Establishment and verification of a prognostic tumor microenvironment-based and immune-related gene signature in colon cancer
Introduction
Colon cancer (CC) has always been among the most frequent malignant cancers, the incidence and fatality rate of which have gradually increased in recent years (1). The causes of colon cancer were still unclear, but some studies showed that the tumorigenesis and development of colon cancer was the result of a combination of factors such as genetics, environment, and lifestyle. But adenocarcinomas accounting for most of the colon cancers usually begin as benign polyps also known as adenomas. Due to the significant progress made in treating CC, effective therapies now not only include surgical resection, radiotherapy, and adjuvant chemotherapy, but immunotherapy as well (2). In the last decade, drugs based on immunotherapy have been widely evaluated to further the development of cancer treatments. As a result, immunotherapy has become an effective treatment for a diverse range of cancers, including colorectal cancer (3,4). Although the treatment of CC has made significant progress, incidences of CC have continued to see a rapid increase, and the 5-year survival rate remains very low. Furthermore, ~80% of CC patients succumb to recurrence of the disease during the first 3 years (5). In addition, due to a lack of diagnostic and predictive biomarkers, newer and more sensitive prognostic immune-related indicators are needed to develop optimal therapeutic strategies.
Evidence that the immune system of a tumor microenvironment (TME) is closely linked to tumor development has increased considerably in the last few years (6-8). The tumor immune system affects the disease progress of cancers (9,10). TME plays a leading role in influencing the occurrence and development of tumor cells of CC. Macrophages, which predominate in the tumor microenvironment, promote colon cancer angiogenesis and facilitate colon cancer migration, invasion, and metastasis. The immune system of the TME comprises of extracellular matrix molecules, stromal cells, immune cells, as well as inflammatory factors (11). In recent years, a number of CC patients have achieved remarkable results through immune checkpoint inhibitors (ICIs), which target and suppress genes such as cytotoxic T lymphocyte antigen 4 (CTLA4), programmed cell death-ligand 1 (PD-L1/CD274), or programmed cell death protein 1 (PD-1/PDCD-1) (12,13). Furthermore, immune-related genes (IRGs) have been confirmed as attractive targets for the regulation of tumor progression. Therefore, incisive information for CC prognosis may be provided by exploring differently expressed IRGs based on stromal and immune scores. Considering this research, there exists an urgent need to construct an immune-linked prognostic biosignature based on the TME in CC to optimize treatment and predict how tumors respond to ICIs.
Many algorithms and online databases have recently been developed to estimate the purity of the TME, which depends on the gene expression pattern data of cancer patients (14-16). For instance, the estimation of stromal and immune cells in malignant tumor tissues using expression data (ESTIMATE) algorithm, which was designed by Yoshihara et al. (14), can be employed to calculate the scores of infiltrating immune and stromal cells, as well as validate tumor purity in different types of malignancies (17-19).
For this reason, we first used the ESTIMATE algorithm in our study to determine the high and low stromal and immune scores of differentially expressed genes (DEGs) in the TME of CC. We then established and validated a robust CC immune-linked gene biosignature hinged on the DEGs, and its clinical utility was also investigated. In addition, the CIBERSORT algorithm and Tumor Immune Estimation Resource (TIMER) database were used to evaluate the relationships between tumor immune infiltration and the risk score in CC samples. In summary, our study suggested that the immunogenomic risk score was closely related to the TME, and was also able to predict the prognosis of CC patients, leading to a more precise and personalized immunotherapy treatment. We present the following article in accordance with the TRIPOD reporting checklist (available at https://dx.doi.org/10.21037/jgo-21-522).
Methods
Data source
Transcriptomic RNA-sequencing data, as well as clinical information of CC patients, were extracted from The Cancer Genome Atlas (TCGA) web resource (https://cancergenome.nih.gov/). The transformed transcripts per million were used to make the data from TCGA similar to microarrays from the Gene Expression Omnibus (GEO) database (https://www.ncbi.nlm.nih.gov/geo/) (20). CC patients were excluded from the study if their follow-up time was <30 days, or if their overall survival (OS) data were unavailable. In total, 423 CC patients were selected for this study based on clinical information and complete effective transcriptional data. For further verification, the datasets GSE17536, GSE38832, and GSE17537 were abstracted from the GEO as verification datasets. The inclusion criteria were the same as the TCGA patients. The GSE17536 dataset comprised 177 primary CC patients, the GSE38832 dataset contained 122 samples, and the GSE17537 contained 55 samples, but the OS time of 6 patients was <30 days in total. Notably, GSE17536, GSE38832, and GSE17537 were all on account of the GPL570 platform. All the patients’ clinical characteristics are summarized in Table S1. The stromal and immune scores of every CC sample were computed through R package ‘ESTIMATE’, which inferred tumor purity in the tumor tissue (14). A comprehensive list of immune-related genes (IRGs) that were all related to the immune system (1,811 in total) was downloaded from the Immunology Database and Analysis Portal (ImmPort) public resource (https://www.immport.org/home) (21). The study was conducted in accordance with the Declaration of Helsinki (as revised in 2013).
