Identifying a CD8T cell signature in the tumor microenvironment to forecast gastric cancer outcomes from sequencing data
Highlight box
Key findings
• The tumor microenvironment (TME) could be critical in carcinogenesis, immune evasion, and treatment response. TME-related genes are good prognostic indicators for gastric cancer (GC).
What is known and what is new?
• TME-related genes could be critical in the evaluation of GC.
• CD8T cell-related subtypes and biomarkers were identified for GC.
What is the implication, and what should change now?
• We have provided novel insights into the diagnosis and treatment of GC based on our findings.
Introduction
Patients with gastric cancer (GC) usually have a poor prognosis because it is often diagnosed at an advanced stage (1). GC is characterized by considerable heterogeneity, making gastroscopy an imperfect tool for early detection. Around 784,000 people worldwide died from GC in 2018, according to the World Health Organization (2,3). Furthermore, it ranks as the fifth most prevalent malignancy, with over 1 million new cases reported annually (2,3). Despite substantial progress in cancer biology, including the identification of risk factors and the development of new treatments for GC in the past decade, there has been minimal improvement in early diagnosis, therapy, prevention, and prognosis prediction (3). Therefore, it is crucial to discover new markers for GC.
Recent studies have attracted increased attention to the potential role of the tumor microenvironment (TME) in carcinogenesis, immune evasion, and treatment response (4,5). The TME consists primarily of immune cells, stromal cells, endothelial cells, extracellular matrix (ECM) molecules, and various cytokines and chemokines (4,5). Immune cells and stromal cells have been found to be of significant value for the diagnosis and prognosis of cancers (4,5). Furthermore, tumor immune cells exhibit heterogeneity, functional plasticity, and phenotypic plasticity, and can have both pro- and anti-tumorigenic effects (6). The precise spatial relationship between different subgroups of immune cells and cancer cells is critical for predicting tumor behavior (7,8). Many researchers acknowledge that the composition of the TME can affect treatment responses, including T cells, B cells, neutrophils, and macrophages (9-11). Prior research has shown that the deletion of cyclic GMP-AMP synthase-stimulator of interferon genes (cGAS-STING) causes tumor-associated macrophages (TAMs) to shift toward a pro-inflammatory subtype and induces apoptosis in GC cells, emphasizing the negative effect of STING in TAMs (12). Therefore, enhancing our comprehension of the spatial relationships between individual cellular and acellular components in the TME and uncovering the underlying mechanism will enhance the diagnosis and treatment of GC.
The development of single-cell messenger RNA (mRNA) sequencing (scRNA-seq) has revolutionized our capability to characterize the transcriptional states of thousands of individual cells, enabling us to conduct impartial analysis of cellular lineages within tissues (13). This technology has successfully identified cell types and described complex subpopulations within organ tissues such as the pancreas, lungs, and various cancers, including melanoma and colorectal cancer (13).
In this study, we initially identified the key prognostic genes related to CD8T cell in TME using GC scRNA-seq data and GC tissue transcriptome data. Cluster analysis was conducted on GC patients to identify distinct subtypes of CD8T cells related to the TME. We also analyzed the clinical characteristics, immune infiltration, and differences in CD8T cells related to the TME among these subtypes. Furthermore, we then developed a risk model to predict prognosis and response to immunotherapy and finally screened seven genes, which could potentially serve as markers and targets for the detection and treatment of GC. We present this article in accordance with the TRIPOD reporting checklist (available at https://jgo.amegroups.com/article/view/10.21037/jgo-24-603/rc).
