Development and validation of a dual-energy CT-based model to estimate the malignant probability of distal gastric wall thickening
Introduction
Gastric cancer (GC) is the fourth most common malignancy and the third leading cause of cancer mortality worldwide (1). East Asian countries such as Japan, China, and Korea, have a higher incidence of gastric cancer than do Western countries (2), with rates of 24 per 100,000 men and 9.8 per 100,000 women (3). With improved awareness of Helicobacter pylori infections, the mortality rate associated with gastric cancer has reduced markedly in recent years through advanced treatments (4-6). Electronic gastroscopy and computed tomography (CT) play important roles in the diagnosis of gastric cancer. In Asian countries, the use of upper gastrointestinal endoscopy screening for gastric cancer has gradually increased and has evolved into a national screening program in some countries (3,7).
CT is widely used in patients admitted to hospital with various complaints, and the role of CT in the evaluation of abdominal pain is well established (8). Although increased gastric wall thickening on CT imaging is not uncommon, this is not always indicative of gastric cancer. Indeed, gastric wall thickening may be due to various reasons such as cancer, normal peristaltic contraction, gastritis, ulcers, or ischemia and other systemic diseases. Previously, the finding of a gastric wall thickness of 1 cm or greater raised suspicions of malignancy (9); however, it is difficult to ascertain the true pathological origins (10). Recently, dual energy spectral CT (DEsCT), composed of 2 X-ray tubes and 2 corresponding detectors, was introduced. The tube voltages are set at a high- and low-energy, allowing for mixed energy images. These different images, such as monoenergetic images at different energies, iodine maps, and virtual non-contrast images, generate large amounts of data which can be processed. Interestingly, DEsCT has been reported to have great potential in the detection of early gastric cancer and may improve the accuracy of TN staging in gastric cancer patients (11,12).
Although endoscopies and biopsies tend to be more accurate than CT scans for gastric cancer, subsequent endoscopic evaluations after the observation of distal gastric wall thickening on CT imaging will lead to increased costs, complications, patient panic, and unnecessary endoscopy appointments. This in turn, may delay the diagnosis and treatment of patients who seek urgent management (10). In addition, many patients have poor gastroscopy screening compliance. Therefore, the clinical use of a multivariable diagnostic model based on DEsCT may be a promising tool for the early screening of gastric cancer.
This study developed and validated a more practicable model based on DEsCT for the screening of early gastric cancer in patients with serendipitous gastric wall thickening. We present the following article in accordance with the TRIPOD reporting checklist (available at https://jgo.amegroups.com/article/view/10.21037/jgo-21-552/rc).
Methods
The study was conducted in accordance with the Declaration of Helsinki (as revised in 2013). The study was approved by the Independent Research Ethics Boards of the First Affiliated Hospital of Nanjing Medical University (No. 2018-SR-043) and informed consent was waived due to the retrospective nature of the study.
Patients
The abdominal CT reports of patients who had undergone DEsCT scanning in the radiology department for any reason from May 2018 to December 2019 were searched using the keywords “distal gastric wall thickening” in the medical imaging database of the First Affiliated Hospital of Nanjing Medical University. The following inclusion criteria were applied: (I) CT report with distal gastric wall thickening and (II) contrast-enhanced DEsCT examination 1 month before endoscopy or surgery. The following exclusion criteria were applied: (I) patients without endoscopy or surgical pathology (n=77), (II) patients with insufficient stomach distension (n=23), and (III) patients with a history of stomach surgery (n=17). Finally, a total of 208 patients were retrospectively reviewed. Clinical information including age, gender, and pathological data were obtained by reviewing the medical records and by telephone inquiry. The assessment of the gastric adenocarcinoma by endoscopy or surgical pathology was confirmed by 2 independent experienced pathologists. Patients were allocated to the training (n=151) cohort or the testing (n=57) cohort by time according to a 7:3 ratio.
