Nomogram model characterized by mutant genes and clinical indexes to identify high-risk patients with stage III/IV colorectal cancer
Introduction
Colorectal cancer (CRC) is one of the most widespread and fatal malignant tumors worldwide. Recent data indicate that the incidence and mortality of CRC ranks third and second, respectively, among all cancers globally (1). In China, CRC is one of the most common malignant tumors of the digestive system. According to the data released by the China Cancer Center in 2019, the incidence and mortality of CRC ranks third and fifth, respectively, among malignant tumors in China, and are still increasing (2). The survival time of CRC patients is closely related to prognosis (3). Tumor staging is defined as the scope, disease degree, and nature of malignant tumor, and is one of the most important indicators for judging the prognosis of CRC. For example, previously published studies have confirmed that stage III–IV CRC at the time of diagnosis is the main prognostic factor affecting overall survival (4). Accurate pathological staging is of significance for the selection of postoperative therapeutic schemes and clinical research. However, the present staging method is suboptimal due to the variation in outcomes that occur among patients at the same stage (5). Therefore, there is an urgent need for relevant indicators or models that help tumor staging to predict CRC prognosis.
With widespread and thorough understanding of molecular genetics, previously published studies have indicated that the occurrence and development of CRC is a complex process involving a series of genes and multistep regulations (6). Genetic changes mainly include genome instability, activation of oncogenes, and inactivation of tumor suppressor genes (7,8). Detecting the mutations of these genes has evaluation value for cancer occurrence, targeted therapy, application and efficacy of immunotherapy, and a guiding role for prognostic prediction. For example, the mutation status of B-Raf proto-oncogene, serine/threonine kinase (BRAF), NRAS proto-oncogene, GTPase (NRAS), Human epidermal growth factor receptor 2 (HER2), and KRAS proto-oncogene, GTPase (KRAS
Besides, different clinical features also lead to different prognosis. There are many factors influencing the prognosis of colorectal cancer, such as gender, age, tumor location, surgical nature, pathological type, pathological stage and tumor diameter, which are commonly used indicators in the study of colorectal prognosis. Studies also found that postoperative complications are important prognostic factors for 1-year mortality of CRC patients. However, most of previous studies only used genetic features or clinical characteristics alone to predict CRC prognosis, ignoring the importance of their combination. Hence, in the present study, we identified both the somatic mutation characteristics and clinical indexes of CRC in The Cancer Genome Atlas (TCGA) cohort, and then constructed a nomogram model for staging. A clinical cohort was then used to further study the nomogram model for the risk of stage III/IV CRC to provide further insight into CRC and to provide a more comprehensive reference for clinical practice. We present the following article in accordance with the TRIPOD reporting checklist (available at http://dx.doi.org/10.21037/jgo-20-548).
Methods
TCGA data screening
Mutation data and clinical information of 536 CRC patients were downloaded from TCGA database (https://tcga-data.nci.nih.gov/docs/publications/tcga/). These comprised 137 cases of rectum cancer and 399 cases of colon cancer. The exclusion criteria were tumor stage information and no clinical information. The screening procedure is outlined in Figure 1A, and a total of 251 TCGA patients were finally enrolled.
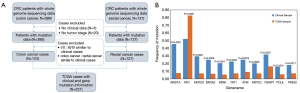
Patients and sample collection
From July 2015 to August 2019, 44 patients with CRC were enrolled from Tianjin Medical University Cancer Institute and Hospital (Tianjin, China). Tumor tissues were sequenced with targeted next-generation sequencing (NGS) by a 1,000-cancer gene panel; paracancerous tissues or leukocytes were used as controls. Clinical information of each patient was collected, such as sex, age, alcohol consumption, pathological type, stage, hypertension history, tumor size, tumor differentiation status, pathological location, recurrence, metastasis, and preoperative serum carcinoembryonic antigen (CEA). The present study was approved by the Ethics Committee of Tianjin Medical University Cancer Institute and Hospital. All participants provided signed informed consent. All procedures performed in this study involving human participants were in accordance with the Declaration of Helsinki (as revised in 2013).
