Development and verification of a hypoxia- and immune-associated prognosis signature for esophageal squamous cell carcinoma
Introduction
Esophageal cancer is one of the most common gastrointestinal malignancies worldwide, with high morbidity and mortality in China (1). Despite the rapid development of multidisciplinary therapies in recent years, the prognosis of esophageal cancer patients is still unsatisfactory (2). Esophageal squamous cell carcinoma (ESCC) and esophageal adenocarcinoma (EAC) are the predominant histological subtypes of esophageal cancer (3). In contrast to western countries, ESCC accounts for approximately 90% of all esophageal cancers in China, and is characterized by rapid progression and poor prognosis.
Hypoxia promotes ESCC progression and metastasis by accelerating tumor angiogenesis and stimulating tumor glycolysis (4,5). Hypoxic environment is significantly related to the poor prognosis in patients with ESCC (6). In addition, hypoxia plays an important role in promoting tumor immunosuppression and immune escape (7). Immune cells including cytotoxic T cells, natural killer cells (NK cells), regulatory T cells (Tregs), activated macrophages, and dendritic cells form the tumor microenvironment (TME) and are involved in the processes of tumor invasion, metastasis, and anti-tumor immune responses (8,9). There is a direct and indirect interaction between hypoxia and immune status in the TME of ESCC (10,11). Nevertheless, the molecular characteristics and mechanisms have not yet been clarified.
Tan et al. (12). revealed that hypoxia is correlated with prognosis and the incidence of immune cell infiltration in patients with esophageal cancer. Our study aimed to develop a prognostic signature composed of hypoxia- and immune-associated genes. Furthermore, we analyzed the correlation between the model and many kinds of infiltrating immune cells. Based on these, we constructed a nomogram model to predict the overall survival (OS) of ESCC patients by integrating the risk score and well-known prognostic factors, with an aim to improve the prognosis of ESCC. We present the following article in accordance with the TRIPOD reporting checklist (available at https://jgo.amegroups.com/article/view/10.21037/jgo-22-69/rc).
Methods
Patients
The RNA-sequencing and clinical data of 173 cases of ESCC and 271 normal tissue samples were obtained from The Cancer Genome Atlas (TCGA) data portal (https://xenabrowser.net/datapages/?dataset=TCGA-ESCA.htseq_counts.tsv&host=https%3A%2F%2Fgdc.xenahubs.net&removeHub=https%3A%2F%2Fxena.treehouse.gi.ucsc.edu%3A443) and the Genotype-Tissue Expression (GTEx) database (https://xenabrowser.net/datapages/?dataset=gtex_RSEM_Hugo_norm_count&host=https%3A%2F%2Ftoil.xenahubs.net&removeHub=http%3A%2F%2F127.0.0.1%3A7222). Then, we combined the 2 sets of samples and performed batch processing on the training set data for analysis. The study was conducted in accordance the Helsinki Declaration (as revised in 2013).
Hypoxia-related genes (HRGs) and immune-related genes (IRGs)
The HRGs list containing 200 genes was downloaded from https://www.gsea-msigdb.org/gsea/msigdb/cards/HALLMARK_HYPOXIA (Table S1). The IRGs list consisting of 1793 genes was retrieved from ImmPort Shared Data (https://immport.org/shared/home), which shares basic cancer immunological data. The IRGs list is shown in the additional file, website: https://cdn.amegroups.cn/static/public/jgo-22-69-1.pdf.
Identification of differentially expressed genes (DEGs), differentially expressed HRGs (DEHRGs), and differentially expressed IRGs (DEIRGs)
DEG analyses were carried out by the DESeq2 method using the edgeR package in the R statistical environment (http://bioconductor.org/packages/edgeR/) (R Development Core Team, Vienna, Austria). A total of 6299 DEGs, including 2164 up-regulated genes and 4135 down-regulated genes, were obtained (with the thresholds of |log2 fold change (FC)| >2.0 and adjusted P<0.05), and the heat map and volcano plot were generated. Based on the intersection of the DEGs and HRGs, 73 genes were obtained as DEHRGs. Similarly, DEIRGs were obtained by intersecting DEGs with IRGs.
Functional enrichment analysis
According to the mRNA expression of the DEHRGs and DEIRGs, Gene Ontology (GO) and Kyoto Encyclopedia of Genes and Genomes (KEGG) enrichment analysis were performed using the R package Cluster Profiler. GO annotation and KEGG pathways were plotted using the GOplot package in R.
