Application of integrin subunit genes in pancreatic cancer and the construction of a prognosis model
Highlight box
Key findings
• The study identifies a novel eight-gene signature based on key differentially expressed integrin subunit genes (KDE-ITGs) that can predict the prognosis of pancreatic adenocarcinoma (PAAD) patients.
• The identified genes are enriched in pathways such as PI3K-Akt signaling, focal adhesion, and extracellular matrix (ECM)-receptor interaction, which are crucial in cancer progression.
• A prognostic model was established, demonstrating significant associations between the risk groups and overall survival, as well as immune cell infiltration patterns.
What is known and what is new?
• Integrins have been recognized for their role in various cancers, but their prognostic value in PAAD has not been fully explored.
• This manuscript adds a new perspective by constructing a prognostic model for PAAD, highlighting the potential of ITGs as biomarkers for patient survival and therapeutic response.
What is the implication, and what should change now?
• The findings suggest that the prognosis model based on eight identified KDE-ITGs could be used to improve patient stratification and personalized treatment strategies in PAAD.
• These findings may lead to changes in clinical practice by incorporating the analysis of KDE-ITG expression levels into prognostic assessments, potentially enhancing decision-making in treatment planning and immunotherapy approaches for PAAD patients. Future clinical trials are necessary to validate the model’s predictive power and its utility in clinical practice.
Introduction
Pancreatic cancer is an extremely aggressive, common malignant tumor of the digestive system with a poor prognosis (1). Currently, surgical resection is the only treatment that has been shown to improve the survival rate of pancreatic cancer patients (1). Most patients are already in the middle or advanced stages at the time of diagnoses, and have poor treatment outcomes regardless of whether they undergo surgery or chemotherapy (2). The 5-year survival rate of such patients is only about 10% (3). Due to the hidden anatomical location of the pancreas, most patients are asymptomatic in the early stage, or only have some non-specific clinical manifestations, such as epigastric or back pain, nausea, abdominal distension, and jaundice (4). Thus, improving the early diagnosis of pancreatic cancer patients, and developing individualized treatment and management plans are particularly important for improving patient prognosis.
Integrins are transmembrane receptors for cell adhesion to the extracellular matrix (ECM). After binding to the ECM, integrins can organize the cytoskeleton and activate intracellular signals to regulate complex cellular behaviors, including survival, proliferation, migration, and various processes (5). A study has found that integrins are abnormally expressed in many tumors and play a role in tumor cell differentiation, cell migration, proliferation, and tumor angiogenesis (6). Abituzumab and intetumumab (both integrin antibodies) have been shown to improve outcomes in colorectal cancer patients expressing high levels of integrin alpha v beta 6 (αvβ6) (7,8). In addition, the activation of integrins lymphocyte function-associated antigen-1 (LFA-1; αLβ2) and very late antigen-4 (VLA-4; α4β1) enhances T-cell activation and adhesion in the tumor immune microenvironment, thereby enhancing the anti-tumor effect of T cells in mouse models of melanoma and colon cancer (9). Due to their important role in tumorigenesis and development, integrins have emerged as a promising target for cancer therapy (10). Thus, in this study, we used bioinformatics methods to construct a prognostic model of integrin subunit genes (ITGs) to provide potential targets for predicting the prognosis of pancreatic adenocarcinoma (PAAD) patients. We present this article in accordance with the TRIPOD reporting checklist (available at https://jgo.amegroups.com/article/view/10.21037/jgo-24-612/rc).
Methods
Data sources
The RNA-sequencing data of 183 PAAD samples, which contained corresponding count and clinical data, were downloaded from The Cancer Genome Atlas (TCGA) database (https://portal.gdc.Cancer.gov/). As there was one non-primary tumor sample, the remaining 182 samples, which comprised four normal samples and 178 PAAD samples, were used for the subsequent analyses. Due to the small number of PAAD samples in TCGA database, 104 normal pancreas samples marked “well preserved” in the SMPTHNTS field were selected using Genotype-Tissue Expression (GTEx) (https://www.gtexportal.org/home/datasets) for the subsequent analyses.
Moreover, the GSE62452 data set, comprising 65 PAAD samples with complete survival information and 61 adjacent non-tumor tissues samples, were retrieved from the Gene Expression Omnibus (GEO) database (https://www.ncbi.nlm.nih.gov/geo/). Additionally, 30 ITGs were acquired from the published literature of Cui et al. (PMID: 34222028) (11). The study was conducted in accordance with the Declaration of Helsinki (as revised in 2013).
Identification of survival-based subtypes based on the rank score of the ITGs
A single-sample gene set enrichment analysis (ssGSEA) of the 178 PAAD samples from TCGA was conducted to obtain the rank score of the ITGs in each sample. Next, the samples were divided into high and low ITG groups based on the optimal threshold rank score that was computed using the surv-cutpoint function in the survminer package (version 0.4.6). Additionally, the survival probability differences between the two groups were compared by a Kaplan-Meier (K-M) survival analysis after combining the survival information of each sample. The same procedures were employed for the GSE62452 data set.
To determine whether there was significant survival difference in the ITG grouping of the PAAD patients, the correlations between the clinical characteristics (age, gender, race, status, pathological distant metastasis (pM), pathological regional lymph node (pN), pathological stage (pStage), pathological primary tumor (pT), origin, pharmaceutical therapy, radiation therapy, and alcohol use history) and PAAD samples in the two ITG groups were investigated. The correlations were analyzed in the 178 PAAD samples from TCGA data set and validated using the GSE62452 data set.
Differential analyses
As mentioned above, due to the lack of normal pancreas samples in the TCGA data set, normal pancreas samples from the GTEx data set were introduced for the differential analyses. A principal component analysis (PCA) and batch correction analysis were initially conducted with the TCGA and GTEx data sets samples. Next, differential analyses were performed on the PAAD and normal samples in both the TCGA-GETx and GSE62452 data sets to identify the differentially expressed genes (DEGs) in each group using limma based on the following criteria: P<0.05 and |log2 (fold change)| >0.5. An overlap analysis was subsequently conducted on the DEGs of each group using jvenn (http://jvenn.toulouse.inra.fr/app/example.html), and the intersecting DEGs were considered the key DEGs. Moreover, the DEGs between the high and low ITG groups in TCGA and GSE62452 data sets were also obtained individually by limma using the same criteria, these DEGs were further overlapped with the key DEGs to identify the key differentially expressed ITGs (KDE-ITGs) using jveen. Finally, clusterProfiler (version 3.8.1) was used to conduct the Kyoto Encyclopedia of Genes and Genomes (KEGG) enrichment analysis of the KDE-ITGs to identify any common pathways that satisfied the following criteria: P<0.05, and count >1.
