 | |
Enhancement pattern | 0.79 | 0.77 | |||||
Heterogeneous | 74 (87.1) | 35 (85.4) | 28 (75.7) | 15 (83.3) | |||
Homogeneous | 11 (12.9) | 6 (14.6) | 9 (24.3) | 3 (16.7) | |||
Radscore | −1.8±0.9 | 0.78±1.2 | <0.001 | −1.6±1.4 | 0.01±1.2 | <0.001 |
Data are presented as n (%) or mean ± standard deviation. CT, computed tomography; CGA, conventional gastric adenocarcinoma; HAS, hepatoid adenocarcinoma of the stomach; AFP, alpha-fetoprotein; CEA, carcinoembryonic antigen; CA19-9, carbohydrate antigen 19-9; CA125, cancer antigen 125; cm, centimeter; HU, Hounsfield units; Radscore, radiomics score.
Radiomics features selection and signature construction
The radiomics features selection was conducted on the portal phase. VOIs were extended to encompass 1,130 three-dimensional radiomic features, of which 997 features exhibited strong reproducibility (ICC >0.75). We conducted the preliminary Mann-Whitney U test and Spearman rank analysis in order to identify 100 features for further selection. After eliminating the redundant features, a set of 15 radiomics features was selected by LASSO (Figure 2). The favorable radiomics signature can be quantified as the Radscore: . Radscore is a multiparameter radiomics signature, Fi is the feature value of the i-th feature, and Wi is the feature weight of the i-th feature. The Radscores in the two cohorts are shown in Table 1. Useful features after LASSO in the training cohort are demonstrated in Table 2.
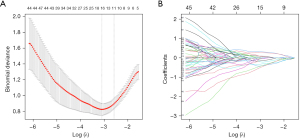
Table 2
Useful features after LASSO | Coefficients |
---|---|
(Intercept) | −0.947607813 |
wavelet.HHH_firstorder_Maximum | −0.548949393 |
wavelet.HHL_glcm_Imc2 | −0.468042456 |
wavelet.HLH_glcm_InverseVariance | −0.42637156 |
log.sigma.3.0.mm.3D_firstorder_Skewness | −0.337821351 |
log.sigma.3.0.mm.3D_glszm_ZoneEntropy | 0.320295709 |
wavelet.LLH_glcm_Imc2 | 0.266089709 |
log.sigma.3.0.mm.3D_glcm_ClusterShade | −0.169327246 |
original_firstorder_90Percentile | −0.112368092 |
wavelet.HHL_glcm_Correlation | 0.107557756 |
wavelet.LLL_firstorder_90Percentile | −0.09326564 |
original_shape_MajorAxisLength | 0.092442496 |
original_shape_Sphericity | −0.079912906 |
log.sigma.4.0.mm.3D_glcm_ClusterShade | −0.070496245 |
wavelet.LLL_firstorder_Mean | −0.054802644 |
original_shape_Maximum2DDiameterRow | 0.032665275 |
LASSO, least absolute shrinkage and selection operator.
Radiomics signature predictive performance
Regardless of the training or test cohort, the Radscore of HAS patients was significantly higher than that of CGA patients (P<0.001 for both). The Radscore based on the portal phase performed well in the training cohort with an AUC of 0.949 [95% confidence interval (CI): 0.895–0.980]. The robust performance was further validated in the test cohort, where it exhibited an AUC of 0.868 (95% CI: 0.749–0.944) (Table 3).
Table 3
Variables | AUC (95% CI) | Sensitivity (%) | Specificity (%) |
---|---|---|---|
Training-AFP | 0.726 (0.639–0.801) | 46.3 | 98.8 |
Test-AFP | 0.681 (0.541–0.800) | 38.9 | 97.3 |
Training-Radscore | 0.949 (0.895–0.980) | 97.6 | 80.0 |
Test-Radscore | 0.868 (0.749–0.944) | 88.9 | 75.7 |
Training-nomogram | 0.970 (0.923–0.992) | 92.7 | 89.4 |
Test-nomogram | 0.905 (0.796–0.968) | 88.9 | 83.8 |
AFP, alpha-fetoprotein; AUC, area under the curve; CI, confidence interval; Radscore, radiomics score.