Methods
Single-cell sequencing analysis of GC
To characterize GC TMEs at single-cell resolution, we employed the tumor immune single-cell hub2 (TISCH2) pipeline (http://tisch.comp-genomics.org/). To annotate cell types, we searched the Gene Expression Omnibus (GEO) database for data from the GSE134520 database. In TISCH2, each dataset is provided with the results of gene set enrichment analysis (GSEA). The GSEA tab uses the MAESTRO workflow to perform hallmark pathway analysis on genes that are up- or down-regulated between clusters (InferCNV method). Each clusters’ differential gene expression will be displayed as a heatmap to show the pathways that are up- or down-regulated. The study was conducted in accordance with the Declaration of Helsinki (as revised in 2013).
Data acquisition from TCGA-GC cohort
A total of 375 mRNA transcriptome profiles, as well as clinical data of TCGA-GC patients, were downloaded from the TCGA database (https://portal.gdc.cancer.gov/repository).
Identification of CD8T cell-related tumor subtypes in GC
This study was conducted to investigate the relationship between CD8T cell-related genes and GC subtypes. We conducted consensus cluster analysis using the consensus clusterplus package (version 1.54.0) on the TCGA-GC cohort. The number of clusters (k), which represents the different groupings in the analysis, was set to range from 2 to 6. We generated heatmaps using the “Pheatmap” package (version 1.0.12). We compared survival times among the subgroups using Kaplan-Meier analysis.
Identification of differentially expressed genes (DEGs) in two GC subtypes
We detected DEGs among GC clusters using the “DEseq2” package in R. We set the cutoff criteria at a P value of 0.05 and a log2 fold change greater than 1.00. We also visualized the DEGs using the “ggplot2” R package. We used the “pheatmap” R package (version 1.0.12) to create a DEG heatmap. We then performed Gene Ontology (GO) and Kyoto Encyclopedia of Genes and Genomes (KEGG) analyses using the “clusterProfiler” package.
The immune activity of two CD8T cell-related clusters in GC
The “immunoeconomics” was employed to investigate the immune functionality of two clusters of CD8T cells in GC. This approach facilitated a detailed analysis of the immune activity associated with these CD8T cell clusters. Furthermore, an evaluation of eight immune checkpoint genes—namely CD274, PDCD1, PDCD1LG2, CTLA4, LAG3, HAVCR2, TIGIT, and SIGLEC15—was performed to compare the immune activity across the two clusters. Visualization of the data was accomplished through the creation of a heatmap and a box plot, utilizing the R packages “pheatmap” and “ggplot2”. To assess the infiltration of immune cells and the activation of immune pathways between the two groups, the Wilcoxon test was applied. A P value of less than 0.05 was considered indicative of statistical significance.
Identification of prognostic CD8T cell-related genes in GC
Using univariate Cox analysis, we identified genes with prognostic values less than 0.05 in patients with GC. Using the R package “venn” (v. 1.11; The R Foundation for Statistical Computing, Vienna, Austria), a Venn diagram was generated. R package “pheatmap” (v. 1.0.12) was used to construct the expression heatmap. To identify signaling pathways of prognostic genes in GC, we performed KEGG pathway enrichment analyses with the clusterProfiler package in R.
The development of a prognostic model based on CD8T cell-related genes
The λ condition was established for variables with nonzero coefficients, based on the minimum criteria. Using the following formula, the risk score was calculated: risk score = sum (expression level × corresponding coefficient). The median risk scores of TCGA-GC patients were used to divide them into low- and high-risk groups. The overall survival (OS) times of the different subgroups were compared using the R package “survival”. Based on Cox proportional hazards, we calculated hazard ratios (HRs) and 95% confidence intervals (CIs).
Statistical analysis
Survival analysis was conducted to identify prognostic genes for GC, with a significance level set at P<0.05. Bilateral P values <0.05 were deemed statistically significant for multiple testing.