Construction of a prognosis model
The 178 PAAD samples in TCGA data set were separated into a training set and an internal validation set based on the ratio of 5:5, with each subset comprising 89 samples, and the GSE62452 data set was used as the external validation set. First, the KDE-ITG expression data were extracted from the 89 samples in the training set and combined with the clinical data. A univariate Cox regression analysis of the combined data was conducted, and a least absolute shrinkage and selection operator (LASSO) analysis of the genes with a P value <0.1 was conducted optimize the prognosis model. The glmnnet package (version 4.0-2) was used to construct a prognosis model with the setting of family parameter as “Cox” to achieve LASSO logistic regression. Further, a 10-fold cross validation was performed to calculate the error rate of different genes. The corresponding genes were selected according to the minimum ramada (λmin) and defined as model genes and included in the prognosis model.
Moreover, to examine the prognostic value of the model, the risk score of every PAAD sample was computed using the risk coefficient obtained by the LASSO analysis and the feature gene expression levels. The formula was expressed as follows: . After separating the PAAD patients into high- and low-risk groups based on the risk score median value, overall survival (OS) curves were plotted for the groups using survminer (version 0.4.8). The efficacy of the risk model was further assessed using the areas under curve (AUCs) of the receiver operating characteristic (ROC) curves. The 1- to 5-year survival time-node ROC curves were plotted for the risk model using the survival ROC package (version 1.16.1). The same procedures were employed using both the internal and external validation (GSE62452) sets to further evaluate the effectiveness of the prognosis model.
Establishment of a nomogram
In addition, to investigate the independent prognosis factors of PAAD, multiple clinical factors (i.e., age, gender, pM, pN, pStage, pT, pharmaceutical therapy, radiation therapy, alcohol use history, Asian, black or African American, white, body of pancreas, head of pancreas, pancreas, and tail of pancreas) and the prognosis model were included in a univariate Cox proportional hazards analysis for independent prognostic assessment. Next, the factors with a P value <0.05 were included in the multivariate Cox proportional hazards analysis (the model was optimized by step function), and the factors with a P value <0.05 were considered independent prognosis factors. Further, a nomogram was established by combining the prognosis model with the independent prognosis factors using rms (version 5.1-4). The discrimination of the nomogram was evaluated by the C-index, and the predictive accuracy of the model was tested by the calibration curves and a decision curve analysis (DCA).
Identification and gene set enrichment analysis (GSEA) of the DEGs between the high- and low-risk groups
To compare the differences between the high- and low-risk groups and investigate the underlying mechanisms, a differential analysis was performed of the high- and low-risk samples in TCGA data set. The selection thresholds of P<0.05 and |log2 (fold change)| ≥0.5 were used to screen the DEGs between the two risk groups, which were defined as the differentially expressed risk genes (DE-RGs). Subsequently, to explore the functions related to the risk groups, the DE-RGs were ranked using the log2 fold change value, and a GSEA was conducted on these genes to identify the enriched GO terms and KEGG pathways using the following selection criteria: P<0.05, and an absolute value of Normalized Enrichment Score (|NES|) >1.
Tumor microenvironment (TME) analysis
The immune infiltration of both the immune and stromal cells in a tumor sample can be obtained using the Estimation of STromal and Immune cells in MAlignant Tumor tissues using Expression data (ESTIMATE) algorithm. The algorithm generated immune scores, stromal scores, and ESTIMATE composite scores, and the differences in the scores between the high- and low-risk groups were compared and visualized in violin plots. Moreover, the proportion of each immune cell was computed by both a ssGSEA and MCPounter, and the proportions between the two risk groups were compared. Finally, statistical calculations were performed to determine the correlations between the model genes and differential immune cells.
Human leukocyte antigen (HLA) genes and immune checkpoints are vital for immune functions. Thus, the expressions of the HLA genes and immune checkpoints in the two risk groups were explored. Next, the expression levels of the immune checkpoints, including indoleamine 2,3-dioxygenase 1 (IDO1), programmed death-ligand 1 (PD-L1, also known as CD274), programmed death-ligand 2 (PD-L2, or PDCD1LG2), T-cell immunoglobulin and mucin domain containing-3 (TIM-3, or HAVCR2), T-cell immunoreceptor with Ig and ITIM domains (TIGIT), cytotoxic T-lymphocyte-associated protein 4 (CTLA-4), programmed death-1 (PD-1, or PDCD1), lymphocyte activation gene 3 (LAG3), inducible co-stimulator (ICOS), and cluster of differentiation 27 (CD27), were compared between the groups. In addition, according to Postow et al. (12), immune checkpoint inhibitors (ICIs) targeting PD-1 and CTLA-4 are related to anti-tumor immunity enhancement, as the tumors escaping immune destruction are associated with the PD-1/PD-L1 and CTLA-4 pathways in cancers. Thus, subclass mapping and the tumor immune dysfunction and exclusion (TIDE) algorithm were used to compare the PD-1 and CTLA-4 data in the high- and low-risk groups.
qPCR validation
Twelve cultured vials with a cell density of 70–90% were divided into four groups, of which HPDE6-C7 were normal cells, and sw1990, panc-1 and bx-pc-3 cells were cancer cells (three cultured vials for each cell line). Cells were each lysed with 1 mL of TRIzol Reagent (Life Technologies, CA, USA), and the total RNA was isolated in accordance with the manufacturer’s instructions. After detecting the concentration and the purity of the RNA, the qualified RNA was reverse-transcribed to complementary DNA (cDNA) using the SureScript-First-strand-cDNA-synthesis-kit (Genecopoeia, Guangzhou, China) before quantitative reverse transcription polymerase chain reaction (qRT-PCR). The qRT-PCR mixture consisted of 3 µL of cDNA, 5 µL of 2× Universal Blue SYBR Green qPCR Master Mix (Servicebio, Wuhan, China), and 1 µL each of forward and reverse primer. The PCR was performed using a BIO-RAD CFX96 Touch TM PCR detection system (Bio-Rad Laboratories, Inc., Hercules, CA, USA) under the following thermal cycling conditions: 40 cycles at 95 °C for 60 s, 95 °C for 20 s, 55 °C for 20 s, and 72 °C for the 30 s. The 2−△△Ct method was used to calculate gene expressions, and Graphpad Prism 5 was applied to plot and calculate the statistical significance of the differences. The primer sequences used in this study are detailed in Table S1.