Targeted NGS gene panel sequencing and mutation analysis
The extraction and purification of DNA were performed with the Genomic DNA extraction kit (Qiagen, Valencia, CA, USA) and Agencourt AMPure XP beads (Agencourt Bioscience, Beverly, MA, USA), respectively. The adaptor library was amplified and linked, and the total library was precisely quantified by the Qubit DNA HS Assay Kit (Invitrogen, Carlsbad, CA, USA) after purification. SeqCap EZ MedExome Enrichment kits (Roche, Basel, Switzerland) were used to capture target sequences, and Roche custom 1,000 gene probes (Roche, Switzerland) were used to capture and elute the hybridization library. After polymerase chain reaction amplification, the constructed library was sequenced using an Illumina HiSeq Xten sequencer (Illumina Inc., San Diego, CA, USA).
SOAPnuke (BGI, Shenzhen, Guangdong, China) was used to filter the sequencing data, removing the sequences containing adapters and the low-quality data. Burrows-Wheeler Aligner (Li H and Durbin R, Trust Sanger Institute, Genome Campus, Cambridge, UK) was applied to map the data to the human reference genome (hg19.fa). The Genome Analysis Toolkit (GATK) (version 2.3.9, Program in Medical and Population Genetics, The Broad Institute of Harvard and MIT, Cambridge, Massachusetts, USA.) was used to re-compare the reads in the interval, calibrate and rearrange the alkali matrix quality values, and calculate the sequence depth and coverage. Insertion/deletion, single-nucleotide variants, copy number variations, and fusion were detected by the GATK, VarScan (version 2.3 8, The Genome Institute, Washington University, Washington, USA), MuTect (version 1.1.4, The Broad Institute of Harvard and MIT, Cambridge, Massachusetts, USA.), CONTRA (Bioinformatics Core Facility, Peter MacCallum Cancer Centre, Victoria, Australia), and a self-developed fusion program.
Kyoto Encyclopedia of Genes and Genomes (KEGG) and Gene Ontology (GO) enrichment analyses
The org.Hs.eg.db package (version 3.10.0, Bioconductor Package Maintainer, bioconductor.org) in R (version 3.6.3, the Institute for Statistics and Mathematics of WU, Austria) was regarded as reference data, and GO term and KEGG pathway enrichment analyses were performed using clusterProfiler package (version 3.14.3, Guangchuang Yu, Southern Medical University, Guangzhou, Guangdong, China). P values were adjusted by Benjamini-Hochberg (BH) method; P<0.05 and q<0.05 were the cut-off criteria.
Construction of the nomogram model
In TCGA cohort, according to the generalized linear model function in R software (version 3.6.3), univariate logistic regression analysis was applied to screen out the clinical indexes and mutation genes associated with tumor stage (P<0.10). Significant factors were screened out by multivariate logistic backward stepwise regression (likelihood ratio) analysis using SPSS version 23.0 software (SPSS, Chicago, IL, USA), and the screening criterion was P<0.05. Based on the selected significant factors, a nomogram model was built using the rms package (version 6.0-0, Frank E. Harrell Jr, Vanderbilt University, Nashville, USA) in R (version 3.6.3).
Assessment of the nomogram model performance
The area under the receiver-operating characteristic (ROC) curve (AUC) was used to assess the discrimination of the nomogram model. The ROC curve was drawn through the pROC package (version 1.16.2, the Proteome Informatics group, SIB Swiss Institute of Bioinformatics, Batiment Amphipole, Lausanne, Switzerland) in the R software (version 3.6.3). The greater the AUC value, the higher the discrimination of the model. The calibration curve was drawn by bootstrapping according to 1,000 resampling procedures. To identify the goodness of fit of the nomogram model, Hosmer-Lemeshow test was conducted using the ResourceSelection package (version 0.3-5) in R software (version 3.6.3). P>0.05 indicated that the model had a good goodness of fit.