Results
Cell types and distributions of GC
The scRNA-Seq dataset from GSE134520 was used in this study. Unsupervised hierarchical clustering analysis of the scRNA-seq data revealed 22 distinct cell subtypes of GC, which differentiated between patients with GC and healthy individuals (Figure 1A). Cell clusters in GC, such as CD8T cells, dendritic cells (DC), fibroblasts cells, gland mucous, malignant cells, mast cells, myofibroblasts cells, pit mucous, and plasma cells, were identified using known cell-type markers (Figure 1B). Furthermore, the distribution of cell types was analyzed in both healthy individuals and GC patients. The distribution of cell types in pancreatic tissue of GC patients compared to healthy individuals included varying percentages of CD8Tex cells (6.42% vs. 0.61%), DC (0.36% vs. 1.48%), fibroblast cells (3.37% vs. 1.31%), malignant cells (2.96% vs. 0.72%), gland mucous (22.62% vs. 11.19%), mast cells (1.41% vs. 0.23%), myofibroblast cells (0.79% vs. 0.11%), pit mucous (57.44% vs. 80.74%), and plasma cells (4.63% vs. 3.61%), as shown in Figure 1C,1D. Interestingly, the ratio of CD8Tex, malignant cells, and gland mucous was higher, consistent with the characteristic feature of cancers with an excessive TME containing malignant cells and infiltrated immune cells, compared to healthy tissue, whereas the ratio of pit mucous decreased in GC.
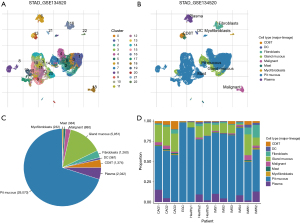
GC subtypes based on the CD8T cell-related genes
Despite the close association of CD8T cells with tumor development (4-7), the specific role of CD8T cell-related genes in GC has yet to be thoroughly investigated. A total of 612 DEGs were identified in CD8T cells compared to other cell types. Through consensus clustering analysis, we classified TCGA-GC patients into different subtypes based on the expression of these 612 DEGs (Figure 2A-2C). Consistency clustering and principal component analysis (PCA) revealed that TCGA-GC patients were effectively stratified into 2 clusters when the clustering variable (k) was 2 (Figure 2A-2D). A heatmap of TCGA-GC patients demonstrated clear separation between the 2 groups (Figure 2E). The OS of patients in the low-risk group 1 was significantly longer than that of those in the high-risk group 2 (HR: 0.663, 95% CI: 0.465–0.945; P=0.02; Figure 2F).
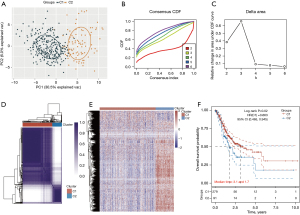
Identification of underlying mechanisms between the two GC groups
We identified 1,285 DEGs between the G1 and G2 groups using a volcano plot (P value <0.05; |log2 fold change| >1). In comparison to G2 group, 34 genes were upregulated and 1,251 were downregulated in the G1 group. The biological roles of the 1,285 DEGs were clarified using KEGG and GO enrichment analyses. According to the KEGG analysis, 34 upregulated genes are mainly involved in viral protein interaction with cytokine and cytokine receptor, tumor necrosis factor (TNF) signaling pathway, interleukin (IL)-17 signaling pathway, and chemokine signaling pathway (Figure 3A), whereas 1,251 downregulated genes are mainly involved in the cGMP-PKG signaling pathway, proteoglycans in cancer, focal adhesion, ECM-receptor interaction, and cell adhesion molecules (CAMs) (Figure 3B). The GO analysis of the biological process results revealed that the upregulated genes were mainly associated with monocyte chemotaxis, mononuclear cell migration, positive regulation of T cell migration, and humoral immune response (Figure 3C), whereas the downregulated genes were linked to ECM organization, extracellular structure organization, cell-substrate adhesion, and cell–matrix adhesion (Figure 3D). Previous studies have demonstrated that proteoglycans involved in cancer, focal adhesion, ECM-receptor interaction, and CAMs serve as tumor markers (14,15). Based on the aforementioned findings, G2 GC subtypes may exhibit higher migratory and proliferative capabilities compared to G1 GC subtypes.