Statistical analysis
The t-test was employed to compare two groups. The one-way analysis of variance (ANOVA) test was used to compare data among more than two groups, followed by Tukey test for multiple comparisons. Significant P values were denoted as follows: *, P<0.05; **, P<0.01; ***, P<0.001; ****, P<0.0001; ns, non-significant.
Results
Identification of survival-based subtypes based on the rank score of the ITGs
Based on an optimal threshold of 2.705361, the 178 PAAD samples from TCGA data set were divided into the high ITG group, which comprised 139 samples, and the low ITG group, which comprised 39 samples. The K-M curves showed that the survival probability of the low ITG group was significantly higher than that of the high ITG group (P=0.048) (Figure 1A). In the GSE62452 validation set, 37 and 28 samples were allocated to the high and low ITG groups, respectively, based on an optimal threshold of 0.5865573. The PAAD samples in the low ITG group had a significantly higher survival probability than those in the high ITG group (P=0.002) (Figure 1B).
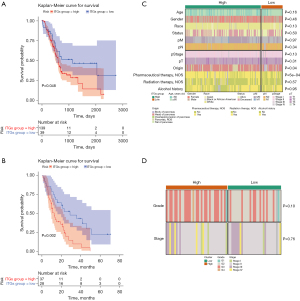
Moreover, the clinical characteristic correlation analysis illustrated that the origin and pharmaceutical therapy distributions differed significantly between the two ITG groups in the TCGA data set (Figure 1C). However, the validation results suggested that there was no significant difference in the distributions of the only two clinical characteristics (i.e., grade and stage) between the two ITG groups (Figure 1D).
Identification of KDE-ITGs and functional annotation
After transformation into transcripts per million (TPM) values in each group, the combination of TCGA and GTEx data sets demonstrated good cluster effects of the normal samples from the GTEx data set and the PAAD samples from the TCGA data set after dimensionality reduction by PCA. Among them, a normal sample in TCGA data set deviated significantly, and was considered an outlier sample and removed. Further, batch effects were found between TCGA and GTEx data sets, and the normal samples in the TCGA data set were well separated from the GTEx samples. After the correction, the normal samples in the two data sets were evenly mixed, indicating that the batch correction effect was good (Figure S1A-S1F).
The differential analysis results revealed that 3,138 DEGs (of which 1,687 were up-regulated, and 1,451 were down-regulated) and 1,422 DEGs (of which 999 were up-regulated, and 423 were down-regulated) were identified in TCGA and GSE62452 data sets, respectively (tables available at https://cdn.amegroups.cn/static/public/jgo-24-612-1.xlsx, https://cdn.amegroups.cn/static/public/jgo-24-612-2.xlsx). Additionally, 131 key DEGs (of which 111 were up-regulated, and 20 were down-regulated) were obtained from the overlap analysis (Figure 2A). In terms of the DEGs between the ITG high and ITG low groups, 5,360 DEGs (of which 4,895 were up-regulated, and 465 were down-regulated) and 617 DEGs (of which 352 were up-regulated, and 265 were down-regulated) were identified in the TCGA and GSE62452 data sets, respectively (tables available at https://cdn.amegroups.cn/static/public/jgo-24-612-3.xlsx, https://cdn.amegroups.cn/static/public/jgo-24-612-4.xlsx). Finally, 22 KDE-ITGs were obtained from the overlap analysis (Figure 2B), which were enriched in eight KEGG pathways, including the phosphatidylinositol 3-kinase (PI3K)-Akt signaling pathway, axon guidance, focal adhesion, the ECM-receptor interaction, small cell lung cancer, the mitogen-activated protein kinase (MAPK) signaling pathway, the Ras-related protein 1 (Rap1) signaling pathway, and the regulation of actin cytoskeleton (Figure 2C).
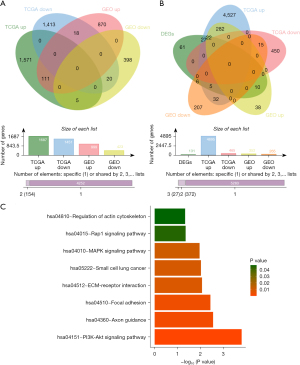
A risk model was built based on the eight model genes
The expression data of the 22 KDE-ITGs were extracted from the training set. After combining these data with OS clinical information, a forest map was generated to display the univariate Cox analysis results, and 19 KDE-ITGs with P values <0.1 were screened out, including epiregulin (EREG), gasdermin-C (GSDMC), family with sequence similarity 83, member A (FAM83A), adaptor protein 1, sigma 3 subunit (AP1S3), family with sequence similarity 83, member D (FAM83D), ephrin-B2 (EFNB2), disc large homolog associated protein 5 (DLGAP5), ephrin-A5 (EFNA5), peptidyl arginine deiminase 1 (PADI1), topoisomerase II alpha (TOP2A), laminin subunit alpha 3 (LAMA3), tripartite motif-containing 29 (TRIM29), mesenchymal epithelial transition factor (MET), integrin subunit alpha-3 (ITGA3), anillin, actin binding protein (ANLN), ankyrin repeat and KH domain-containing protein 2 (AHNAK2), sciellin (SCEL), ceruloplasmin (CP), and galactosaminoglycan (GalNAc)-specific beta-1,3-N-acetylgalactosaminidase 5 (GALNT5) (Figure 3A). Based on the LASSO regression analysis, eight genes (i.e., EREG, FAM83A, DLGAP5, PADI1, LAMA3, MET, CP, and GALNT5) were identified as model genes with the lowest cross validation error (lambda.min =0.0432527) (Figure 3B). The risk score of the eight model genes was calculated as follows: 0.07041298 × exp of EREG + 0.12320185 × exp of FAM83A + 0.14981813 × exp of DLGAP5 − 0.05361542 × exp of PADI1 + 0.26331584 × exp of LAMA3 + 0.20380905 × exp of MET + 0.01074427 × exp of CP − 0.13347795 × exp of GALNT5. Notably, the patients in the low-risk group tended to survive, and the model genes were strongly correlated with the risk score (Figure 3C,3D). The K-M curves illustrated that the low-risk patients had a higher survival probability than the high-risk patients (Figure 3E). Further, the AUCs of the ROC curves in the training set were all greater than 0.7, which suggested that the efficacy of the risk model was good (Figure 3F), and the PCA chart revealed that there were differences between the patients in the two risk groups (Figure 3G).
