Identification of DLAT as a potential therapeutic target via a novel cuproptosis-related gene signature for the prediction of liver cancer prognosis
Highlight box
Key findings
• The study identified a novel gene signature related to cuproptosis for predicting liver cancer (LC) prognosis.
• The DLAT gene emerged as a potential therapeutic target.
What is known and what is new?
• LC is a type of tumor with high incidence.
• This study sheds light on cuproptosis-related genes in LC and their relevance to prognosis.
What is the implication, and what should change now?
• Understanding cuproptosis-related genes could provide insights into LC prognosis.
• DLAT may serve as a target for future treatments.
Introduction
Liver cancer (LC), particularly primary LC, poses a significant global health challenge. Its incidence is increasing, and it has a high mortality rate, making it the fourth leading cause of cancer-related deaths worldwide (1). The highest rates of LC incidence and mortality are observed in Africa and East Asia, with notable increases also occurring in parts of Europe and the United States (2). LC has been an important cause of cancer-related mortality in the United States since the early 2000s, with a fast increase, according to the Surveillance, Epidemiology, and End Results (SEER) (3). LC, especially primary LC, develops in people with a significantly higher incidence of cirrhosis, which may limit the patient’s treatment options due to their general health status and liver dysfunction. For early-stage LC, curative treatments such as surgical resection or local ablation methods like radiofrequency ablation (RFA) or microwave ablation (MWA) are preferred. In intermediate stages, transarterial chemoembolization (TACE) or radiation therapy is employed to manage tumor progression and improve survival rates. For advanced stages, systemic treatments, including targeted and immunotherapy, are utilized to relieve symptoms and enhance life quality. These stage-specific recommendations reflect the latest advancements in LC management, aiming to optimize patient outcomes (4,5). Despite significant advancements in hepatocellular carcinoma (HCC) treatment over the past decade, patient prognosis remains poor (6). This is partly due to the fact that most HCC cases are diagnosed at an advanced stage, making surgical or locoregional interventions impractical (7). Therefore, there is an urgent need for new therapeutic targets to improve the clinical outcome of the disease. The creation of precise prognostic models is essential to enable the use of targeted therapies.
Cuproptosis, a unique type of regulated cell death that is different from other oxidative stress-related cell death mechanisms, such as apoptosis, ferroptosis, and necroptosis, was first reported by Tsvetkov et al. (8). In cuproptosis, cell death is triggered by elevated copper levels within cells. Cells reliant on mitochondrial respiration are particularly vulnerable to copper-induced death, suggesting a connection to the tricarboxylic acid (TCA) cycle. Genes responsible for cell death due to copper exposure have been identified, including FDX1 and six others encoding enzymes related to the lipoic acid pathway, such as lipoyltransferase 1 (LIPT1), lipoyl synthase (LIAS), and dihydrolipoamide dehydrogenase (DLD). Protein targets of lipoylation, like the pyruvate dehydrogenase (PDH) complex, were also discovered. FDX1 regulates DLAT lipoylation. Additionally, copper induces DLAT oligomerization, increasing insoluble DLAT levels, which leads to proteotoxic stress and ultimately cell death.
There is growing evidence that copper ions and genes associated with copper ions have a vital role in the progression of LC (9). The risk of developing LC is strongly associated with the high level of copper ions (8,10). Hepatitis and liver cirrhosis are partly triggered by copper ions, and LC is caused by reactive oxygen species (ROS) (11). Moreover, the cuproptosis-related gene FDX1 has a strong association with the occurrence of LC (11). A study has further explored the molecular classification and gene signature of HCC related to cuproptosis, providing experimental verification and highlighting the prognostic value of cuproptosis-related genes in LC (12).
The goal of this study is to identify a novel cuproptosis-related gene signature and evaluate its prognostic value in LC, with a particular focus on DLAT as a potential therapeutic target. We performed an extensive analysis to compare the expression levels of cuproptosis-related genes in normal liver and LC tissues. This study aimed to evaluate their prognostic significance and investigate the connections between cuproptosis and the tumor immune microenvironment. We present this article in accordance with the TRIPOD reporting checklist (available at https://jgo.amegroups.com/article/view/10.21037/jgo-24-609/rc).
Methods
Datasets
We obtained RNA-seq data and clinical characteristics for LC (primary LC) patients from The Cancer Genome Atlas (TCGA) database up to 13 May 2022 (https://portal.gdc.cancer.gov/). The gene expression profiles were standardized using the scaling technique in the “limma” R package.
Identification of differentially expressed cuproptosis-related genes
We obtained 13 cuproptosis-related genes from a previous article (8), which are shown in Table S1. The expression data were normalized to fragments per kilobase per million (FPKM) values before comparison. The “limma” program was utilized to identify differentially expressed genes (DEGs) with a P value below 0.05. DEGs were annotated as * for P<0.05, ** for P<0.01, and *** for P<0.001. A protein-protein interaction (PPI) network for these DEGs was constructed using the Search Tool for the Retrieval of Interacting Genes/Proteins (STRING), version 11.0 (https://string-db.org/).
Development and validation of the prognostic model for cuproptosis-related genes
The predictive efficacy of cuproptosis-related genes was assessed using Cox regression analysis to explore the relationships between each gene and survival status in the TCGA cohort. With a cut-off P value of 0.01, three survival-related genes (DLAT, LIPT1, and ATP7A) were selected for further investigation. The least absolute shrinkage and selection operator (LASSO) Cox regression model (R package “glmnet”) was employed to filter potential genes and develop the prognostic model. The three genes and their coefficients were retained, and the penalty parameter (λ) was determined using the minimum criteria. The risk score formula was as follows: P = Σ7i Xi * Yi (X: coefficients, Y: gene expression level). The LC patients were divided into low- and high-risk subgroups based on the median risk score, and the OS time was compared using Kaplan-Meier (KM) analysis. Principal component analysis (PCA) based on a 3-gene signature was computed using the “prcomp” function in the “stats” R package. Survival curves for the two groups were generated and compared. R packages “survival”, “survminer”, and “time ROC” were used to generate a comparable receiver operating characteristic (ROC) curve to evaluate the accuracy of this model for predicting survival in LC. The area under the curve (AUC) was calculated using a time-dependent ROC curve for 1-, 2-, and 3-year OS. In addition, AUC of the three genes (DLAT, LIPT1, and ATP7A) were calculated separately. We randomly selected 70% of LC patients to validate the model.
Independent prognostic analysis of the risk score
We collected clinical data, including age and grade, from patients in the TCGA cohort. These variables were analyzed alongside the risk score using our regression model. Both univariate and multivariable Cox regression models were employed in the study.
