The expression and prognostic value of GLYATL1 and its potential role in hepatocellular carcinoma
Introduction
Hepatocellular carcinoma (HCC) accounts for the majority of primary liver cancers and is one of the most malignant tumors and the third leading cause of cancer-related mortality worldwide (1). There are various treatments for HCC, including radical or palliative hepatectomy, intraoperative radiofrequency ablation, transarterial chemoembolization, and other targeted drugs. Among these treatments, radical or palliative hepatectomy is still the most effective strategy of HCC management (2). Though some HCC patients have the opportunity for surgical resection, long-term survival remains poor because of the high rates of recurrence and vascular tumor emboli (3,4). Moreover, the molecular mechanism underlying HCC recurrence remains unclear. Thus, it is significant for us to identify a molecular target that can be regarded as a prognostic indicator for HCC patients.
Glycine-N-acyltransferase (GLYAT) plays an important role in the detoxification of endogenous and exogenous acyl-CoA. In mammals, various carboxylic acid derivatives are combined with an amino acid, and the resulting polypeptide increases water solubility and can be excreted through urine, which occurs mainly in both the liver and kidney (5,6). This process involves mainly the following processes. First, carboxylic acid and coenzyme A activate ATP-dependent carboxylic acid, and then coenzyme A ligase forms Gatley, which is the intermediate product of acyl-CoA. Finally, under the action of GLYAT, the acyl group is transferred to the glycine amino group. Glycine-N-acyltransferase-like 1 (GLYATL1), one of the members of GLYAT that constitutes a gene cluster, is located in the cytoplasm (specifically, the mitochondrion). GLYATL1 was first identified at the mRNA and cellular level in 2007 (7) and encodes an enzyme involved in the catalysis of arylacetyl transfer (8). In contrast to GLYAT, GLYATL1 exerts phenylacetyl-CoA glutamine N-acyltransferase activities in xenobiotic metabolism (9). GLYATL1 is involved not only in normal physiological metabolism but also in tumorigenesis. A previous study demonstrated that the mRNA expression of GLYATL1 was unregulated by androgen and ETS variant transcription factor 1 (ETV1) in prostate cancer (10). Moreover, Kishimoto and colleagues showed that the protein expression of GLYATL1 in HCC tissues was lower than that in non-tumor tissues (9). However, few studies have performed comprehensive analyses of GLYATL1 in cancers. We aimed to evaluate the expression and prognostic value of GLYATL1 and explore the mechanism underlying the connection between GLYATL1 and cancers.
In the present study, we took advantage of data available in public databases to investigate the expression level and prognostic significance of GLYATL1 in different cancers. To better understand the prognostic value and underlying mechanisms of GLYATL1 in HCC, we also investigated the association between its expression level and clinicopathologic features. Moreover, a protein network of predicted associations for GLYATL1 was constructed. Next, we conducted a functional analysis of GLYATL1 in cancers. Finally, we explored the correlation between GLYATL1 and genes involved in glutamine metabolism in HCC. Thus, we hypothesize that GLYATL1 catalyzes glutamine to affect mitochondrial glutamine metabolism, further inhibiting the rapid proliferation of HCC. In summary, our study sheds light on the expression and prognostic value of GLYATL1 across cancers and provides a potential regulatory mechanism underlying the development of HCC.
We present the following article in accordance with the Materials Design Analysis Reporting (MDAR) reporting checklist (available at: http://dx.doi.org/10.21037/jgo-20-186).
Methods
Oncomine database analysis
Oncomine (http://www.oncomine.org) is a web-based cancer microarray database and data-mining platform containing 715 databases and 86,733 samples (11). Thus, we can utilize it to compare differential expression between cancer and normal tissues. In our study, we used the Oncomine database to investigate mRNA levels in different cancer and corresponding non-tumor tissues. The parameters were set as follows: gene: GLYATL1; and data type: mRNA. Other cut-off values were as follows: P value: <1e-4; fold change: >2; and gene rank: top 10%.
UALCAN cancer database analysis
The UALCAN cancer database (http://ualcan.path.uab.edu/index.html) is a comprehensive and systematic web resource that provides publicly available cancer OMICS data, including data from The Cancer Genome Atlas (TCGA) (12). In our study, we first confirmed the expression of GLYATL1 across cancers in the TCGA. We further investigated the GLYATL1 promoter methylation profile based on its clinicopathologic characteristics, including sample type, individual cancer stage, tumor grade, and nodal metastasis status. The level of DNA methylation was indicated by the β-values. The cut-off values of hypermethylation and hypomethylation were defined as 0.7 and 0.3, respectively (13).
PrognoScan database analysis
PrognoScan (http://dna00.bio.kyutech.ac.jp/PrognoScan/index.html) was used to investigate associations between the expression level of GLYATL1 and survival time in patients with different cancers. PrognoScan is a new database that collects all cancer microarray datasets from public databases, including ArrayExpress, and provides researchers a convenient approach for confirming the prognostic value of genes. Overall survival (OS) was used as the study endpoint.
Kaplan-Meier plotter database analysis
We can readily extract RNA-seq data from multiple public databases via Kaplan-Meier plotter (14) (https://kmplot.com/analysis/). We conducted a survival analysis across 21 cancers by plotting Kaplan-Meier curves and compared their differences by the log-rank p-value based on the mRNA RNA-seq data of GLYATL1 from the TCGA. We further used the RNA-seq data of GLYATL1 and the corresponding clinical information from the TCGA to investigate the associations between the expression level and clinicopathologic features in HCC.
