Identification of potential core genes in gastric cancer using bioinformatics analysis
Introduction
Helicobacter pylori (H. pylori) infection, obesity, and excessive intake of edible salt are the main causes of gastric cancer (1). It is well known that bacterial infection is closely related to the occurrence of atrophic gastritis, intestinal metaplasia, dysplasia, and gastric cancer (2). However, epidemiological studies have shown that mass eradication of H. pylori could make pre-existing diseases irreversible (3). Gastric cancer is one of the most heterogeneous cancers in which each cancer patient exhibits a distinct genetic and molecular profile (4). Because they are economical, convenient, and noninvasive, the detection of conventional serum tumor biomarkers (e.g., CEA, CA19-9, and CA72-4) has been widely employed in the diagnosis and evaluation of GC. However, due to their poor specificity and sensitivity, these molecular markers cannot meet the demand of early GC detection. Hence, new and reliable tumor biomarkers are desperately needed. Studies have found that microRNA and methylated DNA can be used as two types of potential molecular biomarkers for the diagnosis of GC (5). In addition, Genome wide study of cancer transcriptomes has also identified many new candidate genes. In contrast, the lists of candidate gene generated from comprehensive gene analysis vary considerably among individual studies. On the other hand, with regard to molecular targeting agents, their target molecules and related genes would be suitable for predicting treatment response more accurately (6). Unfortunately, although extensive research on molecular biomarkers, most of the biomarkers that have been identified have failed in validation studies. Almost advanced GC patients still cannot be treated with a targeted therapy, and no diagnostic markers for secondary prevention have been found. Therefore, it is particularly important to study the molecular biological mechanism of gastric cancer and further identify specific molecular markers or treatment targets (7). In our study, we decided to begin with the discovery of new biological indicators that affect the formation of gastric cancer. We used a bioinformatics method to analyze the expression profile data of differentially expressed genes (DEGs) in gastric cancer and adjacent tissues extracted from Gene Expression Omnibus (GEO). Functional annotation and enrichment pathway analysis of these selected differential genes were carried out by the Database for Annotation,Visualization and Integrated Discovery (DAVID). We then used the protein-protein interaction (PPI) network to select hub genes that are closely related to gastric cancer. Finally, we performed survival analyses of these genes using the Kaplan-Meier plotter bioinformatics analysis platform.
In recent years, abnormal miRNA expression has been found in gastric cancer, indicating that miRNA is also involved in the occurrence of gastric cancer (8). MicroRNAs (miRNAs) are a type of single-stranded non-coding small RNAs that are widely present in eukaryotic cells and are highly conserved during evolution. miRNA is the largest type of gene expression transcriptional regulator factors, which are involved in regulating various biological activities of the human body. Disorders of miRNA expression can lead to diabetes, hypertension and other diseases (9,10). The expression of miRNA is also related to the occurrence, development and metastasis of breast cancer (11), lung cancer (12), osteosarcoma (13), liver cancer (14) and other malignant tumors. Therefore, greater understanding of the roles of miRNAs in gastric carcinogenesis could provide insights into the mechanisms of tumor development and identify diagnostic biomarkers and therapeutic targets. Therefore, in our study, using the OncomiR database to further predict microrNAs is of great importance to provide new ideas and solutions for the treatment of gastric cancer and guide future research. We present the following article in accordance with the STREGA reporting checklist (available at https://dx.doi.org/10.21037/jgo-21-628).
Methods
Data extraction
The GEO (https://www.ncbi.nlm.nih.gov/) is a gene expression database created by the National Center for Biotechnology Information (NCBI), which contains high-throughput gene expression data submitted by global research institutions. In this study, three gene expression profiles (GSE29272, GSE33651, and GSE54129) were selected from the GEO. The GSE29272 dataset included 134 gastric cancer samples and 134 non-cancerous samples. The GSE33651 dataset consisted of 40 gastric cancer samples and 12 non-cancerous samples. The GSE54129 dataset contained 111 gastric cancer samples and 21 non-cancerous samples. The study was conducted in accordance with the Declaration of Helsinki (as revised in 2013).
Data processing of DEGs
The DEGs between gastric cancer and normal samples were detected using the GEO2R online analysis tool (https://www.ncbi.nlm.nih.gov/geo/geo2r/), and the adjusted P value and |log FC| were calculated. The adjusted P<0.01 and |log FC| ≥1.0 were the cutoff criteria for DEGs. The intersecting part in the three datasets was identified using the Venn diagram webtool (http://bioinformatics.psb.ugent.be/webtools/Venn/).