Development of a risk score model and validation model
DEHRGs and DEIRGs associated with prognosis were evaluated using univariate Cox proportional hazards analysis. The genes with prognostic significance were selected with P<0.05 as the threshold and the results were illustrated in a forest plot. Then, the least absolute shrinkage and selection operator (LASSO) method (13) was performed for dimensionality reduction filtering using the glmnet package of R. Redundant factors were removed and corresponding genes were selected. In addition, a prognostic risk score model was constructed according to the genes acquired through Cox regression.
In this formula, Coefi is the risk coefficient of signature genes and Xi indicates the identified genes. Coefi was obtained from Cox analysis. Based on the individual risk scores, the samples were classified into high-risk and low-risk categories using the median risk score as the cut-off, and survival curves were plotted using Kaplan-Meier (KM) analysis. In order to verify the stability of the model, subgroup survival curves were generated based on the high- and low-risk groups combined with clinical variables [including smoking status, body mass index (BMI), radiotherapy status, TNM stage, and gender].
Analysis of the relationship between the risk score model and immune cell infiltration
The relative ratios of 22 infiltrating immune cell types in the specimens were calculated by using the CIBERSORT software (14). CIBERSORT is a deconvolution algorithm that uses a set of reference gene expression values (547 genes) to predict the proportions of 22 infiltrating immune cell types from sample expression data by using support vector regression. The association between the risk score model and immune cell infiltration was analyzed.
Development and evaluation of the nomogram
Univariate analysis and Cox proportional hazards analysis were used to determine the independent prognostic factors related to prognosis, including the risk score model and clinicopathological characteristics of the patients. The nomograms were developed to predict the 1-, 2-, and 3-year OS probability (15,16). A calibration curve was drawn to determine the divergence between the nomogram’s predicted probability and the actual incidence. A calibration curve was drawn to define the discrepancy between the predicted probability of the nomogram and the actual incidence.
Statistical analysis
All statistical analyses were carried out using R software v3.5.2. Independent prognostic factors were determined by using a multivariate Cox regression model. Patient survival time was analyzed using KM curves, and the log-rank test was used for statistical analysis. The area under the curve (AUC) of the survival receiver operating characteristic (ROC) curve was calculated via the survival ROC R software package to validate the performance of the prognostic signature (16). P<0.05 was considered to indicate a statistically significant difference.
Results
Identification DEGs, DEIRGs, and DEHRGs
DEG analysis was carried out using the DESeq2 method. A total of 6,299 DEGs were obtained, including 2,164 up-regulated and 4,135 down-regulated genes (based on log2 FC). The heat map and volcano plot were drawn as shown in Figure 1A,1B. The DEGs and 200 HRGs were intersected, and 73 intersection genes were obtained as DEIRGs (Figure 1C). The DEGs and known IRGs were intersected, and 548 intersection genes were obtained as DEHRGs (Figure 1D).
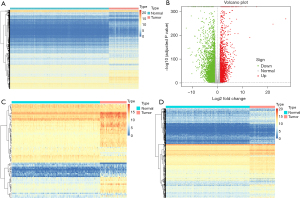
Functional enrichment analysis of DEIRGs and DEHRGs
The biological functions of DEIRGs and DEHRGs were investigated using GO annotation and KEGG pathway analyses. Several hypoxia-related GO terms were identified, such as “small molecule metabolic process”, “extracellular region”, and “transferase activity” (Figure 2A-2C). The most significant hypoxia-related KEGG term was “HIF-1 signaling pathway” (Figure 2D). Several immune-related GO terms were identified, such as “response to chemical”, “extracellular region”, and “signaling receptor binding” (Figure 2E-2G). The most significant immune-related KEGG term was “cytokine-cytokine receptor interaction” (Figure 2H).