Functional enrichment analysis of the DEGs between the low- and high-risk groups
The LC patients in the TCGA cohort were separated into two subgroups based on the median risk score. We used specific criteria [|log2FC| ≥1 and false discovery rate (FDR) <0.05] to filter DEGs across the low- and high-risk groups. Gene Ontology (GO) and Kyoto Encyclopedia of Genes and Genomes (KEGG) analyses based on these DEGs were performed using the “clusterProfiler” program. The “gsva” program was utilized to perform single sample gene set enrichment analysis (ssGSEA), which calculated the scores of infiltrating immune cells and assessed the activity of immune-related pathways.
Establishment of a nomogram based on risk score and clinical variables
We explored the association between clinical variables and the DEG-based signature. Univariate and multivariate Cox regression analyses were performed to determine if risk scores independently predicted prognosis in LC patients when combined with other clinical factors. A nomogram was constructed using clinical variables and the DEG-based risk score to predict 1-, 3-, and 5-year overall survival (OS) probabilities for LC patients. The predictive utility of the nomogram was assessed using the C-index and calibration curve.
RNA extraction and quantitative real-time polymerase chain reaction
According to the manufacturer’s procedure, total RNA from tissues and cells was isolated using Trizol reagent (Invitrogen, Carlsbad, CA, USA). HiScript II (Vazyme, Nanjing, China) was used to synthesize complementary DNA (cDNA), and quantitative real-time polymerase chain reaction (qRT-PCR) for messenger RNA (mRNA) and microRNA (miRNA) was performed on a StepOne Plus Real-Time PCR machine (Applied Biosystems, Waltham, MA, USA) or a LightCycler 480 (Roche, Indianapolis, IN, USA). Glyceraldehyde 3-phosphate dehydrogenase (GAPDH) was the internal standard control for mRNA detection. The data were analyzed by comparing Ct values after each sample was replicated three times. All PCR primers and short hairpin RNA (shRNA) of DLAT were provided by GeneCopoeia (Guangzhou, China), which are shown in Table S2.
Immune cell infiltration analysis
Given that previous research indicated immune infiltration in tumor cells is involved in cancer progression and correlates with prognosis, we analyzed the infiltration levels of immune cells in low-risk and high-risk groups using CIBERSORT, CIBERSORT-ABS, QUANTISEQ, MCP-counter, XCELL, TIMER, and EPIC algorithms. We also examined the expression of various immune checkpoints (Table S3) to predict the efficacy of immune checkpoint blockade therapy. Additionally, the TIMER database (https://cistrome.shinyapps.io/timer/) was utilized to identify the relationship between immune cells and DEGs, enhancing our understanding of the role of DEGs in LC.
Drug sensitivity analysis
The Genomics of Drug Sensitivity in Cancer (http://www.cancerrxgene.org/downloads) database was used to determine the correlation between DLAT and the sensitivity of various antitumor drugs in cancer cell lines. Additionally, drug sensitivity was represented by half maximal inhibitory concentration (IC50), which is the concentration of a drug needed to inhibit 50% of cancer cells.
Clinical specimens
LC tissues and adjacent normal tissues were collected from patients who underwent surgery in the Affiliated Hospital of Nanjing University of Chinese Medicine/Jiangsu Province Hospital of Chinese Medicine and were diagnosed with LC between January 2021 and May 2022. The tissues were stored in liquid nitrogen immediately after collection. The study was conducted in accordance with the Declaration of Helsinki (as revised in 2013). The study was approved by ethics committee of Affiliated Hospital of Nanjing University of Chinese Medicine (No. 2021-SRFA-329) and informed consent was taken from all the patients.
Cell culture
LC cell lines, including QSG7701, Huh-7, JHH-2, HepG2, Hep3B, SNU-423, and Huh-1, were obtained from the Cell Bank/Stem Cell Bank, Chinese Academy of Sciences. Cells were cultured in Dulbecco’s modified Eagle medium (DMEM) supplemented with 10% fetal bovine serum (FBS), 100 U/mL penicillin, and 100 µg/mL streptomycin (NEST Biotechnology, Wuxi, China). Cells were plated in plastic or glass cell culture dishes and maintained at 37 ℃ in a humidified atmosphere containing 5% CO2. The medium was changed every 2–3 days, and cells were passaged using 0.25% trypsin-EDTA (Gibco, Grand Island, NY, USA) when they reached approximately 80–90% confluence. For experimental assays, cells were seeded at appropriate densities in culture plates or dishes and allowed to adhere overnight before treatment or further analysis.
Immunohistochemistry (IHC)
IHC was performed to assess protein expression in paraffin-embedded LC tissues. Tissue sections (4 µm thick) were deparaffinized in xylene and rehydrated through a graded series of ethanol concentrations. Antigen retrieval was carried out by heating the sections in citrate buffer (pH 6.0) at 95 ℃ for 20 minutes. After cooling to room temperature, the sections were blocked with 5% bovine serum albumin (BSA) for 30 minutes to prevent nonspecific binding. The sections were then incubated overnight at 4 ℃ with primary antibodies specific to the target proteins. After washing with phosphate-buffered saline (PBS), the sections were incubated with biotinylated secondary antibodies for 30 minutes at room temperature, followed by incubation with avidin-biotin-peroxidase complex (ABC) for another 30 minutes. The immunoreactivity was visualized using 3,3’-diaminobenzidine (DAB) as the chromogen, and the sections were counterstained with hematoxylin. Images were captured using an upright microscope system (Nikon, Tokyo, Japan). The integrated optical density (IOD) of the stained sections was measured using Image J software (National Institutes of Health, Bethesda, MD, USA), and the average optical density (AOD) was calculated as AOD = IOD/area.
Transwell migration assay
The Transwell migration assay was conducted to evaluate the migratory ability of LC cells. Briefly, 8-µm pore size Transwell inserts (Corning, NY, USA) were used. For the invasion assay, the inserts were coated with 30 µg of Matrigel [Becton Dickinson (BD) Biosciences, Franklin Lakes, NJ, USA] and allowed to solidify at 37 ℃ for 30 minutes. LC cells were serum-starved overnight in DMEM without FBS and then seeded into the upper chamber of the Transwell inserts at a density of 1×105 cells per well in 200 µL of serum-free DMEM. The lower chamber was filled with 600 µL of DMEM containing 10% FBS as a chemoattractant. After 24 hours of incubation at 37 ℃ in a 5% CO2 atmosphere, the non-migrated cells on the upper surface of the membrane were gently removed with a cotton swab. The migrated cells on the lower surface of the membrane were fixed with 4% paraformaldehyde for 15 minutes, stained with 0.1% crystal violet for 20 minutes, and washed with PBS. The number of migrated cells was counted under an inverted microscope (OLYMPUS IX73, Olympus, Tokyo, Japan) in five randomly selected fields.