Validation of the nomogram model
In the clinical cohort, the performance of the nomogram model was also verified in terms of both discrimination and calibration using the same methods described earlier.
Clinical practice of the nomogram model
To assess the clinical applicability of the constructed nomogram model, the decision curve analysis (DCA) was drawn using the rmda package (version 1.6) in R software (version 3.6.3). Threshold probability represents the most beneficial region of the nomogram model.
Statistical analysis
The Fisher exact test with R software (version 3.6.3) was used to determine the statistical differences among groups.
Results
Patients’ characteristics
In total, 251 TCGA cases were downloaded; 124 (49.40%) cases of colon cancer and 127 (50.60%) cases of rectum cancer. Of these 74 (29.48%) were stage I/II, and 177 (70.52%) were stage III/IV. Clinical information of 44 clinical cases is shown in Table 1.
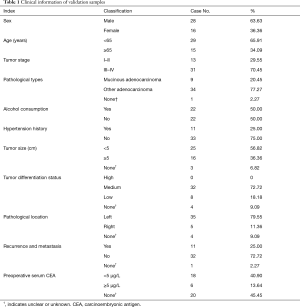
Full table
Gene somatic mutation landscape
Briefly, 1,221 mutations in 239 genes were observed in the clinical cases, and 69,710 mutations involving 15,900 genes were identified in TCGA cases. Additionally, in the 2 cohorts, there were 31 shared mutation genes with frequency >5%. The 31 genes were ARID1A, APC, KMT2D, BRCA2, BRAF, FAT1, ATM, KMT2C, FBXW7, POLE, PREX2, TP53, ZFHX3, BCOR, SMAD4, PTEN, PTPRT, TCF7L2, TRRAP, LRP1B, LRP2, ROS1, GRIN2A, KRAS, COL22A1, FAT4, PIK3CA, ERBB4, LPA, ZNF521, and CTNNB1. Of these, the 3 most common genes in the clinical cases were TP53 (57%), APC (52%), and ARID1A (45%), for TCGA cases, these were APC (83%), TP53 (71%), and KRAS (36%). However, 11 genes were significantly different in mutation frequency between the clinical cohort and TCGA cases, which could be associated with differences in race, age, and sample size between 2 two groups (Figure 1B).
GO term and KEGG pathway enrichment analyses
GO term and KEGG pathway enrichment analyses were performed on the 31 shared genes. These genes were enriched in 588 GO terms, including 544 biologic processes (BPs), 27 cellular components (CCs), and 17 molecular functions (MFs). The top 10 BPs, CCs, and MFs are shown in Figure 2A. These genes were primarily enriched in 81 pathways. The top 30 pathways are shown in Figure 2B.
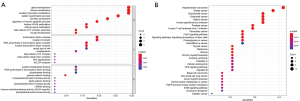
Construction of the stage prediction nomogram model
According to the univariate and multivariate logistic regression analyses, the residual variables were SMAD4, ZFHX3, and PREX2 mutation status; pathological location; and preoperative CEA value, thereby establishing a nomogram model of high-risk stage III/IV CRC (Figure 3). Patients with SMAD4 gene mutations had a higher score than those without SMAD4 gene mutations. However, the opposite was observed for ZFHX3 and PREX2 gene mutations. All of the three genes were enriched in the KEGG pathway of signaling pathways regulating pluripotency of stem cells, indicating that pluripotency of stem cells could be regulated in advanced patients through these gene mutations. The score for right-sided colon cancer was higher than that for left-sided colon cancer. With the increase of preoperative CEA value, the corresponding score showed an upward trend.
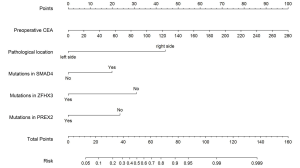
Assessment the performance of the nomogram model
In TCGA cohort, the calibration curve indicated that the probabilities of stage III/IV CRC, as predicted by the nomogram, were in good agreement with the actual probabilities (Figure 4A). According to the Hosmer-Lemeshow test, we obtained a P value of 0.83, which indicating that perfect fit remained. Furthermore, the ROC curve indicated that the nomogram model had good discriminative ability (Figure 4B), with an AUC of 0.76 [95% confidence interval (CI): 0.69–0.84].