Gene Expression Omnibus (GEO) database analysis
We downloaded a gene microarray with survival data (GSE116174) from the GEO (https://www.ncbi.nlm.nih.gov/geo/) to confirm the prognostic value and clinical correlation of GLYATL1 in HCC. The GSE116174 cohort was divided into a high expression group and a low expression group according to the median GLYATL1 expression level. Survival analysis was conducted as described above to investigate the correlation between GLYATL1 expression and clinical features. Then, univariate Cox analysis was used to identify survival-associated variables (P<0.15), which were sequentially subjected to multivariate Cox analysis to investigate independent prognostic factors (P<0.05).
Tumor Immune Estimation Resource (TIMER) database analysis
TIMER (https://cistrome.shinyapps.io/timer/) is a web resource that can help researchers investigate the associations between tumor cells and six immune cell types, including B cells, CD4+ T cells, CD8+ T cells, neutrophils, macrophages and dendritic cells, based on 10,897 samples from 32 cancer types in the TCGA (15). First, a gene module was used to investigate the correlation between GLYATL1 expression and the abundance of immune infiltrates. Then, a survival module was chosen to explore the associations between clinical characteristics and immune infiltration or GLYATL1 expression in a multivariable Cox proportional hazards model. Finally, we utilized a correlation module to identify the correlation between GLYATL1 and key genes involved in glutamine metabolism, including solute carrier family 1, member 5 (SLC1A5), solute carrier family 7, member 11 (SLC7A11), glutaminase (GLS), glutaminase 2 (GLS2), solute carrier family 38, member 5 (SLC38A5), glutamate dehydrogenase 1 (GLUD1), glutamic-oxaloacetic transaminase 1 (GOT1) and glutamic-oxaloacetic transaminase 2 (GOT2). Partial correlation was conditioned on tumor purity.
Search Tool for the Retrieval of Interacting Genes (STRING) database analysis
We constructed protein-protein interaction (PPI) networks of coregulated hub genes via the STRING database (version 11.0), which contains 5,090 total organisms, 24,584,628 proteins and 3,123,056,667 total interactions (https://string-db.org/) (16). The parameters were set as follows: protein name: GLYATL1; and organism: Homo sapiens. A combined score >0.4 suggested a statistically significant interaction, and the top 10 genes were screened as hub genes. Next, the interactions were exported to Cytoscape software (version 3.7.1) for visual presentation. To determine the potential biological functions of the hub genes, Gene Ontology (GO) and Kyoto Encyclopedia of Genes and Genomes (KEGG) pathway analyses of the 10 coregulated hub genes were performed. Only the top 15 GO enrichment pathways [including biological process (BP), cellular component (CC), and molecular function (MF) terms] and the top five KEGG pathways were listed and visualized using a bubble diagram.
Gene set enrichment analysis (GSEA)
GSEA can be used to analyze and interpret genome-wide expression profiles based on biological knowledge (17). The RNA-seq data of HCC patients of the TCGA downloaded from Genomic Data Commons (https://portal.gdc.cancer.gov/) were used to investigate the potential mechanism underlying the development of HCC by GSEA (17). The patients were divided into two groups according to the median mRNA level of GLYATL1: the low expression group and the high expression group. The parameters were set as follows: gene sets database: h. All. V7.0 Symbols. gmt (Hallmarks); number of permutations: 1,000; and permutation type: phenotype. Significantly enriched pathways and genes were defined as those with a P value <0.05 and a false discovery rate (FDR) <0.25. These criteria were used to minimize the occurrence of false-positive results in multiple tests.
Gene Expression Profiling Interactive Analysis (GEPIA)
GEPIA (http://gepia.cancer-pku.cn/), which contains RNA sequencing expression data from the TCGA and Genotype-Tissue Expression (GTEx) project, is an enhanced web server for interactive analyses based on a standard processing pipeline (18). First, we utilized GEPIA to further confirm the mRNA expression of GLYATL1 in human cancers. Then, GEPIA was used to confirm the gene association between GLYATL1 and genes involved in glutamine metabolism. GLYATL1 was set as the X-axis, and genes related to glycolysis were set as the Y-axis. Then, we calculated the correlation coefficient in HCC and normal liver tissues from the TCGA.
Statistical analysis
We utilized SPSS software (version 20.0) and the GEO2R portal (https://www.ncbi.nlm.nih.gov/geo/info/geo2r.html) to conduct the analysis of GSE116174 cohort. The correlations between GLYATL1 and key genes involved in glutamine metabolism were indentified by Spearman’s correlation analysis. Except for the Oncomine database analysis and GEO database analysis, a P value less than 0.05 was considered significant in the present study.
The study was conducted in accordance with the Declaration of Helsinki (as revised in 2013).