Construction of radiomics nomogram
Statistically different indicators were incorporated from the training set into a univariate analysis in which sex, age, radiomic signature, CEA level, and tumor location were associated with the differentiation of HAS from CGA. Multivariable logistic regression analysis revealed AFP and radiomic signature as statistically significant independent predictors (Table 4). AFP and Radscore were integrated into the nomogram. The CT-based radiomics nomogram consisted of five components: points, Radscore, AFP, total points, and predicted probability. The initial “Points” scale was devised to assign scores for each predictor. The final predicted probability was determined by combining the scores derived from AFP and Radscore. Figure 3 illustrates the CT-based radiomics nomogram.
Table 4
Variables | Multivariate analysis | ||
---|---|---|---|
Odds ratio (95% CI) | Wald (χ2 value) | P value | |
AFP | |||
≥20 ng/mL | Ref. | ||
<20 ng/mL | 0.03 (0.000–0.145) | 8.71 | 0.001 |
CEA | |||
≥5 ng/mL | Ref. | ||
<5 ng/mL | 0.240 (0.028–2.087) | 1.67 | 0.20 |
Location | |||
Antrum | Ref. | ||
Cardia/fundus/body | 0.295 (0.037–2.323) | 1.35 | 0.25 |
Maximum diameter (cm) | 1.334 (0.851–2.092) | 1.58 | 0.21 |
Portal phase CT density (HU) | 0.864 (0.723–1.032) | 2.59 | 0.11 |
Delayed phase CT density (HU) | 1.202 (0.991–1.457) | 3.48 | 0.06 |
Radscore | 28.634 (4.568–179.491) | 12.83 | <0.001 |
AFP, alpha-fetoprotein; CEA, carcinoembryonic antigen; CI, confidence interval; CT, computed tomography; HU, Hounsfield units; Radscore, radiomics score.
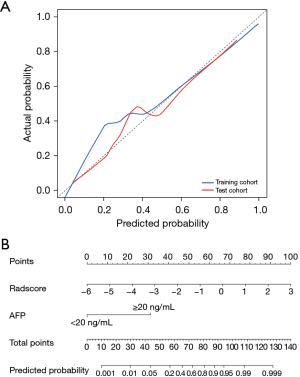
Validation of radiomics nomogram
The nomogram model, comprised of AFP and radiomics signature, was developed to distinguish HAS from CGA in terms of the regression coefficients. The radiomics nomogram achieved excellent diagnostic performance with AUCs of 0.970 and 0.905 in the training and test cohorts, respectively (Table 3). The calibration curves of the nomogram model displayed good agreement between the observed outcome and the prediction for individualized patient of HAS (Figure 3).
Comparison among three models
The nomogram model demonstrated the most superior diagnostic accuracy in both the training cohort (AUC =0.970) and test cohort (AUC =0.905), followed by the radiomics signature model (training cohort, AUC =0.949, P=0.04; test cohort, AUC =0.868, P=0.10). AUC of the former two models significantly outperformed the AFP model in terms of training diagnosis (AUC =0.726, P=1×10−5 for both the nomogram and radiomics signature model) and test diagnosis (AUC =0.681, P=1×10−5 for the nomogram model, P=0.02 for the radiomics signature model). DCA (Figure 4) demonstrated that the nomogram model outperformed both AFP and radiomic signature models in identifying HAS.
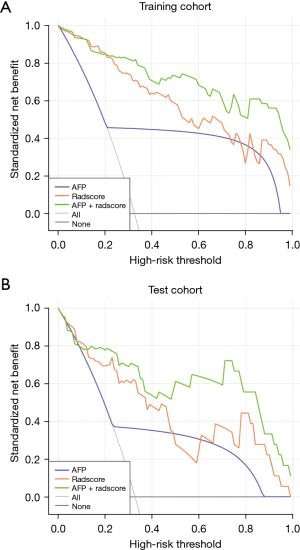
Discussion
The clinical manifestations and imaging features of HAS are similar to those of CGA. However, the surgical procedure for HAS is more complex and the prognosis is worse compared to CGA. The preoperative differentiation of HAS should be given immediate priority. An individualized CT-based radiomics nomogram incorporating a radiomics signature from the portal phase and serum AFP level may offer potential value in distinguishing HAS from CGA prior to surgery. The investigation of radiomics features to differentiate these two malignancies has been limited in existing research.