Gene Ontology (GO) and KEGG pathway analyses of DEGs
Functional enrichment analyses involve the mapping of genes with similar functions and association with biological phenotypes in a gene list. GO annotations fall into three broad categories: Molecular Function (MF), Biological Process (BP), and Cellular Components (CC). KEGG is a widely used database that stores comprehensive data on biological pathways, diseases, genomics, chemicals, and drugs. In this study, GO analysis and KEGG pathway enrichment analysis of DEGs were conducted using the Database for Annotation, Visualization and Integrated Discovery (DAVID) tools (https://david-d.ncifcrf.gov). P<0.01 and gene counts ≥2 were considered statistically significant.
PPI network construction and hub genes identification
The Search Tool for the Retrieval of Interacting Genes (STRING) database (https://string-db.org/) is designed to analyze the PPI network of DEGs, of which a combined score >0.4 was set as the cutoff criterion. Next, the PPI network was visualized using Cytoscape (version 3.7.2). The Molecular Complex Detection (MCODE) of Cytoscape was used to analyze modules in the PPI networks with a degree cut-off =2, node score cut-off =0.2, max depth =100, and k-score =2. Functional enrichment analyses of the genes in the modules were performed using DAVID. The degree of each protein node was calculated using a plugin in Cytoscape. In our research, the top 10 genes were identified as hub genes.
Transcriptional expression levels of hub genes in gastric cancer
The messenger ribonucleic acid (mRNA) expressions of the 10 hub genes between gastric cancer tissues and normal tissues were analyzed using the Gene Expression Profiling Interactive Analysis (GEPIA) online database (http://gepia.cancer-pku.cn/).
Survival analysis of hub genes
The Kaplan-Meier plotter (http://kmplot.com/analysis/) was used to evaluate the prognostic values of the 10 potential hub genes. Eight hundred and seventy-six gastric cancer patients were available for overall survival analysis.
COL4A1 and microRNAs relationship prediction
To understand the role of COL4A1 in the development of gastric cancer, we predicted microRNAs that could interact with COL4A1 via the Target Scan 7.2 online database (http://www.targetscan.org/).
Expression levels of microRNAs in gastric cancer and the relationships with tumor stage, grade, and prognosis
The expression levels of microRNAs in gastric cancer tissues and normal tissues were analyzed based on the OncomiR database (http://www.oncomir.org/), and the relationships with tumor stage, grade, and prognosis were explored.
Statistical analysis
The default parameters in the GEO2R statistical tool was used to analyze the identification of DEGs in the transcription profile.
Results
Identification of DEGs
In our study, we selected three gene expression profiles (GSE29272, GSE33651, and GSE54129). Among them, GSE29272 contained 134 gastric cancer samples and 134 normal samples, GSE33651 included 40 gastric cancer samples and 12 normal samples, and GSE54129 had 111 gastric cancer samples and 21 normal samples (Table 1). Based on the cutoff criteria, a total of 351 DEGs were identified in the gene expression profile GSE29272, including 176 upregulated genes and 175 downregulated genes. In GSE33651, 1,836 DEGs were identified, including 1,401 upregulated genes and 435 downregulated genes. As for GSE54129, 3,897 DEGs were identified, including 1,830 upregulated genes and 2,067 downregulated genes. As shown in the Venn diagram, 78 DEGs were found to be overlapping between the three datasets, of which 53 were significantly upregulated and 25 were downregulated (Figure 1).
Table 1
Dataset ID | Gastric cancer | Normal | Total number |
---|---|---|---|
GSE29272 | 134 | 134 | 268 |
GSE33651 | 111 | 21 | 132 |
GSE54129 | 40 | 12 | 52 |
GEO, Gene Expression Omnibus.
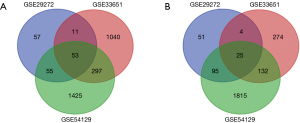
Functional enrichment analyses of DEGs
Functional enrichment analyses of the DEGs was performed using DAVID. The GO analysis results revealed that DEGs were significantly enriched in BPs, including extracellular matrix organization, extracellular structure organization, response to wounding, collagen fibril organization, collagen biosynthetic process, blood vessel development, vasculature development, wound healing, cell adhesion, and biological adhesion (Figure 2A). In the CC, the DEGs were enriched in the extracellular region part, extracellular region, extracellular matrix, proteinaceous extracellular matrix, extracellular matrix part, collagen, extracellular space, platelet alpha granule lumen, and cytoplasmic membrane (Figure 2B). MF analysis indicated that the DEGs were mainly enriched in extracellular matrix structural constituent, platelet-derived growth factor binding, structural molecule activity, growth factor binding, collagen binding, endopeptidase inhibitor activity, peptidase inhibitor activity, integrin binding, enzyme inhibitor activity, and protein complex binding (Figure 2C). Moreover, KEGG pathway analysis results revealed that DEGs were significantly enriched in focal adhesion, complement and coagulation cascades, and extracellular matrix (ECM)-receptor interaction (Figure 2D).