Results
mRNA expression level of GLYATL1 across different cancer and corresponding non-tumor tissues
We first utilized the Oncomine database to investigate the mRNA expression level of GLYATL1 across different cancers. As shown in Figure 1A, the GLYATL1 mRNA levels were significant in four cancers: colorectal cancer, kidney cancer, liver cancer, and prostate cancer. Among these cancers, GLYATL1 was upregulated in colorectal cancer and prostate cancer and downregulated in kidney cancer and liver cancer. Next, the expression of GLYATL1 was verified in the UALCAN cancer database. The results revealed a significant difference in GLYATL1 expression between tumor and normal tissues in breast invasive carcinoma (P<1e-12), cholangiocarcinoma (P=3.53e-05), colon adenocarcinoma (P=1.11e-16), kidney chromophobe carcinoma (P=2.38e-04), clear cell renal cell carcinoma (P=5.67e-07), kidney renal papillary cell carcinoma (P=2.01e-06), HCC (P=1.62e-12), lung adenocarcinoma (P=1.7e-12), lung squamous cell carcinoma (P=1.84e-12), prostate adenocarcinoma (P=1.62e-12), rectal adenocarcinoma (P=5.55e-16), and uterine endometrial carcinoma (P=3.28e-8) (Figure 1B). Considering that there are few normal samples in the TCGA, we integrated the data on normal tissues from the GTEx database and the data on tumor tissues from the TCGA to analyze the expression differences between human tumor tissues and their matched normal tissues. As shown in Figure S1, further validation with the GEPIA database also indicated similar results.
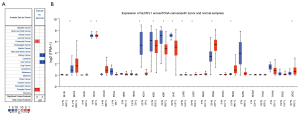
Prognostic effects of GLYATL1 mRNA expression in patients with different cancers
As shown in Table S1, PrognoScan revealed that high GLYATL1 mRNA expression levels indicated poor OS of patients with breast cancer (HR =1.73, P=0.004) and skin melanoma (HR =2.51, P=0.036) but improved OS of patients with renal cell carcinoma (HR =0.20, P=0.040), lung adenocarcinoma (HR =0.56, P=0.034), and colorectal cancer (HR =0.47, P=0.016). To further confirm the association between GLYATL1 mRNA expression and the prognostic value in patients with different cancers, the mRNA RNA-seq data of GLYATL1 in 21 cancers from the TCGA and survival information were used to plot Kaplan-Meier curves, and we compared differences in their log-rank P values using Kaplan-Meier plotter. As shown in Figure 2A and Figure S2, high GLYATL1 mRNA expression levels were associated with improved OS of patients with clear cell renal cell carcinoma (HR =0.49, P=3.1e-06), papillary renal cell carcinoma (HR =0.46, P=0.011), and HCC (HR =0.61, P=0.005). Of note, we found that the GLYATL1 expression level in HCC tissues was significantly lower than that in normal liver tissues, which indicated poor OS. Similar results were also obtained when we further investigated other survival outcomes, including progression-free survival (PFS) and disease-free survival (DSS), in HCC patients (Figure 2B,C,D).
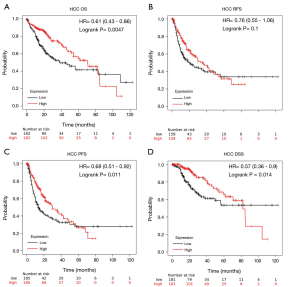
Subanalysis of GLYATL1 mRNA expression and the clinicopathologic features of HCC patients
To determine how GLYATL1 mRNA expression affects the prognosis of HCC patients (based on results from Kaplan-Meier plotter), we further explored the correlation between GLYATL1 mRNA expression and the clinicopathologic features of HCC patients using the RNA-seq data of GLYATL1 and the corresponding clinical information from the TCGA. The results indicated that downregulated GLYATL1 mRNA expression was associated with poor OS in Asian HCC patients, a subgroup of HCC patients from TCGA (HR =0.36, P=0.001) and male HCC patients (HR =0.53, P=0.0065). Regarding a history of alcohol consumption and hepatitis virus, we found a significant association between low GLYATL1 mRNA expression and poor OS in patients with a history of alcohol consumption (HR =0.42, P=0.0071). Surprisingly, unlike other cancer biomarkers, in our study, decreased GLYATL1 mRNA expression was associated with poor OS in HCC patients whose stages were I-II (HR =0.61, P=0.0489), whose grades were 3 (HR =0.46, P=0.0116) or with vascular invasion (HR =0.56, P=0.03). We also used data from the GSE116174 dataset to confirm GLYATL1 expression and clinical data. The results in the GSE116174 cohort indicated that high GLYATL1 mRNA expression indicated improved OS in patients in stages I-II (HR =0.42, P=0.047) and with no vascular invasion (HR =0.29, P=0.0016). Detailed information is listed in Table 1. High-throughput specimens may include immune cell infiltration, and immune cell infiltration could also impact the survival of HCC patients. We further investigated GLYATL1 expression and immune cell infiltration. As shown in Figure S3A, there was a significant association between immune cell infiltration and GLYATL1 expression. Thus, immune cell infiltration was regarded as a critical variable. We drew Kaplan-Meier plots for B cells, CD4+ T cells, CD8+ T cells, neutrophils, macrophages and dendritic cells to visualize the survival differences in the HCC patient cohort from the TCGA, and the results indicated that the expression of these six immune infiltrates did not lead to significant survival differences (Figure S3B). Univariate and multivariate Cox analyses indicated that GLYATL1 expression (HR =0.847, P<0.0001) was an independent prognostic factor for HCC patients (Table 2). We also analyzed immune cell infiltration in the GSE116174 dataset. As presented in Table S2, we obtained results that were similar to those of the TCGA cohort. Validation in the GSE116174 cohort also confirmed the role of GLYATL1 (HR =0.297, P<0.005) as an independent factor (Table 2). Taken together, these results suggest that GLYATL1 is an independent prognostic factor for HCC patients.