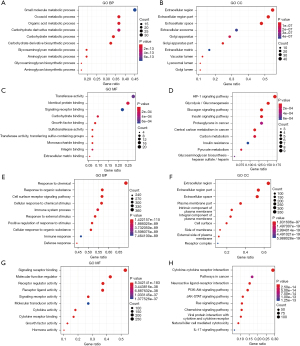
Development of the risk score signature and assessment of its predictive ability
To identified hypoxia-and immune-related prognostic DEGs, 73 DEHRGs and 548 DEIRGs were screened using univariate Cox regression analyses. We obtained 3 DEHRGs genes and 8 DEIRGs genes with significantly effects on patient prognosis, respectively. The results of the Cox analysis are illustrated by forest plots (Figure 3A). Using the LASSO method, a total of 10 genes were obtained by combining 2 sets of factors. Redundant factors were removed, 8 related factors were retained, and a prognostic risk score model was constructed according to the expression values of the 8 factors (Figure 3B-3E). By using the Cox regression model, the risk score of each patient was calculated as follows: risk score = (−0.079 × expression of FABP7) + (−0.143 × expression of TLR1) + (−0.063 × expression of SYTL1) + (0.115 × expression of APLN) + (0.146 × expression of OSM) + (−0.196 × expression of EGFR) + (−0.023 × expression of IL17RD) + (−0.183 × expression of MYH9). Based on this 8-gene-based risk score, a risk prognosis classifier was constructed to classify the samples into high- and low-risk groups according to the median risk score (Figure 4A). Survival curves of high- and low-risk samples were plotted (Figure 4B), which showed that the survival rate of high-risk samples was significantly lower than that of low-risk samples. The risk curve and signature DEG expression patterns are plotted in Figure 4C. In this model, the AUC was 0.715, suggesting that the model could be used for predicting survival (Figure 4D). Heat map of the expression distribution of 8 genes in the model was shown in Figure 4E.
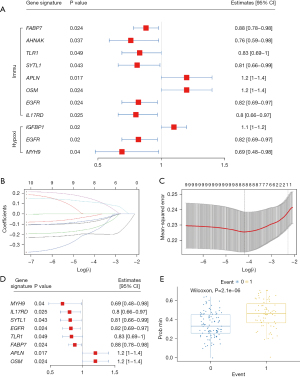
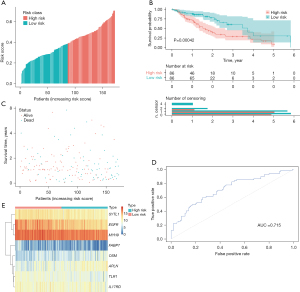
Risk model stability analysis
To determine model stability, the survival curve was plotted based on known clinical variables (including smoking, BMI, radiotherapy, TMN staging, and sex). The clinicopathological characteristics of patients divided into the low- and high-risk groups are listed in Table 1. The risk score model of the 8 clinical variables, such as N0, N1, M0, and male, showed significant intergroup differences (high- and low-risk grouping) in these clinical characteristics, indicating that the predictive efficiency and stability of the model were favorable (Figure 5).
Table 1
Characteristic | Low risk (%) | High risk (%) | P value |
---|---|---|---|
Age | 0.479 | ||
≤65 years | 50 (79.4) | 10 (66.7) | |
>65 years | 13 (20.6) | 5 (33.3) | |
Sex | 0.878 | ||
Female | 9 (14.3) | 3 (20.0) | |
Male | 54 (85.7) | 12 (80.0) | |
Pathologic M | 0.667 | ||
M0 | 57 (90.5) | 14 (93.3) | |
M1 | 3 (4.8) | 0 (0) | |
MX | 3 (4.8) | 1 (6.7) | |
Pathologic N | 0.189 | ||
N0 | 35 (55.6) | 10 (66.7) | |
N1 | 22 (34.9) | 4 (26.7) | |
N2 | 5 (7.9) | 0 (0) | |
N3 | 0 (0) | 1 (6.7) | |
NX | 1 (1.6) | 0 (0) | |
Pathologic T | 0.009 | ||
T1 | 7 (11.1) | 1 (6.7) | |
T2 | 18 (28.6) | 9 (60.0) | |
T3 | 37 (58.7) | 3 (20.0) | |
T4 | 1 (1.6) | 2 (13.3) | |
Neoplasm histologic grade | 0.029 | ||
G1 | 9 (14.3) | 6 (40.0) | |
G2 | 35 (55.6) | 3 (20.0) | |
G3 | 14 (22.2) | 3 (20.0) | |
Missing | 5 (7.9) | 3 (20.0) | |
Radiation therapy | 0.115 | ||
Not reported | 13 (20.6) | 7 (46.7) | |