Identification of potential differentially expressed IRGs
We calculated the messenger RNA (mRNA) expression data, stromal, and immune scores of the 423 individuals with CC by using the ESTIMATE algorithm. We clustered the CC patients into 2 groups via the best cut-off value computed by the X-tile plots (22), and then the ‘edgeR’ package in R (23) was applied to conduct differential analysis to establish the immune/stromal-linked DEGs. The cut-off values for the DEGs were set at log2 fold change (FC) >1 and false discovery rate (FDR) <0.05. To specifically investigate changes of genes related to the immune system, we used the intersection between the DEGs hinged on the scores and the IRGs retrieved from the ImmPort data portal.
Functional enrichment analysis
Kyoto Encyclopedia of Genes and Genomes (KEGG) pathway enrichment evaluation of DEGs was carried out to elucidate the roles of the differentially expressed IRGs. Our Gene Ontology (GO) evaluation included assessing the biological process (BP), cellular component (CC), as well as molecular function (MF) using the “clusterProfiler” R package (24). An FDR <0.05 in the GO and KEGG evaluations indicated significantly enriched IRGs.
Development and verification of the prognostic biosignature
We randomly selected 50% of the patients as the training set to establish the prognostic biosignature. The remaining 50% of the patients were used as an independent test set to confirm the estimation accuracy of the model. Based on these differentially expressed IRGs, we constructed a prognostic model through a univariate analysis using Cox and least absolute shrinkage and selection operator (LASSO), as well as a multivariate regression analysis using Cox. In addition, we computed the risk score depending on a combination of gene expression and Cox coefficient. The risk scorerepresents the number of our model’s genes and the coefficient value estimated from the multivariate Cox regression analysis. Based on the optimal cut‐off value established by the “surv_cutpoint” tool of the “survminer” R package, the CC patient training cohort was clustered into a high-risk group, as well as a low-risk group. Kaplan-Meier survival curves were generated through “survival” in R package. Moreover, the R “timeROC” package was applied to calculate the area under the curve (AUC) of the time-dependent receiver operating characteristic (ROC) curve, and this was used to estimate the accuracy of the prognostic model. Additionally, our prognostic model was also evaluated using the risk score distribution plots, scatter plots of survival status, and the heatmap between the high-risk and low-risk groups. R 3.6.3 was utilized in all the statistical analyses. To confirm the predictive accuracy of our prognostic biosignature, we used the CC patients in the testing cohort, the entire cohort, and the 3 external GEO cohorts (GSE17536, GSE38832, GSE17537).
Independent prognostic factor of the biosignature
To further estimate the prognostic significance of our immune gene risk model in the entire cohort, we performed univariate, as well as multivariate Cox proportional hazards regression analyses of prognostic factors. Pathological stage, T stage, distant metastasis, as well as lymph node metastasis were treated as categorical variables, with age being treated as a continuous variable. Factors in which P was less than 0.05 were identified as independent prognostic variables according to the univariate and multivariate analyses.
Construction of prognostic nomogram
Nomograms are extensively employed to estimate a cancer patient’s prognosis (25). In this study, we developed a nomogram to explore the OS of CC patients over a 1-, 2-, and 3-year period. Our nomogram hinged on the independent prognostic factors determined by the multivariate evaluation, and the calibration curve of the nomogram was plotted to examine its estimation probabilities relative to the observed rates. Age, T model, gene biosignature, the combined model constituting T, as well as the gene biosignature were compared with the decision curve analysis (26).
Clinical application of the model
To explore the estimation potential of this model of CC patients, we evaluated the relationship between our model (risk gene level and risk score) and the clinical characteristics (lymph node metastasis, age, T stage, sex, pathological stage, as well as distant metastasis) of the entire cohort.
Analysis of the relative proportions of immune cell type fractions
We employed the online analytical portal CIBERSORT deconvolution algorithm in the quantification of the relative percentages of 22 tumor-infiltrating immune cells (TIICs) with the default statistical parameter (15). Samples with P>0.05 were excluded. The differences, as well as the correlations among TIICs, were evaluated by using the “ggplot2” and “corrplot” packages of the R software. We examined the differences of the proportions of the 22 TIICs between high- and low-risk groups by Wilcoxon rank-sum test. P<0.05 signified statistical significance. Meanwhile, we also used the TIMER to evaluate the link between the abundance of immune cell invasion and the risk score based on Pearson correlation analysis (27).
Immunophenoscore (IPS) analysis
In this part of the study, we derived the IPS of a CC patient without bias by machine learning, which was determined by effector cells, immunosuppressive cells, MHC molecules, and immunomodulators, thus adding up to 4 major categories of genes (28). The implementation of the R code is available at GitHub (https://github.com/icbi-lab/Immunophenogram/blob/master/IPS.R). We then further compared the 2 CC patient groups through the expression of PD1 and related genes, the IPS, gene response to ICIs supplied by The Cancer Immunome Atlas (TCIA), and by observing differences between the TME and the signature. The IPS ranged from 0 to 10 and reflects the TME of the CC patients. A high PD1_positive IPS reflects a potential response of anti-PD-1/PD-L1 therapy.