CT image acquisition
After fasting for at least 8 hours, patients were required to drink 800–1,000 mL of tap water, which was followed by an intravenous infusion of scopolamine butylbromide (20 mg). All patients lay in the supine position on the scanning table. CT scans covering the entire abdomen, from the dome of the liver to the pelvic floor, were acquired during a breath-hold. All gastric CT studies were performed using a dual-source multi-detector CT (SOMATOM Force, Siemens Healthineers, Forchheim, Germany). This system consists of 2 X-ray tubes (tube A and tube B) and 2 corresponding 96-section detectors. The dual-energy scanning modes were as follows: tube A was operated at 220 mAs/rot at 100 kV, and tube B was operated at 110 mAs/rot at Sn150 kV. The image acquisition layer had a thickness of 1.5 mm and a helical pitch of 1.15, and the rotation time was 0.5 seconds. In each patient, nonionic contrast material (Visipaque; 320 mgI/mL, GE Healthcare, IDA Business Park, Carrigtwohill, Ireland) was injected via the antecubital vein at a rate of 3.0 mL/sec. In total, 90–120 mL (1.5 mL/kg body weight) was injected using a CT-compatible power injector during the arterial and parenchymal phases. Arterial scanning started automatically 10.0 seconds after the trigger attenuation threshold (100 HU) was reached at the level of the supra celiac portion of the abdominal aorta. Parenchymal scanning commenced 40 seconds after completion of the arterial scanning. Two types of images were derived from the reconstruction of the DEsCT imaging for each patient: water- and iodine-based material decomposition images and a set of virtual monochromatic images at energies ranging from 40 to 140 keV.
Image postprocessing and parameter measurement
For all individuals, the DEsCT data were transferred to a workstation (MMWP, Germany) for further analysis. The dual-energy datasets were postprocessed using clinically available dedicated software. Two abdominal radiologists (QXF and NNS, with 3 and 10 years of gastrointestinal imaging experience, respectively) who were blinded to the endoscopic and pathological results reviewed all CT scans in consensus to evaluate the following traits for each study: (I) tumor site (1, angular incisure; 2, antrum; 3, pylorus; 4, wide range), (II) thickness of the distal gastric wall (maximum thickness in dual phase), (III) diphasic shape change (1, no; 2, yes), and (IV) focal enhancement (1, no; 2, yes). The region of interest (ROI) with the same area of 0.2 mm2 was measured 3 times to obtain the DEsCT parameters in each suspicious malignant thickened gastric wall. The DEsCT parameters included different CT attenuations measured by liver virtual noncontrasted (VNC) software (mixed: 60% from 80 kVp, 40% from 140 kVp), iodine concentration (IC) measured on iodine maps, and fat fraction (FF) on dual phase. The spectral curves on the arterial phase (AP_SC) and the venous phase (VP_SC) were assessed to determine whether they were different based on macroscopic changes compared to the normal gastric wall. To reduce individual differences, the IC was normalized (nIC) against that in the aorta according to the following formula: nIC = IC (in lesion)/IC (in aorta).
Statistical analysis
This was a complete-case analysis. For basic imaging traits, categorical variables were compared by using the χ2 test or Fisher’s exact test. Continuous variables were compared by using the Student’s t-test. Variables with a P value <0.1 in a univariate analysis were candidates for the logistic regression model. Backward step-wise selection was applied by using the likelihood ratio test with Akaike’s information criterion as the stopping rule to build the model with the training cohort. A 10-fold cross-validation was subsequently used to generate the best regression model. Statistical analysis was performed using SPSS, version 22.0 (IBM Corp., Armonk, NY, USA) and R software (version 4.0.0, R Project for Statistical Computing; www.r-project.org). A 2-sided P value <0.05 was considered statistically significant. These variables were then included in the logistic regression model.
The DEsCT model consisting of the significant variables was updated based on the cross-validation model. The discrimination performance of the established models was quantified by the receiver operating characteristic curve (ROC) and area under the curve (AUC) value. The Hosmer-Lemeshow and Akaike information criterion was applied for goodness-of-fit test. Diagnostic efficiency was compared between our DEsCT model and the conventional CT model which only consisted of the diphasic shape change and focal enhancement. A predictive nomogram was formulated based on the results of the logistic regression analysis, and the predicted probabilities (Pi) were obtained.
