Weighted gene co-expression network analysis reveals prognostic and diagnostic significance of PAQR4 in patients with early and late hepatocellular carcinoma
Introduction
Hepatocellular carcinoma (HCC) is a solid-organ malignancy affecting the global population, with an increasing mortality and morbidity (1,2). It commonly develops from a pre-existent liver disease and is associated with specific risk factors, including chronic viral types B and C hepatitis, alcohol abuse, and aflatoxin exposure (3,4). Due to the occult onset of HCC and asymptomatic presentation in the early disease stage, only 10–20% of HCC tumors are suitable for surgical removal (5). Due to its high incidence, limited therapeutic strategies, and poor prognosis, it remains a challenge in clinical practice. Thus, it is earnestly required to identify more sensitive biomarkers and clarify the molecular mechanisms of HCC (6-9).
With the emergence of microarray technology and transcriptome analysis, bioinformatics have become the most frequently used means to identify potential biomarkers in a variety of diseases (10-12). Weighted gene co-expression network analysis (WGCNA) is a prevailing biological tool used not only to build gene networks, but also to analyze gene modules and obtain the core gene within modules (13,14). It can be used to obtain potential biomarker genes according to the correlations between gene sets and phenotypes. In recent years, WGCNA has been comprehensively used to investigate multiple diseases (15,16). For example, Liu et al. reported using WGCNA to identify 11 genes related to the progression and prognosis of endometrial cancer (17). Liu et al. have found that CNFN may be used as a potential biomarker for the prediction of lymph node metastases and prognosis in head and neck cancer (15). Liang et al. revealed that WGCNA could be applied to identify and predict the key genes in Alzheimer’s disease (16). Yang et al. used WGCNA to identify CD36 as a novel biomarker or therapeutic target in lupus nephritis (12).
This study aimed to determine novel markers to predict the prognosis and diagnosis of HCC by using multiple databases and various bioinformatics analysis tools. Here, we identified differentially expressed genes (DEGs) between HCC and normal tissue based on data from The Cancer Genome Atlas (TCGA), and constructed a WGCNA co-expression network. After overlapping these 803 DEGs with 837 genes in the blue module, we obtained 466 DEGs that were defined as hub genes. Function annotation of 466 hub genes was also performed. After verification using the GSE36376 dataset, we found the real hub gene, expression of progestin and adipoQ receptor 4 (PAQR4) that could truly predict the prognosis of HCC.
Methods
Data collection
In this study, RNA sequencing data (count value) of gene expression and corresponding clinical data of HCC were obtained from TCGA database, which was downloaded from the University of California Santa Cruz Xena (UCSC Xena; http://xena.ucsc.edu/) database. According to the stage in clinical information, HCC patients were divided into early stage (stage I and stage II) and late stage (stage III and stage IV). Some 260 early HCC tissues, 85 late HCC tissues, and 50 normal adjacent samples from HCC patients were enrolled. We also downloaded the GSE36376 dataset from the Gene Expression Omnibus (GEO) database (https://www.ncbi.nlm.nih.gov/geo/), including of 240 HCC samples and 193 normal tissues with expression profiles and clinical information, to further verify stage-related genes.
DEGs
We used the DESeq2 package in R (https://www.r-project.org/) to screen the DEGs between HCC and normal adjacent samples. A false discovery rate (FDR) of <0.01 and |log2FC| >2 were selected as the cut-off criteria. The R package v3.5.3 (https://cran.r-project.org/bin/windows/base/old/3.5.3/) was utilized to obtain hierarchical clustering analysis of DEGs.
WGCNA construction and module detection
The genes were ranked, and the top 25% were subjected to the subsequent analysis. The WGCNA package in R as a powerful tool was used in gene co-expression network identification. First, Pearson’s correlation coefficient was calculated for all gene pairs to obtain a co-expression similarity matrix. According to the scale-free topology criterion, the adjacency matrix was obtained by raising the soft thresholding power to β=5. Then, the adjacency matrix was transformed into topological overlap matrix, and then in turn converted into a dissimilarity topological overlap matrix, from which a dendrogram was mapped via hierarchical clustering. The cluster tree was dynamically cut to obtain from the cluster. The resulting clusters were co-expression modules containing genes that were considerably interconnected. The modules with |r|>0.5 & P<0.001 were identified as HCC-associated modules.
