A microarray expression profile and bioinformatic analysis of circular RNA in human esophageal carcinoma
Introduction
Esophageal carcinoma (EC) is the eighth-most cause of morbidity and the sixth-most cause of mortality worldwide, and China has the highest incidence of all countries (1). Currently, the overall 5-year survival rate of EC patients remains very low as these patients are almost diagnosed at an advanced stage (2,3). Therefore, it is crucial to identify new biomarkers and therapeutic targets to improve the diagnosis and treatment of EC.
Noncoding RNAs (ncRNAs) are encoded with approximately 98% of the genome and play important roles in gene expression and regulation (4). Noncoding circular RNAs (circRNAs) comprise a group of endogenous RNAs that are generated by exon transcription through a back-splicing mechanism, and include exonic, intronic, and retained-intron circRNAs (5-7). CircRNAs have no 5' to 3' polarity or poly-A tails; therefore, they are widely expressed in a stable manner in mammalian cells. This raises the possibility that circRNAs may serve as biomarkers in disease diagnosis. Recent studies show that circRNAs can provide a novel type of biomarker to identify cancer (8,9), hepatic steatosis (10), and chronic thromboembolic pulmonary hypertension (11). Reportedly, circRNAs play a crucial role in the development of certain diseases, such as heart failure, diabetes, and cancer (12-14). Several studies have found that circRNAs may function as a microRNA (miRNA) sponge and may compete with endogenous RNA networks (15-17). However, little is known about whether circRNAs can be used as biomarkers for the diagnosis and medical treatment of EC.
Although promising findings have been reported regarding circRNAs in some cancers, no studies have profiled circRNA expression in EC. Therefore, we profiled circRNA expression in EC patients and performed bioinformatic analyses to identify potential circRNAs that may be biomarkers and therapeutic targets for the treatment of EC. We present the following article in accordance with the MDAR reporting checklist (available at https://jgo.amegroups.com/article/view/10.21037/jgo-22-137/rc).
Methods
Samples collection for microarray analysis
All tumor samples were obtained from the Panyu Central Hospital and the Third Affiliated Hospital of Southern Medical University between February 7 and May 2, 2017. All patients were male with an average age of 63.40±2.97 years and were diagnosed through clinical pathology (3 patients had moderately differentiated squamous EC; 1 patient had a highly differentiated squamous EC; and 1 had a moderately differentiated tubular adenocarcinoma of the esophagus). All samples were stored at −80 ℃ after collection. The 10 samples, including the 5 tumors and 5 normal adjacent tissues to serve as controls, were sent to Kangcheng (Shanghai, China) for Arraystar circRNA microarray analysis. All patients who participated in this study signed an informed consent form, and this study was approved by institutional ethics board of Panyu Central Hospital (No. K20170003). The Third Affiliated Hospital of Southern Medical University is also informed and agreed with the study. The study was conducted in accordance with the Declaration of Helsinki (as revised in 2013).
RNA isolation
Total RNA was extracted from the samples using TRIzol reagent (Invitrogen Corp., USA) according to the manufacturer’s instructions. After purification, RNA concentration and quality were determined using a NanoDrop ND-1000 spectrophotometer (NanoDrop, DE, USA). The OD260/OD28 and OD260/OD230 ratios were measured, and the RNA quality was considered suitable for research. The RNA integrity was confirmed using a denaturing agarose gel electrophoresis.
Validation of RNA sample quality
Total RNA was extracted from all samples, and the RNA quality was assessed by spectrophotometry. The OD260/OD280 ratios of the 10 samples were between 1.77 and 1.98, whereas the OD260/OD230 ratios were between 1.99 and 2.38, indicating suitable RNA quality.