No | 30 (47.6) | 5 (33.3) | |
Yes | 20 (31.7 | 3 (20.0) | |
BMI exposures | 0.403 | ||
≤24 | 45 (71.4) | 9 (60.0) | |
>24 | 15 (23.8) | 6 (40.0) | |
Missing | 3 (4.8) | 0 (0) | |
Cigarettes per day exposures | 1.000 | ||
≤1 | 9 (14.3) | 3 (20.0) | |
>1 | 27 (42.9) | 7 (46.7) | |
Missing | 27 (42.9) | 5 (33.3) | |
Alcohol history exposures | 0.392 | ||
No | 17 (27.0) | 2 (13.3) | |
Not reported | 2 (3.2) | 0 (0) | |
Yes | 44 (69.8) | 13 (86.7) |
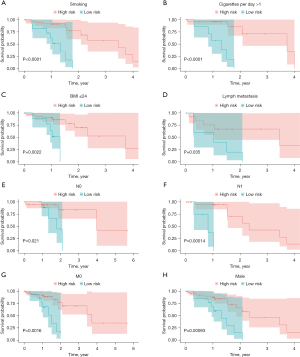
Relationship between the risk score model and immune cell infiltration
As the tumors of the high-risk group were proven to be infiltrated with a large number of immune cells, we further analyzed the correlation between risk score and subtypes of infiltrating immune cells. The results indicated that the levels of infiltrating resting dendritic cells and naïve CD4+ T cells in the high-risk group were lower than those of the low-risk group (P<0.05, Figure 6A-6D). In contrast, the levels of infiltrating activated mast cells, Tregs, and neutrophils in the high-risk group were higher than those of the low-risk group (P<0.05, Figure 6E-6J). Consequently, the high-risk group had abundant immunosuppressive cells, resulting in an immunosuppressive TME, which corresponds to poor prognosis.
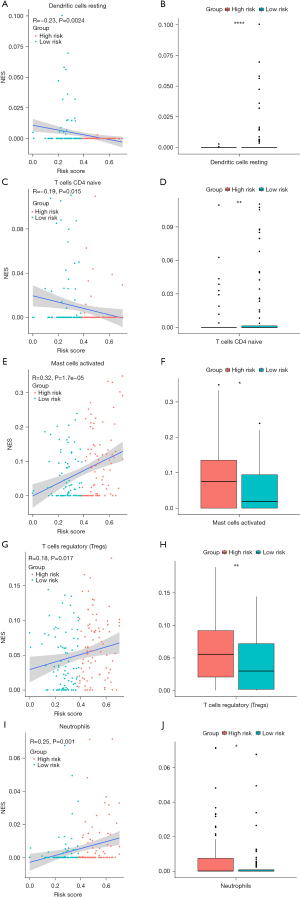
Nomogram model can predict the OS probability of ESCC
The nomogram model was constructed to predict the OS of ESCC patients at 1, 2, and 3 years by integrating the risk score and well-known prognostic factors, including age, BMI, histological grade, and tumor TNM staging information (Figure 7A). The calibration curve approached the ideal curve (black straight line) in the calibration diagram, indicating that the predicted OS probability was compatible with the actual probability (Figure 7B).
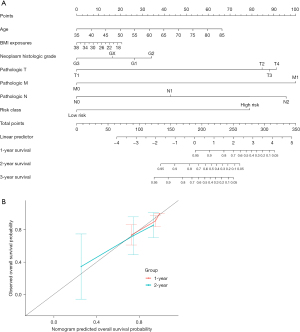
Discussion
Considering the significant differences between the prognoses of ESCC patients, it is crucial to develop a reliable, convenient, and cost-effective prognostic signature to maximize the benefits of personalized treatment and for guiding prognosis. The present study has important guiding value for determining the prognosis of ESCC patients based on gene information obtained from ESCC samples in this study.
Previous studies have indicated that long non-coding RNA (lncRNA) signatures could be used as prognostic tools for patients with ESCC (17,18). Nevertheless, the application of lncRNAs is limited due to their technical specifications and expensive testing costs. A recent study identified an immune-related risk signature to predict the outcome of ESCC patients (19). However, the heterogeneity of the tumor immune microenvironment and tumor hypoxia were not considered in the research. In this study, the comprehensive mining of hypoxia- and immune-associated genes was used to construct a model to aid in prognosis prediction. We identified a series of HRGs and IRGs associated with prognosis in ESCC patients. Moreover, a hypoxia- and immune-associated signature composed of 8 genes was developed as a prognostic classification tool with promising effectiveness and stability. Finally, the nomogram model was constructed to predict the OS of ESCC patients, demonstrating favorable prediction ability.