Statistical analysis
Statistical analyses and visualization were performed using the R software (version 3.6.3) and X-tile software (version 3.6.1). The log-rank test was used in the Kaplan-Meier survival analysis. Student’s t-test and Kruskal-Wallis test were employed for statistical comparison. Univariate and multivariate Cox proportional hazard regression analyses were used to identify independent prognostic factors related to survival. ROC curves were conducted by “timeROC”. If not specified above, a P value <0.05 was considered statistically significant.
Results
The association between stromal/immune scores, clinical features, and OS in CC patients
The overall study flow chart is summarized in Figure S1. As per the ESTIMATE algorithm, the stromal scores ranged between −2,265.66 and 1,992.06, and the immune scores between −944.93 to 3,052.71. We examined the link between the stromal/immune scores and the clinical characteristics of the patients. The data demonstrated a marked negative correlation between immune score and pathological stage (P=0.009), distant metastasis (P=0.001), and the subdivision (P<0.001). But the immune scores were not associated with the remaining two clinical characteristics (P>0.05) (Figure 1A-1E). On the contrary, the stromal scores were not remarkably linked to any clinical manifestations (P>0.05) (Figure 1F-1J). Also, the relationship between ESTIMATE scores and clinical characteristics were analyzed (Figure 1K-1O). Moreover, to investigate the association between stromal/immune scores and prognosis, the 316 patients with low stromal scores, and the 107 patients with high stromal scores, were grouped together via the cut-off value of −86.6, which was generated by a method based on X-tile plots (22) (Figure S2). Similarly, the 313 patients who had high immune scores, and the 110 patients exhibiting low immune scores, were clustered together using the cut-off value of 30.2. In the immune scores cohort, Kaplan-Meier survival curves demonstrated that the OS of patients in the high score group was remarkably shorter than the patients in the low score group (log-rank test P=0.017) (Figure 1P). By contrast, ESTIMATE scores were not associated with OS, for the stromal scores, the findings suggested that patients exhibiting high stromal scores were markedly linked with a lower OS outcome (log-rank test P=0.041) (Figure 1Q,1R).
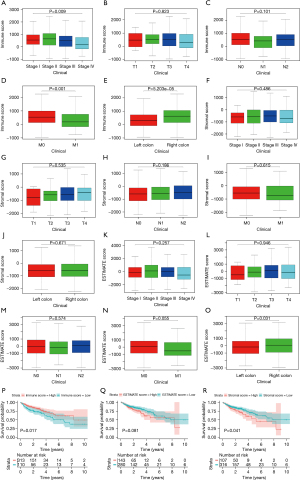
Differential expression of IRGs based on stromal/immune scores
To investigate the differences in gene expression between high- and low-immune/stromal score groups, heatmaps were also used to show different gene expression patterns of different cases, which were part of low or high stromal/immune scores groups (Figure 2A,2B). Also, we showed the distribution of the DEGs by using volcano maps in both the −log10 (FDR) and log2FC dimensions, as well as stromal scores (Figure 2C,2D). The comparison of the overall gene expression of data from the TCGA database between the high- and low- immune/stromal score groups using ‘edger’ revealed 47 upregulated genes and 1,368 downregulated genes (log2FC >1, FDR <0.05). To specifically investigate changes of IRGs, 220 intersection immune genes were chosen for further assessment based on the ImmPort portal (overlap zone in Figure 2E,2F). To explore the pathways and prospective functions of these IRGs, GO and KEGG assessments were also carried out. Figure 2G,2H was made to show the top terms of GO and KEGG, including leukocyte chemotaxis, cell chemotaxis, plasma membrane external side, receptor ligand activity, MHC class II protein complex, viral protein crosstalk with cytokine and cytokine receptor of KEGG, cytokine activity of GO, and cytokine-cytokine receptor crosstalk, which were all associated with the immune pathway.
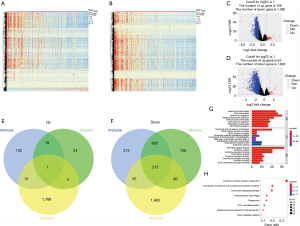
Development and verification of the prognostic risk model
We first applied univariate Cox evaluation to screen 9 IRGs that were linked to the prognosis of the training cohort (Figure 3A). Secondly, Lasso regression was used to obtain 6-candidate prognostic IRGs (Figure 3B,3C). Ultimately, we utilized multivariate Cox assessment to obtain the most appropriate 4 IRGs, including PDIA2, NAFTC1, VEGFC, and CD1B. Three of the 4 IRGs were considered as high hazard genes, all of which were upregulated DEGs, while CD1B was the only low hazard gene (Figure 3D). The risk score of every patient was computed using the following formula: risk score = (0.7937 × expression value of PDIA2) + (0.8356 × expression value of NAFTC1) + (1.159 × expression value of VEGFC) + (−1.7446 × expression value of CD1B). According to the best cut‐off, all training cohort subjects were stratified into 2 different groups: a high-risk group (n=34) and a low-risk group (n=178). The Kaplan-Meier curve based on the log-rank test for OS showed a remarkable difference between the 2 risk groups (P<0.0001) (Figure 3E). Among the training cohort, the median OS time in the low-risk group was more than 10 years, however, in the high-risk group it was less than 5 years. The AUC values of our prognostic risk score model were both 0.78 at 1- and 3-year OS times (Figure 3F). The risk score distribution plot, scatter plots of survival status, and the heatmap between the high- and low-risk groups are indicated in Figure 3G-3I.