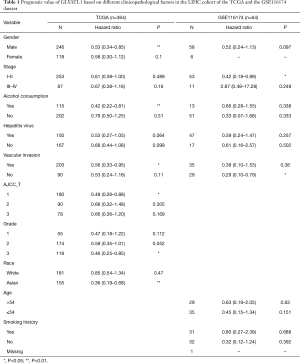
Full table
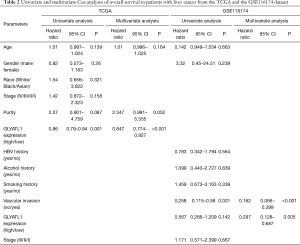
Full table
GLYATL1 promoter methylation profile based on the clinicopathologic characteristics of HCC patients
DNA methylation is a chemical modification of DNA that can alter genetic performance without changing the DNA sequence and control gene expression (19,20). Therefore, we further explored the GLYATL1 promoter methylation profile based on the clinicopathologic characteristics of HCC patients. As shown in Figure 3, the results indicated that the promoter methylation level of GLYATL1 in HCC tissue was significantly higher than that in normal liver tissue (P<1e-12). Next, we performed a subgroup analysis based on clinicopathologic characteristics. Of note, the GLYATL1 promoter methylation profile based on individual cancer stage, tumor grade and nodal metastasis status suggested significant differences (all P<0.0001). These results indicate that high GLYATL1 promoter methylation might suppress the expression level of GLYATL1, leading to the development of HCC.
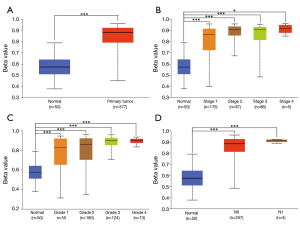
Construction of the PPI network via the STRING database
The STRING database was used to construct PPI networks of GLYATL1 and its coregulated hub genes. The results indicated that GLYATL1 was co-expressed with cyclic nucleotide gated channel alpha 3 (CNGA3), guanine nucleotide-binding protein subunit beta-5 (GNB5), K acetyltransferase 2A (KAT2A), K acetyltransferase 2B (KAT2B), Opsin 4 (OPN4), polyamine oxidase (PAOX), the regulator of G protein signaling 9 (RGS9), RGS9-binding protein (RGS9BP), spermidine/spermine N1-acetyltransferase 1 (SAT1) and spermidine/spermine N1-acetyltransferase 2 (SAT2) (Figure 4A). Among these genes, KAT2A had the maximum combined score (n=0.836), followed by RGS9BP, KAT2B, and OPN4 (n=0.8). Next, GO analysis with CC, BP, and MF terms and KEGG pathway analysis of these 10 coregulated hub genes were conducted. GO analysis showed that “photoreceptor outer segment”, “ciliary membrane” and “Ada2/Gcn5/Ada3 transcription activator complex” in CC; “N-acyltransferase activity”, “N-acetyltransferase activity” and “diamine N-acetyltransferase activity” in MF; and “putrescine catabolic process”, “spermine metabolic process” and “spermidine metabolic process” in BP were the most significantly enriched pathways associated with the 10 coregulated hub genes (all P<0.0001). KEGG pathway analysis revealed that most genes were enriched in “arginine and proline metabolism”, “ferroptosis” and “Notch signaling pathway” (all P<0.01) (Figure 4B).
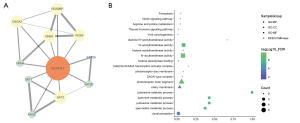
Potential mechanisms of downregulated GLYATL1 that regulate the tumorigenicity of HCC
In our study, GLYATL1 expression was lower in HCC tissues than in normal tissues, and low GLYATL1 expression indicated a poor survival rate of HCC patients. Therefore, GLYATL1 might serve as a novel biomarker for patients with HCC. Compared with GO and KEGG pathway analyses, GSEA is more reliable because of its ability to distinguish expression changes in gene sets. Thus, GSEA was used to further explore the potential biological pathogenesis of GLYATL1 using the HCC patient cohort from the TCGA. As shown in Figure 5A,B,C,D,E,F,G and Table 3, the results indicated that low GLYATL1 expression is associated with bile acid metabolism, xenobiotic metabolism, fatty acid metabolism, coagulation, the peroxisome and adipogenesis.
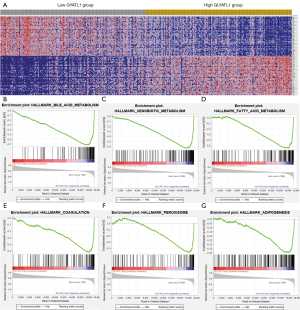
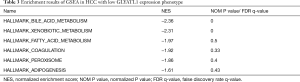
Full table
Correlation analysis of GLYATL1 and genes involved in glutamine metabolism
We utilized TIMER to explore associations between GLYATL1 and the key genes involved in mitochondrial glutamine metabolism based on the expression datasets of HCC and normal liver tissue from the TCGA. As shown in Figure 6, the expression of GLYATL1 showed a significant association with the expression of most genes involved in mitochondrial glutamine metabolism, including SLC1A5 (partial.cor =−0.511, P=2.51e-24), SLC1A11 (partial.cor =−0.315, P=2.24e-09), GLS (partial.cor =−0.293, P=2.82e-08), GLS2 (partial.cor =0.453, P=7.17e-19), SLC38A5 (partial.cor =−0.399, P=1.21e-14), GOT1 (partial.cor =0.195, P=2.77e-04) and GOT2 (partial.cor =0.464, P=7.91e-20), in HCC tissues. As presented in Table 4, GEPIA also confirmed the association between GLYATL1 and genes involved in mitochondrial glutamine metabolism. Taken together, these results suggest that GLYATL1 facilitates the development of HCC by regulating mitochondrial glutamine metabolism. However, more experimental studies are needed to validate this conclusion.