Cell viability and colony formation assays
Cell viability assay
Cell viability was assessed using the Cell Counting Kit-8 (CCK-8, Dojindo, Kumamoto, Japan). LC cells were seeded into 96-well plates at a density of 2×103 cells per well in 100 µL of DMEM containing 10% FBS. After various incubation periods (24, 48, 72, and 96 hours), 10 µL of CCK-8 solution was added to each well, and the plates were incubated for an additional 2 hours at 37 ℃. The absorbance at 450 nm was measured using a microplate reader (Bio-Rad, Hercules, CA, USA) to determine cell viability.
Colony formation assay
For the colony formation assay, LC cells were seeded into 6-well plates at a density of 1,000 cells per well and cultured in DMEM with 10% FBS. The medium was changed every 3–4 days. After 10–14 days, when visible colonies had formed, the cells were fixed with 4% paraformaldehyde for 15 minutes and stained with 0.1% crystal violet for 20 minutes. The plates were washed with PBS, and the number of colonies containing at least 50 cells was counted under a microscope.
Statistical analysis
We utilized single-factor analysis of variance to compare gene expression levels between normal liver and LC tissues. For categorical variables, the Pearson chi-square test was employed. The KM method with a 2-sided log-rank test was employed to compare patient OS among subgroups. We analyzed the independent prognostic efficacy of the risk model using univariate and multivariate Cox regression models. The Mann-Whitney test was conducted to compare immune cell infiltration and immune pathway activation between the two groups. All statistical analyses were performed using R software (v4.0.2; R Foundation for Statistical Computing, Vienna, Austria).
Results
Detection of DEGs in LC and normal tissues
We examined the expression of 13 genes associated with cuproptosis in 374 LC samples and 50 normal tissues using TCGA data. We found that 12 genes had significant differential expression (all P<0.05). Among them, 4 genes (DBT, SLC31A1, GCSH, and FDX1) were downregulated, whereas 8 genes (DLST, DLD, PDHB, PDHA1, LIAS, LIPT1, DLAT, and ATP7A) were upregulated in the tumor group. Figure 1A presents heatmaps showing RNA levels of these genes, with green indicating low expression and red indicating high expression. To further explore interactions among these cuproptosis-related genes, we conducted a PPI analysis, as shown in Figure 1B. We identified LIAS, DLST, GCSH, DLAT, LIPT1, DLD, PDHB, PDHA1, DBT, SLC31A1, ATP7A, and ATP7B as hub genes, using a minimum interaction score of 0.9 (highest confidence) for the PPI analysis. Figure 1C displays a correlation network of all the genes related to cuproptosis, with red indicating positive correlations and blue indicating negative correlations.
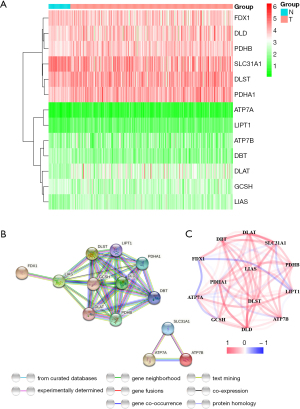
A prognostic gene model was developed using the TCGA cohort
We paired 374 LC samples with samples of patients who had complete survival information. First, we used univariate Cox regression analysis to identify the genes related to survival. Then, we selected three genes (DLAT, LIPT1, and ATP7A) that had a P value of less than 0.01 for further investigation. All of these genes also had a high risk with hazard ratios (HRs) greater than 1 (Figure 2A). The ROC curve of each gene is shown in Figure S1. We created a 3-gene signature based on the LASSO Cox regression analysis (Figure 2B,2C). ATP7A had an AUC of 0.66 at 1-year, 0.561 at 2-year, and 0.563 at 3-year. DLAT had an AUC of 0.695 at 1-year, 0.627 at 2-year, and 0.609 at 3-year. LIPT1 had an AUC of 0.638 at 1-year, 0.558 at 2-year, and 0.588 at 3-year. Among them, DLAT had the best prognostic value for 1, 2, and 3 years. Risk score computational formula = (0.3005 × LIPT1 exp.) + (0.06026 × DLAT exp.) + (0.21313 × ATP7A exp). We divided the 374 patients into low- and high-risk groups based on the median score obtained from the risk score method (Figure 2D). We used PCA to separate the patients with different risks into two clusters (Figure 2E). Patients in the high-risk group (n=158) had more deaths and shorter survival than those in the low-risk group (n=158, Figure 2F, on the right side of the dotted line). The survival time showed a significant difference between the low- and high-risk groups (P<0.05, Figure 2G). In the ROC analysis that considered time dependency, the AUC values were 0.72 for 1-year, 0.60 for 2-year, and 0.62 for 3-year survival (Figure 2H). To ascertain the predictive validity of our 3-gene prognostic model, we employed a simple random sampling (SRS) method to select a subset comprising 70% of the TCGA cohort. This approach ensures that each sample unit has an equal probability of being chosen, thereby maintaining the representativeness and independence of the sample. The characteristic feature of SRS is the complete independence of the selection of each unit, with no fixed pattern or exclusion, ensuring the randomness and fairness of the sample selection. Upon applying the SRS method, we conducted the same statistical analysis on the randomly selected subset. The results demonstrated that this 70% sample maintained consistent AUC values in the time-dependent ROC analysis, with 0.769 and 0.778 for 1-year survival, 0.626 for 2-year survival, and 0.602 for 3-year survival, corroborating the robust predictive capability of our gene signature as reflected in the entire TCGA cohort (Figure S2).
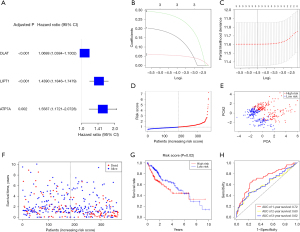
The three genes’ expression and alterations are being validated
We validated the mRNA expression levels (2−ΔΔct) of ATP7A, DLAT, and LIPT1 in 6 LC cell lines (JHH-2, SNU-423, Huh-1, HepG2, Huh-7, and Hep3B) and 1 normal liver cell line (QSG7701) by using PCR (Figure 3A). The expression of the 3 genes was higher in the LC cell lines than in the normal liver cell line. We confirmed the same result in the patient tissues by using IHC (Figure 3B,3C).