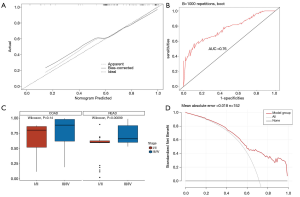
Validation of the nomogram model
Cases lacking pathological location and preoperative CEA information were removed, and 23 samples were retained for model verification. In the clinical cohort, the AUC value of the nomogram was 0.55 (95% CI: 0.28–0.63); the Hosmer-Lemeshow test also showed statistically significance (P=0.01). The calibration curve did not show good calibration, possibly because we had insufficient clinical samples.
Furthermore, in TCGA cohort, we compared the prediction ability of the model in different types of CRC. By scoring, patients with rectal cancer could be well staged (P<0.001, Figure 4C), but there was no difference in the scores of colon cancer patients with different stages (P=0.14, Figure 4C), indicating that the model had different staging abilities in different clinical types.
Clinical applicability of the nomogram model
The DCA for the nomogram model demonstrated that, in our study, the use of the nomogram to stratify the stage of CRC patients was beneficial at all threshold probabilities (Figure 4D).
Discussion
Predicting the risk of stage III/IV CRC in patients has considerable clinical significance. It can assist with other related examinations to adjust follow-up treatment strategies, thereby prolonging patients’ survival time and quality of life. In the present study, we established a nomogram model of high-risk stage III/IV CRC based on mutant genes and clinical information; that is, the mutation status of SMAD4, ZFHX3, and PREX2; pathological location; and preoperative CEA value. Furthermore, this nomogram model was also validated in the clinical cohort.
SMAD4 is the central molecule of the transforming growth factor-β (TGF-β) signaling pathway, and participates in the process of intracellular signaling. The inactivation or low expression of SMAD4 can affect the signal transduction of TGF-β and participate in tumor formation (10). SMAD4 mutation or abnormal expression is most common in pancreatic cancer, and its mutation frequency is about 50% (11), followed by gastric cancer and pancreatic cancer (12,13). In CRC, the mutation frequency of SMAD4 is about 2–20% (14,15). Many studies have shown that SMAD4 also plays an important role in the development and prognosis of CRC. For example, Huang et al. indicated that SMAD4 mutations play vital roles in CRC metastasis, and may be both potential biomarkers and therapeutic targets of CRC metastasis (16). Mizuno et al. also found that SMAD4 mutations are independently associated with poor prognosis among patients undergoing resection of colorectal liver metastases (17). In the present study, we found that the mutation frequencies of SMAD4 in clinical and TCGA cohorts were 23% and 14%, respectively, with no significant difference between them. Univariable and multivariable logistic regression analyses demonstrated that SMAD4 mutations were an independent risk factor for stage III/IV CRC. It could be seen from the nomogram model that patients with SMAD4 mutations were at increased risk of stage III/IV CRC.
Zinc finger homeobox 3 (ZFHX3), a large transcription factor, contains 23 zinc finger domains, 4 homeodomains, and multiple other motifs (18). It was initially regarded as AT motif-binding factor 1 by Ninomiya et al., which represses the transcription of α-fetoprotein through binding to its promoter (19). ZFHX3 is considered a tumor suppressor for human cancers, such as prostate and breast cancers (20,21). Phosphatidylinositol-3,4,5-trisphosphate-dependent Rac exchanger factor 2 (PREX2) can regulate small guanosine triphosphatase Rac. It participates in the process of inhibiting the activity of phosphatase and tensin homolog (PTEN), therefore upregulating the activity of the phosphoinositide 3-kinase signaling pathway (22). PREX2 has been observed to be involved in various types of cancer, including gastric cancer, hepatocellular carcinoma, and pancreatic cancer (23-25). The present research indicated that ZFHX3 mutations occurred in 16% of clinical samples and 8% of TCGA patients, and PREX2 in 18% of the clinical sample and 7% of TCGA patients. Moreover, patients without ZFHX3 and PREX2 mutations were at a higher risk of having stage III/IV CRC than those with mutations. These results are different to those of other cancers, and require further verification (20,21,23-25).
