Integrating T-cell inflammation features for prognosis in hepatocellular carcinoma: a novel predictive model
Highlight box
Key findings
• A prognostic model for hepatocellular carcinoma (HCC) was developed based on T-cell inflammation features.
What is known and what is new?
• T cell-inflamed environments are associated with immune responses in multiple solid tumors.
• A prognostic model for HCC based on T-cell inflammation features was developed and validated.
What is the implication, and what should change now?
• The findings from this study enhance our understanding of prognosis and treatment strategies for HCC, providing valuable implications for personalized medicine and improved patient outcomes. These insights suggest that individualized treatment approaches could be more effectively tailored based on specific disease markers identified in the study. The implications of these findings are profound, potentially guiding treatment decisions, improving patient outcomes, and improving our comprehension of HCC pathogenesis. Future research could focus on the practical application of these insights and their implications in clinical settings.
Introduction
Liver cancer is the third leading cause of cancer death worldwide, with hepatocellular carcinoma (HCC) being the most common type, accounting for 75% to 80% of primary liver cancer cases (1). HCC is often diagnosed at advanced stages, has limited curative treatment options, and involves a poor prognosis, with up to 70% of patients experiencing recurrence within 5 years, even after surgical or ablative treatments (2).
Small molecule-targeted drugs such as sorafenib and lenvatinib have improved the overall survival (OS) for patients with HCC, but resistance remains a significant challenge (3). Since approval of programmed cell death 1/programmed death-ligand 1 (PD-1/PD-L1) immunotherapy by the US Food and Drug Administration (FDA) approved for HCC in 2017, the effectiveness of immunotherapy has garnered significant attention (4). However, the objective response rate (ORR) of PD-1 immune checkpoint inhibitors (ICIs) in advanced HCC remains unsatisfactorily low, at approximately 15%. This underscores the urgent need for precise screening to identify patients who are most likely to benefit from ICI treatment (5).
A key factor influencing immunotherapy efficacy in HCC is the tumor microenvironment (TME), particularly the presence of T-cell inflammation (TCI), which includes critical elements such as interferon-γ (IFN-γ) signaling, cytotoxic molecules, and antigen presentation (6). Leivonen et al. found that TCI not only affects the efficacy of immunotherapy but can also serve as a predictive biomarker for clinical response (7). Patients with a high TCI phenotype show significantly better survival rates to anti-PD-1 treatment (8).
There are few multivariable prediction models for predicting the response of patients with HCC to immunotherapy. Some of the existing models may not adequately account for the impact of the TCI microenvironment or may have limited applicability across different patient groups (9). Therefore, there is an urgent need to develop more robust predictive models that integrate both genomic and immunological features associated with TCI. Traditional clinicopathological parameters such as grading and staging have limitations in predicting the prognosis of patients with HCC and guiding treatment decisions, underscoring the importance of identifying new prognostic biomarkers. This study’s aim was to clarify the unique role of the TCI microenvironment in HCC and to develop and validate a biomarker-based model capable of predicting the response of patients with HCC to immunotherapy. By integrating genomic and immunological markers associated with TCI and particularly focusing on the role of the KLF2 gene during the model validation phase, we aim to provide a holistic understanding of TCI in the context of HCC through a comprehensive approach. We present this article in accordance with the TRIPOD reporting checklist (available at https://jgo.amegroups.com/article/view/10.21037/jgo-2024-874/rc).
Methods
Data collection and processing
The training set data were obtained from The Cancer Genome Atlas (TCGA), while validation data were sourced from the International Cancer Genome Consortium (ICGC) and the OEP000321 dataset within the Integrative Molecular Database of Hepatocellular Carcinoma (HCCDB) (10,11). The TCGA-Liver Hepatocellular Carcinoma (LIHC) dataset comprises 424 samples, including 374 cancer samples and 50 adjacent normal samples. We excluded patients with an OS time of zero days, resulting in data from 347 patients. For the International Cancer Genome Consortium JP Project (ICGC-LIRI-JP) and OEP000321 datasets, application of the same exclusion criteria yielded data from 203 and 158 patients, respectively. Additionally, we included an HCC single-cell RNA-sequencing (RNA-seq) dataset (GSE242889) from the Gene Expression Omnibus (GEO) database, encompassing cancerous and adjacent noncancerous tissues from five patients with HCC. To assess the predictive power of prognostic markers for immunotherapy response, we also analyzed data from the IMvigor210 cohort, consisting of patients with metastatic urothelial carcinoma (mUC) treated with atezolizumab, processed using the “IMvigor210CoreBiologies” R package (The R Foundation for Statistical Computing, Vienna, Austria) (12). For identifying TCI-related genes, we curated 18 genes from previous studies, including PSMB10, HLA-DQA1, HLA-DRB1, CMKLR1, HLA-E, NKG7, CD8A, CCL5, CXCL9, CD27, CXCR6, IDO1, STAT1, CD274 (PD-L1), CD276 (B7-H3), LAG3, PDCD1LG2 (PD-L2), and TIGIT (13).