Results
Clinical and radiological data
Malignant distal gastric wall thickening was observed in 51.0% (106/208) of the patients. The median age of patients with distal gastric wall thickening on CT imaging was 58 years (range, 15–86 years). The results of the basic clinical and conventional CT characteristics and the interobserver reliabilities are summarized in Table 1. Males accounted for the majority (67 patients, 63.2%) of malignant conditions. There were significant differences in the thickening site, thickness, DP shape change, and focal enhancement between benign and malignant patients (P<0.001). Table 2 lists the DEsCT characteristics and the interobserver reliabilities of benign and malignant patients. Univariate analysis showed differences in 10 DEsCT characteristics between benign and malignant distal gastric wall thickening (AP_CM, AP_mixed, AP_IC, AP_nIC, VP_CM, VP_mixed, VP_IC, VP_nIC, AP_SC, and VP_SC; P<0.05). The interreader agreement was moderate for focal enhancement, VP_IC, and VP_nIC with a coefficient of less than 0.600. The other radiology features were good, with the coefficient ranging from 0.608 to 0.935.
Table 1
Characteristics | Benign group (n=102) | Malignant group (n=106) | Agreement† | P | |||
---|---|---|---|---|---|---|---|
Rad 1 | Rad 2 | Rad 1 | Rad 2 | ||||
Median age (years ± SD) | 57.4 (13.3) | 58.3 (12.0) | 0.624 | ||||
Gender | 0.002 | ||||||
Female | 60 (58.8) | 39 (36.8) | |||||
Male | 42 (41.2) | 67 (63.2) | |||||
CT characteristics | |||||||
Site | 0.902 | <0.001 | |||||
Angular incisure | 10 (9.8) | 10 (9.8) | 30 (28.3) | 31 (29.2) | |||
Antrum | 74 (72.5) | 76 (74.5) | 59 (55.7) | 58 (54.7) | |||
Pylorus | 14 (13.7) | 14 (13.7) | 2 (1.9) | 4 (3.8) | |||
Wide range | 4 (3.9) | 2 (2.0) | 15 (14.2) | 13 (12.3) | <0.001 | ||
Thickness (mm ± SD) | 12.8 (2.9) | 12.8 (3.6) | 15.4 (4.9) | 14.1 (4.1) | 0.608 | <0.001 | |
DP_shape change | 47 (46.1) | 32 (31.4) | 87 (82.1) | 88 (83.0) | 0.658 | <0.001 | |
Focal enhancement | 30 (29.4) | 7 (6.9) | 80 (75.5) | 96 (90.6) | 0.548 | <0.001 |
Values are number of findings and values in parentheses are percentages unless indicated otherwise. †, reports by Kappa or ICC test. Rad, radiologist; DP_shape change, diphasic shape change.
Table 2
DEsCT characteristics | Benign group (n=102) | Malignant group (n=106) | Agreement† | P | |||
---|---|---|---|---|---|---|---|
Rad 1 | Rad 2 | Rad 1 | Rad 2 | ||||
AP_VNC (HU) | 22.0 (13.3) | 22.5 (15.3) | 20.0 (17.2) | 20.4 (17.4) | 0.920 | 0.354 | |
AP_CM (HU) | 34.2 (15.3) | 34.1 (16.4) | 43.2 (14.7) | 42.8 (15.2) | 0.926 | <0.001 | |
AP_Mixed (HU) | 54.9 (11.2) | 55.1 (12.9) | 62.1 (17.8) | 61.2 (20.0) | 0.919 | 0.010 | |
AP_IC (mg/mL) | 1.8 (1.0) | 1.7 (1.1) | 2.2 (0.8) | 2.1 (1.2) | 0.654 | 0.001 | |
AP_FF (%) | 20.4 (14.5) | 20.2 (15.6) | 21.0 (14.1) | 19.6 (13.1) | 0.836 | 0.749 | |
AP_nIC (%) | 17.8 (11.4) | 18.4 (13.6) | 22.1 (8.9) | 21.5 (11.6) | 0.721 | 0.003 | |
VP_VNC (HU) | 24.2 (12.0) | 23.7 (14.4) | 21.9 (17.6) | 21.4 (18.5) | 0.903 | 0.256 | |
VP_CM (HU) | 44.7 (14.8) | 45.0 (14.4) | 58.1 (17.5) | 56.9 (17.4) | 0.935 | <0.001 | |
VP_Mixed (HU) | 67.6 (11.7) | 66.9 (13.1) | 79.3 (27.7) | 76.4 (24.4) | 0.853 | 0.001 | |
VP_IC (mg/mL) | 2.5 (2.1) | 2.3 (1.1) | 3.2 (2.2) | 2.9 (1.1) | 0.456 | 0.019 | |
VP_FF (%) | 19.2 (14.4) | 20.2 (14.9) | 21.5 (16.4) | 21.5 (16.8) | 0.895 | 0.280 | |
VP_nIC (%) | 52.2 (39.1) | 50.7 (24.5) | 70.5 (63.7) | 62.2 (26.1) | 0.452 | 0.014 | |
AP_SC | 12 (11.8) | 29 (28.4) | 49 (46.2) | 70 (66.0) | 0.627 | <0.001 | |
VP_SC | 9 (8.8) | 19 (18.6) | 64 (60.4) | 85 (80.2) | 0.720 | <0.001 |
Values are average value of findings and values in parentheses are standard deviation, except for AP_SC and VP_SC, where values are number of findings and values in parentheses are percentages. †, reports by Kappa or ICC test. DEsCT, dual energy spectral CT. Rad, radiologist; AP, arterial phase; VP, venous phase; VNC, virtual non-contrasted; CM, net contrast material; IC, iodine concentration; nIC, normalized iodine concentration; FF, fat fraction; SC, spectral curve.