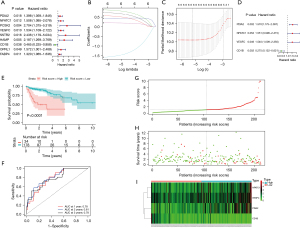
To confirm the precision of our risk model, another risk model was additionally created using the testing dataset, the whole TCGA dataset, and the 3 GEO datasets. We calculated each patient’s risk score from the testing dataset, as well as the whole dataset, and then clustered the patients of the 2 datasets into 2 groups using the optimal cut-off. The results revealed that in the testing cohort the AUC values of 1- and 3-year OS were 0.71 and 0.67, respectively (Figure 4A), while in the whole cohort they were 0.75 and 0.72, respectively (Figure 4B). There were remarkable diversities in the survival curves between the 2 risk groups (P<0.05) (Figure 4C,4D). The risk score distribution plot, scatter plots of survival status, and the gene expression heatmap of the 2 datasets are displayed in Figure 4E-4J.
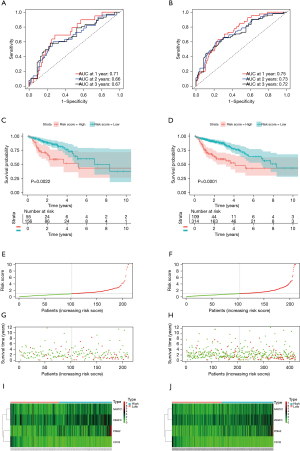
The AUC values for 1-, 2-, and 3-year OS predictions for the 3 validation GEO sets included 0.62, 0.54, and 0.53 for GSE17536 (Figure 5A), which may have resulted from different measurement methods between TCGA and GEO datasets; 0.62, 0.61, and 0.54 for GSE38832 (Figure 5B); and 0.71, 0.68, and 0.71 for GSE17537 (Figure 5C). In the 3 GEO cohorts, similarly remarkable differences in survival curves between the high- and low-risk groups were reported, where patients in the high-risk group exhibited a shorter OS time compared to those in the low-risk group (P<0.05) (Figure 5D-5F). Most importantly, these findings implied that our risk score prognosis model had good robustness and efficiency.
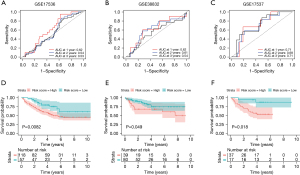
Independent prognostic value analysis
In this study, the results of all our univariate and multivariate regression assessments proved that our biosignature could act as an independent factor for determining CC (P<0.05), and that it was distinct from other clinical parameters (T stage, age, lymph node metastasis, gender, pathological stage, as well as distant metastasis) (Figure 6A,6B). All results also demonstrated that the prognostic biosignature could be utilized to independently estimate the prognosis of CC patients. Following this discovery, the risk score, age, and T stage were visualized by developing a nomogram. Nomograms representing the 1-, 2- and 3-year OS rate of the whole cohort are displayed in Figure 6C. In accordance with the predictive value and observational value, the calibration curve also performed satisfactorily in determining the possibility of 3-year survival (Figure 6D). Moreover, decision curve analysis was employed to compare different models, such as the age model, T model, risk score model, and combined nomogram model in regard to the 1-, 2-, and 3-year OS of CC patients. This data revealed that the combined model performed better than the individual constituents. These results all demonstrated that the nomogram expressed good precision in estimating the OS of CC patients (Figure 6E).
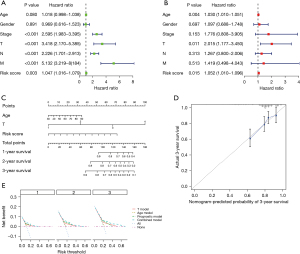
Clinical utility of the model
To further evaluate the association between the composition of our model and clinical variables, we also performed the Chi-square test. The results demonstrated that across the whole dataset, the values of PDIA2 were remarkably higher in cases involving males, advanced lymph node metastasis, advanced distant metastasis, and advanced-stage disease (P<0.05) (Figure 7A-7D). However, the values of CD1B were notably lower in patients with distant metastasis, advanced lymph node metastasis, and advanced-stage disease (Figure 7E-7H). Similar to the PDIA2, as the expression of VEGFC increased, the T stage and lymph node metastasis increased (P<0.05) (Figure 7I,7J). And with advanced T stage, the risk scores were significantly higher (Figure 7K). These results revealed that the IRGs in our prognostic biosignature model were linked to the process, as well as the progression of CC.