Collection and processing of single-cell RNA-seq data
We collected single-cell RNA-seq data of patients with HCC from the GSE242889 dataset (14). The data were analyzed using the “Seurat V4” R package. Initially, we performed quality control by retaining cells with a mitochondrial gene content below 10% and genes expressed in at least three cells within an expression range of 200 to 7,000. Subsequently, the top 2,000 highly variable genes were selected for analysis. To eliminate batch effects among the 10 samples, the “Harmony” R package was employed. Cell clusters were constructed using the FindClusters and FindNeighbors functions and visualized using the t-distributed stochastic neighbor embedding (t-SNE) function. Cells were annotated based on marker genes specific to different cell types. The activity of specific gene sets within each cell was quantified using the ssGSEA function in the “GSVA” R package. The FindMarkers function from the “Seurat V4” R package was used to identify differentially expressed genes (DEGs) between the two groups. DEGs were determined using the Wilcoxon test for statistical significance (adjusted P value <0.05), while other settings were kept at their default values. Genes that showed significant expression differences between cells with high and low TCI scores were regarded as contributors to TCI at the single-cell level. These selected genes were then further examined at the bulk-transcriptome level using weighted gene coexpression network analysis (WGCNA) (15).
Single-sample gene set enrichment analysis (ssGSEA) and gene set enrichment analysis (GSEA)
In this study, we calculated TCI scores for each TCGA-LIHC sample using the ssGSEA method within the gene set variation analysis (“GSVA”) package in R. To uncover signaling pathways potentially linked to TCI, we calculated the GSVA scores for 50 pathways and used the “limma” in R to assess significant differences between the high-risk and low-risk groups.
To further clarify the biological processes (BPs), cellular components (CCs), and molecular functions (MFs) associated with different risk subgroups, we performed GSEA using the “clusterProfiler” package in R on the carbon gene sets (c5.go.v7.5.1.symbols.gmt) between the two risk groups. We adopted the recommended thresholds of false-discovery rate (FDR) <0.25 and |normalized enrichment scores (NES)| >1 as the criteria for significance (16).
WGCNA
In this study, we performed WGCNA using the “WGCNA” package in R, based on bulk RNA-seq data from TCGA-LIHC. First, an optimal soft-thresholding power (β) was determined to meet the requirements for a scale-free network. The weighted adjacency matrix was then converted into a topological overlap matrix (TOM), and the dissimilarity of the TOM (dissTOM) was calculated. Gene clustering and module identification were performed using the dynamic tree-cutting method. Finally, the module exhibiting the highest correlation with the TCI score was identified for further analysis.
Construction of predictive features and survival analysis through integrated machine learning methods
In TCGA bulk RNA-seq data, differential expression analysis was conducted between normal and tumor samples using the “limma” R package, with thresholds set at |log fold change (FC)| >0.585 and an adjusted P value <0.05. The resulting DEGs from the bulk RNA-seq analysis were then intersected with the TCI-related module genes identified via WGCNA. Genes that overlapped at both the bulk and single-cell transcriptomic levels, indicating involvement in TCI, were defined as TCI-related (TCIR) genes.
To develop a robust predictive model with high accuracy, univariate Cox regression analysis was first used to identify HCC TCIR (HTCIR) genes with potential prognostic relevance in the TCGA-LIHC dataset. The ICGC-LIRI-JP and OEP000321 datasets were used for external validation. A total of 101 machine learning models were built using the “Mime” R package, resulting in the final feature, the HCC TCIR score (HTCIRS), for predicting OS in patients with HCC (17). Based on the median HTCIRS risk score, patients from TCGA, ICGC-LIRI-JP, and OEP000321 datasets were categorized into high-risk and low-risk groups. Kaplan-Meier curve analysis was then performed with the “survminer” package in R to identify significant differences in OS, progression-free survival (PFS), and disease-specific survival (DSS) between these groups (log-rank test P<0.05). Furthermore, the “timeROC” R package was used to evaluate the sensitivity and specificity of HTCIRS in predicting OS for patients with HCC via receiver operating characteristic (ROC) curve analysis.
Immune landscape analysis
Using the “IOBR” R package, we collated data related to the cellular characteristics of the TME from a previous study and calculated the enrichment scores for each sample using a standardized approach (18). This allowed for a thorough examination of immunological variations between patients in the high- and low-risk groups. Additionally, we used three approaches—microenvironment cell population (MCP)-counter, ssGSEA, and ESTIMATE—to assess the extent of immune cell infiltration in the samples. We also assessed the correlation of six genes with various immune cells to enhance our understanding of their interactions within the TME (9).
Chemotherapy response and small-molecule drugs
To support personalized treatment strategies, we applied the “pRRophetic” package in R to predict drug sensitivity in patients with HCC based on their HTCIRS risk scores. The pRRophetic tool matches gene expression profiles from patient tissues with those of cancer cell lines to estimate the half-maximal inhibitory concentration (IC50). Differences in IC50 values between high- and low-risk groups were analyzed using the Wilcoxon test, with P<0.05 being set as the threshold for statistical significance (19).
Quantitative reverse-transcription polymerase chain reaction (qRT-PCR) and Western blotting
Total RNA was extracted using TRIzol reagent (Invitrogen, Thermo Fisher Scientific, Waltham, MA, USA) and reverse transcribed into cDNA using the PrimeScript RT reagent Kit (Takara, Japan). qRT-PCR was performed using SYBR Premix Ex TaqTM (Takara Bio, Kusatsu, Japan) according to the manufacturer’s instructions, and gene amplification and detection were carried out using the ABI PRISM 7900 Sequence Detection System (Applied Biosystems, Thermo Fisher Scientific). Proteins were extracted from HCC cells or frozen samples using radio-immunoprecipitation assay (RIPA) buffer and analyzed by Western blotting as previously described (20). The primer sequences are provided in Table S1.