Predictive performance of the malignant distal gastric wall thickening risk nomogram
Logistic regression analysis was used to develop the diagnostic model for malignant distal gastric wall thickening using 151 patients in the training set. The probability model was established as follows: , where βi is the coefficient of each variable xi in the regression model. In the backward stepwise regression analysis, VP_SC [odds ratio (OR): 8.419], focal enhancement (OR 3.741), arterial phase-mixed (OR 1.030), tumor site (OR 0.573), and DP_shape change (OR 2.746) were identified as the 5 candidates for predicting the malignancy of distal gastric wall thickening. These 5 indicators were selected for further cross-validation to adjust the model parameters to obtain the best DEsCT model with only 2 “worrisome” features: focal enhancement (OR 8.291) and VP_SC (OR 5.211). Figure 1 shows the ROC analysis of the DEsCT model and the CT model for predicting the malignancy of distal gastric wall thickening. The AUC of the DEsCT model was 0.803 (95% CI: 0.731 to 0.875) for the training cohort and 0.905 (95% CI: 0.829 to 0.982) for the testing cohort. The AUC of the CT model was 0.776 (95% CI: 0.698 to 0.854) for the training cohort and 0.852 (95% CI: 0.750 to 0.955) for the testing cohort. Figure 2 shows the calibration analysis of the DEsCT model for predicting malignant distal gastric wall thickening. The Hosmer-Lemeshow test yielded a P value of 0.258 for the training cohort of the DEsCT model, suggesting good concordance between the predicted probabilities and the actual class probabilities. A P value of 0.615 was found for the testing cohort of the CT model, suggesting no departure from good fit.
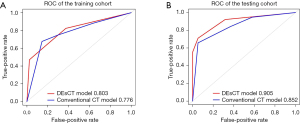
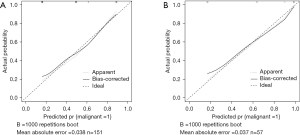
Table 3 summarizes the details of the DEsCT model and the CT model for diagnostic performance. The DEsCT model was superior to the conventional CT model in terms of AUC. For easier clinical use in gastric wall malignancy diagnosis with DEsCT. A nomogram consisting of the 5 indicators of malignant distal gastric wall thickening, including the updated 2 “worrisome” features, was built (Figure 3). Figure 4 presents a clinical case in which the nomogram was used to estimate the probability of distal gastric wall thickening in a patient with malignancy risk.
Table 3
Cohort | SEN, % | SPE, % | PPV, % | NPV, % | ACC | AUC | AUC 95% CI |
---|---|---|---|---|---|---|---|
Training | |||||||
DEsCT model | 69.2 | 94.1 | 97.6 | 47.1 | 0.748 | 0.803 | 0.731–0.875 |
CT model | 76.3 | 79.3 | 85.5 | 67.7 | 0.775 | 0.776 | 0.698–0.854 |
Testing | |||||||
DEsCT model | 81.3 | 85.4 | 68.4 | 92.1 | 0.842 | 0.905 | 0.829–0.982 |
CT model | 80.0 | 76.6 | 42.1 | 94.7 | 0.772 | 0.852 | 0.750–0.955 |
SEN, sensitivity; SPE, specificity; PPV, positive predictive value; NPV, negative predictive value; ACC, accuracy; DEsCT, dual energy spectral CT.