According to the location of the primary tumor, CRC can be divided into right-sided and left-sided CRC. Patients with different tumor locations have different pathogeneses, molecular pathways, and outcomes (26). Patients with right-sided colon cancer have more advanced tumor staging (27). Previously published studies have indicated that right-sided colon cancer has more mutations in BRAF, KRAS, SMAD4, TGF-β, PIK3CA, PTEN, and AKT1 genes, and high microsatellite instability (28). A prospective clinical analysis based on stage III CRC patients who received adjuvant chemotherapy also demonstrated inferior disease-free survival in right-sided colon cancer patients (29). Recent research has indicated that right-sided and left-sided colon cancer has different prognostic significance for recurrence and overall mortality after curative resection, and tumor location could be a prognostic biomarker in stage III CRC (30). Consistent with the results of previous studies, we found that the location of the primary tumor was an independent risk factor for high-risk stage III/IV CRC, and patients with right-sided colon cancer had a higher risk than those with left-sided colon cancer.
Serum CEA is a common specific tumor marker for CRC and is associated with prediction, efficacy of therapy, and the recurrence of CRC (31). Elevated serum CEA level is considered to be associated with reduced survival in patients with CRC (32). Preoperative serum CEA level >5 ng/mL seems to be a risk factor for the recurrence of stage III CRC (33). Moreover, Sisik et al. found that a positive level of CEA can be considered as advanced stage CRC (34). In the current study, we also demonstrated that, as the preoperative CEA value increases, the risk of stage III/IV CRC also increases.
A shortage of this study is that the number of clinical samples were relatively small, the constructed model has not been well validated, and a large sample study should be added for verification. The nomogram model based on molecular biology and clinical information can fully understand the important role of various risk factors in CRC staging. Moreover, the nomogram can integrate more clinicopathological parameters to achieve individualized predictions. It is a calculation chart that replaces complex mathematical formulas and can present the results of regression analyses in an intuitive graphical form, which is of significance for individualized and accurate predictions. Therefore, we are aiming to collect more CRC patients to make this model more accurate and credible, we will continue to increase the number of clinical samples in our further research on our relevant results. Besides, due to the short follow-up time of some patients, the existing survival information cannot be statistically analyzed. Since our model had better staging ability in TCGA cohort, the correlation of advance stage and the outcomes of CRC (such as overall survival, progression-free survival, recurrence and metastasis) based on molecular and clinical features is also our upcoming study to guide the clinical treatment and prognosis prediction of CRC.
The nomogram model of the SMAD4, ZFHX3, and PREX2 mutation status; pathological location; and preoperative CEA value could be applied to predict the risk of stage III/IV in CRC patients.
Acknowledgments
Funding: None.
Footnote
Reporting Checklist: The authors have completed the TRIPOD reporting checklist. Available at http://dx.doi.org/10.21037/jgo-20-548
Data Sharing Statement: Available at http://dx.doi.org/10.21037/jgo-20-548
Conflicts of Interest: All authors have completed the ICMJE uniform disclosure form (available at http://dx.doi.org/10.21037/jgo-20-548). The authors have no conflicts of interest to declare.
Ethical Statement: The authors are accountable for all aspects of the work in ensuring that questions related to the accuracy or integrity of any part of the work are appropriately investigated and resolved. The present study was approved by the Ethics Committee of Tianjin Medical University Cancer Institute and Hospital. All participants provided signed informed consent. All procedures performed in this study involving human participants were in accordance with the Declaration of Helsinki (as revised in 2013).
Open Access Statement: This is an Open Access article distributed in accordance with the Creative Commons Attribution-NonCommercial-NoDerivs 4.0 International License (CC BY-NC-ND 4.0), which permits the non-commercial replication and distribution of the article with the strict proviso that no changes or edits are made and the original work is properly cited (including links to both the formal publication through the relevant DOI and the license). See: https://creativecommons.org/licenses/by-nc-nd/4.0/.