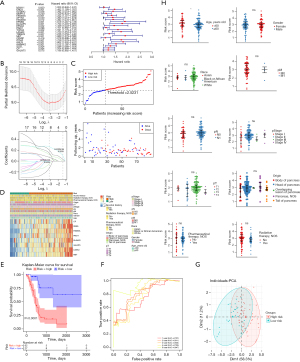
Moreover, the validation results of both the internal and external validation (GSE62452) sets were consistent with those of the training set (Figure S2A-S2E, Figure S3A-S3E). Finally, a significant risk score correlation was only found for one of the clinical characteristics (i.e., age). The patients in <60-year-old subgroup had significantly higher risk scores than those in the ≥60-year-old subgroup (Figure 3H).
The accuracy of nomogram in predicting prognosis
To investigate the independent prognosis factors, multiple clinical factors were included in univariate and multivariate Cox independent prognostic analyses. The p values for radiation therapy, body of pancreas, and risk score were <0.05, and thus these factors were regarded as independent prognosis factors of PAAD (Figure 4A,4B). Further, the ROCs of the final obtained model demonstrated that the AUC values in years one to five all exceeded 0.7 (Figure 4C). A nomogram was also constructed based on the independent prognosis factors and the risk model (Figure 4D). The C-index and corrected C-index of the nomogram were 0.736 and 0.727, respectively, and the calibration curves showed a good consistency between the predicted outcomes of the nomogram and the actual outcomes in terms of 1-, 2- and 3-year OS (Figure 4E). Further, the DCA curves revealed that the net benefit of the original risk model decision changed significantly after the clinical factors were added (Figure 4F).
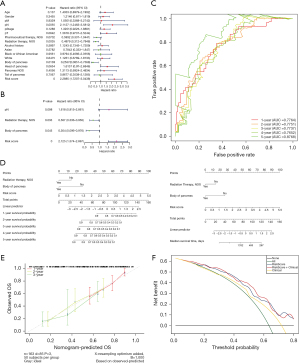
GSEA
In total, 2,371 DE-RGs were found between the two risk groups, of which 2,030 were up-regulated and 341 were down-regulated (Figure 5A,5B). The GSEA results showed that 4,132 GO terms and 202 KEGG pathways were examined, and the top 10 statistically significant enriched terms were visualized (Figure 5C-5F). The main enriched GO biological process (BP) terms included the negative regulation of transcription by RNA polymerase II, the MAPK cascade, and protein polyubiquitination. The main enriched cellular component (CC) terms included the golgi membrane, lysosome, and endosome. The main enriched molecular function (MF) terms included messenger RNA (mRNA) binding, actin binding, endopeptidase activity, and protein kinase activity. In terms of the KEGG pathways, the main enriched pathways included endocytosis, focal adhesion, and tight junction (tables available at https://cdn.amegroups.cn/static/public/jgo-24-612-5.xlsx, https://cdn.amegroups.cn/static/public/jgo-24-612-6.xlsx).
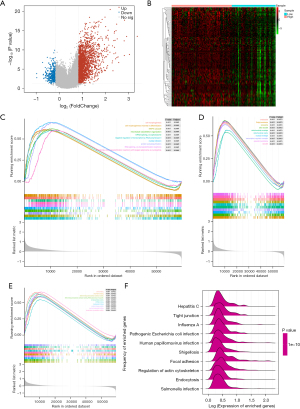
Correlations between the model genes and certain immune cells
First, the results revealed that there was no significant difference between the high- and low-risk groups in terms of the immune scores, stromal scores, or ESTIMATE scores (Figure 6A), and their correlations with the risk score were insignificant (Figure 6B). In addition, the differences in the immune cell proportions calculated by MCPcounter between the risk groups demonstrated that the proportions of T cells, CD8 T cells, cytotoxic lymphocytes, B lineage cells, and neutrophils differed significantly between the risk groups (Figure 6C). The ssGSEA results showed that the differential immune cells were the activated CD4 T cells, activated dendritic cells, CD56 bright natural killer cells, CD56 dim natural killer cells, central memory CD4 T cells, central memory CD8 T cells, eosinophils, gamma delta T cells, monocytes, type 17 T helper cells, and type 2 T helper cells (Figure 6D). Finally, the correlation analysis illustrated that there were significant correlations between various immune cells and the model genes. For example, MET, LAMA, GALNT5, and DLGAP5 were positively correlated with activated CD4 T cells, CD56 bright natural killer cells, central memory CD4 T cells, and type 2 T helper cells. Notably, CP was not correlated with any of the differential immune cells (Figure 6E).
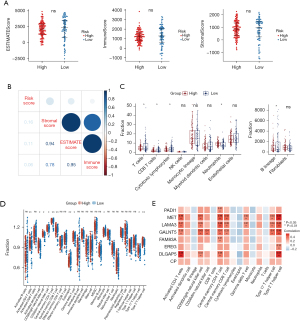
Gene expressions related to immunity and PAAD subtypes
The immune infiltration analysis result revealed that the expression of five HLA genes (i.e., HLA-B, HLA-C, HLA-G, HLA-K, and HLA-V) differed between the risk groups (Figure 7A). Additionally, the expressions of CD274 and LAG3 also differed between the risk groups (Figure 7B). However, the there was no significant difference in the TIDE scores between the risk groups (Figure 7C). The sensitivity comparison results showed that the high-risk group was significantly correlated with CTLA-4R, and the low-risk group was significantly correlated with PD1-R (Figure 7D).
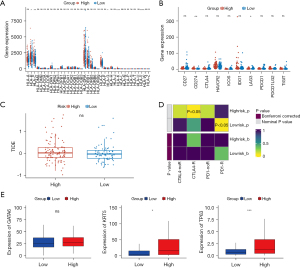
The signature genes of PAAD subtypes (classical and basal-like), including GATA6, KRT5, and TP63, were further analyzed. KRT5 and TP63 were significantly upregulated in the high-risk group (Figure 7E).