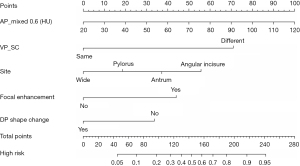
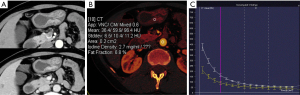
Discussion
In this study, we developed and validated a DEsCT-based model for the noninvasive, individualized prediction of malignancy risk in patients with distal gastric wall thickening on abdominal CT screening. An easy-to-use nomogram was built to assist radiologists and clinicians assess the biological malignancy risk of distal gastric wall thickening in a noninvasive manner. The multivariate logistic regression analysis revealed that 1 conventional CT feature (focal enhancement) and 1 DEsCT feature (VP_SC) were effective variables in distinguishing between benign and malignant biological behaviors. The DEsCT model, composed of the 2 CT features, produced a higher AUC than did the conventional CT scheme composed of only DP_shape change and focal enhancement.
In this study cohort, gastric cancer was 1.8–2.2 times more prevalent in males than females, and this was consistent with our previous study on the distal stomach (13). Gastric cancer is often in an advanced stage at diagnosis and has poor prognosis and high mortality (14). The distal gastric region has the highest risk of malignancy, and most missed gastric cancers are poorly differentiated lesions located in the gastric antrum or lower body (15). Increased thickness in the distal gastric wall is commonly observed in CT scans due to poor image quality, insufficient stomach distention, and excessive peristaltic movements resulting in antral contractions (16). In addition, anatomic studies have shown that gastric smooth muscle, particularly the circular layer, is thicker and denser around the gastric antrum than around the rest of the stomach (16). Increased gastric wall thickness in CT scans was previously considered to indicate pathological gastric cancer, with the malignant thickness threshold ranging from 9.36 to 19 mm (17,18). However, there are several limitations to endoscopies, including the possibility of self-selection bias, high costs, invasiveness, high complication risks, and length of time needed. These limitations of endoscopic examination are unavoidable as the occasional discovery of gastric wall thickening on CT scans followed by further endoscopy. Moreover, some patients with potential gastric cancer are not suited for endoscopy due to severe underlying diseases (19). Therefore, a more accurate evaluation of the distal gastric wall thickness via CT is needed for the early diagnosis and the prevention of unnecessary examinations.
The DEsCT model we built showed improvement in sensitivity and specificity for the diagnosis of malignant distal gastric wall thickness compared to the conventional CT model. Here, sensitivity refers to the model’s ability to designate an individual with gastric cancer. Sensitivity is of important significance for early diagnosis and treatment of the patients with gastric cancer because gastric cancer is always detected in advanced stage. Inversely, specificity is the percentage of true negatives out of all patients with distal gastric wall thickening who do not have gastric cancer. Improved specificity can prevent unnecessary endoscopy in the patients without gastric cancer. During conventional enhancement CT scans, an experienced abdominal radiologist can differentiate malignant distal gastric wall thickening by examining dynamic shape change and focal enhancement. The normal gastric contractions originate spontaneously at a certain frequency (20). However, the gastric wall becomes stiff and the peristaltic waves will disappear after the stomach gets cancerization. Therefore, dynamic shape changes may be a strong indicator of benign gastric wall thickening. The focal enhancement of the gastric wall indicates that the focal area of iodine concentration may be undergoing vascular physiological changes often associated with cancer (21). Recent studies on DEsCT have revealed that the IC on iodine maps, the slope of the spectral curve may be good parameters for evaluating the expression of Ki-67 antigen and providing more accurate TN staging in gastric cancer (11,22,23). In accordance with the literature, the spectral curve in the venous phase is different between benign and malignant patients. Malignant distal gastric wall thickening tends to have a higher arterial phase CT value (62.1±17.8 HU) compared to benign wall thickening (54.9±11.2 HU), suggesting that IC may be indicative of the angiogenic physiology associated with gastric cancer (24,25). Spectral Hounsfield unit curves, obtained by plotting the CT attenuation values of a material for every monochromatic energy from 40 to 140 keV, can help characterize specific tissue types. Indeed, the curves represent the mean attenuation characteristics of the materials (26-28), and may thus explain the different slopes of the spectral curves between the benign and malignant distal gastric wall samples in our study.