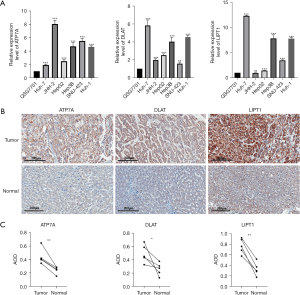
The independent predictive value of the risk model
We conducted both univariate and multivariable Cox regression analyses to determine whether the risk score derived from the gene signature model could independently serve as a prognostic factor. In the TCGA cohorts, the risk score was a significant and independent predictor of poor survival, according to the univariate Cox regression analysis [HR =1.5142, 95% confidence interval (CI): 1.0581–2.1670, Figure 4A]. The multivariable analysis confirmed that the risk score was a prognostic indicator for LC patients (HR =1.4936, 95% CI: 1.0418–2.1411, Figure 4B). We also generated a heatmap of the clinical characteristics for the TCGA cohort (Figure 4C).
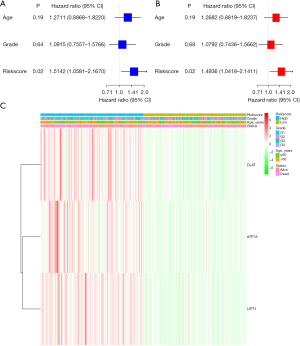
Prognostic signature’s impact on LC progression and subgroup predictive value
We utilized the chi-square test to investigate whether the prognostic signature influenced LC progression and growth. The results (Figure 5) indicated significant differences between high- and low-risk groups in tumor grade (P=0.012), TNM stage (P=0.003), and T stage (P=0.006). Additionally, we conducted stratification analysis to assess the signature’s predictive value across various subgroups. Our findings demonstrated that the DEG-derived model accurately predicted outcomes in males (P=0.007) and the high-risk group (P=0.047). However, its predictive accuracy was limited in other groups (P>0.05) (Figure 6).
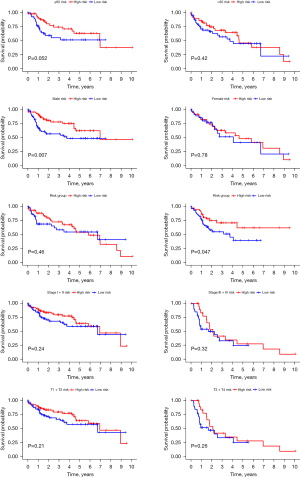
Construction of a nomogram
The nomogram is designed to be a practical tool in the clinical setting, allowing physicians to estimate an individual patient’s prognosis by considering various personalized factors. Its straightforward visual format aids in clear communication with patients regarding their survival probabilities. The reproducibility of this nomogram across different clinical settings is promising, given its incorporation of widely recognized prognostic factors. We used the nomogram to visually assess the survival probability of an individual by combining different prognostic factors. We developed a nomogram that included gender, tumor grade, tumor stage, and risk score to further predict the survival of LC patients. The nomogram showed the estimated survival rates for LC patients at 1, 3, and 5 years (Figure 7A). The calibration plot showed that the actual survival rate matched the estimated value (Figure 7B). The nomogram had an acceptable predictive ability, with a C-index of 0.636.
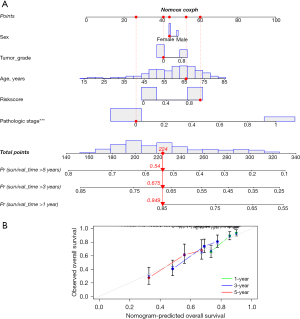
Differential gene expression and pathway analysis in risk model subgroups
We used the R package ‘limma’ to obtain DEGs and further analyzed them to explore changes in gene functions and pathways among the risk model subgroups, based on the criteria of FDR <0.05 and |log2FC| ≥1. In the TCGA cohort, we identified 365 DEGs between the low- and high-risk groups. There were 183 genes that were upregulated and 182 genes that were downregulated in the high-risk group. Then, we performed an analysis of GO enrichment and pathway analysis using the KEGG with the DEGs. The results showed that the DEGs were mainly related to the ECM structure, channel activity, and tumor-related pathways (Figure 8A,8B).
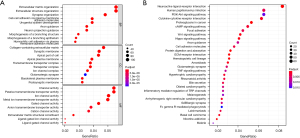
Comparing immunological activation in different subgroups
We used ssGSEA to calculate the enrichment scores of 16 immune cell types and the activity of 13 immune-related pathways in the TCGA cohorts, based on functional analyses. We compared the low- and high-risk groups. In Figure 9A, we saw that mast cells and natural killer (NK) cells were lower in the high-risk group than in the low-risk group. In the TCGA cohort, we found that the high-risk group had higher APC co-stimulation and MHC class I pathway activity than the low-risk group, except for cytolytic activity (Figure 9B). In general, immune cells were lower in high-risk group, which indicated a suppressed immune microenvironment and suggested that cuproptosis might have a close relationship with tumor immunity.
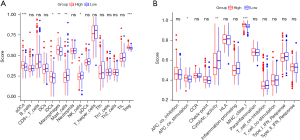
Analysis of the level of immune infiltration using the signature based on DEGs
The heatmap showed the correlation between the signature and immune infiltration by using TIMER, CIBERSORT, CIBERSORT-ABS, XCELL, QUANTISEQ, EPIC, and MCP-counter (Figure S3). We also explored the relationship between risk score and important immune checkpoints (Table S3), considering the importance of checkpoint inhibitor immunotherapies. We observed a significant high expression (P<0.05) of VTCN1, TNFSF18, TNFSF15, TNFSF9, TNFSF4, TNFRSF9, TNFRSF4, NRP1, LGALS9, LAIR1, ICOS, HHLA2, HACVR2, CTLA4, CD276, CD274, CD200R1, CD200, CD86, CD80, and CD44 in the high risk group (Figure 10 and Figure S4).
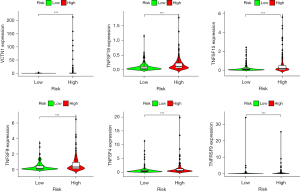
Identification of small molecule drugs
To find potential small molecular drugs based on DEGs, we used the Enrichr database. The top five substances were vitinoin, latamoxef, chlorzoxazone, atrazine, and medrysone, with a significance level of P<0.05 for each one. These compounds based on DEGS analysis have the potential to treat LC. Table 1 shows the details of these substances.