References
- Siegel RL, Miller KD, Jemal A. Cancer statistics, 2020. CA Cancer J Clin 2020;70:7-30. [Crossref] [PubMed]
- Zheng RS, Sun KX, Zhang SW, et al. Report of cancer epidemiology in China, 2015. Zhonghua Zhong Liu Za Zhi 2019;41:19-28. [PubMed]
- Langan RC, Carpizo DR. Modernizing the clinical risk score to more accurately predict survival following resection of colorectal liver metastases. Transl Gastroenterol Hepatol 2019;4:49. [Crossref] [PubMed]
- Joachim C, Macni J, Drame M, et al. Overall survival of colorectal cancer by stage at diagnosis: Data from the Martinique Cancer Registry. Medicine (Baltimore) 2019;98:e16941. [Crossref] [PubMed]
- Ge W, Hu H, Cai W, et al. High-risk Stage III colon cancer patients identified by a novel five-gene mutational signature are characterized by upregulation of IL-23A and gut bacterial translocation of the tumor microenvironment. Int J Cancer 2020;146:2027-35. [Crossref] [PubMed]
- Davies RJ, Miller R, Coleman N. Colorectal cancer screening: prospects for molecular stool analysis. Nat Rev Cancer 2005;5:199-209. [Crossref] [PubMed]
- Grady WM, Markowitz SD. The molecular pathogenesis of colorectal cancer and its potential application to colorectal cancer screening. Dig Dis Sci 2015;60:762-72. [Crossref] [PubMed]
- Ng C, Li H, Wu WKK, et al. Genomics and metagenomics of colorectal cancer. J Gastrointest Oncol 2019;10:1164-70. [Crossref] [PubMed]
- Afrăsânie VA, Marinca MV, Alexa-Stratulat T, et al. KRAS, NRAS, BRAF, HER2 and microsatellite instability in metastatic colorectal cancer - practical implications for the clinician. Radiol Oncol 2019;53:265-74. [Crossref] [PubMed]
- Hahn SA, Schutte M, Hoque AT, et al. DPC4, a candidate tumor suppressor gene at human chromosome 18q21.1. Science 1996;271:350-3. [Crossref] [PubMed]
- Blackford A, Serrano OK, Wolfgang CL, et al. SMAD4 gene mutations are associated with poor prognosis in pancreatic cancer. Clin Cancer Res 2009;15:4674-9. [Crossref] [PubMed]
- Yatagai N, Saito T, Akazawa Y, et al. Frequent loss of heterozygosity of SMAD4 locus and prognostic impacts of SMAD4 immunohistochemistry in gastric adenocarcinoma with enteroblastic differentiation. Hum Pathol 2019;88:18-26. [Crossref] [PubMed]
- Wang F, Xia X, Yang C, et al. SMAD4 Gene Mutation Renders Pancreatic Cancer Resistance to Radiotherapy through Promotion of Autophagy. Clin Cancer Res 2018;24:3176-85. [Crossref] [PubMed]
- Fleming NI, Jorissen RN, Mouradov D, et al. SMAD2, SMAD3 and SMAD4 mutations in colorectal cancer. Cancer Res 2013;73:725-35. [Crossref] [PubMed]
- Yu J, Wu WK, Li X, et al. Novel recurrently mutated genes and a prognostic mutation signature in colorectal cancer. Gut 2015;64:636-45. [Crossref] [PubMed]
- Huang D, Sun W, Zhou Y, et al. Mutations of key driver genes in colorectal cancer progression and metastasis. Cancer Metastasis Rev 2018;37:173-87. [Crossref] [PubMed]
- Mizuno T, Cloyd JM, Vicente D, et al. SMAD4 gene mutation predicts poor prognosis in patients undergoing resection for colorectal liver metastases. Eur J Surg Oncol. 2018;44:684-92. [Crossref] [PubMed]
- Miura Y, Tam T, Ido A, et al. Cloning and characterization of an ATBF1 isoform that expresses in a neuronal differentiation-dependent manner. J Biol Chem 1995;270:26840-8. [Crossref] [PubMed]