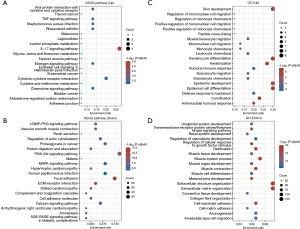
The immune activity of two groups in GC
A comparative analysis of the immune profiles between two distinct cohorts of GC patients was conducted, revealing significant disparities in immune cell populations. Specifically, the G1 cohort exhibited a reduced presence of B cells, CD4+ T cells, endothelial cells, and macrophages when contrasted with the G2 cohort (refer to Figure 4A). Additionally, the box plots illustrated that the expression levels of three genes associated with immune checkpoint inhibitors (ICIs), namely HAVCR2, PDCD1LG2, and TIGIT, were notably diminished in the G1 GC samples compared to those from the G2 cohort (see Figure 4B).
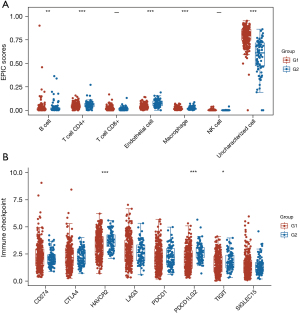
Identification of key CD8T cell-related genes in GC
TCGA-GC patients were analyzed using multivariate Cox regression to identify genes associated with poor prognosis and clarify their role in CD8T cell function. According to this analysis, 842 prognostic genes were identified for GC; Figure 5A shows the top 20 prognostic genes. A Venn diagram was used to further screen out prognostic genes associated with CD8Tex cells (Figure 5B). We eventually identified 23 CD8T cell-related prognostic genes: TCIM, AADAC, SLC2A3, ZNF331, TSC22D3, CMTM3, ZFP36, VIM, CLDND1, GABARAPL1, SOCS3, RGS1, TCEAL9, RGS2, CD59, SPRY1, EMP3, ZEB2, PDE4B, GLIPR1, ERRFI1, and LBH (Figure 5B).
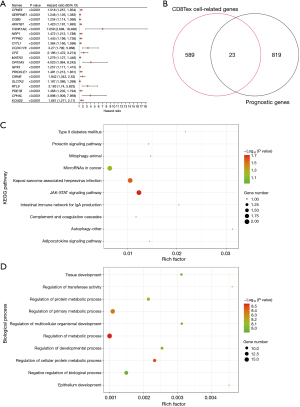
The KEGG enrichment of these genes indicated that they may play a crucial role in GC via Janus kinase-signal transducers and activators oftranscription (JAK-STAT) signaling pathway, microRNAs (miRNAs) in cancer, and autophagy (Figure 5C). According to the GO analysis of the biological process results, most of the upregulated genes were involved in regulation of metabolic process, regulation of transferase activity, and regulation of cellular protein metabolic process (Figure 5D).
The development of a prognostic model based on key CD8T cell-related genes
Through Cox regression analysis, 23 prognostic genes for CD8T cells in GC were selected (Figure 6A). A signature consisting of seven genes was developed based on the optimal λ value (Figure 6A). The risk score was calculated as follows: risk score = CXCR4 expression × (0.1621) + AADAC expression × (0.1237) +SLC2A3 expression × 0.1306 + CMTM3 expression × 0.2336 + RGS2 expression × 0.2292 + CD59 expression × 0.1995 + ZEB2 expression × (−0.4868). Based on this gene signature, TCGA-GC patients were divided into low- and high-risk groups (Figure 6A). There was a higher OS rate for patients with a low-risk score compared to those with a high-risk score (HR: 2.299, 95% CI: 1.626–3.251; P<0.001; Figure 6B). The area under the receiver operating characteristic (ROC) curve (AUC) was used to test prognostic models. The AUCs were 0.62, 0.708, and 0.707, respectively, for 1 year, 3 years, and 5 years (Figure 6C), indicating good prognostication with this model.