Table 1
Index | Name | P value | Adjusted P value | Odds ratio | Combined score |
---|---|---|---|---|---|
1 | Vitinoin CTD 00007069 | <0.001 | <0.001 | 34.75 | 563.85 |
2 | Latamoxef HL60 DOWN | <0.001 | 0.002 | 16.41 | 188.25 |
3 | Chlorzoxazone HL60 DOWN | <0.001 | 0.045 | 10.43 | 77.56 |
4 | Atrazine CTD 00005450 | <0.001 | 0.045 | 8.05 | 59.35 |
5 | Medrysone HL60 DOWN | <0.001 | 0.045 | 12.72 | 90.67 |
Downregulation of DLAT inhibits LC cell migration and proliferation
We performed a performance assessment of DLAT based on the previous analysis, and found that it had the best predictive significance for 1-, 2-, and 3-year outcomes. Therefore, we investigated the function of DLAT. We checked the expression level of DLAT in TCGA and GTEx databases, and found that tumor tissues had higher DLAT expression than normal tissues (Figure 11A). Moreover, high DLAT expression was linked to poor prognosis (Figure 11B). We used qRT-PCR to measure the transfection efficiency of the cells, and observed that the relative expression level of DLAT was significantly reduced after shRNA transfection (Figure 11C). To further explore the role of DLAT in invasion, we conducted transwell assays. The results showed that the invasion and migration rates of huh-7 and huh-1 cells with shRNA transfection were significantly lower than those of the control-transfected cells (Figure 11D).
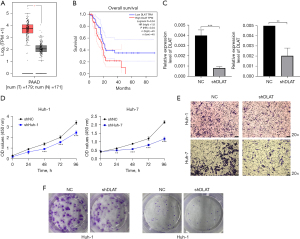
To evaluate the effect of DLAT knockdown on cell proliferation, we conducted CCK-8 assays. The results showed a significant reduction in cell proliferation after DLAT silencing compared to the control group (Figure 11E). Additionally, the colony formation assay demonstrated that DLAT silencing markedly inhibited cell growth (Figure 11F). These findings indicate that DLAT knockdown effectively suppresses the proliferative and invasive abilities of huh-7 and huh-1 cells.
Correlation between DLAT expression and drug resistance
We explored the relationship between the DLAT expression level and the drug IC50 values. We found nine pairs that showed a correlation between drug resistance and the DLAT expression level. These pairs were GSK-2126458 (correlation =−0.430, P<0.001), apitolisib (correlation =−0.387, P=0.002), staurosporine (correlation =−0.380, P=0.003), AT-13387 (correlation =0.369, P=0.004), everolimus (correlation =−0.364, P=0.004), teglarinad (correlation =0.362, P=0.005), CUDC-305 (correlation =0.340, P=0.008), belinostat (correlation =0.338, P=0.008), and daporinad (correlation =0.336, P=0.009) (Figure 12).
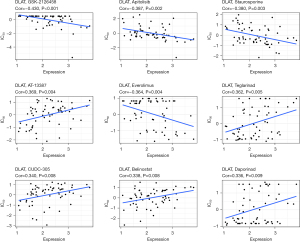
Discussion
The scientific community faces great challenges due to the complexity and heterogeneity of tumors. In recent years, many research studies have used multi-omics strategies, combining information from genomics, transcriptomics, proteomics, metabolomics, and related disciplines, to reveal the diverse and intricate nature of cancer. Sometimes, various factors can impair or cause the failure of the immune system, which is vital for preventing diseases. To improve the understanding and treatment of diseases related to the immune system, such as cancer, researchers have developed new techniques such as immune checkpoint inhibitors, liquid biopsy, and artificial intelligence, which can modulate or enhance the immune response. These technologies have shown promising results in the detection and management of different diseases (13-22).
We identified three key genes (DLAT, LIPT1, and ATP7A) involved in cuproptosis and developed a risk score model that effectively predicts LC patient survival. This model not only provides a novel prognostic tool but also offers potential therapeutic targets. Notably, DLAT emerged as a significant player in LC cell invasion and proliferation, suggesting its potential as a therapeutic target. High-risk patients exhibited fewer mast cells, NK cells, and cytolytic activity, indicating a suppressed immune microenvironment. These findings suggest that cuproptosis influences LC progression and prognosis, highlighting potential therapeutic targets. The clinical implications of these findings are significant, as the risk score model can be used to stratify patients based on their risk, allowing for more personalized treatment approaches. For instance, high-risk patients might benefit from more aggressive treatments or closer monitoring, while low-risk patients could avoid overtreatment. Different mechanisms induced by heavy metals can regulate cell death (10,23,24). Copper accumulation inside cells leads to the formation of lipoylated protein aggregates in mitochondria and destabilization of Fe-S cluster proteins, resulting in cuproptosis (25,26). This challenges the assumption that oxidative stress is the primary driver of metal toxicity and underscores the role of mitochondria in regulating cell death. Our pathway analysis revealed upregulation of several cancer-related pathways, such as the PI3K-AKT, Wnt, and Hippo signaling pathways, in high-risk patients. This suggests that cuproptosis is involved in cancer progression through these pathways, offering new avenues for targeted therapy (27-29). In our study, we examined the expression levels of 13 genes related to cuproptosis in both normal liver tissues and LC tissues. The role and investigation of these genes in LC have not been extensively studied. We observed significant differences in tumor grade, tumor stage, and T stage between low- and high-risk groups based on the correlation analysis between the signature and clinical characteristics. The model also showed good performance for predicting prognosis in males. Furthermore, we identified five small molecules based on DEGs that could be potential drugs for LC. We calculated AUC to determine the best prognostic value of 1-, 2- and 3-year survival and selected DLAT as a candidate for further validation. We confirmed the tumorigenic role of DLAT in LC cells and discovered that DLAT was significantly associated with drug resistance via bioinformatic analysis. Future exploration of the mechanism of how DLAT promotes cancer growth is warranted.
The risk score model, incorporating DLAT, LIPT1, and ATP7A, demonstrated strong predictive ability for LC patient survival in the TCGA cohort. High-risk patients had significantly shorter survival times and more advanced tumor progression. This model can be used to stratify patients based on their risk, allowing for more personalized treatment approaches. For instance, high-risk patients might benefit from more aggressive treatments or closer monitoring, while low-risk patients could avoid overtreatment. Additionally, the model’s ability to correlate clinical attributes and immune cell infiltration provides valuable insights into the tumor microenvironment, suggesting potential immunotherapeutic strategies. Mast cells, which are part of the body’s immune system, play a dual role in cancer. On one hand, they can promote tumor growth by supporting blood vessel formation (angiogenesis) and helping the tumor invade surrounding tissues. On the other hand, they can also fight against tumors by activating a range of immune responses. When mast cells are present in the tumor microenvironment, they can release substances that attract other immune cells, such as T cells, which can attack and destroy cancer cells (30-32). We also noted that the high-risk group had lower levels of mast cells and NK cells, which are important for the immune response against tumors. However, they had higher levels of APC co-stimulation and MHC class I pathways, suggesting potential activation within the tumor microenvironment (33-37). However, it is essential to acknowledge the limitations of the model’s predictive power, as reflected by the relatively modest AUC values. The AUC values, while indicative of the model’s ability to discriminate between events and non-events, are not without their shortcomings. To enhance the model’s predictive capacity, in future studies, it is proposed a multifaceted approach that involves integrating additional features relevant to the target, refining model parameters, and exploring alternative modeling techniques. This comprehensive strategy is designed to improve the discrimination between events and non-events, which is crucial for prognostic models in clinical settings. By adjusting the probability thresholds used for classifying risk categories, we aim to achieve a more nuanced stratification that can better reflect the complexity of tumor biology and patient heterogeneity. These improvements are expected to address the modest AUC values and provide a more robust tool for clinicians to predict patient outcomes in LC.