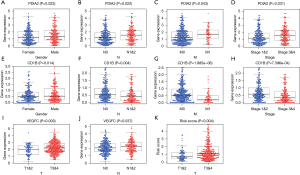
Relationship between immune cell invasion and the prognostic risk model
By using the CIBERSORT algorithm, we predicted the difference of immune invasion between low- and high-risk CC patients in the 22 subpopulations of immune cells. The percentage of immune cells in CC differed remarkably between the high- and low-risk groups, as indicated in Figure 8A,8B. Figure 8C also indicates a high proportion of the plasma cells, activated memory CD4 T cells, resting memory CD4 T cells, and resting dendritic cells mainly invaded patients in the low-risk group. On the contrary, a high proportion of activated dendritic cells invaded patients in the low-risk group as well. Furthermore, the percentage of distinct TIICs exhibited a weak to moderate association (Figure 8D). These data implied that the different immune cell invasions in CC patients could be utilized as a prognostic indicator, as well as immunotherapy targets. Moreover, we explored if the risk score could reflect the TME according to the TIMER database. Higher risk scores were associated with growing numbers of TIICs, consisting of B-cells, CD4 T-cells, and macrophages (P<0.05) (Figure 9).
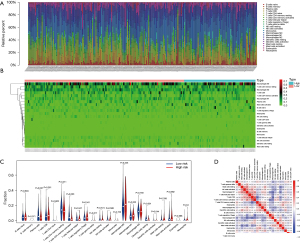
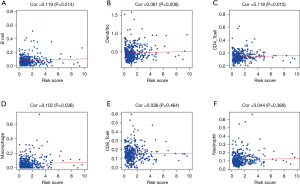
The signature and the response to ICIs
In our study, we investigated the correlation between the IPS and the risk signature of the 4 IRGs (Figure 10). We found that in the CTLA4_ positive + PD-1_ negative and CTLA4_negative + PD-1_negative types, the low-risk group exhibited a higher IPS than the high-risk group (P<0.05) (Figure 10A). Interestingly, there was only a significant difference in expression between these 2 groups (P<0.05) (Figure 10B). These results indicate that low-risk patients with the 4 identified IRGs had a better response to ICIs.
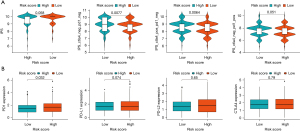
Discussion
CC remains a common digestive system tumor (1), and recent research has documented how a TME plays a key role in the progression and invasion of tumors (29-31). For this reason, our study primarily focused on analyzing differentially expressed IRGs based on the TME of CC patients. These data were then used to establish a signature related to the immune system to predict the OS of CC patients. Additionally, we verified the effectiveness of this prognostic signature in internal and external validation datasets.
The Kaplan-Meier results revealed that low immune scores and high stromal scores were related to a worse OS. For this reason, we classified the patients into high-score and low-score groups to identify the prognostic potential for the differentially expressed IRGs. In the training cohort, through the results of our univariate analysis by using Cox and LASSO, as well as the results of our multivariate Cox analysis, we were able to establish an immune-related 4-mRNA signature, which was then employed to compute the risk scores of CC samples. The AUC values for the 1- and 3-year OS in our prognostic risk model for the training cohort were 0.78 and 0.78, respectively. Additionally, the risk model was verified in the testing cohort, the entire cohort, and the GEO cohorts. The results of our study thus show that the genes (PDIA2, NAFTC1, VEGFC, and CD1B) in our prognostic biosignature may be used as prognostic markers for CC, and show great potential to be used in clinical applications.
In recent years, several studies have developed CC prognosis classifiers based on the expression of multiple genes to accurately predict the prognosis of CC. For example, some studies constructed a model by calculating immune and stromal scores by using the ESTIMATE algorithm to identify DEGs (32-34). Other studies, based on using IRGs to identify DEGs, have also been increasing in number (35-37). However, these studies do not combine the TME with IRGs. In our study, we first identified DEGS related to immune and stromal scores in the TME. We then focused on the prognostic differentially expressed IRGs and developed a novel prognostic model related to immunity. CC patients without OS data or who had a follow-up time <30 days were excluded to avoid bias toward survival. Based on our univariate Cox and LASSO analysis, as well as multivariate Cox regression analysis, we then concluded that our prognostic biosignature could be applied as an independent prognostic factor. Using the prognostic signature along with a patient’s age and T stage, we built one nomogram to predict OS for CC individuals.