Sample labeling, microarray hybridization, and analysis
The Arraystar human circRNA microarray (Arraystar, Rockville, USA) was used to detect and analyze the 10 samples. Total RNAs were digested with RNase R (Epicentre, Inc.) to exclude linear RNA and enrich circRNAs. The enriched circRNAs were then amplified and transcribed with fluorescent complementary RNA (cRNA) using a random priming method (Arraystar Super RNA Labeling Kit; Arraystar). cRNAs with the label were purified using the RNeasy Mini Kit (Qiagen, Germany). After the labeled cRNAs were fragmented, a blocking agent and the fragmentation buffer mixture was heated to 60 ℃ for 30 minutes, and a hybridization buffer was added to dilute the labeled cRNAs. Then, the hybridization solution was dispensed onto a gasket slide, which was assembled with the circRNA expression microarray slide. The slides were incubated for 17 hours at 65 ℃ in an Agilent hybridization oven. Finally, the hybridized arrays were washed, fixed, and scanned using an Agilent G2505C Scanner. Differentially expressed (DE) circRNAs were identified using the Arraystar platform. The circRNAs were considered to be significantly expressed genes if they had a fold change (FC) values >1.5 and P<0.05. Their cluster pattern, scatterplot, and volcano plot were analyzed (18,19).
Prediction of circRNA-miRNA interactions and construction of the circRNA-miRNA coexpression network
CircRNA has been shown to act as an endogenous miRNA sponge and inhibits miRNA-mediated gene expression through miRNA sequestration. To further analyze our results, the circRNA-microRNA interaction was predicted using Arraystar’s in-house software (miRNA target prediction) based on TargetScan and miRanda (20,21). To identify potential associations between the circRNA and miRNA, we selected 50 significantly DE circRNAs (including 35 upregulated and 15 downregulated circRNAs). These 50 circRNAs were screened using a threshold of FC >2.0 and P<0.05, and a coexpression network was constructed using Cytoscape 3.4.0. The resulting nodes represented the 5 putative miRNAs that were the most connected functionally to each circRNA.
Spearman correlation test of DE circRNAs in EC patients
To further examine whether there was a correlation between the expression of each of the 50 DE circRNAs and the incidence of EC, the Spearman correlation test was performed on the expression levels using Excel 2007 (Microsoft Office). Then, the circRNAs were divided into two groups. The first group had a PCC of between 0.95 and 1, while the second group had a Pearson correlation coefficient (PCC) of between −1 and −0.95. The circRNA with the greatest correlation was selected as the hub gene to construct the coexpression network using Cytoscape. Finally, the hub circRNA and its 5 connected miRNA response elements (MREs) were subjected to further bioinformatic analysis of circRNA-miRNA interactions, miRNA-mRNA interactions, miRNA target gene prediction. Kyoto Encyclopedia of Genes and Genomes (KEGG) analysis was performed using TarBase, which was included in the software of ClueGO from Cytoscape.
Prediction of cancer-related circRNA-miRNA and KEGG pathway
To determine whether the DE of the 50 circRNAs was related to EC tumorigenesis, we used the DIANA-MiRpath v.3 platform to predict cancer pathway miRNAs and cancer-related miRNAs using a KEGG reverse search, in TarBase 7.0, based on a P value threshold of 0.05 (22). The predicted miRNAs were identified from the initial list of 221 MREs obtained from the Arraystar analysis. After the cancer-related circRNA-miRNA axis was identified, the target genes of the miRNAs were predicted using the miTarBase software option included in CluePedia of Cytoscape. Finally, the KEGG pathway of circRNA-miRNA-mRNAs was predicted using ClueGO in Cytoscape. The axis predicting a cancer-related pathway was further analyzed for its protein association using the string software option in ClueGO. A P value of ≤0.05 was set as the threshold.
PCR sample collection
All tissue samples were collected from the Panyu Central Hospital and the Third Affiliated Hospital of Southern Medical University between October 6, 2014, and May 2, 2017. All patients were male with an average age of 63.20±6.20 years and were diagnosed through pathology (14 patients had squamous EC, and 1 patient had adenocarcinoma). The samples were stored at −80 ℃ after collection. Samples (n=5) of EC tissues and adjacent normal tissue were sent for circRNA microarray analysis, The samples (n=15), comprising 15 tumors and 15 normal adjacent tissues used as controls, were analyzed for the gene expressions of hsa_circRNA_401955, and hsa_circRNA_100375, and the top 3 upregulated and the top 5 downregulated circRNAs were identified using real-time polymerase chain reaction (RT-PCR).