DLAT was identified as the most important gene in the risk score model for predicting survival. DLAT is part of the PDH complex, which is involved in mitochondrial respiration and the TCA cycle. The PDH complex converts pyruvate into acetyl-CoA, which enters the TCA cycle to produce energy and biosynthetic precursors (8). The progression of cancer, the resistance to chemotherapy, and the treatment of various malignancies, such as HCC, stomach cancer, prostate cancer, lymphocytic leukemia, and others, are associated with DLAT. High levels of DLAT have been identified as an independent prognostic factor for shorter OS in LC patients (38). This suggests that DLAT expression levels could be used to stratify patients based on their prognosis, with higher levels indicating a poorer outcome. DLAT and its related genes are mainly involved in several key biological functions. These include cell metabolism, where DLAT is a critical component of the PDH complex, playing a central role in cellular energy production through the TCA cycle, which is essential for the generation of ATP and biosynthetic precursors necessary for cell growth and proliferation. DLAT has also been implicated in promoting tumor cell invasion and proliferation, with its role in metabolic reprogramming allowing cancer cells to adapt to the high metabolic demands of rapid growth and division. Additionally, DLAT is significantly associated with the level of immune cell infiltration and immune checkpoints in LC, with high DLAT expression correlating with a suppressed immune microenvironment characterized by reduced cytolytic activity and lower levels of immune cells such as NK cells and mast cells (38). These findings highlight the multifaceted role of DLAT in LC biology and underscore its potential as a therapeutic target. By targeting DLAT, it may be possible to modulate the tumor microenvironment and improve patient outcomes.
Copper-induced cell death (cuproptosis) significantly impacts the immune microenvironment, mutation landscape, and biological behavior of LC. Cuproptosis leads to the accumulation of copper within cells, causing mitochondrial dysfunction and the formation of lipoylated protein aggregates. This process disrupts the normal function of immune cells, leading to a suppressed immune microenvironment characterized by reduced mast cells, NK cells, and cytolytic activity (39-41). The mutation landscape of LC is also affected, as copper accumulation can induce genetic instability and mutations. Additionally, cuproptosis influences the biological behavior of LC by promoting tumor cell invasion and proliferation. DLAT plays a crucial role in this process. As a key component of the PDH complex, DLAT is involved in mitochondrial respiration and energy production. Its elevated expression in LC tissues suggests that it may contribute to the metabolic reprogramming of cancer cells, enhancing their survival and proliferation under stress conditions induced by copper accumulation. Furthermore, DLAT’s role in promoting cell invasion and drug resistance highlights its potential as a therapeutic target. By targeting DLAT, it may be possible to modulate the effects of cuproptosis on the immune microenvironment and tumor progression, offering new avenues for LC treatment.
DLAT is a member of the PDH complex, which participates in mitochondrial respiration and the TCA cycle. The PDH complex transforms pyruvate into acetyl-CoA, which joins the TCA cycle to generate energy and biosynthetic precursors (42-45). As a gene related to cuproptosis, we found that it was elevated in LC tissues. The risk model for DLAT also provided us with valuable insights into potential targets for prognosis and treatment of LC. Lipoic acid is transferred from the H-protein of the glycine cleavage pathway to the E2 subunits of 2KDHs by LIPT1, which is a lipoyltransferase-1. Mitochondrial disorders are also caused by LIPT1 mutations, which disrupt the metabolism of lipoic acid (46). The lipoic acid pathway and mitochondrial respiration are associated with the enzyme LIPT1, indicating a strong connection with the TCA cycle, which ultimately leads to cell death caused by copper. A copper transporter that moves copper from enterocytes to the blood is ATP7A, which relocates to the basolateral membrane through a transporter (47,48). The buildup of copper in enterocytes is caused by ATP7A mutation. This impairment leads to copper deficiency in several connective tissues (49). In mice with Menke’s disease, Tsvetkov et al.’s study revealed increased accumulation of copper inside cells; this copper accumulation causes cell death in vivo (8). We have identified three genes (DLAT, LIPT1, and ATP7A) that are involved in cuproptosis in the prognostic model. The risk model based on these three genes can predict the prognosis. However, more research is needed to understand how these genes interact in cuproptosis. Cuproptosis, a newly discovered form of physiological cell death, requires further investigation in the future. For example, Tsvetkov et al. showed that DLAT lipoylation was reduced by the removal of FDX1, suggesting that the lipoylation of proteins is dependent on the cell death caused by copper (8).
The involvement of FDX1 in the lipoylation process is still unclear. Researchers also demonstrated that the binding of copper to lipoylated DLAT increased the formation of DLAT oligomers through disulfide bonds (8). However, they did not explain the link between copper and the formation of disulfide bonds. Despite this, the authors presented a new perspective on the important connection between copper-induced death and mitochondrial metabolism in cells. We found that the DEGs mainly participated in channel-related activities by comparing DEGs among different risk groups. This is consistent with the nature of cuproptosis. In the KEGG pathway analysis, several pathways related to cancer, such as the PI3K-AKT signaling pathway, the wnt signaling pathway, and the hippo signaling pathway, showed upregulation. Therefore, we can reasonably infer that cuproptosis is involved in the progression of cancer.
Cancer immunosurveillance, a vital defense against cancer, involves the recognition and elimination of developing tumor cells by the immune system. However, the immune system can also facilitate the development of tumors. Cancer immunoediting refers to the role of the immune system in both protecting the host and promoting the tumor. In the high-risk group, mast cells and NK cells were at a relatively lower level, whereas aDCs, iDCs, and Treg cells were abundant. This indicates that the immune system of the high-risk population was impaired. The activation of the tumor microenvironment is indicated by the high levels of APC co-stimulation and MHC class I in the high-risk group. However, the high-risk group showed low cytolytic activity, indicating impaired T cell function, which supports tumor growth (50).
The mechanism of cuproptosis, especially in LC, is still poorly understood. We identified 12 genes that showed differential expression in LC and had the ability to regulate cuproptosis. We evaluated the prognostic value of these genes related to cuproptosis in the early stages and provided theoretical support for future research. However, more research is needed to determine whether these regulators, which have been reported in other studies, have similar roles in cuproptosis pathways in LC.