Biochemical indicators cannot clearly identify the subtypes of gastric adenocarcinoma. The serum AFP level significantly increases in 40–60% of patients with HAS, including asymptomatic individuals (20,23). Previous studies have regarded it as a standalone predictive parameter for HAS diagnosis and prognosis (24,25). Yang et al. reported that elevated serum AFP was important for HAS, but adding it into HAS diagnosis criteria may not be necessary (1). In this study, the serum AFP model exhibited an AUC of only 0.726 and 0.681 in the training and test cohorts, respectively, which aligns with previous research.
On multiphase CT, CGA is commonly featured with focal enhancement on the arterial phase and persistent enhancement on the portal phase (26). HAS often displays slight to moderate heterogenous enhancement (27). The peak CT density of HAS usually appears during the portal phase, of which the blood supply is weaker than that of CGA (28). The contrast agent diffuses into the interstitial space of gastric tumor, and its clearance is impeded by fibrosis during the portal phase (29,30), which reveals potential for distinguishing HAS.
The hepatocellular differentiation area in HAS can coexist with the adenocarcinoma area, whereas CGA exclusively consists of the adenocarcinoma area. Radscore is derived from radiomics features that have been selected through a high-throughput radiomics feature selection process. In this study, it serves as an independent predictor for preoperative distinguishment between HAS and CGA, which may suggest the presence of a hepatocellular differentiation area. We found that the diagnostic accuracy of Radscore was significantly higher than that of the AFP model, yet lower than that of the nomogram model. Our radiomics nomogram, integrating radiomics signature and serum AFP level, yielded a sensitivity of 92.7% and 89.4% and specificity of 88.9% and 83.8% in the two cohorts, respectively. The increased cost of the CT-based radiomics nomogram, in comparison to traditional CT, primarily encompasses the expenses associated with serum biochemical examination (AFP) for patients and the time required for doctors to delineate lesions during clinical application. However, these additional costs do not impose an excessive burden on patients. The radiomics nomogram, serving as a quantitative biomarker and predictive tool, was shown to have the capability to decipher tumor heterogeneity on a macroscopic scale.
There are several deficiencies in this study. Firstly, it should be noted that this single center study comprised a limited number of cases; external validation in a larger cohort is required to confirm the robustness of the radiomics nomogram. Secondly, the inclusion of supplementary imaging features may be necessary in subsequent investigations through a comprehensive imaging examination. The identification of LN and hepatic metastasis may necessitate enhanced CT and magnetic resonance scans; however, an accurate diagnosis is contingent upon pathological findings. CT venography may need underwent for imaging diagnosis of portal vein thrombosis in the trunk and/or branches. PET plays a crucial role in the preoperative assessment of gastric adenocarcinoma metabolism, metastasis, and postoperative recurrence. Eventually, with further exploration into the depth of infiltration prediction, postoperative long-term quality of life, and automated segmentation, radiomics analysis has the potential to yield more significant advancements in terms of HAS and CGA.
Conclusions
In a nutshell, radiomics analysis offers promise for differentiating HAS from CGA, and the CT-based radiomics nomogram is likely to have significant clinical implications on HAS distinction.
Acknowledgments
Funding: This work was supported by
Footnote
Reporting Checklist: The authors have completed the TRIPOD reporting checklist. Available at https://jgo.amegroups.com/article/view/10.21037/jgo-24-210/rc
Data Sharing Statement: Available at https://jgo.amegroups.com/article/view/10.21037/jgo-24-210/dss
Peer Review File: Available at https://jgo.amegroups.com/article/view/10.21037/jgo-24-210/prf
Conflicts of Interest: All authors have completed the ICMJE uniform disclosure form (available at https://jgo.amegroups.com/article/view/10.21037/jgo-24-210/coif). The authors have no conflicts of interest to declare.