Patients and specimens
In 2024, the Liver Cancer Research Institute of the Affiliated Hospital of Nantong University collected 32 pairs of rapid-frozen HCC samples and matched adjacent nontumor tissue samples from patients with HCC undergoing curative resection. All 32 pairs were included in the qRT-PCR analysis, and 10 pairs were randomly chosen to assess KLF2 protein expression.
Human samples and immunohistochemistry
Ninety primary HCC patient samples were collected by the Department of Hepatic Surgery at the Affiliated Hospital of Nantong University between February 2022 and December 2023 for tissue microarray (TMA) analysis. The inclusion criteria were as follows: (I) no treatment prior to surgery and (II) postoperative pathological confirmation of primary HCC. All participants provided informed consent, and the study received approval from the Ethics Committee of the Affiliated Hospital of Nantong University (No. 2024-L061). The study was conducted in accordance with the Declaration of Helsinki (as revised in 2013).
The construction of the TMA was conducted according to the protocol described in a previous study (21). Briefly, two experienced pathologists evaluated the HCC tissues and corresponding nontumor tissues and marked areas representing tumor tissues on paraffin blocks. Dual-core biopsy samples with a diameter of 1 mm were collected from these designated sites and placed in specific locations in the recipient paraffin block. Sections 4 µm in thickness were then cut and mounted on slides coated with 3-aminopropyltriethoxysilane (Shanghai Biochip Co., Ltd., Shanghai, China). For immunohistochemical (IHC) analysis, tumor sections were selectively stained using an anti-KLF2 antibody (dilution 1:300) as previously detailed (21). Imaging was performed using a standard microscope (Olympus, Tokyo, Japan) or CaseViewer software (3DHISTECH, Budapest, Hungary).
Statistical analysis
All statistical analyses were performed using R software (version 4.1.2). Differences in clinical characteristics between the training and internal validation sets were assessed with the chi-square test. The Wilcoxon test, a nonparametric approach, was used to compare two variables that were not normally distributed. For DEGs, significance was determined using P values adjusted for the FDR. Kaplan-Meier analysis, along with the log-rank test, was used to evaluate the OS differences between subgroups. Spearman correlation was applied to examine associations between risk scores and immune cell infiltration. The t-test was used to analyze qRT-PCR data. A P value of less than 0.05 was considered statistically significant unless otherwise noted.
Results
Characteristics of TCI genes at the single-cell level
We obtained single-cell RNA-seq data from 38,390 cells derived from 10 patients with HCC. Using specific marker genes, we classified the cells into eight major categories: B cells (IGHG3, IGLC2, IGHG1), T cells (CD69, CCL5, IFNG), cycling cells (MKI67, STMN1, TOP2A), endothelial cells (FCN3, AKAP12, CAVIN2), mast cells (TPSB2, TPSAB1, CPA3), myeloid cells (S100A9, C1QB, C1QA), mesenchymal cells (ACAT2, TAGLN, RGS5), and hepatocytes (ALB, APOA2, TTR) (Figure 1A). The classification of these cell types is essential to understanding the complexity of the TME in HCC, as different cell types contribute uniquely to both tumor progression and immune response. Volcano plots were used to display the marker genes for each cell cluster (Figure 1B). To quantify the activity of TCI within different cell types, we used the ssGSEA function in the “GSVA” package to calculate the expression levels of 18 TCI gene sets across all cells (Figure 1C). Among the eight cell types, notable TCI activity was observed in T cells, myeloid cells, and cycling cells (Figure 1D). Cells were categorized into high- and low-TCI groups based on their activity levels, resulting in the identification of 1,206 DEGs between these groups for further analysis (table available at https://cdn.amegroups.cn/static/public/tau-2024-632-1.xlsx). The analysis of DEGs between high- and low-TCI groups helps identify key biomarkers and pathways associated with immune activation and immune evasion, which could potentially serve as therapeutic targets or predictive biomarkers for immunotherapy responses.
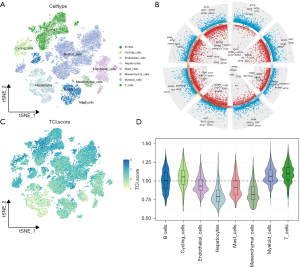
Identification of central modules and TCIR genes in bulk RNA-seq
In this study, the ssGSEA algorithm was employed to calculate TCI activity scores for each TCGA-LIHC sample, providing phenotypic data for subsequent WGCNA. Modules significantly correlated with TCI scores were identified through WGCNA applied to the TCGA-LIHC dataset. Specifically, 1,206 TCIR DEGs from single-cell RNA sequencing were used to construct a coexpression network after outliers were removed (Figure 2A). A soft threshold power of 5 was selected to achieve a scale-free topology. The minimum number of genes per module was set to 60, and MEDissThres, referring to the “Module Eigengenes Dissimilarity Threshold”, was adjusted to 0.25, leading to the identification of six distinct modules (Figure 2B). It was found that the brown module was closely correlated to the TCI scores in single-cell RNA-seq (correlation =0.75; Figure 2C). A scatter plot of gene significance GS versus module membership MM within the brown module showed a strong correlation (correlation =0.83; P<0.001; Figure 2D), indicating that genes in this module may have functional relevance to TCI.