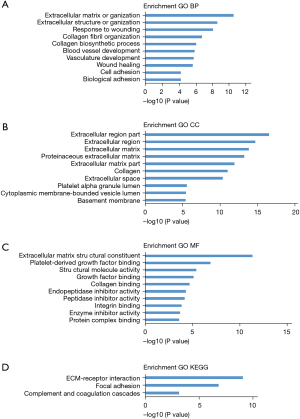
PPI network construction and hub genes identification
The STRING database was used to predict protein interactions among the DEGs. The PPI network of DEGs with 58 nodes and 268 edges was constructed using Cytoscape (Figure 3A). As shown in Figure 3B and Table 2, the top 10 genes in the PPI network were identified based on the connectivity degree, and the results revealed that Fibronectin 1 (FN1) was the gene with a degree of connectivity of 31, followed by collagen type I alpha 1 chain (COL1A1; degree =23), collagen type III alpha 1 chain (COL3A1; degree =23), TIMP metallopeptidase inhibitor 1 (TIMP1; degree =23), collagen type I alpha 2 chain (COL1A2; degree =22), secreted protein acidic and cysteine rich (SPARC; degree =21), C-X-C motif chemokine ligand 8 (CXCL8; degree =18), collagen type IV alpha 1 chain (COL4A1; degree =18), lysyl oxidase (LOX; degree =18), and serpin family E member 1 (SERPINE1; degree =18). Therefore, the top 10 genes are hub genes that we need to focus on. All of these hub genes were upregulated in gastric cancer.
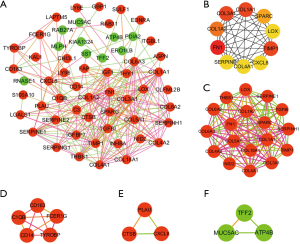
Table 2
Gene symbol | Gene description | Degree |
---|---|---|
FN1 | Fibronectin 1 | 31 |
COL1A1 | Collagen type I alpha 1chain | 23 |
COL3A1 | Collagen type III alpha 1 chain | 23 |
TIMP1 | TIMP metallopeptidase inhibitor 1 | 23 |
COL1A2 | Collagen type I alpha 2 chain | 22 |
SPARC | Secreted protein acidic and cysteine rich | 21 |
CXCL8 | C-X-C motif chemokine ligand 8 | 18 |
COL4A1 | Collagen type IV alpha 1 chain | 18 |
LOX | Lysyl oxidase | 18 |
SERPINE1 | Serpin family E member 1 | 18 |
Four modules were selected to analyze the PPI network by using MCODE (Figure 3C-3F). DAVID was then used to conduct functional enrichment analyses of the most significant module genes (score =15.647). The results showed that the GO terms and KEGG pathway of the module genes largely involved ECM and cell adhesion (Table 3).
Table 3
Category | Term | Description | Count | P value |
---|---|---|---|---|
BP term | GO:0030198 | Extracellular matrix organization | 9 | 1.13E-13 |
BP term | GO:0043062 | Extracellular structure organization | 9 | 4.43E-12 |
BP term | GO:0007155 | Cell adhesion | 8 | 7.32E-06 |
BP term | GO:0022610 | Biological adhesion | 8 | 7.38E-06 |
CC term | GO:0005576 | Extracellular region | 17 | 1.93E-12 |
CC term | GO:0044421 | Extracellular region part | 16 | 1.44E-15 |
CC term | GO:0031012 | Extracellular matrix | 16 | 2.81E-22 |
CC term | GO:0005578 | Proteinaceous extracellular matrix | 15 | 1.81E-20 |
MF term | GO:0005198 | Structural molecule activity | 10 | 2.55E-08 |
MF term | GO:0005201 | Extracellular matrix structural constituent | 9 | 6.15E-14 |
MF term | GO:0048407 | Platelet-derived growth factor binding | 5 | 6.60E-10 |
MF term | GO:0005518 | Collagen binding | 5 | 1.15E-07 |
KEGG pathway | hsa04512 | ECM-receptor interaction | 10 | 3.16E-15 |
KEGG pathway | hsa04510 | Focal adhesion | 10 | 1.02E-11 |
DEG, differentially expressed gene; KEGG, Kyoto Encyclopedia of Genes and Genomes.
Validation of mRNA expression of hub genes
The mRNA expressions of the 10 hub genes were further verified using the GEPIA database. The results indicated that the 10 genes were upregulated in gastric cancer, and the difference was statistically significant (P<0.05, Figure 4).