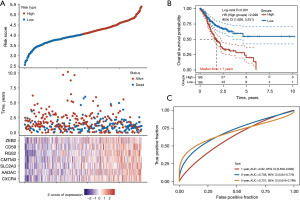
Discussion
According to a series of studies, TME plays a significant role in carcinogenesis, immune evasion, and treatment response (4,5). For example, knockout of cGAS-STING leads to the polarization of TAMs towards a pro-inflammatory subtype, and triggers apoptosis in GC cells (12). Several studies have found that the context of TME affects immunotherapy responses and chemotherapy benefits, as well as patient prognosis (16,17). According to Guo et al., GC cells overexpress T cell-positive regulators, which promote T cell proliferation (17). Increasing CD8+ T cells infiltration further enhanced anti-tumor efficacy of anti-PD-1 in GC (18). Thus, T cells for TME might be important in the development of GC. However, the relationship between T cells and GC remains largely unexplored.
The CD8+ T cells-related genes were used to group divide TCGA-GC patients into two groups (high- and low-risk). The expression of CD8+ T cells-related genes had a positive correlation with prognosis in patients with GC. The immune activity of the low-risk G1 group was lower than that of high-risk G2 group. These results suggest that GC tumors can be classified into two subgroups based on the CD8+ T cells-related genes. A prognostic model consisting of seven CD8+ T cells-related genes (CXCR4, AADAC, SLC2A3, CMTM3, RGS2, CD59, and ZEB2) was developed for patients with GC.
There was a previous article similar to our research. We summarized the differences between the previous article and our manuscript. However, the most significant difference lies in the methods used for selecting genes. In the research conducted by Zhu et al., 174 immune-related genes were identified, and a novel risk model was subsequently developed (19). However, they did not perform any further screening of these genes. In our study, combined with univariate Cox analysis, we ultimately identified 23 CD8T cell-related prognostic genes: TCIM, AADAC, SLC2A3, ZNF331, TSC22D3, CMTM3, ZFP36, VIM, CLDND1, GABARAPL1, SOCS3, RGS1, TCEAL9, RGS2, CD59, SPRY1, EMP3, ZEB2, PDE4B, GLIPR1, ERRFI1, and LBH. Moreover, using the Cox regression model to prioritize the 23 CD8T cell-related genes, we finally selected 7 genes: CXCR4, AADAC, SLC2A3, CMTM3, RGS2, CD59, and ZEB2. Our findings have the potential to provide novel insights into the diagnosis and treatment of GC.
Knockdown of C-X-C chemokine receptor type 4 (CXCR4) inhibited the malignant phenotypes of the cancer stem cells (CSCs) in vitro and curtailed tumorigenesis and liver metastasis in GC (20). Solute carrier family 2, facilitated glucose transporter member 3 (SLC2A3) promotes macrophage infiltration by glycolysis reprogramming in GC (21). Hsa_circ_0043603 promotes the progression of esophageal squamous cell carcinoma by regulating arylacetamide deacetylase (AADAC) expression (22). Moreover, CKLF-like MARVEL transmembrane domain-containing protein 3 (CMTM3) inhibits cell migration and invasion and correlates with favorable prognosis via the STAT3/Twist1/EMT signaling pathway in GC (23,24). In GC, the deposition of the regulator of G-protein signaling 2 (RGS2) increased with the increase of clinical stage (25). Lipoplex-mediated silencing of membrane regulators (CD46, CD55, and CD59) enhances complement-dependent anti-tumor activity of trastuzumab and pertuzumab (26). Zinc finger E-box-binding homeobox 2 (ZEB2) promotes the metastasis of GC and modulates epithelial–mesenchymal transition of GC cells (27).
This study has some limitations. Further verification of these findings needs to be conducted by examining these seven genes in vivo and in vitro. It is also important to consider the related molecular mechanisms in future research.