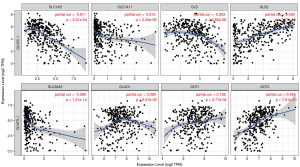
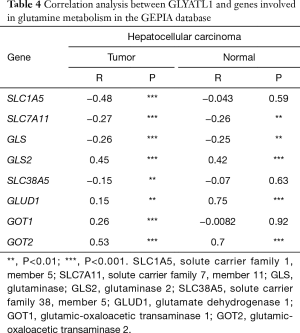
Full table
Discussion
GLYATL1 is involved in both normal physiological metabolism and tumorigenesis. However, the prognostic value of GLYATL1 is still unknown. Here, to our knowledge, this is the first report on the expression level and prognostic value of GLYATL1 across different cancers. Notably, we found that the expression of GLYATL1 in HCC tissues was lower than that in normal liver tissues and indicated poor OS. Moreover, univariate and multivariate Cox analyses revealed that GLYATL1 could be an independent prognostic factor for patients with HCC. Interestingly, decreased GLYATL1 mRNA expression was associated with poor OS in HCC patients with stages I-II disease. Additionally, the promoter methylation level of GLYATL1 in HCC tissue was significantly higher than that in normal liver tissue. The PPI network was constructed with GLYATL1 and its coregulated hub genes. Furthermore, our study suggests that a low expression level of GLYATL1 in HCC is positively associated with xenobiotic metabolism and significantly associated with the expression of most genes involved in mitochondrial glutamine metabolism, such as SLC1A5, SLC1A11, GLS and SLC38A5. We further hypothesize that GLYATL1 catalyzes glutamine to affect mitochondrial glutamine metabolism, further inhibiting the rapid proliferation of HCC. In summary, we conducted a systematic assessment of the potential role of GLYATL1 in HCC and determined that GLYATL1 can be regarded as a novel and potential prognostic biomarker for HCC patients.
In the present study, the mRNA expression level of GLYATL1 across different cancers was explored by the Oncomine database and confirmed by the UALCAN cancer database. The results of the two databases indicated that consistent with prior studies (9,10), the expression of GLYATL1 was significantly downregulated in HCC, while it was significantly upregulated in prostate adenocarcinoma. Our findings regarding its expression in BRCA were in line with those from a previous study (21). Interestingly, compared with normal tissues, GLYATL1 expression in kidney cancer tissues, including kidney chromophobe, clear cell renal cell carcinoma and kidney renal papillary cell carcinoma, was lower. We further plotted Kaplan-Meier curves to evaluate the prognostic value of GLYATL1 in 21 cancer types using Kaplan-Meier plotter. The results demonstrated that high GLYATL1 mRNA expression levels were associated with improved OS in many cancers, including clear cell renal cell carcinoma, liver HCC, bladder carcinoma, cervical squamous cell carcinoma, lung adenocarcinoma, ovarian cancer, pancreatic ductal adenocarcinoma, pheochromocytoma, and paraganglioma. Surprisingly, the expression of the GLYATL1 gene was not significantly associated with OS (P>0.05), though it exhibited significantly different expression between prostate adenocarcinoma and adjacent normal samples. Notably, we found that in HCC, both GLYATL1 expression and its prognostic value (including OS) were statistically significant. Moreover, univariate and multivariate Cox analyses revealed that GLYATL1 could be an independent prognostic factor for patients with HCC. We further conducted a subgroup analysis based on clinicopathologic characteristics. Surprisingly, unlike other prognostic biomarkers, decreased GLYATL1 mRNA expression was associated with poor OS in HCC patients with stages I–II. In the HCC cohort from the TCGA, decreased GLYATL1 mRNA indicated poor survival for patients with an Edmondson grade of 3. An Edmondson grade of 3 indicates that HCC cells are poorly differentiated. A previous study suggested that GLYAT may be repressed in dedifferentiated HCC cells (9). Thus, an Edmondson grade of 3 may lower the expression of GLYATL1, resulting in a poor survival rate. However, the mechanism underlying the association between stages I-II and the prognostic value of GLYATL1 is still unclear. These results suggest that the prognostic value of GLYATL1 might be associated with clinicopathologic characteristics.
DNA methylation is a common and critical epigenetic event that can lead to gene silencing at the level of pretranscription (22). Abnormal DNA methylation in the promoter might cause silencing of tumor suppressor genes or aberrant activation of oncogenes. As a result, abnormal DNA methylation is considered a hallmark of human tumors (23). We also explored the GLYATL1 promoter methylation profile in HCC patients. The results suggested that the level of GLYATL1 promoter methylation was significantly increased in HCC tissues compared with that in normal tissues. Similar results were also found in the subgroup analysis based on clinicopathologic characteristics, including individual cancer stage, tumor grade, and nodal metastasis status. Therefore, we postulate that high GLYATL1 promoter methylation might suppress the expression level of GLYATL1, leading to the development of HCC.