RT-PCR detection
Total RNA was extracted from all samples according to the processing instructions. First-strand cDNA was synthesized using 1 µg of total RNA per sample (Gene copoeia, Inc., USA). Subsequently, the cDNA samples were amplified (Gene copoeia, Inc., USA) in a final volume of 20 µL in an ABI Vii7 dx instrument (ABI, USA). Amplifications were performed as follows: 2 minutes at 50 ℃, followed by denaturation for 30 seconds at 95 ℃, and 45 cycles of 95 ℃ for 5 seconds, and 60 ℃ for 34 seconds. The experiments were carried out in triplicate. β-actin was used as an endogenous reference control. The relative gene expression level was calculated using the 2−ΔΔCt method. The primer pairs for circRNAs and β-actin are shown in Table 1.
Table 1
Gene | Primer (5'-3') |
---|---|
hsa_circRNA_401955 | F: AAATTTCAGTATTTGCTGTCAAAACA; R: CTTCCCTGTTGGGAGAAACA |
hsa_circRNA_100375 | F: TGTCTCCCATTCCCGTCTTC; R: TAGTCCACCTCATTCTGCCC |
hsa_circRNA_102034 | F: CAGACAAAGACAGCAGGTTCC; R: TGTTGGAACTCTCTCTGGGG |
hsa_circRNA_100191 | F: AGGAGGATGAGATGCCAGTT; R: CTGGGAGGGATGGAGAAACG |
hsa_circRNA_101009 | F: TACTTCTCCAGCAACCCCTG; R: GGAGAGCAACTACAGTATCCTCA |
hsa_circRNA_102459 | F: AGACGATCTCTCTGAGGCCTA; R: CAGCAGGTGGTAGAACTCCT |
hsa_circRNA_037767 | F: TCAGCATCCCCAGTTACGAG; R: CGATGGCCTTGACCTCATTG |
hsa_circRNA_043621 | F: GCTGACCTGGAGATGCAGAT; R: TGTCTCATACTTGGTGCGGA |
hsa_circRNA_087961 | F: GGGCGTGATCATGAAAGGTG; R: CCGCAGACCTCCTCATTCTA |
hsa_circRNA_404474 | F: TTCCCGACCTCCAAGTACAC; R: TCCTCTAGCATGGCCTTCTG |
β-actin-F | F: ACTCTTCCAGCCTTCCTTCC; R: GTACTTGCGCTCAGGAGGAG |
RT-PCR, real-time polymerase chain reaction.
Statistical analysis
Agilent Feature Extraction software (version 11.0.1.1) was used to analyze the acquired array images. The R software package limma was used for data quantile normalization and analysis. The significance of the differences between the tumor and normal adjacent tissue was estimated using a t-test. CircRNAs with FC >1.5 and P<0.05 were selected as significantly DE circRNAs for the cluster, scatter, and volcano plot analyses. P<0.05 was considered statically significant. A Spearman correlation test was performed using the correlation coefficient method of data analysis in Excel 2007.
Data access
Microarray data for all the samples have been deposited in the Gene Expression Omnibus (GEO) under the accession number of GSE103104.
Results
Validation of the RNA sample quality
RNA sample quality was assessed by electrophoresis. RNA integrity was shown to be suitable, and no evidence of DNA contamination was observed (Figure 1A).
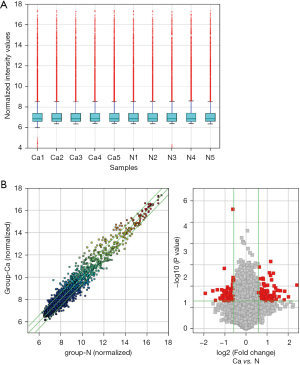
CircRNA expression profiling of EC patients
The Arraystar Human circRNA Microarray was used to identify DE circRNAs in the EC tumors and the normal adjacent tissues. A total of 11,926 circRNAs were detected by the microarray. After normalization of the raw data, screening for log2 FC values greater than 1.5 and P values less than 0.05 showed that 102 circRNAs were significantly upregulated and 67 were downregulated in the tumor tissues. However, FC values greater than 2.0 and P values less than 0.05 showed that only 35 circRNAs were significantly upregulated and 15 were downregulated (Table 2). Hsa_circRNA_043621 and hsa_circRNA_102459 were identified as having the most significant upregulated and downregulated fold changes, respectively. Scatterplot and volcano plot visualization were constructed to identify differences in expression of the circRNAs in the tumor and normal adjacent tissues (Figure 1B). Hierarchical clustering analysis at the expression levels of the circRNAs was performed for the samples (Figure 2).