Conclusions
In conclusion, CD8Tex cells related to TME are closely related to tumor classification and immunity in patients with GC. As a prognostic predictor of GC, the CD8Tex cell-related signature showed good performance. There may be new insights into the diagnosis and treatment of GC resulting from these findings.
Acknowledgments
Funding: This work was supported by
Footnote
Reporting Checklist: The authors have completed the TRIPOD reporting checklist. Available at https://jgo.amegroups.com/article/view/10.21037/jgo-24-603/rc
Peer Review File: Available at https://jgo.amegroups.com/article/view/10.21037/jgo-24-603/prf
Conflicts of Interest: All authors have completed the ICMJE uniform disclosure form (available at https://jgo.amegroups.com/article/view/10.21037/jgo-24-603/coif). The authors have no conflicts of interest to declare.
Ethical Statement: The authors are accountable for all aspects of the work in ensuring that questions related to the accuracy or integrity of any part of the work are appropriately investigated and resolved. The study was conducted in accordance with the Declaration of Helsinki (as revised in 2013).
Open Access Statement: This is an Open Access article distributed in accordance with the Creative Commons Attribution-NonCommercial-NoDerivs 4.0 International License (CC BY-NC-ND 4.0), which permits the non-commercial replication and distribution of the article with the strict proviso that no changes or edits are made and the original work is properly cited (including links to both the formal publication through the relevant DOI and the license). See: https://creativecommons.org/licenses/by-nc-nd/4.0/.
References
- Smyth EC, Nilsson M, Grabsch HI, et al. Gastric cancer. Lancet 2020;396:635-48. [Crossref] [PubMed]
- Bray F, Ferlay J, Soerjomataram I, et al. Global cancer statistics 2018: GLOBOCAN estimates of incidence and mortality worldwide for 36 cancers in 185 countries. CA Cancer J Clin 2018;68:394-424. [Crossref] [PubMed]
- Qian Y, Zhai E, Chen S, et al. Single-cell RNA-seq dissecting heterogeneity of tumor cells and comprehensive dynamics in tumor microenvironment during lymph nodes metastasis in gastric cancer. Int J Cancer 2022;151:1367-81. [Crossref] [PubMed]
- Hinshaw DC, Shevde LA. The Tumor Microenvironment Innately Modulates Cancer Progression. Cancer Res 2019;79:4557-66. [Crossref] [PubMed]
- Elhanani O, Ben-Uri R, Keren L. Spatial profiling technologies illuminate the tumor microenvironment. Cancer Cell 2023;41:404-20. [Crossref] [PubMed]
- Moutafi M, Rimm DL. Putting the Microenvironment into the Immunotherapy Companion Diagnostic. Clin Cancer Res 2021;27:3812-4. [Crossref] [PubMed]
- Carstens JL, Correa de Sampaio P, Yang D, et al. Spatial computation of intratumoral T cells correlates with survival of patients with pancreatic cancer. Nat Commun 2017;8:15095. [Crossref] [PubMed]
- Keren L, Bosse M, Marquez D, et al. A Structured Tumor-Immune Microenvironment in Triple Negative Breast Cancer Revealed by Multiplexed Ion Beam Imaging. Cell 2018;174:1373-1387.e19. [Crossref] [PubMed]
- Huang YK, Wang M, Sun Y, et al. Macrophage spatial heterogeneity in gastric cancer defined by multiplex immunohistochemistry. Nat Commun 2019;10:3928. [Crossref] [PubMed]
- Cabrita R, Lauss M, Sanna A, et al. Tertiary lymphoid structures improve immunotherapy and survival in melanoma. Nature 2020;577:561-5. [Crossref] [PubMed]