STRING was used to construct the PPI network based on the coexpression of GLYATL1 with CNGA3, GNB5, KAT2A, KAT2B, OPN4, PAOX, RGS9, RGS9BP, SAT1, and SAT2. A mutation in CNGA3 can lead to achromatopsia (24). Most investigations on the role of GNB5 have concentrated on neuronal signaling (25). OPN4 is a G protein-coupled receptor. In the latest study, scientists found that targeting OPN4 could suppress PKC/RAF/MEK/ERK signaling and cell growth and lead to apoptosis in lung cancer cells (26). KAT2A and KAT2B participate in lysine acetylation, a common posttranslational modification that regulates multiple BPs (27). Moreover, accumulating evidence has revealed that the abnormal expression of KAT2A and KAT2B occurs in various cancers, including colon cancer and HCC, and contributes to resistance to cancer therapeutics (28,29). A previous study indicated that RGS9 was downregulated in lung adenocarcinoma and that there was an indirect association between RGS9, Kras and p53 (30). RGS9BP, the binding protein of RGS9, plays a role in bladder cancer (31). SAT1 and SAT2 are members of SAT, a regulator of polyamine catabolism that is involved in the inflammatory response and associated with the growth of several cancers, such as non-small cell lung cancer (32,33). Recently, Ou et al. demonstrated that p53 regulates the reactive oxygen species stress response and ferroptosis via the activation of SAT1 (34). These results indicate the roles of these co-expressed genes in the development of various cancers, but the synergistic pathways of GLYATL1 and its co-expressed genes in cancers remain elusive. Thus, we conducted GO and KEGG pathway analyses to explore the potential mechanism. The results indicated that these genes are enriched mainly in amine substance metabolic processes. However, the detailed mechanism by which GLYATL1, along with its co-expressed genes, engages in the process of oncogenesis via amine substance metabolic processes is still unknown.
Another important aspect of this study is that the expression of GLYATL1 in HCC was associated with xenobiotic metabolism. By analyzing the mRNA level of GLYATL1 from the TCGA HCC cohort using GSEA, we found that low GLYATL1 expression was predominantly negatively enriched in xenobiotic metabolism and other metabolism pathways, including bile acid metabolism, fatty acid metabolism, coagulation, peroxisome and adipogenesis. Since GLYAT plays a role in the conjugation of carboxylic acids to glycine (6), which is a key metabolite in the rapid proliferation of tumor cells, inhibiting glycine uptake or biosynthesis may impair the growth of cancer cells by slowing the synthesis of nucleic acids (35). GLYATL1 is an acyltransferase that is involved in xenobiotic metabolism in the liver. It can transfer an acyl group to the N-terminus of glutamine and produce N2-acyl-L-glutamine, which is excreted in the urine (9). Thus, we should understand the role of glutamine in material metabolism, especially xenobiotic metabolism and mitochondrial glutamine metabolism, if we want to determine the association between GLYATL1 and mitochondrial glutamine metabolism in HCC. Glutamine, the most abundant circulating amino acid in human plasma, is essential for mitochondrial metabolism, supporting the rapid proliferation of tumor cells (36-38). Previous studies have shown that inhibiting mitochondrial glutamine metabolism could result in a strong cancer-fighting effect (39), such as apoptosis (40). Under certain conditions, the more glutamine GLYATL1 conjugates in xenobiotic metabolism, the less efficient glutamine is for mitochondrial glutamine metabolism. Thus, we conjecture that GLYATL1 catalyzes glutamine and affects mitochondrial glutamine metabolism, subsequently suppressing the inexorable growth of HCC cells. This ratiocination helps us illuminate the reason why GLYATL1 expression in HCC tissues was downregulated and indicated a poor survival rate of HCC patients.
We must acknowledge potential limitations to our analysis. First, we analyzed the impact of immune cell infiltration, but the number of related variables was limited, and we did not use our HCC samples to verify the expression and prognostic value of GLYATL1. Second, although we found that the prognostic value of GLYATL1 might be associated with clinicopathologic characteristics, it was not corrected by multiple hypothesis testing. Third, we did not compare the predictive power of GLYATL1 with other classifications. Finally, the association between the expression of GLYATL1 and xenobiotic metabolism in HCC was explored by GSEA and confirmed with the TIMER and GEPIA databases. Our hypothesis should be further verified by in vitro and animal experiments.
In summary, we performed a comprehensive analysis of the role of GLYATL1 in different cancers. In particular, low GLYATL1 expression is associated with a poor prognosis and is an independent prognostic factor for patients with HCC. Moreover, GLYATL1 is associated with glutamine in xenobiotic metabolism in HCC. Therefore, GLYATL1 can be regarded as a potential prognostic indicator for HCC patients.
Acknowledgments
Thank Lijie Gan for providing a lot of statistical suggestions. And American Journal Experts provided our team with premium editing service.
Funding: This work was supported by the National Natural Science Foundation of China (Grant Nos. 81701560 and 81972792), the National Science Foundation of Guangdong Province, People’s Republic of China (Grant Nos. 2020A1515010149, 2019A1515011676, 2017A030313530, and 2017A030313548), the Guangzhou Science and Technology Projects (No. 201904010021) and the High-level Hospital Construction Project (Grant No. DFJH2019015).