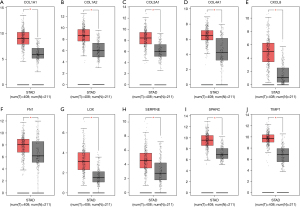
Survival analysis of hub genes
The Kaplan-Meier plotter was used to investigate the prognostic values of the 10 potential hub genes. Our results showed that high expressions of COL1A1 (P=8.2E-05) (Figure 5A), COL2A2 (P=0.0016) (Figure 5B), SERPINE1 (P=0.0013) (Figure 5C), COL4A1 (P=6.4E-07) (Figure 5D), TIMP1 (P=2.2E-10) (Figure 5E), FN1 (P=1.3E-05) (Figure 5F), SPARC (P=0.009) (Figure 5G), and were significantly correlated with worse survival probability for gastric cancer patients. However, COL3A1 and LOX were not notable markers of survival prognosis (P=0.069 and P=0.06, respectively) (Figure 5H,5I). In addition, one significantly upregulated gene, CXCL8 (P=1.4E-05) (Figure 5J), was found to be associated with favorable overall survival in gastric cancer patients.
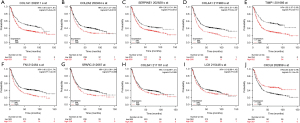
COL4A1 and microRNAs interaction prediction results
Among seven meaningful genes, we found that there were few studies on COL4A1 in gastric cancer. Therefore, we conducted further research on COL4A1. The Target Scan database predicted that microRNA-29a-3p, miR-29b-3p, and miR-29c-3p binded directly to the 3'UTR of COL4A1 mRNA, and were post-transcriptional regulators of COL4A1 (Figure 6A).
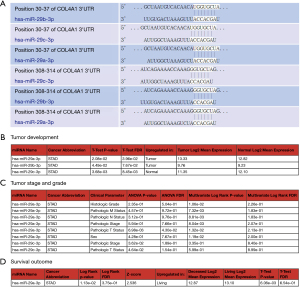
Analyses of expression levels of microRNAs and the relationships with tumor stage, grade, and prognosis in gastric cancer
The OncomiR database search revealed that the expressions of miR-29a-3p and miR-29b-3p in gastric cancer tissues were higher than that in normal tissues (P=2.08e-02, P=4.49e-02); however, the expression of miR-29c-3p in gastric cancer tissues was lower than that in normal tissues (P=3.68e-03) (Figure 6B). The expression of mir-29a-3p was associated with the pathological Tumor (T) status (P=6.98e-03), pathological Lymph Node (N) status (P=3.12e-01), pathological Metastasis (M) status (P=4.57e-01), histological grade (P=2.35e-01), pathological stage (P=5.94e-01), and sex (P=4.28e-01) of gastric cancer patients. The expression of mir-29c-3p was related to the pathological T status (P=4.64e-02) and pathological stage (P=3.62e-02) of gastric cancer patients.
The relationship between the expression of mir-29b-3p and the staging of gastric cancer was not obtained from the database (Figure 6C). Moreover, survival outcomes showed that the expression of mir-29a-3p was higher in living patients than that in deceased patients (P=1.13e-02). However, the survival results of mir-29b-3p and mir-29c-3p were not obtained from the database (Figure 6D).
Discussion
Gastric cancer is a common type of malignant tumor in the clinic. Its high morbidity and mortality have become a serious problem affecting human health. Most patients with gastric cancer are already in advanced stages at first diagnosis, and the diagnosis and treatment effects are poor (15-17). At present, the molecular mechanism of the development and metastasis of gastric cancer is still unclear. Therefore, we further exploration of the mechanisms of the occurrence, development, invasion, metastasis, and recurrence of gastric cancer are needed. There is also a pressing need to identify important auxiliary markers to evaluate the progression and prognosis of gastric cancer, which may provide new information regarding gastric cancer. The goal is to achieve early diagnosis and reduce the mortality of patients with gastric cancer. With the development of microarray technology and high-throughput technology, and the widespread use of these techniques to predict potential therapeutic targets for cancer, we have been able to detect the causes of cancer by examining genome-wide aberrations.
In the present study, the gene expression profiles of the GSE29272, GSE33651, and GSE54129 datasets were used to identify co-expressed DEGs between gastric cancer samples and normal samples. A total of 78 DEGs were obtained, including 53 upregulated DEGs and 25 downregulated DEGs. Functional enrichment analyses were used to investigate interactions among the DEGs, which were mainly enriched in GO BPs such as extracellular matrix organization, extracellular structure organization, response to wounding, collagen fibril organization, collagen biosynthetic process, blood vessel development, vasculature development, wound healing, cell adhesion, and biological adhesion, as well as pathways related to focal adhesion, complement and coagulation cascades, and ECM-receptor interaction (18-21). The most significant module genes in the PPI were significantly enriched in ECM and cell adhesion. Our results also suggested that these GO and pathways may play key roles in the carcinogenesis and progression of gastric cancer. For example, ECM and cell adhesion have been verified to be a substantial part of tumor development and progression (21).