In this study, we combined conventional CT features, which rely upon the experience of the radiologist, with the quantitative characteristics of DEsCT to build an easy-to-use nomogram to predict the malignancy risk in patients with distal gastric wall thickening. To estimate the probability of distal gastric wall thickening with malignancy risk for a specific patient via nomogram, we reviewed the 5 CT features, calculated the sum scores, and the found the vertical line which intersected with the predicted probability of malignancy risk.
There were some limitations to this study. First, the study included only patients with suspected distal gastric wall thickness on CT scans. Patients with normal gastric wall thickness, those with other gastric wall thickness in other regions, and those who were strongly suspected of gastric tumor without obvious gastric wall thickening were excluded. Second, the training sensitivity of DEsCT model is 7% lower than conventional CT model, but 1% higher in the testing data. As the testing set is independent and the data distribution is consistent with the training set, the diagnostic efficiency is possible to increase. But more numbers of patients are need to improve the outcomes in the following study.
Conclusions
The nomogram, based on DP_shape change, focal enhancement, AP_nIC, and VP_SC features, may provide an alternative to conventional radiological methods for the assessment and management of patients with distal gastric wall thickening on DEsCT scans.
Acknowledgments
Funding: This study was supported by a Key Social Development Program for the Ministry of Science and Technology of Jiangsu Province (BE2017772, XSL).
Footnote
Reporting Checklist: The authors have completed the TRIPOD reporting checklist. Available at https://jgo.amegroups.com/article/view/10.21037/jgo-21-552/rc
Data Sharing Statement: Available at https://jgo.amegroups.com/article/view/10.21037/jgo-21-552/dss
Conflicts of Interest: All authors have completed the ICMJE uniform disclosure form (available at https://jgo.amegroups.com/article/view/10.21037/jgo-21-552/coif). The authors have no conflicts of interest to declare.
Ethical Statement: The authors are accountable for all aspects of the work in ensuring that questions related to the accuracy or integrity of any part of the work are appropriately investigated and resolved. The study was conducted in accordance with the Declaration of Helsinki (as revised in 2013). The study was approved by the Independent Research Ethics Boards of the First Affiliated Hospital of Nanjing Medical University (No. 2018-SR-043) and informed consent was waived due to the retrospective nature of the study.
Open Access Statement: This is an Open Access article distributed in accordance with the Creative Commons Attribution-NonCommercial-NoDerivs 4.0 International License (CC BY-NC-ND 4.0), which permits the non-commercial replication and distribution of the article with the strict proviso that no changes or edits are made and the original work is properly cited (including links to both the formal publication through the relevant DOI and the license). See: https://creativecommons.org/licenses/by-nc-nd/4.0/.
References
- Ferlay J, Soerjomataram I, Dikshit R, et al. Cancer incidence and mortality worldwide: sources, methods and major patterns in GLOBOCAN 2012. Int J Cancer 2015;136:E359-86. [Crossref] [PubMed]
- Siegel R, Ward E, Brawley O, et al. Cancer statistics, 2011: the impact of eliminating socioeconomic and racial disparities on premature cancer deaths. CA Cancer J Clin 2011;61:212-36. [Crossref] [PubMed]
- Hamashima C, Shabana M, Okada K, et al. Mortality reduction from gastric cancer by endoscopic and radiographic screening. Cancer Sci 2015;106:1744-9. [Crossref] [PubMed]
- Amiri M, Janssen F, Kunst AE. The decline in stomach cancer mortality: exploration of future trends in seven European countries. Eur J Epidemiol 2011;26:23-8. [Crossref] [PubMed]