Ethical Statement: The authors are accountable for all aspects of the work in ensuring that questions related to the accuracy or integrity of any part of the work are appropriately investigated and resolved. The study was conducted in accordance with the Declaration of Helsinki (as revised in 2013). The study was approved by the Institutional Review Board of Affiliated Hospital of Nanjing University of Chinese Medicine (No. 2024NL-123-02) and the requirement for individual consent for this retrospective analysis was waived.
Open Access Statement: This is an Open Access article distributed in accordance with the Creative Commons Attribution-NonCommercial-NoDerivs 4.0 International License (CC BY-NC-ND 4.0), which permits the non-commercial replication and distribution of the article with the strict proviso that no changes or edits are made and the original work is properly cited (including links to both the formal publication through the relevant DOI and the license). See: https://creativecommons.org/licenses/by-nc-nd/4.0/.
References
- Yang X, Wang A, Li J, et al. Prognostic significance of preoperative serum tumor markers in hepatoid adenocarcinoma of stomach (HAS). BMC Cancer 2023;23:53. [Crossref] [PubMed]
- Lim TH, Kim JW, Kim MJ. CT Findings of Hepatic Metastasis from Hepatoid Adenocarcinoma of the Rectum Mimicking Hepatocellular Carcinoma: A Case Report. J Korean Soc Radiol 2024;85:409-14. [Crossref] [PubMed]
- Zhou K, Wang A, Ao S, et al. The prognosis of hepatoid adenocarcinoma of the stomach: a propensity score-based analysis. BMC Cancer 2020;20:671. [Crossref] [PubMed]
- Wang Y, Sun L, Li Z, et al. Hepatoid adenocarcinoma of the stomach: a unique subgroup with distinct clinicopathological and molecular features. Gastric Cancer 2019;22:1183-92. [Crossref] [PubMed]
- Nagtegaal ID, Odze RD, Klimstra D, et al. The 2019 WHO classification of tumours of the digestive system. Histopathology 2020;76:182-8. [Crossref] [PubMed]
- Zhu H, Li Q, Qian L. Liver metastasis from hepatoid adenocarcinoma of the stomach: a case report and literature review. Front Oncol 2024;14:1297062. [Crossref] [PubMed]
- Li M, Mei YX, Wen JH, et al. Hepatoid adenocarcinoma-Clinicopathological features and molecular characteristics. Cancer Lett 2023;559:216104. [Crossref] [PubMed]
- Huang ZN, Huang YQ, Hong QQ, et al. Long-term prognostic benefit of adjuvant chemotherapy for patients with hepatoid adenocarcinoma of the stomach after radical resection: A national multicenter study. Eur J Surg Oncol 2023;49:106975. [Crossref] [PubMed]
- Xia R, Zhou Y, Wang Y, et al. Hepatoid Adenocarcinoma of the Stomach: Current Perspectives and New Developments. Front Oncol 2021;11:633916. [Crossref] [PubMed]
- Ge DF, Wang YK, Li YY, et al. Assessing clinical pathological characteristics and gene expression patterns associated with hepatoid adenocarcinoma of the stomach. Clin Transl Oncol 2024;26:2674-84. [Crossref] [PubMed]
- Yang X, Wu Y, Wang A, et al. Immunohistochemical characteristics and potential therapeutic regimens of hepatoid adenocarcinoma of the stomach: a study of 139 cases. J Pathol Clin Res 2024;10:e343. [Crossref] [PubMed]
- Giganti F, Tang L, Baba H. Gastric cancer and imaging biomarkers: Part 1 - a critical review of DW-MRI and CE-MDCT findings. Eur Radiol 2019;29:1743-53. [Crossref] [PubMed]
- Findlay JM, Antonowicz S, Segaran A, et al. Routinely staging gastric cancer with (18)F-FDG PET-CT detects additional metastases and predicts early recurrence and death after surgery. Eur Radiol 2019;29:2490-8. [Crossref] [PubMed]