RT-qPCR validation of the eight model genes
To further verify the expression levels of the eight model genes (i.e., CP, EREG, PADI1, FAM83A, DLGAP5, GALNT5, LAMA3, and MET), RT-qPCR was performed between the normal cells (HPDE6-C7) and three types of cancer cells (i.e., sw1990, panc-1, and bx-pc-3). The expression levels of the eight model genes differed significantly in the majority of the comparison groups (P<0.05). Consistent with the results of the differential analysis of the TCGA and GEO data sets, the expression levels of all eight genes were significantly higher in the cancer samples than the normal samples. Thus, these eight model genes were considered the prognosis genes of PAAD (Figure 8 and Table S2).
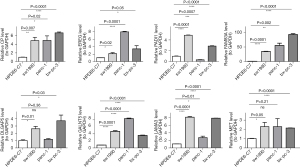
Discussion
Current research indicates that integrins have potential research value in cancer therapy. On one hand, integrin antibodies or antagonists (such as Vitaxin, Echistatin, and Cyclo-RGDfV) can specifically bind to integrins on the surface of cancer cells, thereby inhibiting the interaction between these integrins and the ECM. This mechanism effectively prevents the migration and metastasis of tumor cells. On the other hand, integrins can also serve as targets for immunotherapy by enhancing T cell infiltration and activity to promote anti-tumor immune responses. For example, combining integrin-targeted drugs with immune checkpoint inhibitors (such as PD-1/PD-L1 antibodies) may significantly improve the efficacy of cancer immunotherapy. In addition, some studies have examined the correlation between integrins and the prognosis of different cancers, such as hilar cholangiocarcinoma and gastric cancer (13,14); however, to date, no studies have examined the association between integrins and the prognosis of pancreatic cancer. Thus, we combined the ITGs to construct a prognostic model of pancreatic cancer, thus providing a new direction for the treatment of pancreatic cancer.
In the differential gene analysis, we identified 22 ITGs using an overlap analysis between the normal group and the tumor group, and the high ITG group and the low ITG group. The 22 ITGs were enriched in eight KEGG pathways, the PI3K-Akt signaling pathway, axon guidance, focal adhesion, the ECM-receptor interaction, small cell lung cancer, the MAPK signaling pathway, the Rap1 signaling pathway, and the regulation of actin cytoskeleton. A study has shown that integrin binding to the ECM leads to the activation of endogenous oncogenes and inhibits apoptosis, and this process is closely related to the activation of the PI3K-Akt signaling pathway (5). Integrins and receptor tyrosine kinases co-regulate the activation of a series of mitogenic and pro-survival signals, including the Ras-extracellular signal-regulated kinase (ERK) and PI3K-AKT signaling pathways (15). Focal adhesion (16), the ECM-receptor interaction (17), the MAPK signaling pathway (18), the Rap1 signaling pathway (19), and the regulation of actin cytoskeleton (20) are also closely related to integrins.
We constructed a prognostic model based on eight KDE-ITGs (i.e., EREG, FAM83A, DLGAP5, PADI1, LAMA3, MET, CP and GALNT5). Previous studies have shown that these eight genes are associated with the occurrence and development of tumors (21-28). The EREG is a member of the epidermal growth factor family of proteins. Epiregulin, one of the ligands of EGFR, is expressed at low levels in most normal tissues (29). However, in tumor tissues, the expression of EREG is elevated, and the EGFR signaling pathway is activated to regulate cancer cell proliferation, survival, metastasis, and angiogenesis (29). EREG can also induce epithelial-mesenchymal transition (EMT) in oral squamous cell carcinoma through the Janus kinase 2 (JAK2)/signal transducer and activator of transcription 3 (STAT3) and interleukin-6 (IL-6) signaling pathways (30), and EREG and matrix metalloproteinases-1 (MMP-1) overexpression increases the survival of early breast cancer cells (31). In addition, EREG overexpression causes lung cancer by activating the PI3K/AKT and mitogen-activated protein kinase kinase (MEK)/extracellular signal regulated kinase (ERK) pathways (32). Consistent with the above findings, we found that high EREG expression in pancreatic cancer is a risk factor associated with poor prognosis.
Parameswaran et al. found that FAM83A expression is significantly elevated in human and mouse pancreatic cancer and is critical for pancreatic cancer cell growth and tumorigenesis. In pancreatic cancer cells, increased FAM83A expression was shown to activate MEK/ERK survival signaling and prevent pancreatic cancer cell death (33). Ma et al. also found that the high expression of FAM83A is associated with a poor prognosis in pancreatic cancer patients, which is consistent with the findings of our study (34). In addition, FAM83A stimulates EMT through the PI3K/AKT/Snail pathway in non-small cell lung cancer and can be used as a diagnostic and prognostic marker for non-small cell lung cancer (35). In lung adenocarcinoma, FAM83A induces PD-L1 expression through the ERK signaling pathway to induce tumor immune escape, which is associated with a poor prognosis (36).
DLGAP5, also known as hepatocellular carcinoma up-regulated protein or KIAA0008, is a cell cycle regulatory protein that is mainly involved in a series of biological activities, such as the cell cycle, spindle assembly, and microtubule motor activity (37). The knockdown of DLGAP5 has been shown to suppress cell proliferation, which leads to gap-2 or mitosis (G2/M) phase arrest and apoptosis in ovarian cancer (38). It is related to the occurrence and development of liver cancer (39), pancreatic cancer (40), lung cancer (41), and ovarian cancer (42).
PADI1 is a member of the peptidylarginine deiminase (PAD) family. Research has shown that PAD can post-translationally convert arginine residues into neutrally charged citrulline (43). The deletion of PADI1 has been shown to inhibit tumorigenesis in triple-negative breast cancer (44). The knockdown of PADI1 has been shown to inhibit the migration and invasion of CFPAN-1 and HPAC cells by activating the ERK1/2-p38 signaling pathway (45).
LAMA3 is a gene that is methylated in a variety of tumors (46,47). Abnormally methylated LAMA3 is involved in the occurrence and development of various malignant tumors, such as pancreatic cancer, gastric cancer, head and neck tumors, and lung cancer (46,47).
MET, a proto-oncogene encoding tyrosine kinase receptor c-MET, can induce tumorigenesis, invasion, and migration by activating downstream pathways, such as PI3K/AKT, RAS/ERK/MAPK, and Wnt/β-catenin (48). The aberrant expression of MET has been widely observed in various malignancies, especially non-small cell lung cancer, and hepatocellular carcinoma (49,50).