- Hedrick CC, Malanchi I. Neutrophils in cancer: heterogeneous and multifaceted. Nat Rev Immunol 2022;22:173-87. [Crossref] [PubMed]
- Miao L, Qi J, Zhao Q, et al. Targeting the STING pathway in tumor-associated macrophages regulates innate immune sensing of gastric cancer cells. Theranostics 2020;10:498-515. [Crossref] [PubMed]
- Bridges K, Miller-Jensen K. Mapping and Validation of scRNA-Seq-Derived Cell-Cell Communication Networks in the Tumor Microenvironment. Front Immunol 2022;13:885267. [Crossref] [PubMed]
- Mao X, Xu J, Wang W, et al. Crosstalk between cancer-associated fibroblasts and immune cells in the tumor microenvironment: new findings and future perspectives. Mol Cancer 2021;20:131. [Crossref] [PubMed]
- De Martino D, Bravo-Cordero JJ. Collagens in Cancer: Structural Regulators and Guardians of Cancer Progression. Cancer Res 2023;83:1386-92. [Crossref] [PubMed]
- Zeng D, Zhou R, Yu Y, et al. Gene expression profiles for a prognostic immunoscore in gastric cancer. Br J Surg 2018;105:1338-48. [Crossref] [PubMed]
- Guo Y, Zhang Y, Cen K, et al. Construction and validation of a signature for T cell-positive regulators related to tumor microenvironment and heterogeneity of gastric cancer. Front Immunol 2023;14:1125203. [Crossref] [PubMed]
- Zhou X, Fang D, Liu H, et al. PMN-MDSCs accumulation induced by CXCL1 promotes CD8(+) T cells exhaustion in gastric cancer. Cancer Lett 2022;532:215598. [Crossref] [PubMed]
- Zhu ZG, Wang Z, Wu Q, et al. Identification of the CD8 + T-cell Related Signature for Predicting the Prognosis of Gastric Cancer Based on Integrated Analysis of Bulk and Single-cell RNA Sequencing Data. J Immunother 2024;47:239-48. [Crossref] [PubMed]
- Zhao H, Jiang R, Zhang C, et al. The regulatory role of cancer stem cell marker gene CXCR4 in the growth and metastasis of gastric cancer. NPJ Precis Oncol 2023;7:86. [Crossref] [PubMed]
- Yao X, He Z, Qin C, et al. SLC2A3 promotes macrophage infiltration by glycolysis reprogramming in gastric cancer. Cancer Cell Int 2020;20:503. [Crossref] [PubMed]
- Wang X, Liu Z, Du Y, et al. Hsa_circ_0043603 promotes the progression of esophageal squamous cell carcinoma by sponging miR-1178-3p and regulating AADAC expression. Heliyon 2023;9:e19807. [Crossref] [PubMed]
- Su Y, Lin Y, Zhang L, et al. CMTM3 inhibits cell migration and invasion and correlates with favorable prognosis in gastric cancer. Cancer Sci 2014;105:26-34. [Crossref] [PubMed]
- Yuan W, Li T, Mo X, et al. Knockdown of CMTM3 promotes metastasis of gastric cancer via the STAT3/Twist1/EMT signaling pathway. Oncotarget 2016;7:29507-19. [Crossref] [PubMed]
- Yang S, Sun B, Li W, et al. Fatty acid metabolism is related to the immune microenvironment changes of gastric cancer and RGS2 is a new tumor biomarker. Front Immunol 2022;13:1065927. [Crossref] [PubMed]
- Mamidi S, Cinci M, Hasmann M, et al. Lipoplex mediated silencing of membrane regulators (CD46, CD55 and CD59) enhances complement-dependent anti-tumor activity of trastuzumab and pertuzumab. Mol Oncol 2013;7:580-94. [Crossref] [PubMed]
- Dai YH, Tang YP, Zhu HY, et al. ZEB2 promotes the metastasis of gastric cancer and modulates epithelial mesenchymal transition of gastric cancer cells. Dig Dis Sci 2012;57:1253-60. [Crossref] [PubMed]