Hypoxia is a strong aggravator of ESCC progression. It can accelerate tumor glycolysis, angiogenesis, cell proliferation, metastasis, and radio- and chemo-resistance (5,20). Hypoxia, or hypoxic tension, has become one of the hallmarks of the TME (21). Rapid tumor growth requires a large amount of nutrients and oxygen supply, which triggers tumor angiogenesis. However, tumor angiogenesis is highly abnormal and inefficient. Oxygenation of the perivascular tumor area depends on the diffusion gradient relative to the intravascular oxygen partial pressure (PO2), which leads to hypoxia in remote areas (22,23). Studies have confirmed the existence of tumor hypoxia in ESCC, and hypoxia is considered to be a key risk factor of poor prognosis in ESCC patients (23,24).
Furthermore, hypoxia can promote and maintain an immunosuppressive TME (25). Hypoxia mediates immunosuppression mainly by restraining T cell migration into tumor tissue or accelerating T cell apoptosis (26). Besides, hypoxia drives immune evasion through the up-regulation of HIF-1α secretion (27).
In the present study, we found that hypoxia and immune status were correlated with the prognosis of ESCC. The HRGs MYH9 and EGFR were associated with ESCC prognosis, while the IRGs FABP7, TLR1, SYTL1, APLN, OSM, EGFR, and IL17RD were associated with ESCC prognosis. Furthermore, a correlation was also observed between hypoxia, immune status, and survival information. Therefore, these HRGs and IRGs deserve further investigation.
The important roles of the characteristic genes identified in this study have been reported in a variety of cancer types including ESCC. Myosin heavy chain 9 (MYH9) deletion inhibits glycolysis, migration, and invasion of gastric cancer cells in the hypoxic TME (28). MHY9 is a novel mutant gene in ESCC. The expression of MYH9 was significantly up-regulated in ESCC, and was associated with lymph node metastasis in ESCC patients. Moreover, down-regulation of MYH9 gene expression can inhibit cell migration, invasion, and gene expression changes related to angiogenesis and epithelial-mesenchymal transition (EMT) (29). In addition, as the substrate of protein tyrosine phosphatase 1B (PTP1B), MYH9 can be dephosphorylated by PTP1B, thereby up-regulating the expression of epidermal growth factor receptor (EGFR) and enhancing cell migration and invasion in ESCC (30). EGFR is a key molecule in the pathophysiology of ESCC and is highly expressed on the surface of ESCC cells (31). Genetic variants in EGFR are associated with the prognosis of ESCC patients after radical resection (32). Oncostatin M (OSM) regulates tumor infiltration of immune cells and is associated with the outcome of cholangiocarcinoma (33). The expression of OSM is up-regulated in hepatocellular carcinoma (HCC) (34). OSM induces tumor necrosis factor-α (TNF-α) secretion and CD68+ macrophage aggregation. In addition, the expression of OSM is correlated with a low OS rate in HCC (34). Apelin (APLN) is abnormally up-regulated in HCC and is correlated with poor outcome (35). Inhibition of APLN can potently remodel the TME, reduce angiogenesis, and effectively inhibit tumor growth. Moreover, up-regulation of APLN was associated with poor outcome (36). Interleukin-17 receptor D (IL17RD), an immune-related gene, is correlated with the outcome of cervical squamous cell carcinoma (37), muscle-invasive bladder cancer (38), and medullary thyroid cancer (39). Synaptotagmin-like 1 (SYTL1), also known as Slp1, promotes leukemogenesis and facilitates the interaction between leukemic cells and bone marrow stroma (40). The up-regulation of toll-like receptor 1 (TLR1) is associated with better outcomes of pancreatic ductal adenocarcinoma (PDAC) patients (41). TLR1 is highly expressed in breast cancer and may serve as a biomarker of its pathogenesis and progression (42). A high level of fatty acid binding protein 7 (FABP7) is correlated with poor prognosis in several types of malignant tumors (43). A recent study demonstrated that FABP7 was a favorable biomarker for predicting better response to neoadjuvant chemotherapy (NAC) in breast cancer patients (44).
Immune cell infiltration is a key factor affecting tumor progression and prognosis. In recent years, a series of researchers have reported that immune cell infiltration could provide promising signatures for the prognostic prediction of colon cancer (45) and lung adenocarcinoma (46). In this study, we found higher infiltration of Tregs, activated mast cells, and neutrophils in the high-risk group based on our model. Zhang et al. have also reported higher infiltration of Tregs in high-risk patients, which was consistent with our results (19). These findings suggest that high-risk patients are more likely to present with negative immunomodulatory infiltrating cells. Meanwhile, our data contributes to the understanding of immune status of different risk groups, which will be helpful for clinical practice.