- Ninomiya T, Mihara K, Fushimi K, et al. Regulation of the alpha-fetoprotein gene by the isoforms of ATBF1 transcription factor in human hepatoma. Hepatology 2002;35:82-7. [Crossref] [PubMed]
- Sun X, Zhou Y, Otto KB, et al. Infrequent mutation of ATBF1 in human breast cancer. J Cancer Res Clin Oncol 2007;133:103-5. [Crossref] [PubMed]
- Sun X, Frierson HF, Chen C, et al. Frequent somatic mutations of the transcription factor ATBF1 in human prostate cancer. Nat Genet 2005;37:407-12. [Crossref] [PubMed]
- Pandiella A, Montero JC. Molecular pathways: P-Rex in cancer. Clin Cancer Res 2013;19:4564-9. [Crossref] [PubMed]
- Guo B, Liu L, Yao J, et al. miR-338-3p suppresses gastric cancer progression through a PTEN-AKT axis by targeting P-REX2a. Mol Cancer Res 2014;12:313-21. [Crossref] [PubMed]
- Yang MH, Yen CH, Chen YF, et al. Somatic mutations of PREX2 gene in patients with hepatocellular carcinoma. Sci Rep 2019;9:2552. [Crossref] [PubMed]
- Yang J, Gong X, Ouyang L, et al. PREX2 promotes the proliferation, invasion and migration of pancreatic cancer cells by modulating the PI3K signaling pathway. Oncol Lett 2016;12:1139-43. [Crossref] [PubMed]
- Lee GH, Malietzis G, Askari A, et al. Is right-sided colon cancer different to left-sided colorectal cancer? - a systematic review. Eur J Surg Oncol 2015;41:300-8. [Crossref] [PubMed]
- Yang J, Du XL, Li ST, et al. Characteristics of Differently Located Colorectal Cancers Support Proximal and Distal Classification: A Population-Based Study of 57,847 Patients. PLoS One 2016;11:e0167540. [Crossref] [PubMed]
- Hsu YL, Lin CC, Jiang JK, et al. Clinicopathological and molecular differences in colorectal cancer according to location. Int J Biol Markers 2019;34:47-53. [Crossref] [PubMed]
- Sinicrope FA, Mahoney MR, Smyrk TC, et al. Prognostic impact of deficient DNA mismatch repair in patients with stage III colon cancer from a randomized trial of FOLFOX-based adjuvant chemotherapy. J Clin Oncol 2013;31:3664-72. [Crossref] [PubMed]
- Shida D, Inoue M, Tanabe T, et al. Prognostic impact of primary tumor location in Stage III colorectal cancer-right-sided colon versus left-sided colon versus rectum: a nationwide multicenter retrospective study. J Gastroenterol 2020;55:958-68. [Crossref] [PubMed]
- Tampellini M, Ottone A, Alabiso I, et al. The prognostic role of baseline CEA and CA 19-9 values and their time-dependent variations in advanced colorectal cancer patients submitted to first-line therapy. Tumour Biol 2015;36:1519-27. [Crossref] [PubMed]
- Zhai H, Huang J, Yang C, et al. Serum CEA and CA19-9 Levels are Associated with the Presence and Severity of Colorectal Neoplasia. Clin Lab 2018;64:351-6. [Crossref] [PubMed]
- Neki K, Eto K, Kosuge M, et al. Identification of the Risk Factors for Recurrence of Stage III Colorectal Cancer. Anticancer Res 2019;39:5721-4. [Crossref] [PubMed]
- Sisik A, Kaya M, Bas G, et al. CEA and CA 19-9 are still valuable markers for the prognosis of colorectal and gastric cancer patients. Asian Pac J Cancer Prev 2013;14:4289-94. [Crossref] [PubMed]
(English Language Editor: R. Scott)