- Young JJ, Pahwa A, Patel M, et al. Ligaments and Lymphatic Pathways in Gastric Adenocarcinoma. Radiographics 2019;39:668-89. [Crossref] [PubMed]
- Tabari A, Chan SM, Omar OMF, et al. Role of Machine Learning in Precision Oncology: Applications in Gastrointestinal Cancers. Cancers (Basel) 2022;15:63. [Crossref] [PubMed]
- Nie T, Liu D, Ai S, et al. A radiomics nomogram analysis based on CT images and clinical features for preoperative Lauren classification in gastric cancer. Jpn J Radiol 2023;41:401-8. [Crossref] [PubMed]
- Zhao H, Liang P, Yong L, et al. Development and external validation of a radiomics model for assessment of HER2 positivity in men and women presenting with gastric cancer. Insights Imaging 2023;14:20. [Crossref] [PubMed]
- Wang R, Liu H, Liang P, et al. Radiomics analysis of CT imaging for differentiating gastric neuroendocrine carcinomas from gastric adenocarcinomas. Eur J Radiol 2021;138:109662. [Crossref] [PubMed]
- Chen T, Wu J, Cui C, et al. CT-based radiomics nomograms for preoperative prediction of diffuse-type and signet ring cell gastric cancer: a multicenter development and validation cohort. J Transl Med 2022;20:38. [Crossref] [PubMed]
- Lin JX, Wang ZK, Hong QQ, et al. Assessment of Clinicopathological Characteristics and Development of an Individualized Prognostic Model for Patients With Hepatoid Adenocarcinoma of the Stomach. JAMA Netw Open 2021;4:e2128217. [Crossref] [PubMed]
- In H, Solsky I, Palis B, et al. Validation of the 8th Edition of the AJCC TNM Staging System for Gastric Cancer using the National Cancer Database. Ann Surg Oncol 2017;24:3683-91.
- Hallinan JT, Venkatesh SK. Gastric carcinoma: imaging diagnosis, staging and assessment of treatment response. Cancer Imaging 2013;13:212-27. [Crossref] [PubMed]
- Rajasekaran P, Pattnaik B, Mishra M, et al. Alpha-Fetoprotein (AFP)-Negative Hepatoid Adenocarcinoma of the Stomach and its Associated Uncommon Features With a Review. Int J Surg Pathol 2023;31:872-8. [Crossref] [PubMed]
- Li L, Yang X, Ji W, et al. Emphasis on the clinical relationship between alpha-fetoprotein and hepatoid adenocarcinoma of the stomach: a retrospective study. BMC Gastroenterol 2023;23:142. [Crossref] [PubMed]
- Zhang ZR, Wu J, Li HW, et al. Hepatoid adenocarcinoma of the stomach: Thirteen case reports and review of literature. World J Clin Cases 2020;8:1164-71. [Crossref] [PubMed]
- Chen XH, Ren K, Liang P, et al. Spectral computed tomography in advanced gastric cancer: Can iodine concentration non-invasively assess angiogenesis? World J Gastroenterol 2017;23:1666-75. [Crossref] [PubMed]
- Chang MY, Kim HJ, Park SH, et al. CT features of hepatic metastases from hepatoid adenocarcinoma. Abdom Radiol (NY) 2017;42:2402-9. [Crossref] [PubMed]
- Fu Y, Zhu H, Peng WJ. Gastric Hepatoid Adenocarcinoma: Differentiation From Gastric Adenocarcinoma With Dynamic Contrast-Enhanced Computed Tomographic Findings. J Comput Assist Tomogr 2019;43:887-91. [Crossref] [PubMed]
- Miles KA. Tumour angiogenesis and its relation to contrast enhancement on computed tomography: a review. Eur J Radiol 1999;30:198-205. [Crossref] [PubMed]
- Tang L, Li ZY, Li ZW, et al. Evaluating the response of gastric carcinomas to neoadjuvant chemotherapy using iodine concentration on spectral CT: a comparison with pathological regression. Clin Radiol 2015;70:1198-204. [Crossref] [PubMed]