However, there were several limitations in our research. First, we included approximately 200 HRGs and 1,793 IRGs in the present study, which might not be sufficient for a comprehensive analysis. Second, tumor hypoxia and immunosuppressive TME were considered to be highly heterogeneous. Considering the characteristics of the tumor, the composition varies according to the location and time of tumor progression, and the predictive ability of our 8-gene hypoxia- and immune-associated signature might vary according to different regions of tumor tissue. Lastly, this research lacked a large cohort and longer follow-up for further validation. Thus, the hypoxia- and immune-associated signature constructed in this study requires further validation by more prospective, multicenter studies.
Conclusions
In conclusion, the present study identified a series of hypoxia- and immune-related genes associated with the prognosis of ESCC patients. Furthermore, we established a practicable and reproducible hypoxia- and immune-associated risk signature for ESCC and revealed new information related to the hypoxia and immune status of ESCC. Ultimately, a nomogram model was constructed to predict the OS of ESCC which demonstrated favorable prediction ability. We believe that our model may contribute to individualized management, follow-up plans, and treatment strategies for ESCC patients.
Acknowledgments
Funding: This work was supported by The Science and Technology Project Foundation of Suzhou (Nos. SS201852, SS202093, and SYSD2020061); the Science and Education for Health Foundation of Suzhou for Youth (No. KJXW2019074); and the Youth Medical Science and Technology Innovation Project of Xuzhou Health Committee (No. XWKYHT20200024).
Footnote
Reporting Checklist: The authors have completed the TRIPOD reporting checklist. Available at https://jgo.amegroups.com/article/view/10.21037/jgo-22-69/rc
Conflicts of Interest: All authors have completed the ICMJE uniform disclosure form (available at https://jgo.amegroups.com/article/view/10.21037/jgo-22-69/coif). The authors have no conflicts of interest to declare.
Ethical Statement: The authors are accountable for all aspects of the work in ensuring that questions related to the accuracy or integrity of any part of the work are appropriately investigated and resolved. The study was conducted in accordance the Helsinki Declaration (as revised in 2013). All data in this article is obtained from the public database.
Open Access Statement: This is an Open Access article distributed in accordance with the Creative Commons Attribution-NonCommercial-NoDerivs 4.0 International License (CC BY-NC-ND 4.0), which permits the non-commercial replication and distribution of the article with the strict proviso that no changes or edits are made and the original work is properly cited (including links to both the formal publication through the relevant DOI and the license). See: https://creativecommons.org/licenses/by-nc-nd/4.0/.
References
- Bray F, Ferlay J, Soerjomataram I, et al. Global cancer statistics 2018: GLOBOCAN estimates of incidence and mortality worldwide for 36 cancers in 185 countries. CA Cancer J Clin 2018;68:394-424. [Crossref] [PubMed]
- Baba Y, Yoshida N, Kinoshita K, et al. Clinical and Prognostic Features of Patients With Esophageal Cancer and Multiple Primary Cancers: A Retrospective Single-institution Study. Ann Surg 2018;267:478-83. [Crossref] [PubMed]
- Rustgi AK, El-Serag HB. Esophageal carcinoma. N Engl J Med 2014;371:2499-509. [Crossref] [PubMed]
- Mao Y, Wang Y, Dong L, et al. Hypoxic exosomes facilitate angiogenesis and metastasis in esophageal squamous cell carcinoma through altering the phenotype and transcriptome of endothelial cells. J Exp Clin Cancer Res 2019;38:389. [Crossref] [PubMed]