Among the 4 IRGs, to our best knowledge, there have not been any reports about the relationship between protein disulfide isomerase family a member 2 (PDIA2) and cancer prognosis. Until now, its main function has been associated with protein processing and translocation, although previous studies also suggest that PDIA2 may be involved in antigen presentation (38). The overexpression of nuclear factor of activated T-cells, cytoplasmic 1 (NAFTC1) has been documented as being associated with a lower OS and metastatic capacity in CC (39,40). It has also been noted to promote cell proliferation and growth in pancreatic cancer. All of these factors indicate that NFATc1 may play an important role in carcinogenesis (41). Vascular endothelial growth factor C (VEGFC) is one kind of lymphangiogenesis inducer, with its overexpression reported to modulate lymphangiogenesis and stimulate metastasis in cancer cells (42). Previous studies have demonstrated that VEGFC, by combining with CCL21/CCR7, promotes colorectal cancer invasion by disrupting the endothelial lymphatic barrier and lymphangiogenesis in cases of pancreatic, breast, and lung cancer (43-46). The main function of CD1B is to encode a transmembrane glycoprotein of the CD1 family, and its expression has been associated with the prognosis for localized prostate cancer (47). Despite this, there were no reports about the relationship between CD1B and CC. Therefore, detailed interactions between the IRGs and CC require further analysis.
The characteristics of immune infiltration are of great significance for studying the interaction between immunity and tumors. Given the importance and significance of the immune system during the progression and process of cancer, we calculated the percentage of 22 IRGs of every CC individual to investigate the correlation between TIICs and the risk score. TIMER database results revealed that the risk score was positively relevant to the invasion of B cells, macrophages, and CD4 T-cells. However, the data of CIBERSORT indicated that resting memory CD4 T cells, activated memory CD4 T cells, plasma cells, activated dendritic cells, and resting dendritic cells invaded more in the low-risk group. This differed from the TIMER database, and was the result of a difference between the 2 algorithms. Studies have reported that macrophages are associated with colorectal cancer progression and M2-like macrophages may induce CC cell invasion via matrix metalloproteinases (48,49). Furthermore, eliminating disease-causing CD4 T-cells and inducing anti-tumor CD8 T-cells activity can inhibit the occurrence of CC (50). Nevertheless, the 2 algorithms indicated that our risk score was related to immune status.
Through exploring the association between the IPS and biosignature, the expression of PD1 was found to be significantly higher in the high-risk group. Furthermore, the IPS increased more in the low-risk group when compared to the high-risk group, although the P value was not significant. It is suggested that the biosignature may represent the immunogenic TME of CC. From this we can deduce that CC patients with a lower risk score had a better response to ICIs.
Taken together, we established a novel TME-based IRG risk score model to estimate the prognosis of CC patients. However, the present study has some limitations. Firstly, our risk model was only verified using one dataset, and for this reason more samples should be analyzed to verify the general applicability of our model. Secondly, we did not perform in vivo or in vitro studies to validate our results, which means our study lacked research using actual CC cells or animal models.
Conclusions
In conclusion, we identified and verified 4 novel TME-based IRGs to estimate OS of CC patients. These 4 IRGs included PDIA2, NAFTC1, VEGFC, and CD1B. Most notably, the immune infiltration of our biosignature was evaluated in our study, and the prognostic biosignature reflected the immune status of the CC samples.
Acknowledgments
We appreciate all the members of the department of Hepatobiliary surgery who gave us support and advice in this study.
Funding: This study was financially supported by the Dalian University of Technology and Liaoning Cancer Hospital Medical Engineering Cross-Research Fund (No. LD202002) and the Natural Science Foundation of Liaoning Province (No. 20180550781).
Footnote
Reporting Checklist: The authors have completed the TRIPOD reporting checklist. Available at https://dx.doi.org/10.21037/jgo-21-522
Conflicts of Interest: All authors have completed the ICMJE uniform disclosure form (available at https://dx.doi.org/10.21037/jgo-21-522). The authors have no conflicts of interest to declare.
Ethical Statement: The authors are accountable for all aspects of the work in ensuring that questions related to the accuracy or integrity of any part of the work are appropriately investigated and resolved. The study was conducted in accordance with the Declaration of Helsinki (as revised in 2013). Institutional ethical approval and informed consent were waived.
Open Access Statement: This is an Open Access article distributed in accordance with the Creative Commons Attribution-NonCommercial-NoDerivs 4.0 International License (CC BY-NC-ND 4.0), which permits the non-commercial replication and distribution of the article with the strict proviso that no changes or edits are made and the original work is properly cited (including links to both the formal publication through the relevant DOI and the license). See: https://creativecommons.org/licenses/by-nc-nd/4.0/.