A PPI network was constructed to screen the interactions of DEGs and 10 hub genes were identified, including FN1, COL1A1, COL3A1, TIMP1, COL1A2, SPARC, CXCL8, COL4A1, LOX, and SERPINE1, all of which were upregulated in gastric cancer. The 10 hub genes were further verified using the GEPIA database, and we found that the mRNA expression levels of these 10 hub genes in cancer tissues were significantly higher than in normal tissues. Finally, the survival analysis results showed that overexpression of FN1, COL1A1, TIMP1, COL1A2, SPARC, COL4A1, and SERPINE1 was associated with poor prognosis in gastric cancer patients, whereas the overexpression of COL3A1 and LOX was not meaningful. Interestingly, the overexpression of CXCL8 was a favorable prognostic factor in gastric cancer patients, which provides new ideas for gastric cancer treatment strategy.
H. pylori infections are the main causes of gastric cancer. Studies have found that H. pylori-infected gastric ulcers express higher MMP-7, MMP-9, and TIMP1 than NSAID-related ulcers (22). TIMP1 is expressed strongly in epithelial cells and weakly in inflammatory cells in H. pylori-infected gastric mucosa, but not present in uninfected subjects (23). In addition, the methylation levels of LOX, HAND1, THBD, and p41ARC are still elevated in cases with past H. pylori infection compared to those that were H. pylori negative. And the association of the methylation levels in LOX and HAND1 with GC seems to be more dependent on active H. pylori infection (24). Gastric epithelial cells produce chemokines, especially CXCL8, which is a recurrent feature of H. pylori infection. Compared with H. pylori-negative cases, the expression of CXCL8 mRNA and the production of CXCL8 by antral biopsies is increased in H. pylori-infected patients (25). Moreover,the serum CXCL8 concentration of H. pylori-positive gastric cancer patients is higher than those without carcinoma (26,27). Consequently, in these hub genes, TIMP1, LOX and CXCL8 were clearly related to H. pylori infections.
After reading a large number of literatures on these meaningful genes, we found that FN1, COL1A1, TIMP1, COL1A2, SPARC, and SERPINE1 have been widely reported in the tumorigenesis and progression of gastric cancer. Meanwhile, studies relating to COL4A1 in gastric cancer were relatively scarce. Therefore, in this paper we will focus on COL4A1.
COL4A1 is a vital member of the collagen family and is involved in the formation of the extracellular matrix in most connective and embryonic tissues (28-30). Previous studies have found that intracranial hemorrhage, renal insufficiency, cataracts, and Raynaud’s phenomenon are manifestations of diseases associated with COL4A1, which were multisystemic and could be explained by the vulnerability of small blood vessels (31,32). Among the aforementioned hub genes, COL4A1 is the least studied in tumors, and the correlation between COL4A1 and tumors has been rarely reported. Previous studies have identified the role of COL4A1 in breast cancer, urothelial carcinoma, and oral squamous cell carcinoma. For example, highly-expressed COL4A1 was found to promote the proliferation and migration of ductal cancer cells by activating extracellular matrix receptors in breast tissues. COL4A1 regulates a variety of cellular functions by activating the phosphatidylinositol 3-kinase/protein kinase B (PI3K/AKT) pathway in urothelial carcinoma (33). Also, COL4A1 could be a potential diagnostic biomarker for oral squamous cell carcinoma. Recently, it was reported that COL4A1 could cause recurrence of gastric cancer by activating the PI3K/AKT pathway (33,34), which was similar to the mechanism of urothelial carcinoma. In addition, it has been reported that COL4A1 could be associated with resistance to trastuzumab in gastric cancer (35). These studies could explain why patients with gastric cancer had a poor prognosis after complete surgical resection and the use of molecularly targeted drugs. Therefore, the discovery that COL4A1 could cause recurrence and drug resistance of gastric cancer was of great importance.
Importantly, accumulating evidence suggests that miRNAs play important roles in a variety of biological processes and the dysfunction of miRNAs is related to many diseases including cancer (7). Although dysregulation of miRNAs has been observed in various types of cancers, the molecular mechanisms by which miRNAs regulate the process of carcinogenesis and the behavior of cancer cells are still largely unknown. Currently, altered miRNA expression patterns collected from different study cohorts have been observed in GC. In addition, some deregulated miRNAs (e.g., miR-21, miR-375, miR-124, miR-125b) have been shown to affect cell proliferation, apoptosis, motility and invasion by changing the expression of oncogenes and tumor suppressor factors, thereby promoting gastroesophageal carcinogenesis (15). Our study predicted three microRNAs that could regulate the target gene, COL4A1, including miR-29a-3p, miR-29b-3p, and miR-29c-3p. The OncomiR database was used to analyze of expression levels of microRNAs as well as the relationships with tumor stage, grade, and prognosis in gastric cancer. Due to the limited sample sizes, our research is limited. Verifying the exact expressions and survival results of these three microRNAs in gastric cancer requires numerous experiments. The three family members of miR-29 are miR-29a, miR-29b, and miR-29c, which share similar biological functions and the same region AGCACCA. The miR-29 family is widely involved in tumor cell proliferation (36), apoptosis (37), differentiation (38), and metastasis (39). The study found that miR-29a-3p is expressed in H. pylori-positive gastric mucosa and gastric epithelial cells infected with H. pylori (40). MiR-29a may play the role of a double-edged sword in the development of gastric cancer. In the early stages, it may promote the development of gastric cancer, but inhibit the progression of gastric cancer in the late stages. Maegdefessel et al. found that inhibition of miR-29b decreased the progression of rat abdominal aortic aneurysms (41). Yang et al. reported that miR-29b was an intercellular adhesion regulator that enhanced the migration of oral squamous cell carcinoma cells (42). In addition, miR-29c-3p has been shown to act as a tumor suppressor (43), and was downregulated in numerous tumors, such as esophageal cancer (44), breast cancer (45), nasopharyngeal carcinoma (46), neck cancer (47), hepatocellular carcinoma (48), gallbladder cancer (49), and colon cancer (50). This further suggested that the low expression of miR-29c was related to tumor differentiation, TNM staging, lymph node metastasis, and poor prognosis.