- Zhang Q, Zhang J, Fu Z, et al. Hypoxia-induced microRNA-10b-3p promotes esophageal squamous cell carcinoma growth and metastasis by targeting TSGA10. Aging (Albany NY) 2019;11:10374-84. [Crossref] [PubMed]
- Shao N, Han Y, Song L, et al. Clinical significance of hypoxia-inducible factor 1α, and its correlation with p53 and vascular endothelial growth factor expression in resectable esophageal squamous cell carcinoma. J Cancer Res Ther 2020;16:269-75. [Crossref] [PubMed]
- Lin W, Wu S, Chen X, et al. Characterization of Hypoxia Signature to Evaluate the Tumor Immune Microenvironment and Predict Prognosis in Glioma Groups. Front Oncol 2020;10:796. [Crossref] [PubMed]
- Baba Y, Nomoto D, Okadome K, et al. Tumor immune microenvironment and immune checkpoint inhibitors in esophageal squamous cell carcinoma. Cancer Sci 2020;111:3132-41. [Crossref] [PubMed]
- Zheng Y, Chen Z, Han Y, et al. Immune suppressive landscape in the human esophageal squamous cell carcinoma microenvironment. Nat Commun 2020;11:6268. [Crossref] [PubMed]
- Maghsudlu M, Farashahi Yazd E. Heat-induced inflammation and its role in esophageal cancer. J Dig Dis 2017;18:431-44. [Crossref] [PubMed]
- Chang WH, Lai AG. The pan-cancer mutational landscape of the PPAR pathway reveals universal patterns of dysregulated metabolism and interactions with tumor immunity and hypoxia. Ann N Y Acad Sci 2019;1448:65-82. [Crossref] [PubMed]
- Tan L, Cheng D, Wen J, et al. Identification of prognostic hypoxia-related genes signature on the tumor microenvironment in esophageal cancer. Math Biosci Eng 2021;18:7743-58. [Crossref] [PubMed]
- Tibshirani R. The lasso method for variable selection in the Cox model. Stat Med 1997;16:385-95. [Crossref] [PubMed]
- Newman AM, Liu CL, Green MR, et al. Robust enumeration of cell subsets from tissue expression profiles. Nat Methods 2015;12:453-7. [Crossref] [PubMed]
- Iasonos A, Schrag D, Raj GV, et al. How to build and interpret a nomogram for cancer prognosis. J Clin Oncol 2008;26:1364-70. [Crossref] [PubMed]
- Heagerty PJ, Lumley T, Pepe MS. Time-dependent ROC curves for censored survival data and a diagnostic marker. Biometrics 2000;56:337-44. [Crossref] [PubMed]
- Li W, Liu J, Zhao H. Identification of a nomogram based on long non-coding RNA to improve prognosis prediction of esophageal squamous cell carcinoma. Aging (Albany NY) 2020;12:1512-26. [Crossref] [PubMed]
- Zhang L, Li P, Liu E, et al. Prognostic value of a five-lncRNA signature in esophageal squamous cell carcinoma. Cancer Cell Int 2020;20:386. [Crossref] [PubMed]
- Zhang C, Luo Y, Zhang Z, et al. Identification of a Prognostic Immune Signature for Esophageal Squamous Cell Carcinoma to Predict Survival and Inflammatory Landscapes. Front Cell Dev Biol 2020;8:580005. [Crossref] [PubMed]
- Zhang H, Si J, Yue J, et al. The mechanisms and reversal strategies of tumor radioresistance in esophageal squamous cell carcinoma. J Cancer Res Clin Oncol 2021;147:1275-86. [Crossref] [PubMed]
- Buckley AM, Lynam-Lennon N, O'Neill H, et al. Targeting hallmarks of cancer to enhance radiosensitivity in gastrointestinal cancers. Nat Rev Gastroenterol Hepatol 2020;17:298-313. [Crossref] [PubMed]
- Horsman MR, Mortensen LS, Petersen JB, et al. Imaging hypoxia to improve radiotherapy outcome. Nat Rev Clin Oncol 2012;9:674-87. [Crossref] [PubMed]
- Peerlings J, Van De Voorde L, Mitea C, et al. Hypoxia and hypoxia response-associated molecular markers in esophageal cancer: A systematic review. Methods 2017;130:51-62. [Crossref] [PubMed]
- Jing X, Yang F, Shao C, et al. Role of hypoxia in cancer therapy by regulating the tumor microenvironment. Mol Cancer 2019;18:157. [Crossref] [PubMed]
- Chang CH, Qiu J, O'Sullivan D, et al. Metabolic Competition in the Tumor Microenvironment Is a Driver of Cancer Progression. Cell 2015;162:1229-41. [Crossref] [PubMed]
- Joyce JA, Fearon DT. T cell exclusion, immune privilege, and the tumor microenvironment. Science 2015;348:74-80. [Crossref] [PubMed]