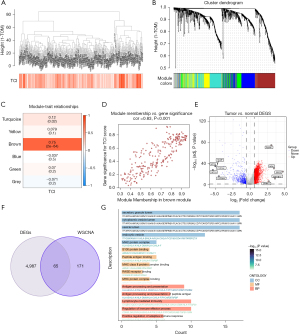
A volcano plot (Figure 2E) was created to visualize the DEGs between tumor and normal liver tissues in TCGA-LIHC bulk RNA-seq dataset (|logFC| >0.585 and adjusted P<0.05). By intersecting the 236 genes from the brown module with the DEGs identified in the bulk RNA-seq analysis, 65 overlapping genes were found (Figure 2F). These genes were designated as HTCIR genes involved in TCI at both the overall and single-cell transcriptomic levels. Gene Ontology (GO) enrichment analysis of HTCIR genes (Figure 2G) showed involvement in BP such as antigen processing and presentation, peptide antigen processing and presentation, and regulation of immune effector processes; CC such as vesicular lumens and major histocompatibility complex (MHC) protein complexes; and MF such as peptide antigen binding and binding to MHC class II or MHC protein complexes. These results suggest that these genes play a key role in immune response and may serve as potential targets for immunotherapy in HCC.
Univariate Cox regression analysis was then conducted on these 65 HTCIR genes, identifying 36 genes with P values less than 0.05 as significant (Table S2). The gene list from TCGA was further cross-referenced with external datasets ICGC-LIRI-JP and OEP000321, with 32 shared genes being extracted (Table S3) for further analysis.
Development of a predictive signature via integrated machine learning
To create a reliable T-cell inflamed related signature (TCIRS), an ensemble approach involving 101 machine learning algorithms was applied to analyze 32 prognostic genes identified through univariate Cox regression. TCGA-LIHC dataset was used as the training set, while ICGC-LIRI-JP and OEP000321 served as validation sets. Through use of a 10-fold cross-validation framework, 101 predictive models were fitted within the training set, and the concordance index (C-index) for both the training and validation sets were calculated, as shown in Figure 3A [Random Survival Forest (RSF) model: C-index 0.787; CoxBoost + RSF model: C-index 0.782; lasso + RSF model: C-index 0.78].
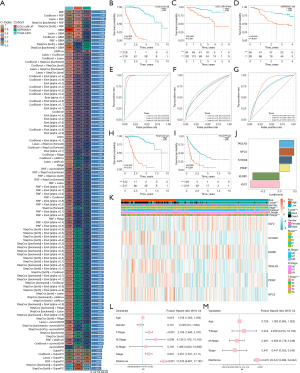
With this model, risk scores for each patient across the three cohorts were calculated, and all patients were categorized into high- or low-risk groups based on the median risk score. In all three datasets, patients in the high-risk group exhibited significantly lower OS compared to those in the low-risk group (log-rank P<0.001) (Figure 3B-3D). ROC curve analysis showed that in the TCGA-LIHC dataset, the TCIRS achieved area under the curve (AUC) values of 0.97, 0.98, and 0.98 at 1, 2, and 3 years, respectively. In the ICGC-LIRI-JP validation set, the AUCs were 0.68, 0.72, and 0.67, respectively. while in the OEP000321 dataset, they were 0.82, 0.73, and 0.74. respectively (Figure 3E-3G). Similarly, progression-free interval (PFI) and DSS were significantly higher in the low-risk group than in the high-risk group (log-rank P<0.001) (Figure 3H,3I). From the 101 models, the one with the highest average C-index was chosen, which consisted of six genes as follows: KLF2 × −0.3671386 + KLRB1 × –0.3387989 + PRNP × 0.1274045 + S100A9 × 0.1599944 + NPC2 × 0.1870806 + TAGLN2 × 0.1873979 (Figure 3J).
The association between HTCIRS and various clinical characteristics was assessed. In the TCGA-LIHC dataset, significant differences in T stage, M stage, and overall stage were found between high-risk and low-risk groups (Chi-squared test P<0.05). The expression patterns of the six genes in these groups are shown in the heatmap in Figure 3K. Univariate and multivariate Cox regression analyses were also conducted to determine whether the risk score could act as an independent prognostic factor. Compared to other common clinicopathological parameters, the risk score for patients with HCC was demonstrated to be an independent prognostic predictor (Figure 3L,3M).