- Honda M, Hiki N, Kinoshita T, et al. Long-term Outcomes of Laparoscopic Versus Open Surgery for Clinical Stage I Gastric Cancer: The LOC-1 Study. Ann Surg 2016;264:214-22. [Crossref] [PubMed]
- Kim N. Chemoprevention of gastric cancer by Helicobacter pylori eradication and its underlying mechanism. J Gastroenterol Hepatol 2019;34:1287-95. [PubMed]
- Leung WK, Wu MS, Kakugawa Y, et al. Screening for gastric cancer in Asia: current evidence and practice. Lancet Oncol 2008;9:279-87. [Crossref] [PubMed]
- Aisenberg GM, Grimes RM. Computed tomography in patients with abdominal pain and diarrhoea: does the benefit outweigh the drawbacks? Intern Med J 2013;43:1141-4. [Crossref] [PubMed]
- Insko EK, Levine MS, Birnbaum BA, et al. Benign and malignant lesions of the stomach: evaluation of CT criteria for differentiation. Radiology 2003;228:166-71. [Crossref] [PubMed]
- Akbas A, Bakir H, Dasiran MF, et al. Significance of Gastric Wall Thickening Detected in Abdominal CT Scan to Predict Gastric Malignancy. J Oncol 2019;2019:8581547. [Crossref] [PubMed]
- Pan Z, Pang L, Ding B, et al. Gastric cancer staging with dual energy spectral CT imaging. PLoS One 2013;8:e53651. [Crossref] [PubMed]
- Shi C, Zhang H, Yan J, et al. Decreased stage migration rate of early gastric cancer with a new reconstruction algorithm using dual-energy CT images: a preliminary study. Eur Radiol 2017;27:671-80. [Crossref] [PubMed]
- Sitarz R, Skierucha M, Mielko J, et al. Gastric cancer: epidemiology, prevention, classification, and treatment. Cancer Manag Res 2018;10:239-48. [Crossref] [PubMed]
- Digklia A, Wagner AD. Advanced gastric cancer: Current treatment landscape and future perspectives. World J Gastroenterol 2016;22:2403-14. [Crossref] [PubMed]
- Kim SJ, Choi CW. Common Locations of Gastric Cancer: Review of Research from the Endoscopic Submucosal Dissection Era. J Korean Med Sci 2019;34:e231. [Crossref] [PubMed]
- Pickhardt PJ, Asher DB. Wall thickening of the gastric antrum as a normal finding: multidetector CT with cadaveric comparison. AJR Am J Roentgenol 2003;181:973-9. [Crossref] [PubMed]
- Cho SG, Kim WH, Lee KH, et al. Wall Thickening of The Gastric Antrum: Is It a Pseudolesion or a Tumor? J Korean Radiol Soc 1999;40:281-7. [Crossref]
- Tongdee R, Kongkaw L, Tongdee T. A study of wall thickness of gastric antrum: comparison among normal, benign and malignant gastric conditions on MDCT scan. J Med Assoc Thai 2012;95:1441-8. [PubMed]
- Vargo JJ 2nd. Sedation-related complications in gastrointestinal endoscopy. Gastrointest Endosc Clin N Am 2015;25:147-58. [Crossref] [PubMed]
- Forrest AS, Hennig GW, Jokela-Willis S, et al. Prostaglandin regulation of gastric slow waves and peristalsis. Am J Physiol Gastrointest Liver Physiol 2009;296:G1180-90. [Crossref] [PubMed]
- Ilson DH. Angiogenesis in gastric cancer: hitting the target? Lancet 2014;383:4-6. [Crossref] [PubMed]
- Cheng SM, Ling W, Zhu J, et al. Dual Energy Spectral CT Imaging in the assessment of Gastric Cancer and cell proliferation: A Preliminary Study. Sci Rep 2018;8:17619. [Crossref] [PubMed]
- Xie ZY, Chai RM, Ding GC, et al. T and N Staging of Gastric Cancer Using Dual-Source Computed Tomography. Gastroenterol Res Pract 2018;2018:5015202. [Crossref] [PubMed]
- Park DJ, Thomas NJ, Yoon C, et al. Vascular endothelial growth factor a inhibition in gastric cancer. Gastric Cancer 2015;18:33-42. [Crossref] [PubMed]
- Chen XH, Ren K, Liang P, et al. Spectral computed tomography in advanced gastric cancer: Can iodine concentration non-invasively assess angiogenesis? World J Gastroenterol 2017;23:1666-75. [Crossref] [PubMed]
- Machida H, Tanaka I, Fukui R, et al. Dual-Energy Spectral CT: Various Clinical Vascular Applications. Radiographics 2016;36:1215-32. [Crossref] [PubMed]
- Silva AC, Morse BG, Hara AK, et al. Dual-energy (spectral) CT: applications in abdominal imaging. Radiographics 2011;31:1031-46; discussion 1047-50. [Crossref] [PubMed]
- Zhang X, Zheng C, Yang Z, et al. Axillary Sentinel Lymph Nodes in Breast Cancer: Quantitative Evaluation at Dual-Energy CT. Radiology 2018;289:337-46. [Crossref] [PubMed]