Table 2
circRNA | P value | FDR | FC | Predicted MREs | ||||
---|---|---|---|---|---|---|---|---|
MRE1 | MRE2 | MRE3 | MRE4 | MRE5 | ||||
Upregulation | ||||||||
hsa_circRNA_028826 | 0.0361 | 0.5643 | 2.0161 | hsa-miR-93-3p | hsa-miR-7843-5p | hsa-miR-4530 | hsa-miR-4685-5p | hsa-miR-92a-2-5p |
hsa_circRNA_053294 | 0.0091 | 0.5642 | 2.0285 | hsa-miR-875-3p | hsa-miR-584-5p | hsa-miR-3074-5p | hsa-miR-3622a-3p | hsa-miR-4286 |
hsa_circRNA_101287 | 0.0296 | 0.5643 | 2.5694 | hsa-miR-499a-3p | hsa-miR-526b-5p | hsa-miR-363-3p | hsa-miR-208a-5p | hsa-miR-578 |
hsa_circRNA_100375 | 0.0421 | 0.5668 | 2.6894 | hsa-miR-324-3p | hsa-miR-29a-5p | hsa-miR-580-3p | hsa-miR-485-3p | hsa-miR-149-5p |
hsa_circRNA_405813 | 0.0186 | 0.5642 | 2.0341 | hsa-miR-665 | hsa-miR-6884-5p | hsa-miR-4459 | hsa-miR-1285-3p | hsa-miR-1273g-3p |
hsa_circRNA_401955 | 0.0275 | 0.5643 | 2.1596 | hsa-miR-1277-5p | hsa-miR-3064-5p | hsa-miR-642a-5p | hsa-miR-6504-5p | hsa-miR-141-5p |
hsa_circRNA_404905 | 0.0310 | 0.5643 | 2.3807 | hsa-miR-8060 | hsa-miR-185-5p | hsa-miR-5008-3p | hsa-miR-4793-3p | hsa-miR-656-5p |
hsa_circRNA_102339 | 0.0006 | 0.5320 | 2.2427 | hsa-miR-875-5p | hsa-miR-215-5p | hsa-miR-192-5p | hsa-let-7a-2-3p | hsa-miR-208a-5p |
hsa_circRNA_100045 | 0.0163 | 0.5642 | 2.0009 | hsa-miR-764 | hsa-miR-149-3p | hsa-miR-650 | hsa-miR-659-5p | hsa-miR-93-3p |
hsa_circRNA_406487 | 0.0330 | 0.5643 | 2.2969 | hsa-miR-297 | hsa-miR-541-3p | hsa-miR-155-5p | hsa-miR-153-5p | hsa-miR-4775 |
hsa_circRNA_092512 | 0.0396 | 0.5643 | 2.0555 | hsa-miR-4763-3p | hsa-miR-3619-5p | hsa-miR-7150 | hsa-miR-1207-5p | hsa-miR-6756-5p |
hsa_circRNA_103923 | 0.0445 | 0.5713 | 2.0984 | hsa-miR-429 | hsa-miR-23b-5p | hsa-miR-200b-3p | hsa-miR-105-3p | hsa-miR-656-5p |
hsa_circRNA_001655 | 0.0313 | 0.5643 | 2.9594 | hsa-miR-6813-5p | hsa-miR-5001-5p | hsa-miR-762 | hsa-miR-4498 | hsa-miR-185-3p |
hsa_circRNA_400082 | 0.0158 | 0.5642 | 2.7868 | hsa-miR-485-5p | hsa-miR-648 | hsa-miR-574-5p | hsa-miR-454-3p | hsa-let-7g-3p |
hsa_circRNA_101693 | 0.0280 | 0.5643 | 2.3458 | hsa-miR-1301-3p | hsa-miR-384 | hsa-miR-141-5p | hsa-miR-646 | hsa-miR-373-5p |
hsa_circRNA_033628 | 0.0165 | 0.5642 | 3.0872 | hsa-miR-4763-3p | hsa-miR-612 | hsa-miR-3615 | hsa-miR-6511b-5p | hsa-miR-635 |