Potential molecular mechanisms related to HTCIRS in bulk transcriptomics
To clarify the molecular mechanisms linked to HTCIRS and HCC prognosis, a functional enrichment analysis was performed. The GSEA based on GO gene sets revealed that the high-risk group showed enrichment in regulation of chromosome segregation, condensed chromosome centromeric region, and nuclear chromosome (Figure 4A). In contrast, the low-risk group showed enrichment in alpha amino acid catabolic process, amino acid catabolic process, monocarboxylic acid catabolic process, and organic acid catabolic process (Figure 4B). GSVA analysis revealed that the high-risk group had increased activity in pathways like hallmark G2M checkpoint, hallmark MYC target V1, hallmark E2F targets, and hallmark glycolysis, whereas the low-risk group was more active in hallmark bile acid metabolism, hallmark fatty acid metabolism, and hallmark xenobiotic metabolism (Figure 4C). The correlation analysis between HTCIRS and hallmark pathway scores further supported these findings (Figure 4D), suggesting that HTCIRS is closely related to cancer-associated BPs and metabolic pathways. To evaluate the association between hallmark pathways and HCC prognosis, Kaplan-Meier curve analysis was conducted. The results showed that pathways positively correlated with HTCIRS, such as glycolysis, were linked to poor prognosis (Figure 4E). Conversely, pathways inversely correlated with HTCIRS, including fatty acid metabolism, were linked to a favorable prognosis (Figure 4F). These results indicate that the activation or inhibition of specific pathways may result in distinct prognostic outcomes across HTCIRS risk subgroups.
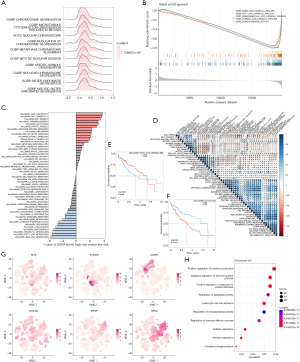
To investigate the role of HTCIRS in the TME at the single-cell transcriptomic level, we analyzed the expression patterns of KLF2, S100A9, KLRB1, TAGLN2, PRNP, and NPC2 across different cell types (Figure 4G). The results showed that these genes were mainly expressed in immune cells, including T cells, myeloid cells, and B cells. Subsequently, cells were categorized into high- and low-risk score groups, and differential analysis was performed. GO enrichment analysis of DEGs identified multiple BPs significantly enriched in the studied gene set. Notably, significant enrichment was observed in the regulation of immune effector processes and leukocyte cell-cell adhesion, indicating that these genes may be involved in modulating immune responses (Figure 4H) (22).
Relationship of HTCIRS with the tumor-immune microenvironment
Through the “IOBR” package in R, a comprehensive analysis of the TME of HCC was conducted. It was revealed that patients with low HTCIRS exhibited significantly higher levels of immune cell infiltration, including for T cells, B cells, and macrophages, compared to those with high HTCIRS, indicating a higher level of immune activation in the low-HTCIRS group (Figure 5A). Immune cell infiltration levels in the samples were quantified using MCP-counter, ssGSEA, and ESTIMATE methods. The correlations between the six genes and different immune cell types were also calculated (Figure 5B). To evaluate immune infiltration in LIHC samples, the ESTIMATE algorithm was used to compute immune, stromal, ESTIMATE, and tumor purity scores for HTCIRS risk subgroups. The findings revealed that the high-risk group had significantly elevated immune and ESTIMATE scores, while tumor purity in the high-risk group was notably lower compared to that in the control group (Figure 5C-5F).
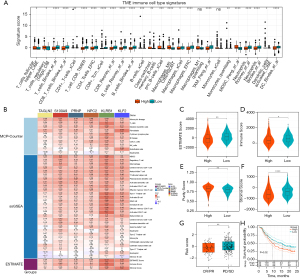
To comprehensively evaluate the role of HTCIRS in immunotherapy for HCC, a systematic analysis was performed. Initially, a detailed analysis of the IMvigor-210 cohort was conducted based on comprehensive prognostic and treatment-related information of the patient population. The results showed that the prognosis was better in the low-risk group, indicating greater benefits from immunotherapy. The distribution of HTCIRS across various response levels showed that HTCIRS scores were significantly lower in the response group [complete response (CR) or partial response (PR)] than in the nonresponse group [progressive disease (PD) or stable disease (SD)] (P<0.05; Figure 5G,5H). Further assessment of drug sensitivity across the different HTCIRS risk groups revealed that small-molecule inhibitors (e.g., gefitinib, axitinib, and erlotinib) were significantly more effective in the low-HTCIRS group (all P values <0.05; Figure S1A-S1C). In contrast, the high-risk group showed greater sensitivity to chemotherapeutic agents (e.g., gemcitabine, doxorubicin, and docetaxel) (Figure S1D-S1F). Overall, these findings suggest that patients with high HTCIRS may respond better to chemotherapy, whereas those with low HTCIRS could benefit more from targeted therapies or immunotherapy. Recent studies have shown that capecitabine has significant anti-tumor efficacy in HCC patients who have failed sorafenib treatment, particularly in metronomic schedules, and significant objective radiological responses have been observed in some cohort studies (23,24). These studies further support chemotherapeutic agents as a treatment option for HCC patients who fail immunotherapy. Given the higher sensitivity to chemotherapeutic agents such as gemcitabine observed in the high-risk group, we suggest that future research further explore the use of chemotherapy drugs, especially in patients who have failed tyrosine kinase inhibitors and ICIs.
Validation and identification of key-molecule KLF2 in the HTCIRS index
To further confirm the role of the HTCIRS index in HCC, KLF2, the gene with the highest coefficient in the index, was chosen for additional investigation. The expression of KLF2 messenger RNA (mRNA) was initially assessed in 32 pairs of HCC samples and matched nontumor liver tissues (Figure 6A). The results indicated that KLF2 mRNA expression was significantly higher in the adjacent nontumor tissues compared to the tumor tissues (P<0.001). Western blot analysis performed on 10 pairs of HCC tumors and adjacent nontumor liver tissues showed similar results (Figure 6B).