References
- Bray F, Ferlay J, Soerjomataram I, et al. Global cancer statistics 2018: GLOBOCAN estimates of incidence and mortality worldwide for 36 cancers in 185 countries. CA Cancer J Clin 2018;68:394-424. [Crossref] [PubMed]
- André T, Boni C, Mounedji-Boudiaf L, et al. Oxaliplatin, fluorouracil, and leucovorin as adjuvant treatment for colon cancer. N Engl J Med 2004;350:2343-51. [Crossref] [PubMed]
- Basile D, Garattini SK, Bonotto M, et al. Immunotherapy for colorectal cancer: where are we heading? Expert Opin Biol Ther 2017;17:709-21. [Crossref] [PubMed]
- Ganesh K, Stadler ZK, Cercek A, et al. Immunotherapy in colorectal cancer: rationale, challenges and potential. Nat Rev Gastroenterol Hepatol 2019;16:361-75. [Crossref] [PubMed]
- Dasari A, Messersmith WA. New strategies in colorectal cancer: biomarkers of response to epidermal growth factor receptor monoclonal antibodies and potential therapeutic targets in phosphoinositide 3-kinase and mitogen-activated protein kinase pathways. Clin Cancer Res 2010;16:3811-8. [Crossref] [PubMed]
- Locy H, de Mey S, de Mey W, et al. Immunomodulation of the Tumor Microenvironment: Turn Foe Into Friend. Front Immunol 2018;9:2909. [Crossref] [PubMed]
- Gajewski TF, Schreiber H, Fu YX. Innate and adaptive immune cells in the tumor microenvironment. Nat Immunol 2013;14:1014-22. [Crossref] [PubMed]
- Chen YP, Zhang Y, Lv JW, et al. Genomic Analysis of Tumor Microenvironment Immune Types across 14 Solid Cancer Types: Immunotherapeutic Implications. Theranostics 2017;7:3585-94. [Crossref] [PubMed]
- Marshall HT, Djamgoz MBA. Immuno-Oncology: Emerging Targets and Combination Therapies. Front Oncol 2018;8:315. [Crossref] [PubMed]
- Popovic A, Jaffee EM, Zaidi N. Emerging strategies for combination checkpoint modulators in cancer immunotherapy. J Clin Invest 2018;128:3209-18. [Crossref] [PubMed]
- Hanahan D, Weinberg RA. Hallmarks of cancer: the next generation. Cell 2011;144:646-74. [Crossref] [PubMed]
- Singh PP, Sharma PK, Krishnan G, et al. Immune checkpoints and immunotherapy for colorectal cancer. Gastroenterol Rep (Oxf) 2015;3:289-97. [Crossref] [PubMed]
- Markman JL, Shiao SL. Impact of the immune system and immunotherapy in colorectal cancer. J Gastrointest Oncol 2015;6:208-23. [PubMed]
- Yoshihara K, Shahmoradgoli M, Martínez E, et al. Inferring tumour purity and stromal and immune cell admixture from expression data. Nat Commun 2013;4:2612. [Crossref] [PubMed]
- Newman AM, Liu CL, Green MR, et al. Robust enumeration of cell subsets from tissue expression profiles. Nat Methods 2015;12:453-7. [Crossref] [PubMed]
- Şenbabaoğlu Y, Gejman RS, Winer AG, et al. Tumor immune microenvironment characterization in clear cell renal cell carcinoma identifies prognostic and immunotherapeutically relevant messenger RNA signatures. Genome Biol 2016;17:231. [Crossref] [PubMed]
- Alonso MH, Aussó S, Lopez-Doriga A, et al. Comprehensive analysis of copy number aberrations in microsatellite stable colon cancer in view of stromal component. Br J Cancer 2017;117:421-31. [Crossref] [PubMed]
- Shah N, Wang P, Wongvipat J, et al. Regulation of the glucocorticoid receptor via a BET-dependent enhancer drives antiandrogen resistance in prostate cancer. Elife 2017;6:27861. [Crossref] [PubMed]
- Priedigkeit N, Watters RJ, Lucas PC, et al. Exome-capture RNA sequencing of decade-old breast cancers and matched decalcified bone metastases. JCI Insight 2017;2:e95703 [Crossref] [PubMed]
- Wagner GP, Kin K, Lynch VJ. Measurement of mRNA abundance using RNA-seq data: RPKM measure is inconsistent among samples. Theory Biosci 2012;131:281-5. [Crossref] [PubMed]
- Bhattacharya S, Dunn P, Thomas CG, et al. ImmPort, toward repurposing of open access immunological assay data for translational and clinical research. Sci Data 2018;5:180015 [Crossref] [PubMed]
- Camp RL, Dolled-Filhart M, Rimm DL. X-tile: a new bio-informatics tool for biomarker assessment and outcome-based cut-point optimization. Clin Cancer Res 2004;10:7252-9. [Crossref] [PubMed]
- Robinson MD, McCarthy DJ, Smyth GK. edgeR: a Bioconductor package for differential expression analysis of digital gene expression data. Bioinformatics 2010;26:139-40. [Crossref] [PubMed]
- Yu G, Wang LG, Han Y, et al. clusterProfiler: an R package for comparing biological themes among gene clusters. OMICS 2012;16:284-7. [Crossref] [PubMed]
- Iasonos A, Schrag D, Raj GV, et al. How to build and interpret a nomogram for cancer prognosis. J Clin Oncol 2008;26:1364-70. [Crossref] [PubMed]