Therefore, further research and experiments on COL4A1 and its corresponding microRNAs are particularly important. Our next research project will construct COL4A1 knockdown and overexpression stable gastric cancer cell lines, verify the function of COL4A1 in gastric cancer, and explore the downstream mechanism pathways involved in the development of gastric cancer. It will also combine with the predicted microRNAs to explore the upstream mechanism pathways, thereby providing a new method for the treatment of gastric cancer.
Conclusions
In this study, we identified central genes related to gastric cancer using a bioinformatics methods, which will provide new ideas for the targeted treatment of gastric cancer. However, the limitation of our research lies in the lack of laboratory evidence. Therefore, further laboratory studies are needed to verify these findings.
Acknowledgments
Funding: This study was supported by the Key Research and Development Projects of Jiangsu Province (grant no: BE2018659).
Footnote
Reporting Checklist: The authors have completed the STREGA reporting checklist. Available at https://dx.doi.org/10.21037/jgo-21-628
Conflicts of Interest: All authors have completed the ICMJE uniform disclosure form (available at https://dx.doi.org/10.21037/jgo-21-628). The authors have no conflicts of interest to declare.
Ethical Statement: The authors are accountable for all aspects of the work in ensuring that questions related to the accuracy or integrity of any part of the work are appropriately investigated and resolved. The study was conducted in accordance with the Declaration of Helsinki (as revised in 2013). Institutional ethical approval and informed consent were waived.
Open Access Statement: This is an Open Access article distributed in accordance with the Creative Commons Attribution-NonCommercial-NoDerivs 4.0 International License (CC BY-NC-ND 4.0), which permits the non-commercial replication and distribution of the article with the strict proviso that no changes or edits are made and the original work is properly cited (including links to both the formal publication through the relevant DOI and the license). See: https://creativecommons.org/licenses/by-nc-nd/4.0/.
References
- Al-Mahrouqi H, Parkin L, Sharples K. Incidence of stomach cancer in oman and the other gulf cooperation council countries. Oman Med J 2011;26:258-62. [Crossref] [PubMed]
- Hatakeyama M. Helicobacter pylori and gastric carcinogenesis. J Gastroenterol 2009;44:239-48. [Crossref] [PubMed]
- Befrits R, Sjöstedt S, Tour R, et al. Long-term effects of eradication of Helicobacter pylori on relapse and histology in gastric ulcer patients: a two-year follow-up study. Scand J Gastroenterol 2004;39:1066-72. [Crossref] [PubMed]
- Chen X, Leung SY, Yuen ST, et al. Variation in gene expression patterns in human gastric cancers. Mol Biol Cell 2003;14:3208-15. [Crossref] [PubMed]
- Zheng TH, Zhao JL, Guleng B. Advances in Molecular Biomarkers for Gastric Cancer. Crit Rev Eukaryot Gene Expr 2015;25:299-305. [Crossref] [PubMed]
- Matsuoka T, Yashiro M. Biomarkers of gastric cancer: Current topics and future perspective. World J Gastroenterol 2018;24:2818-2832. [Crossref] [PubMed]
- Shimonosono M, Arigami T, Yanagita S, et al. The association of human endogenous retrovirus-H long terminal repeat-associating protein 2 (HHLA2) expression with gastric cancer prognosis. Oncotarget 2018;9:22069-78. [Crossref] [PubMed]
- Ueda T, Volinia S, Okumura H, et al. Relation between microRNA expression and progression and prognosis of gastric cancer: A microRNA expression analysis. Lancet Oncol 2010;11:136-146. [Crossref] [PubMed]
- Bartel DP. MicroRNAs: Target recognition and regulatory functions. Cell 2009;136:215-233. [Crossref] [PubMed]