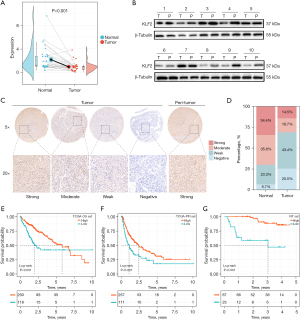
To further explore the biological characteristics of KLF2 at the clinical level, TMAs were constructed with tumor tissues and corresponding normal liver tissues from 90 patients with HCC, which was followed by IHC staining. The results demonstrated that KLF2 expression was strong or moderate in 70.0% of the adjacent nontumor tissues (63/90) (Figure 6C,6D). In TCGA-LIHC cohort, low expression of KLF2 was associated with poor prognosis (P<0.001) (Figure 6E); similarly, in the PFI outcomes, low KLF2 expression was also associated with adverse outcomes (P<0.001) (Figure 6F). In our TMA cohort, low KLF2 expression was significantly linked to more advanced clinical staging and a greater number of tumors (Table S4), whereas high KLF2 expression was strongly associated with longer postoperative OS (P<0.001) (Figure 6G).
These findings highlight the importance of KLF2 as a potential prognostic marker in HCC and support its use in assessing tumor aggressiveness and patient prognosis.
Discussion
This study used a TCI gene expression profile of 18 genes associated with IFN-γ signaling, cytotoxic effector molecules, antigen presentation, and T cell-activating cytokines as a reflection of TME characteristics to evaluate ICI response. The HTCIRS was used to stratify patients with HCC by risk and to evaluate their sensitivity to immunotherapy and first-line treatments, including tyrosine kinase inhibitors and chemotherapeutic agents. Results showed that the low-risk group had notable immune activation and increased sensitivity to small molecule-targeted therapies.
In contrast to earlier research that has concentrated only on prognostic value, our study integrated single-cell level analysis with bulk transcriptomics to reveal unique expression patterns across different cell types in the TME, such as liver cancer cells, immune cells, and stromal cells (25). This identified multiple potential biomarkers and therapeutic targets that play key roles in HCC progression and treatment response, supporting the value of the HTCIRS and validating it for prognosis, small-molecule drug sensitivity, and immunotherapy efficacy.
Among the genes included in the HTCIRS, most are closely related to immune responses or tumor progression. The expression of KLF2, which had the highest coefficient in the HTCIRS, is primarily regulated by hypermethylation, indicating poor prognosis in HCC. KLF2 is a transcription factor that plays a major role in the nucleus and is extensively involved in regulating cell development, differentiation, survival, and other key BPs (26). In oral squamous cell carcinoma, KLF2 regulates genes related to the cell cycle and apoptosis, such as promoting the expression of cell cycle inhibitors, thereby inhibiting tumor cell proliferation (27). Single-cell level expression analysis showed the high expression of KLF2 in immune cells. Our study suggests that KLF2 is a potential protective factor in HCC.
In addition, our study found that HTCIR genes such as KLRB1, NPC2, PRNP, S100A9, and TAGLN2 also play significant roles in HCC, which is consistent with findings in the existing literature. KLRB1, a C-type lectin receptor, has been shown to play a crucial role in T-cell activation and immune responses. It has been suggested that KLRB1 may be involved in regulating the immune microenvironment in various malignancies, including HCC (28). NPC2, a gene involved in lipid metabolism, is particularly associated with processes related to cell membranes and endosomes. NPC2 has been implicated in liver cancer cell metabolism and plays a role in shaping the immune microenvironment. Suk et al. suggest that NPC2 may influence tumor progression by modulating lipid metabolism, and its dysregulation has been linked to malignancies, including HCC (29). Additionally, NPC2 could serve as a novel therapeutic target for HCC treatment. PRNP, primarily known for its involvement in the nervous system, has more recently been associated with immune evasion mechanisms in tumors. Research has shown that PRNP promotes tumor progression in HCC by facilitating immune escape. Its expression in HCC has been linked to poor prognosis and advanced disease stages, making it a potential target for therapeutic interventions aimed at disrupting immune evasion in tumors (30). S100A9, an immune-related gene, has been extensively studied in HCC. It plays a critical role in inflammatory responses and is involved in immune evasion. S100A9 is associated with the regulation of various immune cells and cytokines, contributing to tumor immune escape and progression. Elevated expression of S100A9 in HCC has been found to correlate with poor prognosis, further supporting its potential as a therapeutic target (31). Finally, TAGLN2, a key player in extracellular matrix remodeling, has been associated with tumor invasiveness and metastasis. Leung et al. suggest that TAGLN2 influences the immune microenvironment, promoting tumor growth and metastasis in HCC. This makes TAGLN2 a promising target for therapeutic strategies aimed at inhibiting tumor progression and immune evasion in HCC (32). The expression patterns of these genes in HCC not only deepen our understanding of tumor immune evasion mechanisms but also provide important insights for the development of new immune therapy targets and prognostic biomarkers for HCC.