- Vito A, El-Sayes N, Mossman K. Hypoxia-Driven Immune Escape in the Tumor Microenvironment. Cells 2020;9:992. [Crossref] [PubMed]
- Fang X, Bai Y, Zhang L, et al. Silencing circSLAMF6 represses cell glycolysis, migration, and invasion by regulating the miR-204-5p/MYH9 axis in gastric cancer under hypoxia. Biosci Rep 2020;40:BSR20201275. [Crossref] [PubMed]
- Yang B, Liu H, Bi Y, et al. MYH9 promotes cell metastasis via inducing Angiogenesis and Epithelial Mesenchymal Transition in Esophageal Squamous Cell Carcinoma. Int J Med Sci 2020;17:2013-23. [Crossref] [PubMed]
- Pan BQ, Xie ZH, Hao JJ, et al. PTP1B up-regulates EGFR expression by dephosphorylating MYH9 at Y1408 to promote cell migration and invasion in esophageal squamous cell carcinoma. Biochem Biophys Res Commun 2020;522:53-60. [Crossref] [PubMed]
- Kikuchi O, Ohashi S, Horibe T, et al. Novel EGFR-targeted strategy with hybrid peptide against oesophageal squamous cell carcinoma. Sci Rep 2016;6:22452. [Crossref] [PubMed]
- Wang YL, Yuan Y, Luo XX, et al. Genetic Variants in EGFR/PLCE1 Pathway Are Associated with Prognosis of Esophageal Squamous Cell Carcinoma after Radical Resection. Curr Med Sci 2019;39:385-90. [Crossref] [PubMed]
- Liu Q, Lan T, Song Y, et al. Oncostatin M expression and TP53 mutation status regulate tumor-infiltration of immune cells and survival outcomes in cholangiocarcinoma. Aging (Albany NY) 2020;12:21518-43. [Crossref] [PubMed]
- Yang X, Shao C, Duan L, et al. Oncostatin M promotes hepatic progenitor cell activation and hepatocarcinogenesis via macrophage-derived tumor necrosis factor-α. Cancer Lett 2021;517:46-54. [Crossref] [PubMed]
- Chen H, Wong CC, Liu D, et al. APLN promotes hepatocellular carcinoma through activating PI3K/Akt pathway and is a druggable target. Theranostics 2019;9:5246-60. [Crossref] [PubMed]
- Uribesalgo I, Hoffmann D, Zhang Y, et al. Apelin inhibition prevents resistance and metastasis associated with anti-angiogenic therapy. EMBO Mol Med 2019;11:e9266. [Crossref] [PubMed]
- Wang Q, Vattai A, Vilsmaier T, et al. Immunogenomic Identification for Predicting the Prognosis of Cervical Cancer Patients. Int J Mol Sci 2021;22:2442. [Crossref] [PubMed]
- Jin K, Qiu S, Jin D, et al. Development of prognostic signature based on immune-related genes in muscle-invasive bladder cancer: bioinformatics analysis of TCGA database. Aging (Albany NY) 2021;13:1859-71. [Crossref] [PubMed]
- Chang YS, Chang CC, Huang HY, et al. Detection of Molecular Alterations in Taiwanese Patients with Medullary Thyroid Cancer Using Whole-Exome Sequencing. Endocr Pathol 2018;29:324-31. [Crossref] [PubMed]
- Yokoyama T, Nakatake M, Kuwata T, et al. MEIS1-mediated transactivation of synaptotagmin-like 1 promotes CXCL12/CXCR4 signaling and leukemogenesis. J Clin Invest 2016;126:1664-78. [Crossref] [PubMed]
- Lanki M, Seppänen H, Mustonen H, et al. Toll-like receptor 1 predicts favorable prognosis in pancreatic cancer. PLoS One 2019;14:e0219245. [Crossref] [PubMed]
- Shi S, Xu C, Fang X, et al. Expression profile of Toll-like receptors in human breast cancer. Mol Med Rep 2020;21:786-94. [PubMed]
- Kagawa Y, Umaru BA, Ariful I, et al. Role of FABP7 in tumor cell signaling. Adv Biol Regul 2019;71:206-18. [Crossref] [PubMed]
- Xie Q, Xiao YS, Jia SC, et al. FABP7 is a potential biomarker to predict response to neoadjuvant chemotherapy for breast cancer. Cancer Cell Int 2020;20:562. [Crossref] [PubMed]
- Zhou R, Zhang J, Zeng D, et al. Immune cell infiltration as a biomarker for the diagnosis and prognosis of stage I-III colon cancer. Cancer Immunol Immunother 2019;68:433-42. [Crossref] [PubMed]
- Zuo S, Wei M, Wang S, et al. Pan-Cancer Analysis of Immune Cell Infiltration Identifies a Prognostic Immune-Cell Characteristic Score (ICCS) in Lung Adenocarcinoma. Front Immunol 2020;11:1218. [Crossref] [PubMed]
(English Language Editor: C. Betlazar-Maseh)