Functional annotation
The functional annotation, including Gene Ontology (GO) classification and Kyoto Encyclopedia of Genes and Genomes (KEGG) pathway enrichment analysis, were performed with GeneCoDis3 (http://genecodis.genyo.es/). The GO and KEGG enrichment analyses were visualized in ggplot2 package in R. Significant enrichment was defined by P<0.05.
Identification of tumor stage related genes
According to the clinical data, the HCC group was further divided into an early group (stage I and II) and late group (stage III). The expression difference of stage related genes in different clinical tumor stages (early stage vs. late stage) was performed. The multiple comparisons between different clinical tumor stages were performed with Tukey’s honest significant difference test. Statistical significance was considered when P<0.05.
Validation of real hub genes in the published GSE36376 dataset
The expression of tumor stage related genes was validated with the GSE36376 dataset. We downloaded the gene expression matrix file of GSE36376 from the GEO database and annotated the probe according to the annotation file of the GPL10558 platform. The dataset GSE36376 was used to examine the tissue samples consisting of 240 HCC patients and 193 normal controls. According to the clinical data, HCC samples were further divided into early stage (stage I and II) and late stage (stage III). The DEGs were identified using the Wilcoxon test and statistically significant DEGs were defined with the criteria of P.05.
UALCAN analysis
The University of Alabama Cancer database (UALCAN; http://ualcan.path.uab.edu/) is a premier public resource for further exploring TCGA gene expression data. We analyzed PAQR4 expression on the basis of multiple clinic pathological features in HCC samples from TCGA. The expression and prognosis data for PAQR4 were obtained using UALCAN, and Kaplan-Meier curves were produced using online tools.
Receiver operating characteristic (ROC) curve analyses
ROC analysis is a commonly used binary assessment method that evaluates the diagnostic value of the transcriptional expression of any gene of interest in differentiating disease from healthy samples. ROC analysis was performed with the “pROC” package in R to determine the optimal PAQR4 cut-off point and to evaluate the diagnostic value of PAQR4 expression by calculation of the area under the curve (AUC). When the AUC was greater than 0.8, it was considered to have diagnostic value.
Confirmation by quantitative reverse transcription polymerase chain reaction
A total of 12 tissues samples of early HCC patients (n=3) and normal adjacent (n=3) and late HCC patients (n=3) and normal adjacent (n=3) were obtained. This study complied with the principles of the Declaration of Helsinki (as revised in 2013). This study was approved by the ethics committee of Sichuan Provincial People’s Hospital, University of Electronic Science and Technology of China (No. 2020161). All participants provided their written informed consent.
Total RNA was isolated with an RNA simple total RNA kit (Tiangen, Beijing, China). We reverse-transcribed RNA (2 µg) using a Fast Quant RT Kit (Tiangen, China). Quantitative reverse transcription polymerase chain reaction (qRT-PCR) was conducted using the Super Real PreMix Plus SYBR Green (Tiangen, China) on an ABI 7500 real-time PCR system (Thermo Fisher Scientific, Waltham, MA, USA). The 2-∆∆Ct method was used to analyze the expression level of PAQR4. Human GAPDH and ACTB were used as endogenous controls.
Validating the expression of PAQR4 in the published GSE76427 dataset
The expression of PAQR4 was validated with the GSE76427 dataset, which was downloaded from the GEO. The dataset GSE76427 was used to examine the tissue samples consisting of 90 early HCC patients, 24 late HCC patients, and 50 normal controls. Gene set enrichment analysis (GSEA; http://www.broadinstitute.org/gsea/index.jsp) was carried out to uncover the key pathway in the PAQR4 high expression group and PAQR4 low expression group. The annotated gene sets of version 6.0 were downloaded from the Molecular Signatures Database (MSigDB; http://software.broadinstitute.org/gsea/msigdb/index.jsp). The inclusion criteria were normalized P<0.05 and FDR <25%.