hsa_circRNA_043621 | 0.0089 | 0.5642 | 5.3218 | hsa-miR-223-3p | hsa-miR-4268 | hsa-miR-3692-3p | hsa-miR-657 | hsa-miR-6871-3p |
hsa_circRNA_405551 | 0.0382 | 0.5643 | 2.3020 | hsa-miR-6895-5p | hsa-miR-378i | hsa-miR-1236-3p | hsa-miR-6736-5p | hsa-miR-892b |
hsa_circRNA_404474 | 0.0174 | 0.5641 | 3.5533 | hsa-miR-6743-3p | hsa-miR-3157-5p | hsa-miR-1205 | hsa-miR-378h | hsa-miR-5009-5p |
hsa_circRNA_048574 | 0.0266 | 0.5643 | 2.4765 | hsa-miR-4725-3p | hsa-miR-6858-5p | hsa-miR-5698 | hsa-miR-4723-5p | hsa-miR-7843-5p |
hsa_circRNA_084900 | 0.0051 | 0.5642 | 2.3135 | hsa-miR-4778-3p | hsa-miR-877-3p | hsa-miR-6881-3p | hsa-miR-5196-3p | hsa-miR-6809-3p |
hsa_circRNA_061346 | 0.0123 | 0.5642 | 2.6457 | hsa-miR-4778-3p | hsa-miR-5196-3p | hsa-miR-5193 | hsa-miR-877-3p | hsa-miR-103a-2-5p |
hsa_circRNA_027446 | 0.0193 | 0.5642 | 2.5080 | hsa-miR-129-5p | hsa-miR-331-3p | hsa-miR-6882-3p | hsa-miR-3925-3p | hsa-miR-1236-3p |
hsa_circRNA_001244 | 0.0025 | 0.5598 | 2.4528 | hsa-miR-6861-5p | hsa-miR-619-5p | hsa-miR-1303 | hsa-miR-125a-3p | hsa-miR-5787 |
hsa_circRNA_406748 | 0.0440 | 0.5713 | 3.5072 | hsa-miR-5683 | hsa-miR-588 | hsa-miR-4538 | hsa-miR-6740-5p | hsa-miR-7110-3p |
hsa_circRNA_067209 | 0.0400 | 0.5643 | 2.3571 | hsa-miR-8082 | hsa-miR-146b-3p | hsa-miR-6876-3p | hsa-miR-4534 | hsa-miR-7112-3p |
hsa_circRNA_101877 | 0.0024 | 0.5598 | 2.1485 | hsa-miR-449c-5p | hsa-miR-27a-3p | hsa-miR-27b-3p | hsa-miR-887-5p | hsa-miR-636 |
hsa_circRNA_404864 | 0.0498 | 0.5758 | 2.3992 | hsa-miR-4742-3p | hsa-miR-4753-3p | hsa-miR-4516 | hsa-miR-7847-3p | hsa-miR-4708-3p |
hsa_circRNA_050998 | 0.0031 | 0.5642 | 2.2380 | hsa-miR-433-3p | hsa-miR-2277-5p | hsa-miR-937-5p | hsa-miR-1178-5p | hsa-miR-5089-5p |
hsa_circRNA_006226 | 0.0459 | 0.5713 | 2.2582 | hsa-miR-548a-5p | hsa-miR-548i | hsa-miR-4668-3p | hsa-miR-548ab | hsa-miR-548ar-5p |
hsa_circRNA_090364 | 0.0098 | 0.5642 | 2.5156 | hsa-miR-378h | hsa-miR-378f | hsa-miR-378b | hsa-miR-378c | hsa-miR-378a-3p |
hsa_circRNA_089761 | 0.0318 | 0.5643 | 4.0300 | hsa-miR-3529-3p | hsa-miR-6891-3p | hsa-miR-554 | hsa-miR-5196-3p | hsa-miR-384 |
hsa_circRNA_071312 | 0.0340 | 0.5643 | 2.2288 | hsa-miR-4476 | hsa-miR-4722-5p | hsa-miR-377-3p | hsa-miR-1207-5p | hsa-miR-3140-5p |
hsa_circRNA_074306 | 0.0159 | 0.5642 | 2.8455 | hsa-miR-4763-3p | hsa-miR-3157-5p | hsa-miR-1249-5p | hsa-miR-198 | hsa-miR-6797-5p |