- Vickers AJ, Elkin EB. Decision curve analysis: a novel method for evaluating prediction models. Med Decis Making 2006;26:565-74. [Crossref] [PubMed]
- Li T, Fan J, Wang B, et al. TIMER: A Web Server for Comprehensive Analysis of Tumor-Infiltrating Immune Cells. Cancer Res 2017;77:e108-10. [Crossref] [PubMed]
- Charoentong P, Finotello F, Angelova M, et al. Pan-cancer Immunogenomic Analyses Reveal Genotype-Immunophenotype Relationships and Predictors of Response to Checkpoint Blockade. Cell Rep 2017;18:248-62. [Crossref] [PubMed]
- Radisky DC, Bissell MJ. Cancer. Respect thy neighbor! Science 2004;303:775-7. [Crossref] [PubMed]
- Wu T, Dai Y. Tumor microenvironment and therapeutic response. Cancer Lett 2017;387:61-8. [Crossref] [PubMed]
- Gonzalez H, Hagerling C, Werb Z. Roles of the immune system in cancer: from tumor initiation to metastatic progression. Genes Dev 2018;32:1267-84. [Crossref] [PubMed]
- Luo R, Guo W, Wang H. A comprehensive analysis of tumor microenvironment-related genes in colon cancer. Clin Transl Oncol 2021;23:1769-81. [Crossref] [PubMed]
- Wu B, Tao L, Yang D, et al. Development of an Immune Infiltration-Related Eight-Gene Prognostic Signature in Colorectal Cancer Microenvironment. Biomed Res Int 2020;2020:2719739 [Crossref] [PubMed]
- Zhang X, Zhao H, Shi X, et al. Identification and validation of an immune-related gene signature predictive of overall survival in colon cancer. Aging (Albany NY) 2020;12:26095-120. [Crossref] [PubMed]
- Li M, Wang H, Li W, et al. Identification and validation of an immune prognostic signature in colorectal cancer. Int Immunopharmacol 2020;88:106868 [Crossref] [PubMed]
- Zhang H, Qin C, Gan H, et al. Construction of an Immunogenomic Risk Score for Prognostication in Colon Cancer. Front Genet 2020;11:499. [Crossref] [PubMed]
- Chen H, Luo J, Guo J. Development and validation of a five-immune gene prognostic risk model in colon cancer. BMC Cancer 2020;20:395. [Crossref] [PubMed]
- Walker AK, Soo KY, Levina V, et al. N-linked glycosylation modulates dimerization of protein disulfide isomerase family A member 2 (PDIA2). FEBS J 2013;280:233-43. [Crossref] [PubMed]
- Ding W, Tong Y, Zhang X, et al. Study of Arsenic Sulfide in Solid Tumor Cells Reveals Regulation of Nuclear Factors of Activated T-cells by PML and p53. Sci Rep 2016;6:19793. [Crossref] [PubMed]
- Tripathi MK, Deane NG, Zhu J, et al. Nuclear factor of activated T-cell activity is associated with metastatic capacity in colon cancer. Cancer Res 2014;74:6947-57. [Crossref] [PubMed]
- Buchholz M, Schatz A, Wagner M, et al. Overexpression of c-myc in pancreatic cancer caused by ectopic activation of NFATc1 and the Ca2+/calcineurin signaling pathway. EMBO J 2006;25:3714-24. [Crossref] [PubMed]
- Skobe M, Hawighorst T, Jackson DG, et al. Induction of tumor lymphangiogenesis by VEGF-C promotes breast cancer metastasis. Nat Med 2001;7:192-8. [Crossref] [PubMed]
- Tacconi C, Correale C, Gandelli A, et al. Vascular endothelial growth factor C disrupts the endothelial lymphatic barrier to promote colorectal cancer invasion. Gastroenterology 2015;148:1438-51.e8. [Crossref] [PubMed]
- Zhao B, Cui K, Wang CL, et al. The chemotactic interaction between CCL21 and its receptor, CCR7, facilitates the progression of pancreatic cancer via induction of angiogenesis and lymphangiogenesis. J Hepatobiliary Pancreat Sci 2011;18:821-8. [Crossref] [PubMed]
- Tutunea-Fatan E, Majumder M, Xin X, et al. The role of CCL21/CCR7 chemokine axis in breast cancer-induced lymphangiogenesis. Mol Cancer 2015;14:35. [Crossref] [PubMed]
- Xu Y, Liu L, Qiu X, et al. CCL21/CCR7 promotes G2/M phase progression via the ERK pathway in human non-small cell lung cancer cells. PLoS One 2011;6:e21119 [Crossref] [PubMed]
- Lee CH, Chen LC, Yu CC, et al. CD1B Prognostic Value of in Localised Prostate Cancer. Int J Environ Res Public Health 2019;16:4723. [Crossref] [PubMed]
- Wei C, Yang C, Wang S, et al. Crosstalk between cancer cells and tumor associated macrophages is required for mesenchymal circulating tumor cell-mediated colorectal cancer metastasis. Mol Cancer 2019;18:64. [Crossref] [PubMed]
- Vinnakota K, Zhang Y, Selvanesan BC, et al. M2-like macrophages induce colon cancer cell invasion via matrix metalloproteinases. J Cell Physiol 2017;232:3468-80. [Crossref] [PubMed]
- Gu T, De Jesus M, Gallagher HC, et al. Oral IL-10 suppresses colon carcinogenesis via elimination of pathogenicCD4+ T-cells and induction of antitumor CD8+ T-cell activity. Oncoimmunology 2017;6:e1319027 [Crossref] [PubMed]