Statistical analysis
Most of the statistical analysis has been done by the bioinformatic tools mentioned above. In addition, the GraphPad Prism Software, version 7.0 was used for the statistical analysis of experimental data. The results are expressed as means ± standard deviations (SDs). Spearman correlation coefficients were calculated to evaluate the correlations. The multiple comparisons between different clinical tumor stages were performed with Tukey’s honest significant difference test. P<0.05 was considered to indicate a significant difference.
Results
Identification of DEGs
Under the threshold of FDR <0.01 and |log2FC| >2, 803 DEGs were obtained between HCC and normal adjacent samples, of which 175 were down-regulated and 627 were up-regulated. A heat map of the top 100 DEGs is displayed in Figure 1. All genes were ranked and the top 25% were selected for subsequent WGCNA.
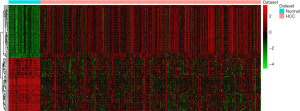
WGCNA construction
A co-expression network analysis was performed to develop hub modules and genes using the WGCNA R package. The power β=5 was screened to ensure a scale-free network (Figure 2A). Different color distribution was used to represent the co-expression module, and we identified 7 modules (Figure 2B), including a brown module, red module, turquoise module, yellow module, green module, black module, and blue module. A heat map of the top 400 genes is demonstrated in Figure 2C. To understand the connections and interactions among these 7 co-expressed modules, we also analyzed the connectivity of eigengenes. An eigengene adjacency heatmap was generated (Figure 3A). Compared with the other modules, the red module and blue module had higher correlations with HCC. The module trait relationship is displayed in Figure 3B, and the results uncovered that the red module was negatively with associated with cancer traits (P=4E-58 and |r|=0.69) and the blue module was positively with associated with cancer traits (P=2E-36 and |r|=0.58), suggesting that the genes in the blue module might play an important role in the occurrence and development of HCC, and the genes in the red module might inhibit the occurrence and development of HCC. Thus, the blue module was selected for further analysis. We performed an intramodular analysis for the blue module, and the module membership and gene significance showed a very meaningful correlation (cor =0.68 and P value =6.4E-115), indicating that 837 genes in the blue module tended to be remarkably correlated with HCC (Figure 3C). The blue module was suitable for identifying the hub genes associated with HCC.
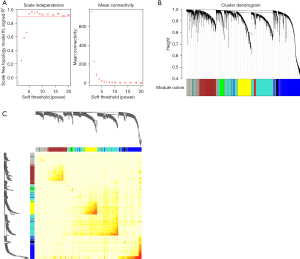
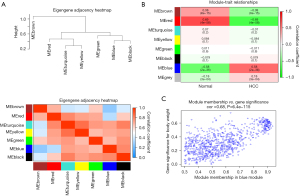
Hub genes identification and function annotation
After overlapping these all DEGs with 837 genes of blue module, we obtained 466 DEGs that were defined as hub genes (Figure S1). The GO analysis of the 466 hub genes is exhibited in Figure 4A. All hub genes were shown to be related to mitotic cell cycle (P=3.26E-43), cell division (P=1.62E-39), protein binding (P=6.25E-17), and ATP binding (P=3.98E-15). The KEGG pathway enrichment analysis uncovered that cell cycle (P=5.80E-26) and the p53 signaling pathway (P=1.04E-08) were significantly enriched pathways (Figure 4B).
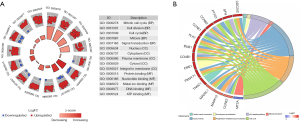
Identification and verification of genes associated with early- and late-stage HCC
According to the clinical data, HCC samples were further divided into early stage (stage I and II) and late stage (stage III), and the correlation between the intersecting genes and staging was analyzed. We found that 239 of the 466 hub genes were related to staging. Next, the expression of 239 hub genes was verified in using the GSE36376 dataset. Only the expression of PAQR4 was consistent with our TCGA integration results (Figure 5A,5B).