hsa_circRNA_055755 | 0.0410 | 0.5643 | 2.1324 | hsa-miR-7156-3p | hsa-miR-6845-5p | hsa-miR-103a-2-5p | hsa-miR-211-5p | hsa-miR-4685-3p |
Downregulation | ||||||||
hsa_circRNA_102459 | 0.0219 | 0.5642 | 3.8287 | hsa-miR-508-5p | hsa-miR-766-3p | hsa-miR-328-3p | hsa-miR-761 | hsa-miR-497-3p |
hsa_circRNA_102034 | 0.0168 | 0.5642 | 2.9900 | hsa-miR-26b-3p | hsa-miR-382-5p | hsa-miR-181a-3p | hsa-miR-330-5p | hsa-miR-125a-3p |
hsa_circRNA_060102 | 0.0338 | 0.5643 | 2.1602 | hsa-miR-4459 | hsa-miR-5787 | hsa-miR-6736-5p | hsa-miR-4270 | hsa-miR-6804-5p |
hsa_circRNA_101009 | 0.0365 | 0.5643 | 2.3586 | hsa-miR-653-5p | hsa-miR-509-3p | hsa-miR-584-3p | hsa-miR-370-3p | hsa-miR-93-3p |
hsa_circRNA_406963 | 0.0362 | 0.5643 | 2.1734 | hsa-miR-4668-5p | hsa-miR-4739 | hsa-miR-3153 | hsa-miR-608 | hsa-miR-372-5p |
hsa_circRNA_102033 | 0.0278 | 0.5643 | 2.2037 | hsa-miR-382-5p | hsa-miR-330-5p | hsa-miR-26b-3p | hsa-miR-489-3p | hsa-miR-514a-5p |
hsa_circRNA_100191 | 0.0148 | 0.5642 | 2.5797 | hsa-miR-647 | hsa-miR-588 | hsa-miR-660-3p | hsa-miR-661 | hsa-miR-552-3p |
hsa_circRNA_085362 | 0.0375 | 0.5643 | 2.1945 | hsa-miR-1248 | hsa-miR-1205 | hsa-miR-623 | hsa-miR-5002-5p | hsa-miR-4753-3p |
hsa_circRNA_037767 | 0.0466 | 0.5713 | 2.7396 | hsa-miR-1205 | hsa-miR-6772-3p | hsa-miR-3907 | hsa-miR-1184 | hsa-miR-4664-3p |
hsa_circRNA_050898 | 0.0204 | 0.5642 | 2.0588 | sa-miR-3909 | hsa-miR-4691-5p | hsa-miR-1226-5p | hsa-miR-6792-3p | hsa-miR-6762-3p |
hsa_circRNA_100239 | 0.0165 | 0.5642 | 2.1057 | hsa-miR-602 | hsa-miR-22-3p | hsa-miR-320b | hsa-miR-320a | hsa-miR-761 |
hsa_circRNA_101319 | 0.0477 | 0.5729 | 2.0857 | hsa-miR-138-5p | hsa-miR-135a-3p | hsa-miR-338-3p | hsa-miR-135b-5p | hsa-miR-135a-5p |
hsa_circRNA_103942 | 0.0395 | 0.5643 | 2.3015 | hsa-miR-382-5p | hsa-miR-183-3p | hsa-miR-134-3p | hsa-miR-328-5p | hsa-miR-541-3p |
hsa_circRNA_054220 | 0.0148 | 0.5642 | 2.2275 | hsa-miR-513b-5p | hsa-miR-4435 | hsa-miR-6780b-5p | hsa-miR-335-3p | hsa-miR-4665-5p |
hsa_circRNA_402901 | 0.0015 | 0.5320 | 2.0481 | hsa-miR-6845-3p | hsa-miR-4279 | hsa-miR-450a-2-3p | hsa-miR-520h | hsa-miR-520g-3p |
There were 35 upregulated and 15 downregulated circRNAs that had a fold change of more than 2 and a P value less than 0.05. MREs, miRNA response elements; FDR, false discovery rate. FC, fold change.