CP, a multi-copper oxidase, is activated in different pathological conditions, such as infection, inflammation, diabetes, and trauma (51). Elevated serum CP levels have been reported in lung, colon, epithelial ovarian, and cholangiocarcinoma (52-54). Mukae et al. showed that the level of CP expression in urine was positively correlated with the tumor grade and pT stage of non-muscle invasive bladder cancer, and was positively correlated with tumor growth and progression in bladder cancer tissues (27).
GALNT5 encodes a protein that is a membrane-bound polypeptide n-acetyl galactosyltransferase. Detarya et al. found that GALNT5 mediates the occurrence and progression of cholangiocarcinoma by activating AKT/ERK signaling (55).
The PRESENT study showed that the DEGs in the high- and low-risk groups were mainly enriched in endocytosis, focal adhesion, and tight junction, suggesting that the integrin genes may mediate the progression of pancreatic cancer through the above mechanism.
In the immune infiltration analysis, a series of immune cells (e.g., activated dendritic cells, CD56 bright natural killer cells, CD56 dim natural killer cells, and th17 cells) were highly expressed in the low-risk group. The sensitization of dendritic cells, the most powerful antigen-presenting cells, by tumor-associated antigens can enhance the anti-tumor immune response of specific cytotoxic T lymphocytes (56). In most cases, breast cancer (57) and liver cancer (58) patients with a large number of infiltrated dendritic cells in the TME exhibit a more favorable prognosis. Natural killer cells play an anti-cancer role in many tumor types, including head and neck cancer, pharyngeal cancer, and pancreatic cancer (59). In addition, high levels of th17 cells in the TME are associated with a favorable prognosis for ovarian cancer patients (60). Therefore, the collective evidence suggests that the low-risk group may possess a heightened immune-mediated tumor-killing capacity compared to the high-risk group.
Among the classic major histocompatibility complex class I (MHCI) molecules, we found HLA-B was highly expressed in the low-risk group, which suggests the enhanced ability to present the tumor antigen and the stronger immune killing effect in the low-risk group. In addition, the expressions of HLA-G, HLA-K, and HLA-V were significantly down-regulated in the low-risk group of pancreatic cancer patients. As an immune tolerance factor, the expression of HLA-G has been shown to be associated with a poor clinical prognosis in patients with breast cancer, esophageal cancer, gastric cancer, and hepatocellular carcinoma (61). HLA-K and HLA-V have been less studied, but we speculate that they may play a pro-tumor role in pancreatic cancer due to their low expression in the low-risk group.
Immunotherapy with checkpoint inhibitors is a promising therapy for patients with advanced malignant tumors. The differential expression of checkpoints and the treatment sensitivity analysis of the different groups suggested that the high-risk group was sensitive to anti-CD274 and CTLA-4 treatments, while the low-risk group was sensitive to anti-LAG3 and PD1 treatments. These findings provide valuable clues for the immuno-targeted therapy of pancreatic cancer.
PAAD is classified into classical and basal-like subtypes. In the classical subtype, genes related to pancreatic differentiation, particularly GATA6, are highly expressed. This subtype is typically associated with a higher degree of differentiation, exhibiting typical pancreatic glandular characteristics and a better prognosis. In contrast, the basal-like subtype is characterized by high expression of genes related to EMT and stem cell features, such as KRT5 and TP63, which are associated with lower differentiation levels and a poorer prognosis. KRT5 and TP63 are significantly upregulated in the high-risk group, suggesting a closer resemblance of the high-risk group to the basal-like subtype.
In conclusion, we constructed an eight-gene model based on ITGs that effectively predicted the survival and prognosis of pancreatic cancer patients. We also analyzed the immune microenvironment and immune checkpoints, and our findings provide a new direction for the immunotherapy of pancreatic cancer. However, our study still had a number of limitations. Our study was based on data sets from public databases, and employed PCR for validation, more studies are needed to verify its predictive power. Moreover, the mechanisms by which integrins regulate the prognosis of pancreatic cancer need to be further investigated.
Conclusions
The eight identified KDE-ITGs in PAAD were used to establish a new prognosis model, which might have clinical application, especially in immunotherapy.
Acknowledgments
We would like to thank TCGA database for providing the PAAD data set and the GEO database for providing the GSE62452 data set.
Funding: This work was supported by funding from
Footnote
Reporting Checklist: The authors have completed the TRIPOD reporting checklist. Available at https://jgo.amegroups.com/article/view/10.21037/jgo-24-612/rc
Peer Review File: Available at https://jgo.amegroups.com/article/view/10.21037/jgo-24-612/prf
Conflicts of Interest: All authors have completed the ICMJE uniform disclosure form (available at https://jgo.amegroups.com/article/view/10.21037/jgo-24-612/coif). The authors have no conflicts of interest to declare.
Ethical Statement: The authors are accountable for all aspects of the work in ensuring that questions related to the accuracy or integrity of any part of the work are appropriately investigated and resolved. The study was conducted in accordance with the Declaration of Helsinki (as revised in 2013).
Open Access Statement: This is an Open Access article distributed in accordance with the Creative Commons Attribution-NonCommercial-NoDerivs 4.0 International License (CC BY-NC-ND 4.0), which permits the non-commercial replication and distribution of the article with the strict proviso that no changes or edits are made and the original work is properly cited (including links to both the formal publication through the relevant DOI and the license). See: https://creativecommons.org/licenses/by-nc-nd/4.0/.