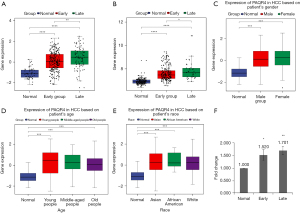
UALCAN analysis
We evaluated PAQR4 expression on the basis of multiple clinic pathological features in HCC samples using UALCAN. The expression level of PAQR4 was significantly elevated in HCC patients compared to the normal controls in subgroup analyses based on gender, age, and race (Figure 5C-5E). The 12 tissues samples of early HCC patients (n=3) and normal adjacent (n=3) and late HCC patients (n=3) and normal adjacent (n=3) were used to perform the qRT-PCR confirmation experiment. As displayed in Figure 5F, PAQR4 was more up-regulated in early and late HCC patients than normal control in our qRT-PCR results, which was consistent with our integration results, suggesting that the results were convincing. The prognostic value of PAQR4 was analyzed by UALCAN. It was revealed that PAQR4 was associated with the prognosis of patients with HCC. The low expression of PAQR4 had a better prognosis than high expression (Figure 6A). We also assessed the diagnostic value of PAQR4 in HCC. Therefore, PAQR4 (AUC =0.869) was capable of discriminating HCC and normal controls (Figure 6B).
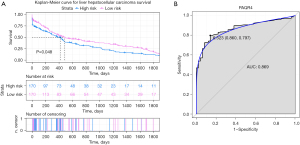
Validation in a published the GEO dataset (GSE76427)
The expression pattern and KEGG enrichment of PAQR4 was verified using the GSE76427 dataset. The results indicated that PAQR4 was up-regulated in both early and late HCC patients than in normal controls, which was consistent with our integration and qRT-PCR results (Figure 7A). In addition, high expression of PAQR4 was significantly associated with cell cycle, DNA replication, and the p53 signaling pathway (Figure 7B-7D). This suggests that PAQR4 may be involved in the progression of HCC by regulating these functions.
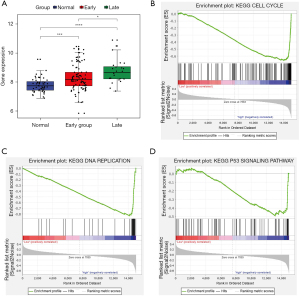
Discussion
As an aggressive malignancy, HCC is a leading cause of cancer-related death in the world, due to a lack of effective treatment strategies (3). Early diagnosis and effective treatment of HCC are critically important. Therefore, it is an urgent need to seek effective biomarkers and therapeutic targets that are significant in guiding the therapy of HCC. In the current study, we examined the gene expression profile of TCGA to screen the DEGs. Here, we obtained 803 DEGs between HCC and normal adjacent samples using FDR <0.01 and |log2FC| >2 as the cutoff criteria. We applied WGCNA to divide the genes into 7 co-expression modules. After relating the modules to cancer traits, the blue module was suitable for excavating the hub genes associated with the HCC. We screened genes related to staging from all hub genes. After verification using the GSE36376 dataset, PAQR4 was identified as the real hub gene behind the pathogenesis of HCC. Furthermore, the expression of PAQR4 was validated by qRT-PCR. Finally, we performed ROC curve analysis and survival analysis to evaluate diagnostic and prognosis value of PAQR4.
The KEGG pathway enrichment analysis of 466 hub genes showed that cell cycle and the p53 signaling pathway were significantly enriched pathways. The cell cycle pathway controls multiple cell cycle genes, and is a potential regulator for cancer treatment. Telmisartan suppresses HCC cell growth by modulating cell cycle pathway (18). Methyl-cantharidimide inhibits the proliferation of HCC cells by regulating cell cycle arrest, indicating that methyl-cantharidimide has a cytotoxic effect on HCC cells via inducing cell cycle arrest (19). The CCL14 gene is considered a new prognostic regulator and tumor suppressor of HCC by regulating cell cycle (20). The p53 pathway is one of the typical pathways that control cell-cycle progression, cell growth, and apoptosis, and has been reported to exert important function in the progression of cancer (21,22). The CD147 gene accelerates reprogramming of glucose metabolism and cell growth in HCC cells via suppressing the p53-dependent signaling pathway (23). Pseudogene PDIA3P1 facilitates cell growth, migration, and invasion in HCC through regulating the p53 pathway (24). The PRRX1 gene plays a crucial role in metastasis and apoptosis of HCC cells via modulating the p53 signaling pathway (25). Therefore, we speculated that cell cycle and the p53 signaling pathway might play a crucial role in the occurrence of HCC.