- Lewis BP, Burge CB, Bartel DP. Conserved seed pairing, often flanked by adenosines, indicates that thousands of human genes are microRNA targets. Cell 2005;120:15-20. [Crossref] [PubMed]
- Iorio MV, Ferracin M, Liu CG, et al. MicroRNA gene expression deregulation in human breast cancer. Cancer Res 2005;65:7065-7070. [Crossref] [PubMed]
- Rabinowits G, Gerçel-Taylor C, Day JM, et al. Exosomal microRNA: A diagnostic marker for lung cancer. Clin Lung Cancer 2009;10:42-46. [Crossref] [PubMed]
- Sampson VB, Yoo S, Kumar A, et al. MicroRNAs and potential targets in osteosarcoma Front Pediatr 2015;3:69. Review. [Crossref] [PubMed]
- Callegari E, Gramantieri L, Domenicali M, et al. MicroRNAs in liver cancer: A model for investigating pathogenesis and novel therapeutic approaches. Cell Death Differ 2015;22:46-57. [Crossref] [PubMed]
- Shao CM, Shao QS, Yao HB, et al. Association of SOX9 expression and prognosis in patients with gastric cancer. Zhonghua Wei Chang Wai Ke Za Zhi 2012;15:736-9. [PubMed]
- Siegel RL, Miller KD, Jemal A. Cancer statistics, 2016. CA Cancer J Clin 2016;66:7-30. [Crossref] [PubMed]
- Karimi P, Islami F, Anandasabapathy S, et al. Gastric cancer: descriptive epidemiology, risk factors, screening, and prevention. Cancer Epidemiol Biomarkers Prev 2014;23:700-13. [Crossref] [PubMed]
- Chen Q, Yu D, Zhao Y, et al. Screening and identification of hub genes in pancreatic cancer by integrated bioinformatics analysis. J Cell Biochem 2019;120:19496-508. [Crossref] [PubMed]
- Eke I, Cordes N. Focal adhesion signaling and therapy resistance in cancer. Semin Cancer Biol 2015;31:65-75. [Crossref] [PubMed]
- Malik R, Lelkes PI, Cukierman E. Biomechanical and biochemical remodeling of stromal extracellular matrix in cancer. Trends Biotechnol 2015;33:230-6. [Crossref] [PubMed]
- Okegawa T, Pong RC, Li Y, et al. The role of cell adhesion molecule in cancer progression and its application in cancer therapy. Acta Biochim Pol 2004;51:445-57. [Crossref] [PubMed]
- Cheng HC, Yang HB, Chang WL, et al. Expressions of MMPs and TIMP-1 in gastric ulcers may differentiate H. pylori-infected from NSAID-related ulcers. Scientific World Journal 2012;2012:539316 [Crossref] [PubMed]
- Bodger K, Ahmed S, Pazmany L, et al. Altered gastric corpus expression of tissue inhibitors of metalloproteinases in human and murine Helicobacter infection. Journal of Clinical Pathology 2008;61:72-78. [Crossref] [PubMed]
- Shin CM, Kim N, Jung Y, et al. Role of Helicobacter pylori infection in aberrant DNA methylation along multistep gastric carcinogenesis. Cancer Sci 2010;101:1337-46. [Crossref] [PubMed]
- Ando T, Kusugami K, Ohsuga M, et al. Interleukin-8 activity correlates with histological severity in Helicobacter pylori-associated antral gastritis. Am J Gastroenterol 1996;91:1150-6. [PubMed]
- Konturek SJ, Starzynska T, Konturek PC, et al. Helicobacter pylori and CagA status, serum gastrin, interleukin-8 and gastric acid secretion in gastric cancer. Scand J Gastroenterol 2002;37:891-8. [Crossref] [PubMed]
- Asfaha S, Dubeykovskiy AN, Tomita H, et al. Mice that express human interleukin-8 have increased mobilization of immature myeloid cells, which exacerbates inflammation and accelerates colon carcinogenesis. Gastroenterology 2013;144:155-66. [Crossref] [PubMed]
- Li F, Wang NN, Chang X, et al. Bioinformatics analysis suggests that COL4A1 may play an important role in gastric carcinoma recurrence. J Dig Dis 2019;20:391-400. [Crossref] [PubMed]
- Gould DB, Phalan FC, van Mil SE, et al. Role of COL4A1 in small-vessel disease and hemorrhagic stroke. N Engl J Med 2006;354:1489-96. [Crossref] [PubMed]
- Weng YC, Sonni A, Labelle-Dumais C, et al. COL4A1 mutations in patients with sporadic late-onset intracerebral hemorrhage. Ann Neurol 2012;71:470-7. [Crossref] [PubMed]
- Tonduti D, Pichiecchio A, La Piana R, et al. COL4A1-related disease: raised creatine kinase and cerebral calcification as useful pointers. Neuropediatrics. 2012;43:283-8. [Crossref] [PubMed]