Conclusions
Our results showed that cuproptosis was closely related to LC, as most genes involved in cuproptosis had different expression levels in normal and LC tissues. Furthermore, the score derived from our risk signature based on three genes related to cuproptosis was a reliable predictor of OS in the TCGA cohorts. The DEGs between the high-risk and low-risk groups were related to tumor immunity. We used DEGs to identify small molecules that could treat LC. We discovered that DLAT was a good prognostic marker for LC. We also confirmed the tumorigenic role and drug resistance of DLAT. Our study reveals a novel gene signature for prognostic prediction of LC patients and provides a basis for future research on the link between genes related to cuproptosis and immunology in LC. DLAT will also be a potential target for further investigation.
Acknowledgments
Funding: This study was supported in part by grants from
Footnote
Reporting Checklist: The authors have completed the TRIPOD reporting checklist. Available at https://jgo.amegroups.com/article/view/10.21037/jgo-24-609/rc
Data Sharing Statement: Available at https://jgo.amegroups.com/article/view/10.21037/jgo-24-609/dss
Peer Review File: Available at https://jgo.amegroups.com/article/view/10.21037/jgo-24-609/prf
Conflicts of Interest: All authors have completed the ICMJE uniform disclosure form (available at https://jgo.amegroups.com/article/view/10.21037/jgo-24-609/coif). The authors have no conflicts of interest to declare.
Ethical Statement: The authors are accountable for all aspects of the work in ensuring that questions related to the accuracy or integrity of any part of the work are appropriately investigated and resolved. The study was conducted in accordance with the Declaration of Helsinki (as revised in 2013). The study was approved by ethics committee of Affiliated Hospital of Nanjing University of Chinese Medicine (No. 2021-SRFA-329) and informed consent was taken from all the patients.
Open Access Statement: This is an Open Access article distributed in accordance with the Creative Commons Attribution-NonCommercial-NoDerivs 4.0 International License (CC BY-NC-ND 4.0), which permits the non-commercial replication and distribution of the article with the strict proviso that no changes or edits are made and the original work is properly cited (including links to both the formal publication through the relevant DOI and the license). See: https://creativecommons.org/licenses/by-nc-nd/4.0/.
References
- Llovet JM, Kelley RK, Villanueva A, et al. Hepatocellular carcinoma. Nat Rev Dis Primers 2021;7:6. [Crossref] [PubMed]
- Toh MR, Wong EYT, Wong SH, et al. Global Epidemiology and Genetics of Hepatocellular Carcinoma. Gastroenterology 2023;164:766-82. [Crossref] [PubMed]
- Nautsch F, Ludwig JM, Xing M, et al. Racial Disparities and Sociodemographic Differences in Incidence and Survival Among Pediatric Patients in the United States With Primary Liver Cancer: A Surveillance, Epidemiology, and End Results (SEER) Population Study. J Clin Gastroenterol 2018;52:262-7. [Crossref] [PubMed]
- Yacoub JH, Hsu CC, Fishbein TM, et al. Therapies for hepatocellular carcinoma: overview, clinical indications, and comparative outcome evaluation-part one: curative intention. Abdom Radiol (NY) 2021;46:3528-39. [Crossref] [PubMed]
- Koza A, Bhogal RH, Fotiadis N, et al. The Role of Ablative Techniques in the Management of Hepatocellular Carcinoma: Indications and Outcomes. Biomedicines 2023;11:1062. [Crossref] [PubMed]
- Yu M, Chen Z, Zhou Q, et al. PARG inhibition limits HCC progression and potentiates the efficacy of immune checkpoint therapy. J Hepatol 2022;77:140-51. [Crossref] [PubMed]
- Forner A, Reig M, Bruix J. Hepatocellular carcinoma. Lancet 2018;391:1301-14. [Crossref] [PubMed]
- Tsvetkov P, Coy S, Petrova B, et al. Copper induces cell death by targeting lipoylated TCA cycle proteins. Science 2022;375:1254-61. [Crossref] [PubMed]
- Cobine PA, Brady DC. Cuproptosis: Cellular and molecular mechanisms underlying copper-induced cell death. Mol Cell 2022;82:1786-7. [Crossref] [PubMed]
- Xie J, Yang Y, Gao Y, et al. Cuproptosis: mechanisms and links with cancers. Mol Cancer 2023;22:46. [Crossref] [PubMed]
- Zhang Z, Zeng X, Wu Y, et al. Cuproptosis-Related Risk Score Predicts Prognosis and Characterizes the Tumor Microenvironment in Hepatocellular Carcinoma. Front Immunol 2022;13:925618. [Crossref] [PubMed]
- Chen Z, Du D, Li J, et al. Cuproptosis-related molecular classification and gene signature of hepatocellular carcinoma and experimental verification. Transl Cancer Res 2024;13:1268-89. [Crossref] [PubMed]
- Hoffmann O, Wormland S, Bittner AK, et al. Programmed death receptor ligand-2 (PD-L2) bearing extracellular vesicles as a new biomarker to identify early triple-negative breast cancer patients at high risk for relapse. J Cancer Res Clin Oncol 2023;149:1159-74. [Crossref] [PubMed]
- Wagner B, Dührsen U, Hüttmann A, et al. Genetic Variants of the NKG2C/HLA-E Receptor-Ligand Axis Are Determinants of Progression-Free Survival and Therapy Outcome in Aggressive B-Cell Lymphoma. Cancers (Basel) 2020;12:3429. [Crossref] [PubMed]
- Rohn H, Schwich E, Tomoya Michita R, et al. HLA-G 3' untranslated region gene variants are promising prognostic factors for BK polyomavirus replication and acute rejection after living-donor kidney transplant. Hum Immunol 2020;81:141-6. [Crossref] [PubMed]
- Dhouioui S, Laaribi AB, Boujelbene N, et al. Association of HLA-G 3'UTR polymorphisms and haplotypes with colorectal cancer susceptibility and prognosis. Hum Immunol 2022;83:39-46. [Crossref] [PubMed]
- Babay W, Boujelbene N, Ben Yahia H, et al. Prognostic significance of high circulating sHLA-G in ovarian carcinoma. HLA 2021;98:357-65. [Crossref] [PubMed]