The gene PAQR4 belongs to the PAQR4 family, and is involved in biological processes such as cell proliferation, cell cycle, cell differentiation, and cell death (26-28). It has been reported that PAQR4 plays key roles in multiple cancers. It is markedly elevated in breast cancer tissues and has a tumorigenic effect on breast cancers (26). Overexpression of PAQR4 may be involved in the initiation and progression of non-small-cell lung cancer (28). Expression of PAQR4 is significantly increased in gastric cancer tissues and cell lines, and is an oncogene involved in tumorigenesis (29). High expressed PAQR4 was associated with a worse clinical outcome of non-small-cell lung cancer and can be considered as a potential therapeutic target for non-small-cell lung cancer treatment (30). Overexpressed PAQR4 was detected in prostate cancer tissues and cell lines, which was positively correlated with poor prognosis (31). In our study, PAQR4 was considered as the real hub gene in pathogenesis of HCC. We found that PAQR4 was associated with the prognosis and diagnostic of patients with HCC. The low expression of PAQR4 had a better prognosis than high expression. Similarly, a recent study indicated that HCC patients with high PAQR4 expression have a poor prognosis and speculated that PAQR4 up-regulation in HCC may be due to PAQR4 hypomethylation and PAQR4 copy gain (32). In addition, Zhao et al. suggested that PAQR4 has a tumorigenic effect on HCC progression by activating PI3K/AKT pathway (33). These results showed that PAQR4 might be involved in the occurrence and development of HCC.
In conclusion, starting from TCGA gene profiles of HCC, we obtained 803 DEGs (175 down-regulated and 628 up-regulated) between HCC and normal adjacent samples. Through WGCNA, the blue module was selected to identify the hub genes associated with the HCC. After overlapping all DEGs with the 837 genes of the blue module, we obtained 466 DEGs that were defined as hub genes. Among them, 239 of the 466 hub genes were related to staging. After verification with the GSE36376 dataset, PAQR4 was identified as the real hub gene of HCC. Survival analysis results indicated that high expressed PAQR4 was associated with poor prognosis in patients with HCC, suggesting that PAQR4 might be a novel target for the treatment of HCC. Some limitations of our study should be mentioned. The number of samples used for qRT-PCR confirmation was small. More samples are needed to validate expression of PAQR4. As a pilot study, we are currently focusing on revealing the prognostic and diagnostic significance of PAQR4 in patients with early and late HCC. We are trying to collect patients with HCC samples. Further functional experiments are needed to understand the biological functions and pathogenesis of PAQR4. In addition, in vivo and in vitro experiments are necessary to uncover the biological functions and pathogenesis of PAQR4 in HCC in future work.
Acknowledgments
Funding: This study was supported by Scientific and Technological Transformative Project of Sichuan Scientific Research Institutions (No. 14010127), and the Project of Science and Technology Department of Sichuan Province (No. 2016JY0020).
Footnote
Data Sharing Statement: Available at https://jgo.amegroups.com/article/view/10.21037/jgo-22-168/dss
Conflicts of Interest: All authors have completed the ICMJE uniform disclosure form (available at https://jgo.amegroups.com/article/view/10.21037/jgo-22-168/coif). The authors have no conflicts of interest to declare.
Ethical Statement: The authors are accountable for all aspects of the work in ensuring that questions related to the accuracy or integrity of any part of the work are appropriately investigated and resolved. This study complied with the principles of the Declaration of Helsinki (as revised in 2013). The study was approved by the ethics committee of Sichuan Provincial People’s Hospital, University of Electronic Science and Technology of China (No. 2020161) and informed consent was taken from all the patients.