This study involved certain limitations, including the lack of extensive transcriptomic and single-cell data from our HCC samples which limited our ability to directly associate findings with specific pathological or molecular features such as liver disease background (e.g., viral vs. non-viral chronic liver diseases) and liver reserve function. The absence of detailed clinical information regarding liver disease history and liver reserve function restricted our capacity to fully evaluate how these factors might influence immunotherapy responses. Future research with larger sample size and datasets to further validate and expand these findings is recommended. Additionally, functional experiments and clinical trials would help in clarifying the practical application of the HTCIRS in HCC prognosis and treatment, particularly considering the variability in treatment responses related to different liver disease backgrounds and liver function status.
Conclusions
This study developed a HTCIRS based on extensive single-cell transcriptomics and bulk-transcriptomics data. The results indicated that HTCIRS can effectively predict patient survival and recurrence risk, demonstrating high clinical utility. Further experimental validation demonstrated that the transcription factor KLF2 is pivotal in regulating the immune microenvironment of HCC, serving as a protective factor that lowers the risk of HCC development and progression. These findings offer a new theoretical basis and potential targets for personalized treatment strategies in HCC, providing valuable support for clinical decision-making and management in the era of precision medicine.
Acknowledgments
Funding: This study was supported by
Footnote
Reporting Checklist: The authors have completed the TRIPOD reporting checklist. Available at https://jgo.amegroups.com/article/view/10.21037/jgo-2024-874/rc
Data Sharing Statement: Available at https://jgo.amegroups.com/article/view/10.21037/jgo-2024-874/dss
Peer Review File: Available at https://jgo.amegroups.com/article/view/10.21037/jgo-2024-874/prf
Conflicts of Interest: All authors have completed the ICMJE uniform disclosure form (available at https://jgo.amegroups.com/article/view/10.21037/jgo-2024-874/coif). The authors have no conflicts of interest to declare.
Ethical Statement: The authors are responsible for all aspects of the work to ensure that any issues related to the accuracy or integrity of any part of the work are appropriately investigated and resolved. The study was conducted in accordance with the Declaration of Helsinki (as revised in 2013). All samples were acquired with informed consent from the patients, and the study protocol received approval from the Ethics Committee of the Affiliated Hospital of Nantong University (No. 2024-L061).
Open Access Statement: This is an Open Access article distributed in accordance with the Creative Commons Attribution-NonCommercial-NoDerivs 4.0 International License (CC BY-NC-ND 4.0), which permits the non-commercial replication and distribution of the article with the strict proviso that no changes or edits are made and the original work is properly cited (including links to both the formal publication through the relevant DOI and the license). See: https://creativecommons.org/licenses/by-nc-nd/4.0/.
References
- Huang DQ, Singal AG, Kanwal F, et al. Hepatocellular carcinoma surveillance - utilization, barriers and the impact of changing aetiology. Nat Rev Gastroenterol Hepatol 2023;20:797-809. [Crossref] [PubMed]
- Zhang X, El-Serag HB, Thrift AP. Predictors of five-year survival among patients with hepatocellular carcinoma in the United States: an analysis of SEER-Medicare. Cancer Causes Control 2021;32:317-25. [Crossref] [PubMed]
- Manfredi GF, Celsa C, John C, et al. Mechanisms of Resistance to Immunotherapy in Hepatocellular Carcinoma. J Hepatocell Carcinoma 2023;10:1955-71. [Crossref] [PubMed]
- Tümen D, Heumann P, Gülow K, et al. Pathogenesis and Current Treatment Strategies of Hepatocellular Carcinoma. Biomedicines 2022;10:3202. [Crossref] [PubMed]
- Nakatsu G. Toward a postbiotic era of microbiome science: Opportunities to advance immunotherapies for hepatocellular carcinoma. J Gastroenterol Hepatol 2022;37:34-8. [Crossref] [PubMed]
- Garris CS, Luke JJ. Dendritic Cells, the T-cell-inflamed Tumor Microenvironment, and Immunotherapy Treatment Response. Clin Cancer Res 2020;26:3901-7. [Crossref] [PubMed]
- Leivonen SK, Pollari M, Brück O, et al. T-cell inflamed tumor microenvironment predicts favorable prognosis in primary testicular lymphoma. Haematologica 2019;104:338-46. [Crossref] [PubMed]
- Ott PA, Bang YJ, Piha-Paul SA, et al. T-Cell-Inflamed Gene-Expression Profile, Programmed Death Ligand 1 Expression, and Tumor Mutational Burden Predict Efficacy in Patients Treated With Pembrolizumab Across 20 Cancers: KEYNOTE-028. J Clin Oncol 2019;37:318-27. [Crossref] [PubMed]