- Zhang S, Yu S, Duan H, et al. Revealing prognostic and tumor microenvironment characteristics of cuproptosis in bladder cancer by genomic analysis. Front Genet 2022;13:997573. [Crossref] [PubMed]
- Li X, Jiang P, Li R, et al. Analysis of cuproptosis in hepatocellular carcinoma using multi-omics reveals a comprehensive HCC landscape and the immune patterns of cuproptosis. Front Oncol 2022;12:1009036. [Crossref] [PubMed]
- Wu G, Hu Q, Chen H, et al. Cuproptosis-related signature predicts prognosis, immunotherapy efficacy, and chemotherapy sensitivity in lung adenocarcinoma. Front Oncol 2023;13:1127768. [Crossref] [PubMed]
- Zhu Z, Zhao Q, Song W, et al. A novel cuproptosis-related molecular pattern and its tumor microenvironment characterization in colorectal cancer. Front Immunol 2022;13:940774. [Crossref] [PubMed]
- Li H, Zu X, Hu J, et al. Cuproptosis depicts tumor microenvironment phenotypes and predicts precision immunotherapy and prognosis in bladder carcinoma. Front Immunol 2022;13:964393. [Crossref] [PubMed]
- Tong X, Tang R, Xiao M, et al. Targeting cell death pathways for cancer therapy: recent developments in necroptosis, pyroptosis, ferroptosis, and cuproptosis research. J Hematol Oncol 2022;15:174. [Crossref] [PubMed]
- Jiang X, Stockwell BR, Conrad M. Ferroptosis: mechanisms, biology and role in disease. Nat Rev Mol Cell Biol 2021;22:266-82. [Crossref] [PubMed]
- Lin CH, Chin Y, Zhou M, et al. Protein lipoylation: mitochondria, cuproptosis, and beyond. Trends Biochem Sci 2024;49:729-44. [Crossref] [PubMed]
- Chen T, Liang L, Wang Y, et al. Ferroptosis and cuproptposis in kidney Diseases: dysfunction of cell metabolism. Apoptosis 2024;29:289-302. [Crossref] [PubMed]
- Bock FJ, Tait SWG. Mitochondria as multifaceted regulators of cell death. Nat Rev Mol Cell Biol 2020;21:85-100. [Crossref] [PubMed]
- Zischka H, Lichtmannegger J, Schmitt S, et al. Liver mitochondrial membrane crosslinking and destruction in a rat model of Wilson disease. J Clin Invest 2011;121:1508-18. [Crossref] [PubMed]
- Ercal N, Gurer-Orhan H, Aykin-Burns N. Toxic metals and oxidative stress part I: mechanisms involved in metal-induced oxidative damage. Curr Top Med Chem 2001;1:529-39. [Crossref] [PubMed]
- Majorini MT, Colombo MP, Lecis D. Few, but Efficient: The Role of Mast Cells in Breast Cancer and Other Solid Tumors. Cancer Res 2022;82:1439-47. [Crossref] [PubMed]
- Dalton DK, Noelle RJ. The roles of mast cells in anticancer immunity. Cancer Immunol Immunother 2012;61:1511-20. [Crossref] [PubMed]
- Khazaie K, Blatner NR, Khan MW, et al. The significant role of mast cells in cancer. Cancer Metastasis Rev 2011;30:45-60. [Crossref] [PubMed]
- Braumüller H, Mauerer B, Andris J, et al. The Cytokine Network in Colorectal Cancer: Implications for New Treatment Strategies. Cells 2022;12:138. [Crossref] [PubMed]
- Mastrogiovanni M, Vargas P, Rose T, et al. The tumor suppressor adenomatous polyposis coli regulates T lymphocyte migration. Sci Adv 2022;8:eabl5942. [Crossref] [PubMed]
- Wilke CM, Kryczek I, Zou W. Antigen-presenting cell (APC) subsets in ovarian cancer. Int Rev Immunol 2011;30:120-6. [Crossref] [PubMed]
- Nanda NK, Birch L, Greenberg NM, et al. MHC class I and class II molecules are expressed in both human and mouse prostate tumor microenvironment. Prostate 2006;66:1275-84. [Crossref] [PubMed]
- Shigematsu Y, Amori G, Tanaka K, et al. MHC class I loss is associated with biliary/progenitor cell features and "cold" tumor-immune microenvironment in hepatocellular carcinoma. Virchows Arch 2023;483:177-86. [Crossref] [PubMed]
- Zhang P, Zhao JH, Yuan LX, et al. DLAT is a promising prognostic marker and therapeutic target for hepatocellular carcinoma: a comprehensive study based on public databases. Sci Rep 2023;13:17295. [Crossref] [PubMed]
- Liu WQ, Lin WR, Yan L, et al. Copper homeostasis and cuproptosis in cancer immunity and therapy. Immunol Rev 2024;321:211-27. [Crossref] [PubMed]
- Mo JQ, Zhang SY, Li Q, et al. Immunomodulation of cuproptosis and ferroptosis in liver cancer. Cancer Cell Int 2024;24:22. [Crossref] [PubMed]
- Zhao R, Sukocheva O, Tse E, et al. Cuproptosis, the novel type of oxidation-induced cell death in thoracic cancers: can it enhance the success of immunotherapy? Cell Commun Signal 2024;22:379. [Crossref] [PubMed]
- Goh WQ, Ow GS, Kuznetsov VA, et al. DLAT subunit of the pyruvate dehydrogenase complex is upregulated in gastric cancer-implications in cancer therapy. Am J Transl Res 2015;7:1140-51. [PubMed]
- Huang X, Gan G, Wang X, et al. The HGF-MET axis coordinates liver cancer metabolism and autophagy for chemotherapeutic resistance. Autophagy 2019;15:1258-79. [Crossref] [PubMed]
- Li C, He C, Xu Y, et al. Alternol eliminates excessive ATP production by disturbing Krebs cycle in prostate cancer. Prostate 2019;79:628-39. [Crossref] [PubMed]
- Mayer RL, Schwarzmeier JD, Gerner MC, et al. Proteomics and metabolomics identify molecular mechanisms of aging potentially predisposing for chronic lymphocytic leukemia. Mol Cell Proteomics 2018;17:290-303. [Crossref] [PubMed]
- Cronan JE. Progress in the Enzymology of the Mitochondrial Diseases of Lipoic Acid Requiring Enzymes. Front Genet 2020;11:510. [Crossref] [PubMed]
- Monty JF, Llanos RM, Mercer JF, et al. Copper exposure induces trafficking of the menkes protein in intestinal epithelium of ATP7A transgenic mice. J Nutr 2005;135:2762-6. [Crossref] [PubMed]
- Ravia JJ, Stephen RM, Ghishan FK, et al. Menkes Copper ATPase (Atp7a) is a novel metal-responsive gene in rat duodenum, and immunoreactive protein is present on brush-border and basolateral membrane domains. J Biol Chem 2005;280:36221-7. [Crossref] [PubMed]
- Myint ZW, Oo TH, Thein KZ, et al. Copper deficiency anemia: review article. Ann Hematol 2018;97:1527-34. [Crossref] [PubMed]
- Sivori S, Pende D, Quatrini L, et al. NK cells and ILCs in tumor immunotherapy. Mol Aspects Med 2021;80:100870. [Crossref] [PubMed]
(English Language Editor: J. Jones)