References
- Cai J, Chen H, Lu M, et al. Advances in the epidemiology of pancreatic cancer: Trends, risk factors, screening, and prognosis. Cancer Lett 2021;520:1-11. [Crossref] [PubMed]
- Mizrahi JD, Surana R, Valle JW, et al. Pancreatic cancer. Lancet 2020;395:2008-20. [Crossref] [PubMed]
- Sheets S.S.F.: Pancreas cancer. Accessed 3/21/2024. Available online: https://seer.cancer.gov/statfacts/html/pancreas.html
- Schmidt-Hansen M, Berendse S, Hamilton W. Symptoms of Pancreatic Cancer in Primary Care: A Systematic Review. Pancreas 2016;45:814-8. [Crossref] [PubMed]
- Liu F, Wu Q, Dong Z, et al. Integrins in cancer: Emerging mechanisms and therapeutic opportunities. Pharmacol Ther 2023;247:108458. [Crossref] [PubMed]
- Chen JR, Zhao JT, Xie ZZ. Integrin-mediated cancer progression as a specific target in clinical therapy. Biomed Pharmacother 2022;155:113745. [Crossref] [PubMed]
- Brzozowska E, Deshmukh S. Integrin Alpha v Beta 6 (αvβ6) and Its Implications in Cancer Treatment. Int J Mol Sci 2022;23:12346. [Crossref] [PubMed]
- Élez E, Kocáková I, Höhler T, et al. Abituzumab combined with cetuximab plus irinotecan versus cetuximab plus irinotecan alone for patients with KRAS wild-type metastatic colorectal cancer: the randomised phase I/II POSEIDON trial. Ann Oncol 2015;26:132-40. [Crossref] [PubMed]
- Slack RJ, Macdonald SJF, Roper JA, et al. Emerging therapeutic opportunities for integrin inhibitors. Nat Rev Drug Discov 2022;21:60-78. [Crossref] [PubMed]
- Li ZH, Zhou Y, Ding YX, et al. Roles of integrin in tumor development and the target inhibitors. Chin J Nat Med 2019;17:241-51. [Crossref] [PubMed]
- Cui K, Wu X, Gong L, et al. Comprehensive Characterization of Integrin Subunit Genes in Human Cancers. Front Oncol 2021;11:704067. [Crossref] [PubMed]
- Postow MA, Callahan MK, Wolchok JD. Immune Checkpoint Blockade in Cancer Therapy. J Clin Oncol 2015;33:1974-82. [Crossref] [PubMed]
- Sun Q, Dong X, Shang Y, et al. Integrin αvβ6 predicts poor prognosis and promotes resistance to cisplatin in hilar cholangiocarcinoma. Pathol Res Pract 2020;216:153022. [Crossref] [PubMed]
- Desnoyers A, González C, Pérez-Segura P, et al. Integrin ανβ6 Protein Expression and Prognosis in Solid Tumors: A Meta-Analysis. Mol Diagn Ther 2020;24:143-51. [Crossref] [PubMed]
- Danen EH, Yamada KM. Fibronectin, integrins, and growth control. J Cell Physiol 2001;189:1-13. [Crossref] [PubMed]
- Huang MW, Lin CD, Chen JF. Integrin activation, focal adhesion maturation and tumor metastasis. Sheng Li Xue Bao 2021;73:151-9. [PubMed]
- Jia B, Yu S, Yu D, et al. Mycotoxin deoxynivalenol affects myoblast differentiation via downregulating cytoskeleton and ECM-integrin-FAK-RAC-PAK signaling pathway. Ecotoxicol Environ Saf 2021;226:112850. [Crossref] [PubMed]
- Sheng W, Chen C, Dong M, et al. Calreticulin promotes EGF-induced EMT in pancreatic cancer cells via Integrin/EGFR-ERK/MAPK signaling pathway. Cell Death Dis 2017;8:e3147. [Crossref] [PubMed]
- Shah S, Brock EJ, Ji K, et al. Ras and Rap1: A tale of two GTPases. Semin Cancer Biol 2019;54:29-39. [Crossref] [PubMed]
- Romero S, Le Clainche C, Gautreau AM. Actin polymerization downstream of integrins: signaling pathways and mechanotransduction. Biochem J 2020;477:1-21. [Crossref] [PubMed]
- Xu J, Lu W. FAM83A exerts tumor-suppressive roles in cervical cancer by regulating integrins. Int J Oncol 2020;57:509-21. [Crossref] [PubMed]
- Cheng WL, Feng PH, Lee KY, et al. The Role of EREG/EGFR Pathway in Tumor Progression. Int J Mol Sci 2021;22:12828. [Crossref] [PubMed]
- Chen M, Zhang S, Wang F, et al. DLGAP5 promotes lung adenocarcinoma growth via upregulating PLK1 and serves as a therapeutic target. J Transl Med 2024;22:209. [Crossref] [PubMed]
- Zhu C, Liu C, Chai Z. Role of the PADI family in inflammatory autoimmune diseases and cancers: A systematic review. Front Immunol 2023;14:1115794. [Crossref] [PubMed]
- Wang Y, Li G, Wang Z, et al. Multi-omics analysis of LAMB3 as a potential immunological and biomarker in pan-cancer. Front Mol Biosci 2023;10:1157970. [Crossref] [PubMed]
- Socinski MA, Pennell NA, Davies KD. MET Exon 14 Skipping Mutations in Non-Small-Cell Lung Cancer: An Overview of Biology, Clinical Outcomes, and Testing Considerations. JCO Precis Oncol 2021;5:PO.20.00516.