Footnote
Reporting Checklist: The authors have completed the MDAR reporting checklist. Available at http://dx.doi.org/10.21037/jgo-20-186
Data Sharing Statement: Available at http://dx.doi.org/10.21037/jgo-20-186
Conflicts of Interest: All authors have completed the ICMJE uniform disclosure form (available at: http://dx.doi.org/10.21037/jgo-20-186). The authors have no conflicts of interest to declare.
Ethical Statement: The authors are accountable for all aspects of the work in ensuring that questions related to the accuracy or integrity of any part of the work are appropriately investigated and resolved. In our study, the statement of ethics approval and the informed consent were not required because all the liver sample information was downloaded from public databases, like The Cancer Genome Atlas (TCGA) and Gene Expression Omnibus (GEO). The study was conducted in accordance with the Declaration of Helsinki (as revised in 2013).
Open Access Statement: This is an Open Access article distributed in accordance with the Creative Commons Attribution-NonCommercial-NoDerivs 4.0 International License (CC BY-NC-ND 4.0), which permits the non-commercial replication and distribution of the article with the strict proviso that no changes or edits are made and the original work is properly cited (including links to both the formal publication through the relevant DOI and the license). See: https://creativecommons.org/licenses/by-nc-nd/4.0/.
References
- Guo Z, Zhong J, Jiang J, et al. Comparison of Survival of Patients with BCLC Stage A Hepatocellular Carcinoma After Hepatic Resection or Transarterial Chemoembolization: A Propensity Score-Based Analysis. Ann Surg Oncol 2014;21:3069-76. [Crossref] [PubMed]
- Zhong JH, Rodríguez AC, Ke Y, et al. Hepatic Resection as a Safe and Effective Treatment for Hepatocellular Carcinoma Involving a Single Large Tumor, Multiple Tumors, or Macrovascular Invasion. Medicine (Baltimore) 2015;94:e396. [Crossref] [PubMed]
- Portolani N, Coniglio A, Ghidoni S, et al. Early and Late Recurrence After Liver Resection for Hepatocellular Carcinoma. Ann Surg 2006;243:229-35. [Crossref] [PubMed]
- Sun W, Cabrera R. Systemic Treatment of Patients with Advanced, Unresectable Hepatocellular Carcinoma: Emergence of Therapies. J Gastrointest Cancer 2018;49:107-15. [Crossref] [PubMed]
- Schachter D, Taggart JV. Benzoyl coenzyme A and hippurate synthesis. J Biol Chem 1953;203:925-34. [PubMed]
- Badenhorst CP, van der Sluis R, Erasmus E, et al. Glycine conjugation: importance in metabolism, the role of glycine N-acyltransferase, and factors that influence interindividual variation. Expert Opin Drug Metab Toxicol 2013;9:1139-53. [Crossref] [PubMed]
- Zhang H, Lang Q, Li J, et al. Molecular Cloning and Characterization of a Novel Human Glycine-N-acyltransferase Gene GLYATL1, Which Activates Transcriptional Activity of HSE Pathway. Int J Mol Sci 2007;8:433-44. [Crossref]
- Barfeld SJ, East P, Zuber V, et al. Meta-analysis of prostate cancer gene expression data identifies a novel discriminatory signature enriched for glycosylating enzymes. BMC Med Genomics 2014;7:513. [Crossref] [PubMed]
- Matsuo M, Terai K, Kameda N, et al. Designation of enzyme activity of glycine-N-acyltransferase family genes and depression of glycine-N-acyltransferase in human hepatocellular carcinoma. Biochem Biophys Res Commun 2012;420:901-6. [Crossref] [PubMed]
- Eich ML, Chandrashekar DS, Rodriguez PAM, et al. Characterization of glycine-N-acyltransferase like 1 (GLYATL1) in prostate cancer. Prostate 2019;79:1629-39. [Crossref] [PubMed]
- Rhodes DR, Yu J, Shanker K, et al. ONCOMINE: a cancer microarray database and integrated data-mining platform. Neoplasia 2004;6:1-6. [Crossref] [PubMed]
- Chandrashekar DS, Bashel B, Balasubramanya SAH, et al. UALCAN: A Portal for Facilitating Tumor Subgroup Gene Expression and Survival Analyses. Neoplasia 2017;19:649-58. [Crossref] [PubMed]
- Shinawi T, Hill VK, Krex D, et al. DNA methylation profiles of long- and short-term glioblastoma survivors. Epigenetics 2013;8:149-56. [Crossref] [PubMed]
- Nagy Á, Lánczky A, Menyhárt O, et al. Validation of miRNA prognostic power in hepatocellular carcinoma using expression data of independent datasets. Sci Rep 2018;8:9227. [Crossref] [PubMed]
- Li T, Fan J, Wang B, et al. TIMER: A Web Server for Comprehensive Analysis of Tumor-Infiltrating Immune Cells. Cancer Res 2017;77:e108-10. [Crossref] [PubMed]
- Franceschini A, Szklarczyk D, Frankild S, et al. STRING V9.1: Protein-Protein Interaction Networks, with Increased Coverage and Integration. Nucleic Acids Res 2013;41:D808-15. [Crossref] [PubMed]