Open Access Statement: This is an Open Access article distributed in accordance with the Creative Commons Attribution-NonCommercial-NoDerivs 4.0 International License (CC BY-NC-ND 4.0), which permits the non-commercial replication and distribution of the article with the strict proviso that no changes or edits are made and the original work is properly cited (including links to both the formal publication through the relevant DOI and the license). See: https://creativecommons.org/licenses/by-nc-nd/4.0/.
References
- Torre LA, Bray F, Siegel RL, et al. Global cancer statistics, 2012. CA Cancer J Clin 2015;65:87-108. [Crossref] [PubMed]
- Costentin C. Hepatocellular carcinoma surveillance. Presse Med 2017;46:381-5. [Crossref] [PubMed]
- Llovet JM, Zucman-Rossi J, Pikarsky E, et al. Hepatocellular carcinoma. Nat Rev Dis Primers 2016;2:16018. [Crossref] [PubMed]
- European Association for Study of Liver. EASL-EORTC clinical practice guidelines: management of hepatocellular carcinoma. Eur J Cancer 2012;48:599-641. [Crossref] [PubMed]
- Tian N, Shangguan W, Zhou Z, et al. Lin28b is involved in curcumin-reversed paclitaxel chemoresistance and associated with poor prognosis in hepatocellular carcinoma. J Cancer 2019;10:6074-87. [Crossref] [PubMed]
- Falzone L, Salomone S, Libra M. Evolution of Cancer Pharmacological Treatments at the Turn of the Third Millennium. Front Pharmacol 2018;9:1300. [Crossref] [PubMed]
- Ren Z, Ma X, Duan Z, et al. Diagnosis, Therapy, and Prognosis for Hepatocellular Carcinoma. Anal Cell Pathol (Amst) 2020;2020:8157406. [Crossref] [PubMed]
- Christofi T, Baritaki S, Falzone L, et al. Current Perspectives in Cancer Immunotherapy. Cancers (Basel) 2019;11:1472. [Crossref] [PubMed]
- Ko KL, Mak LY, Cheung KS, et al. Hepatocellular carcinoma: recent advances and emerging medical therapies. F1000Res 2020;9:eF1000 Faculty Rev-620.
- Wang Y, Wu N, Liu J, et al. FusionCancer: a database of cancer fusion genes derived from RNA-seq data. Diagn Pathol 2015;10:131. [Crossref] [PubMed]
- Wang Y, Chen L, Ju L, et al. Novel Biomarkers Associated With Progression and Prognosis of Bladder Cancer Identified by Co-expression Analysis. Front Oncol 2019;9:1030. [Crossref] [PubMed]
- Yang H, Li H. CD36 identified by weighted gene co-expression network analysis as a hub candidate gene in lupus nephritis. PeerJ 2019;7:e7722. [Crossref] [PubMed]
- Langfelder P, Horvath S. WGCNA: an R package for weighted correlation network analysis. BMC Bioinformatics 2008;9:559. [Crossref] [PubMed]
- Li J, Zhou D, Qiu W, et al. Application of Weighted Gene Co-expression Network Analysis for Data from Paired Design. Sci Rep 2018;8:622. [Crossref] [PubMed]
- Liu B, Huang G, Zhu H, et al. Analysis of gene coexpression network reveals prognostic significance of CNFN in patients with head and neck cancer. Oncol Rep 2019;41:2168-80. [PubMed]
- Liang JW, Fang ZY, Huang Y, et al. Application of Weighted Gene Co-Expression Network Analysis to Explore the Key Genes in Alzheimer's Disease. J Alzheimers Dis 2018;65:1353-64. [Crossref] [PubMed]
- Liu J, Zhou S, Li S, et al. Eleven genes associated with progression and prognosis of endometrial cancer (EC) identified by comprehensive bioinformatics analysis. Cancer Cell Int 2019;19:136. [Crossref] [PubMed]