- Mukae Y, Ito H, Miyata Y, et al. Ceruloplasmin Levels in Cancer Tissues and Urine Are Significant Biomarkers of Pathological Features and Outcome in Bladder Cancer. Anticancer Res 2021;41:3815-23. [Crossref] [PubMed]
- Detarya M, Lert-Itthiporn W, Mahalapbutr P, et al. Emerging roles of GALNT5 on promoting EGFR activation in cholangiocarcinoma: a mechanistic insight. Am J Cancer Res 2022;12:4140-59. [PubMed]
- Riese DJ 2nd, Cullum RL. Epiregulin: roles in normal physiology and cancer. Semin Cell Dev Biol 2014;28:49-56. [Crossref] [PubMed]
- Wang Y, Jing Y, Ding L, et al. Epiregulin reprograms cancer-associated fibroblasts and facilitates oral squamous cell carcinoma invasion via JAK2-STAT3 pathway. J Exp Clin Cancer Res 2019;38:274. [Crossref] [PubMed]
- Farooqui M, Bohrer LR, Brady NJ, et al. Epiregulin contributes to breast tumorigenesis through regulating matrix metalloproteinase 1 and promoting cell survival. Mol Cancer 2015;14:138. [Crossref] [PubMed]
- Chen KY, Tseng CH, Feng PH, et al. 3-Nitrobenzanthrone promotes malignant transformation in human lung epithelial cells through the epiregulin-signaling pathway. Cell Biol Toxicol 2022;38:865-87. [Crossref] [PubMed]
- Parameswaran N, Bartel CA, Hernandez-Sanchez W, et al. A FAM83A Positive Feed-back Loop Drives Survival and Tumorigenicity of Pancreatic Ductal Adenocarcinomas. Sci Rep 2019;9:13396. [Crossref] [PubMed]
- Ma Z, Zhou Z, Zhuang H, et al. Identification of Prognostic and Therapeutic Biomarkers among FAM83 Family Members for Pancreatic Ductal Adenocarcinoma. Dis Markers 2021;2021:6682697. [Crossref] [PubMed]
- Zhou F, Geng J, Xu S, et al. FAM83A signaling induces epithelial-mesenchymal transition by the PI3K/AKT/Snail pathway in NSCLC. Aging (Albany NY) 2019;11:6069-88. [Crossref] [PubMed]
- Zhou F, Wang X, Liu F, et al. FAM83A drives PD-L1 expression via ERK signaling and FAM83A/PD-L1 co-expression correlates with poor prognosis in lung adenocarcinoma. Int J Clin Oncol 2020;25:1612-23. [Crossref] [PubMed]
- Tang N, Dou X, You X, et al. Pan-cancer analysis of the oncogenic role of discs large homolog associated protein 5 (DLGAP5) in human tumors. Cancer Cell Int 2021;21:457. [Crossref] [PubMed]
- Zhang H, Liu Y, Tang S, et al. Knockdown of DLGAP5 suppresses cell proliferation, induces G(2)/M phase arrest and apoptosis in ovarian cancer. Exp Ther Med 2021;22:1245. [Crossref] [PubMed]
- Tsou AP, Yang CW, Huang CY, et al. Identification of a novel cell cycle regulated gene, HURP, overexpressed in human hepatocellular carcinoma. Oncogene 2003;22:298-307. [Crossref] [PubMed]
- Ke MJ, Ji LD, Li YX. Bioinformatics analysis combined with experiments to explore potential prognostic factors for pancreatic cancer. Cancer Cell Int 2020;20:382. [Crossref] [PubMed]
- Tagal V, Wei S, Zhang W, et al. SMARCA4-inactivating mutations increase sensitivity to Aurora kinase A inhibitor VX-680 in non-small cell lung cancers. Nat Commun 2017;8:14098. [Crossref] [PubMed]
- Chen X, Thiaville MM, Chen L, et al. Defining NOTCH3 target genes in ovarian cancer. Cancer Res 2012;72:2294-303. [Crossref] [PubMed]
- Vossenaar ER, Zendman AJ, van Venrooij WJ, et al. PAD, a growing family of citrullinating enzymes: genes, features and involvement in disease. Bioessays 2003;25:1106-18. [Crossref] [PubMed]
- Qin H, Liu X, Li F, et al. PAD1 promotes epithelial-mesenchymal transition and metastasis in triple-negative breast cancer cells by regulating MEK1-ERK1/2-MMP2 signaling. Cancer Lett 2017;409:30-41. [Crossref] [PubMed]
- Ji T, Ma K, Chen L, et al. PADI1 contributes to EMT in PAAD by activating the ERK1/2-p38 signaling pathway. J Gastrointest Oncol 2021;12:1180-90. [Crossref] [PubMed]
- Pan Z, Li L, Fang Q, et al. Analysis of dynamic molecular networks for pancreatic ductal adenocarcinoma progression. Cancer Cell Int 2018;18:214. [Crossref] [PubMed]
- Ii M, Yamamoto H, Taniguchi H, et al. Co-expression of laminin β3 and γ2 chains and epigenetic inactivation of laminin α3 chain in gastric cancer. Int J Oncol 2011;39:593-9. [PubMed]
- Zhang Y, Xia M, Jin K, et al. Function of the c-Met receptor tyrosine kinase in carcinogenesis and associated therapeutic opportunities. Mol Cancer 2018;17:45. [Crossref] [PubMed]
- Ichimura E, Maeshima A, Nakajima T, et al. Expression of c-met/HGF receptor in human non-small cell lung carcinomas in vitro and in vivo and its prognostic significance. Jpn J Cancer Res 1996;87:1063-9. [Crossref] [PubMed]
- Fu R, Jiang S, Li J, et al. Activation of the HGF/c-MET axis promotes lenvatinib resistance in hepatocellular carcinoma cells with high c-MET expression. Med Oncol 2020;37:24. [Crossref] [PubMed]
- Gitlin JD. Transcriptional regulation of ceruloplasmin gene expression during inflammation. J Biol Chem 1988;263:6281-7. [Crossref] [PubMed]
- Senra Varela A, Lopez Saez JJ, Quintela Senra D. Serum ceruloplasmin as a diagnostic marker of cancer. Cancer Lett 1997;121:139-45. [Crossref] [PubMed]
- Matsuoka R, Shiba-Ishii A, Nakano N, et al. Heterotopic production of ceruloplasmin by lung adenocarcinoma is significantly correlated with prognosis. Lung Cancer 2018;118:97-104. [Crossref] [PubMed]
- Han IW, Jang JY, Kwon W, et al. Ceruloplasmin as a prognostic marker in patients with bile duct cancer. Oncotarget 2017;8:29028-37. [Crossref] [PubMed]
- Detarya M, Sawanyawisuth K, Aphivatanasiri C, et al. The O-GalNAcylating enzyme GALNT5 mediates carcinogenesis and progression of cholangiocarcinoma via activation of AKT/ERK signaling. Glycobiology 2020;30:312-24. [Crossref] [PubMed]
- Banchereau J, Steinman RM. Dendritic cells and the control of immunity. Nature 1998;392:245-52. [Crossref] [PubMed]
- Patente TA, Pinho MP, Oliveira AA, et al. Human Dendritic Cells: Their Heterogeneity and Clinical Application Potential in Cancer Immunotherapy. Front Immunol 2019;9:3176. [Crossref] [PubMed]
- Lu Z, Zuo B, Jing R, et al. Dendritic cell-derived exosomes elicit tumor regression in autochthonous hepatocellular carcinoma mouse models. J Hepatol 2017;67:739-48. [Crossref] [PubMed]
- Habif G, Crinier A, André P, et al. Targeting natural killer cells in solid tumors. Cell Mol Immunol 2019;16:415-22. [Crossref] [PubMed]
- Bilska M, Pawłowska A, Zakrzewska E, et al. Th17 Cells and IL-17 As Novel Immune Targets in Ovarian Cancer Therapy. J Oncol 2020;2020:8797683. [Crossref] [PubMed]
- van de Water RB, Krijgsman D, Houvast RD, et al. A Critical Assessment of the Association between HLA-G Expression by Carcinomas and Clinical Outcome. Int J Mol Sci 2021;22:8265. [Crossref] [PubMed]