- Meuwissen ME, Halley DJ, Smit LS, et al. The expanding phenotype of COL4A1 and COL4A2 mutations: clinical data on 13 newly identified families and a review of the literature. Genet Med 2015;17:843-53. [Crossref] [PubMed]
- Miyake M, Hori S, Morizawa Y, et al. Collagen type IV alpha 1 (COL4A1) and collagen type XIII alpha 1 (COL13A1) produced in cancer cells promote tumor budding at the invasion front in human urothelial carcinoma of the bladder. Oncotarget 2017;8:36099-114. [Crossref] [PubMed]
- Ryu D, Lee C. Expression quantitative trait loci for PI3K/AKT pathway. Medicine (Baltimore) 2017;96:e5817 [Crossref] [PubMed]
- Huang R, Gu W, Sun B, et al. Identification of COL4A1 as a potential gene conferring trastuzumab resistance in gastric cancer based on bioinformatics analysis. Mol Med Rep 2018;17:6387-96. [Crossref] [PubMed]
- Li Y, Wang F, Xu J, et al. Progressive miRNA expression profiles in cervical carcinogenesis and identification of HPV-related target genes for miR-29. J Pathol 2011;224:484-95. [Crossref] [PubMed]
- Pekarsky Y, Koval A, Hallas C, et al. Tcl1 enhances Akt kinase activity and mediates its nuclear translocation. Proc Natl Acad Sci U S A 2000;97:3028-33. [Crossref] [PubMed]
- Kapinas K, Kessler C, Ricks T, et al. miR-29 modulates Wnt signaling in human osteoblasts through a positive feedback loop. J Biol Chem 2010;285:25221-31. [Crossref] [PubMed]
- Gebeshuber CA, Zatloukal K, Martinez J. miR-29a suppresses tristetraprolin, which is a regulator of epithelial polarity and metastasis. EMBO Rep 2009;10:400-5. [Crossref] [PubMed]
- Sun F, Ni Y, Zhu H, et al. microRNA-29a-3p, Up-Regulated in Human Gastric Cells and Tissues with H.Pylori Infection, Promotes the Migration of GES-1 Cells via A20-Mediated EMT Pathway. Cell Physiol Biochem. 2018;51:1250-1263. [Crossref] [PubMed]
- Maegdefessel L, Azuma J, Toh R, et al. Inhibition of microRNA-29b reduces murine abdominal aortic aneurysm development. J Clin Invest 2012;122:497-506. [Crossref] [PubMed]
- Yang CN, Deng YT, Tang JY, et al. MicroRNA-29b regulates migration in oral squamous cell carcinoma and its clinical significance. Oral Oncol 2015;51:170-7. [Crossref] [PubMed]
- Schmitt MJ, Margue C, Behrmann I, et al. MiRNA-29: a microRNA family with tumor-suppressing and immune-modulating properties. Curr Mol Med 2013;13:572-85. [Crossref] [PubMed]
- Ding DP, Chen ZL, Zhao XH, et al. miR-29c induces cell cycle arrest in esophageal squamous cell carcinoma by modulating cyclin E expression. Carcinogenesis 2011;32:1025-32. [Crossref] [PubMed]
- Bhardwaj A, Singh H, Rajapakshe K, et al. Regulation of miRNA-29c and its downstream pathways in preneoplastic progression of triple-negative breast cancer. Oncotarget 2017;8:19645-60. [Crossref] [PubMed]
- Liu N, Tang LL, Sun Y, et al. MiR-29c suppresses invasion and metastasis by targeting TIAM1 in nasopharyngeal carcinoma. Cancer Lett 2013;329:181-8. [Crossref] [PubMed]
- Hudcova K, Raudenska M, Gumulec J, et al. Expression profiles of miR-29c, miR-200b and miR-375 in tumour and tumour-adjacent tissues of head and neck cancers. Tumour Biol 2016;37:12627-33. [Crossref] [PubMed]
- Wang B, Li D, Sidler C, et al. A suppressive role of ionizing radiation-responsive miR-29c in the development of liver carcinoma via targeting WIP1. Oncotarget 2015;6:9937-50. [Crossref] [PubMed]
- Shu YJ, Bao RF, Jiang L, et al. MicroRNA-29c-5p suppresses gallbladder carcinoma progression by directly targeting CPEB4 and inhibiting the MAPK pathway. Cell Death Differ 2017;24:445-57. [Crossref] [PubMed]
- Chen G, Zhou T, Li Y, et al. p53 target miR-29c-3p suppresses colon cancer cell invasion and migration through inhibition of PHLDB2. Biochem Biophys Res Commun 2017;487:90-5. [Crossref] [PubMed]
(English Language Editor: A. Kassem)