- Oura K, Tadokoro T, Fujihara S, et al. Telmisartan inhibits hepatocellular carcinoma cell proliferation in vitro by inducing cell cycle arrest. Oncol Rep 2017;38:2825-35. [Crossref] [PubMed]
- Huang X, Xie W, Yu X, et al. Methyl-Cantharidimide Inhibits Growth of Human Hepatocellular Carcinoma Cells by Inducing Cell Cycle Arrest and Promoting Apoptosis. Front Oncol 2019;9:1234. [Crossref] [PubMed]
- Zhu M, Xu W, Wei C, et al. CCL14 serves as a novel prognostic factor and tumor suppressor of HCC by modulating cell cycle and promoting apoptosis. Cell Death Dis 2019;10:796. [Crossref] [PubMed]
- Till JE, Yoon C, Kim BJ, et al. Oncogenic KRAS and p53 Loss Drive Gastric Tumorigenesis in Mice That Can Be Attenuated by E-Cadherin Expression. Cancer Res 2017;77:5349-59. [Crossref] [PubMed]
- Turrell FK, Kerr EM, Gao M, et al. Lung tumors with distinct p53 mutations respond similarly to p53 targeted therapy but exhibit genotype-specific statin sensitivity. Genes Dev 2017;31:1339-53. [Crossref] [PubMed]
- Huang Q, Li J, Xing J, et al. CD147 promotes reprogramming of glucose metabolism and cell proliferation in HCC cells by inhibiting the p53-dependent signaling pathway. J Hepatol 2014;61:859-66. [Crossref] [PubMed]
- Kong Y, Zhang L, Huang Y, et al. Pseudogene PDIA3P1 promotes cell proliferation, migration and invasion, and suppresses apoptosis in hepatocellular carcinoma by regulating the p53 pathway. Cancer Lett 2017;407:76-83. [Crossref] [PubMed]
- Fan M, Shen J, Liu H, et al. Downregulation of PRRX1 via the p53-dependent signaling pathway predicts poor prognosis in hepatocellular carcinoma. Oncol Rep 2017;38:1083-90. [Crossref] [PubMed]
- Zhang H, Han R, Ling ZQ, et al. PAQR4 has a tumorigenic effect in human breast cancers in association with reduced CDK4 degradation. Carcinogenesis 2018;39:439-46. [Crossref] [PubMed]
- Wang L, Zhang R, You X, et al. The steady-state level of CDK4 protein is regulated by antagonistic actions between PAQR4 and SKP2 and involved in tumorigenesis. J Mol Cell Biol 2017;9:409-21. [Crossref] [PubMed]
- Wu B, Liu R. PAQR4 promotes cell proliferation and metastasis through the CDK4-pRB-E2F1 pathway in non-small-cell lung cancer. Onco Targets Ther 2019;12:3625-33. [Crossref] [PubMed]
- Feng Y, Sun T, Yu Y, et al. MicroRNA-370 inhibits the proliferation, invasion and EMT of gastric cancer cells by directly targeting PAQR4. J Pharmacol Sci 2018;138:96-106. [Crossref] [PubMed]
- Xu P, Jiang L, Yang Y, et al. PAQR4 promotes chemoresistance in non-small cell lung cancer through inhibiting Nrf2 protein degradation. Theranostics 2020;10:3767-78. [Crossref] [PubMed]
- Ye J, Gao M, Guo X, et al. Breviscapine suppresses the growth and metastasis of prostate cancer through regulating PAQR4-mediated PI3K/Akt pathway. Biomed Pharmacother 2020;127:110223. [Crossref] [PubMed]
- Qu C, Ma T, Yan X, et al. Overexpressed PAQR4 predicts poor overall survival and construction of a prognostic nomogram based on PAQR family for hepatocellular carcinoma. Math Biosci Eng 2022;19:3069-90. [Crossref] [PubMed]
- Zhao G, Shi X, Sun Z, et al. PAQR4 promotes the development of hepatocellular carcinoma by activating PI3K/AKT pathway. Acta Biochim Biophys Sin (Shanghai) 2021;53:1602-13. [Crossref] [PubMed]
(English Language Editor: J. Jones)