- Song F, Wang CG, Mao JZ, et al. PANoptosis-based molecular subtyping and HPAN-index predicts therapeutic response and survival in hepatocellular carcinoma. Front Immunol 2023;14:1197152. [Crossref] [PubMed]
- Chen D, Gao J, Ren L, et al. A signature based on NKG2D ligands to predict the recurrence of hepatocellular carcinoma after radical resection. Cancer Med 2023;12:6337-47. [Crossref] [PubMed]
- Jiang Z, Wu Y, Miao Y, et al. HCCDB v2.0: Decompose Expression Variations by Single-cell RNA-seq and Spatial Transcriptomics in HCC. Genomics Proteomics Bioinformatics 2024;22:qzae011. [Crossref] [PubMed]
- Chi H, Zhao S, Yang J, et al. T-cell exhaustion signatures characterize the immune landscape and predict HCC prognosis via integrating single-cell RNA-seq and bulk RNA-sequencing. Front Immunol 2023;14:1137025. [Crossref] [PubMed]
- Ayers M, Lunceford J, Nebozhyn M, et al. IFN-γ-related mRNA profile predicts clinical response to PD-1 blockade. J Clin Invest 2017;127:2930-40. [Crossref] [PubMed]
- Li K, Zhang R, Wen F, et al. Single-cell dissection of the multicellular ecosystem and molecular features underlying microvascular invasion in HCC. Hepatology 2024;79:1293-309. [Crossref] [PubMed]
- Liu J, Shi Y, Zhang Y. Multi-omics identification of an immunogenic cell death-related signature for clear cell renal cell carcinoma in the context of 3P medicine and based on a 101-combination machine learning computational framework. EPMA J 2023;14:275-305. [Crossref] [PubMed]
- Song F, Lu CL, Wang CG, et al. Uncovering the mechanism of Kang-ai injection for treating intrahepatic cholangiocarcinoma based on network pharmacology, molecular docking, and in vitro validation. Front Pharmacol 2023;14:1129709. [Crossref] [PubMed]
- Liu Z, Liu L, Weng S, et al. Machine learning-based integration develops an immune-derived lncRNA signature for improving outcomes in colorectal cancer. Nat Commun 2022;13:816. [Crossref] [PubMed]
- Geeleher P, Cox NJ, Huang RS. Clinical drug response can be predicted using baseline gene expression levels and in vitro drug sensitivity in cell lines. Genome Biol 2014;15:R47. [Crossref] [PubMed]
- Gao C, Chang L, Xu T, et al. AKR1C1 overexpression leads to lenvatinib resistance in hepatocellular carcinoma. J Gastrointest Oncol 2023;14:1412-33. [Crossref] [PubMed]
- Li J, Hsu HC, Mountz JD, et al. Unmasking Fucosylation: from Cell Adhesion to Immune System Regulation and Diseases. Cell Chem Biol 2018;25:499-512. [Crossref] [PubMed]
- Harkus U, Wankell M, Palamuthusingam P, et al. Immune checkpoint inhibitors in HCC: Cellular, molecular and systemic data. Semin Cancer Biol 2022;86:799-815. [Crossref] [PubMed]
- Naimi A, Mohammed RN, Raji A, et al. Tumor immunotherapies by immune checkpoint inhibitors (ICIs); the pros and cons. Cell Commun Signal 2022;20:44. [Crossref] [PubMed]
- Trevisani F, Brandi G, Garuti F, et al. Metronomic capecitabine as second-line treatment for hepatocellular carcinoma after sorafenib discontinuation. J Cancer Res Clin Oncol 2018;144:403-14. [Crossref] [PubMed]
- Granito A, Marinelli S, Terzi E, et al. Metronomic capecitabine as second-line treatment in hepatocellular carcinoma after sorafenib failure. Dig Liver Dis 2015;47:518-22. [Crossref] [PubMed]
- Mao J, Song F, Zhang Y, et al. Development and validation of a chromatin regulator signature for predicting prognosis hepatocellular carcinoma patient. J Gastrointest Oncol 2024;15:397-414. [Crossref] [PubMed]
- Chen XQ, Ma J, Xu D, et al. Comprehensive analysis of KLF2 as a prognostic biomarker associated with fibrosis and immune infiltration in advanced hepatocellular carcinoma. BMC Bioinformatics 2023;24:270. [Crossref] [PubMed]
- Kou Y, Zhang Y, Rong X, et al. Simvastatin inhibits proliferation and promotes apoptosis of oral squamous cell carcinoma through KLF2 signal. J Oral Biosci 2023;65:347-55. [Crossref] [PubMed]
- Fang S, Zhou Y. Deciphering the role of KLRB1: a novel prognostic indicator in hepatocellular carcinoma. BMC Gastroenterol 2024;24:210. [Crossref] [PubMed]
- Suk FM, Wang YH, Chiu WC, et al. Secretory NPC2 Protein-Mediated Free Cholesterol Levels Were Correlated with the Sorafenib Response in Hepatocellular Carcinoma. Int J Mol Sci 2021;22:8567. [Crossref] [PubMed]
- Wang X, Chen D, Shi Y, et al. Copper and cuproptosis-related genes in hepatocellular carcinoma: therapeutic biomarkers targeting tumor immune microenvironment and immune checkpoints. Front Immunol 2023;14:1123231. [Crossref] [PubMed]
- Zhong C, Niu Y, Liu W, et al. S100A9 Derived from Chemoembolization-Induced Hypoxia Governs Mitochondrial Function in Hepatocellular Carcinoma Progression. Adv Sci (Weinh) 2022;9:e2202206. [Crossref] [PubMed]
- Leung WK, Ching AK, Chan AW, et al. A novel interplay between oncogenic PFTK1 protein kinase and tumor suppressor TAGLN2 in the control of liver cancer cell motility. Oncogene 2011;30:4464-75. [Crossref] [PubMed]
(English Language Editor: J. Gray)