- Subramanian A, Tamayo P, Mootha VK, et al. Gene set enrichment analysis: a knowledge-based approach for interpreting genome-wide expression profiles. Proc Natl Acad Sci U S A 2005;102:15545-50. [Crossref] [PubMed]
- Tang Z, Li C, Kang B, et al. GEPIA: a web server for cancer and normal gene expression profiling and interactive analyses. Nucleic Acids Res 2017;45:W98-W102. [Crossref] [PubMed]
- Li W, Wang Y, Zhu J, et al. Differential DNA methylation may contribute to temporal and spatial regulation of gene expression and the development of mycelia and conidia in entomopathogenic fungus Metarhizium robertsii. Fungal Biol 2017;121:293-303. [Crossref] [PubMed]
- Demetriou CA, van Veldhoven K, Relton C, et al. Biological embedding of early-life exposures and disease risk in humans: a role for DNA methylation. Eur J Clin Invest 2015;45:303-32. [Crossref] [PubMed]
- Wang J, Shidfar A, Ivancic D, et al. Overexpression of lipid metabolism genes and PBX1 in the contralateral breasts of women with estrogen receptor-negative breast cancer. Int J Cancer 2017;140:2484-97. [Crossref] [PubMed]
- Bird A. DNA methylation patterns and epigenetic memory. Gene Dev 2002;16:6-21. [Crossref] [PubMed]
- Cruickshanks HA, McBryan T, Nelson DM, et al. Senescent cells harbour features of the cancer epigenome. Nat Cell Biol 2013;15:1495-506. [Crossref] [PubMed]
- Wissinger B, Gamer D, Gle HJ, et al. CNGA3 Mutations in Hereditary Cone Photoreceptor Disorders. Am J Hum Genet 2001;69:722-37. [Crossref] [PubMed]
- Shamseldin HE, Masuho I, Alenizi A, et al. GNB5 mutation causes a novel neuropsychiatric disorder featuring attention deficit hyperactivity disorder, severely impaired language development and normal cognition. Genome Biol 2016;17:195. [Crossref] [PubMed]
- Wang Q, Zhang T, Chang X, et al. Targeting Opsin4/Melanopsin with a novel small molecule suppresses PKC/RAF/MEK/ERK signaling and inhibits lung adenocarcinoma progression. Mol Cancer Res 2020;18:1028-38. [Crossref] [PubMed]
- Choudhary C, Kumar C, Gnad F, et al. Lysine Acetylation Targets Protein Complexes and Co-Regulates Major Cellular Functions. Science 2009;325:834-40. [Crossref] [PubMed]
- Bondy-Chorney E, Denoncourt A, Sai Y, et al. Nonhistone targets of KAT2A and KAT2B implicated in cancer biology (1). Biochem Cell Biol 2019;97:30-45. [Crossref] [PubMed]
- Hirano G, Izumi H, Kidani A, et al. Enhanced expression of PCAF endows apoptosis resistance in cisplatin-resistant cells. Mol Cancer Res 2010;8:864-72. [Crossref] [PubMed]
- Valdmanis PN, Roy-Chaudhuri B, Kim HK, et al. Upregulation of the microRNA cluster at the Dlk1-Dio3 locus in lung adenocarcinoma. Oncogene 2015;34:94-103. [Crossref] [PubMed]
- Yang Z, Li C, Fan Z, et al. Single-cell Sequencing Reveals Variants in ARID1A, GPRC5A and MLL2 Driving Self-renewal of Human Bladder Cancer Stem Cells. Eur Urol 2017;71:8-12. [Crossref] [PubMed]
- Pegg AE, Casero RJ. Current status of the polyamine research field. Methods Mol Biol 2011;720:3-35. [Crossref] [PubMed]
- Babbar N, Hacker A, Huang Y, et al. Tumor necrosis factor alpha induces spermidine/spermine N1-acetyltransferase through nuclear factor kappaB in non-small cell lung cancer cells. J Biol Chem 2006;281:24182-92. [Crossref] [PubMed]
- Ou Y, Wang SJ, Li D, et al. Activation of SAT1 engages polyamine metabolism with p53-mediated ferroptotic responses. Proc Natl Acad Sci U S A 2016;113:E6806-12. [Crossref] [PubMed]
- Jain M, Nilsson R, Sharma S, et al. Metabolite profiling identifies a key role for glycine in rapid cancer cell proliferation. Science 2012;336:1040-4. [Crossref] [PubMed]
- Gao P, Tchernyshyov I, Chang TC, et al. c-Myc suppression of miR-23a/b enhances mitochondrial glutaminase expression and glutamine metabolism. Nature 2009;458:762-5. [Crossref] [PubMed]
- Son J, Lyssiotis CA, Ying H, et al. Glutamine supports pancreatic cancer growth through a KRAS-regulated metabolic pathway. Nature 2013;496:101-5. [Crossref] [PubMed]
- Wise DR, DeBerardinis RJ, Mancuso A, et al. Myc regulates a transcriptional program that stimulates mitochondrial glutaminolysis and leads to glutamine addiction. Proc Natl Acad Sci U S A 2008;105:18782-7. [Crossref] [PubMed]
- Wise DR, Thompson CB. Glutamine addiction: a new therapeutic target in cancer. Trends Biochem Sci 2010;35:427-33. [Crossref] [PubMed]
- Yuneva MO, Fan TW, Allen TD, et al. The metabolic profile of tumors depends on both the responsible genetic lesion and tissue type. Cell Metab 2012;15:157-70. [Crossref] [PubMed]