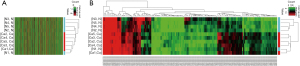
Prediction of the MREs of circRNAs and construction of the circRNA-miRNA coexpression network
CircRNA-miRNA interactions were predicted using the Arraystar in-house software (miRNA target prediction). A total of 221 MREs were predicted to combine with the DE circRNAs based on our predefined FC >2.0 and P<0.05 thresholds (Table 2). A circRNA-miRNA coexpression network was constructed to show the potential associations among the circRNAs and miRNAs (Figure 3).
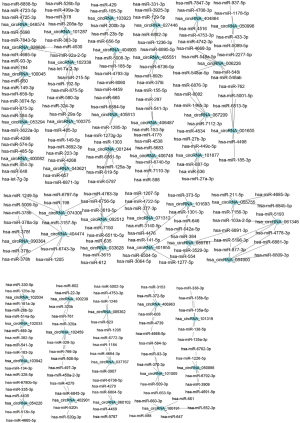
Spearman correlation test of DE circRNAs
The PCC value was analyzed using Spearman’s correlation test. Values between 0.95 and 1 and between −1 and −0.95 were chosen to calculate the association between the two circRNAs. The result showed that hsa_circRNA_401955 had 6 PCC values (0.970 with hsa_circRNA_406487, 0.976 with hsa_circRNA_092512, 0.981 with hsa_circRNA_400082, 0.958 with hsa_circRNA_404864, 0.973 with hsa_circRNA_074306, and 0.990 with hsa_circRNA_055755) within the above ranges and was therefore considered the most connected circRNA. The relation network of the hub circRNA and its connected circRNAs is shown in Figure 4. Red and green nodes represent upregulated and downregulated circRNAs, respectively. The circRNA-miRNA coexpression network showed that hsa_circRNA_401955 was connected to hsa-miR-141-5p, hsa-miR-642a-5p, hsa-miR-1277-5p, hsa-miR-3064-5p, and hsa-miR-6504-5p. The combined sites between the circRNA and the MREs were predicted by Arraystar in-house software (miRNA target prediction) (Figure 5). KEGG pathway analysis showed that 4 primary pathways (including mRNA surveillance, cytoskeleton actin regulation, the spliceosome and NOD-like receptor signaling pathway) in the target gene of MREs were associated with the hub circRNA (P<0.05; Figure 6).
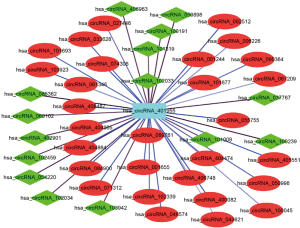
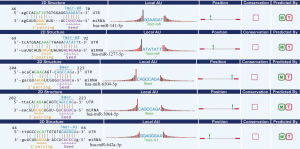
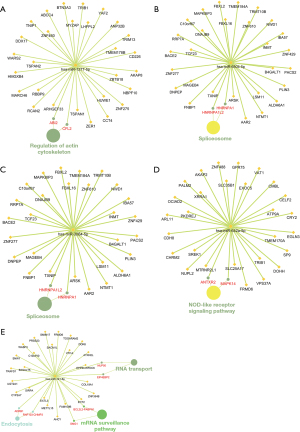
Prediction of cancer-related circRNA-miRNA target genes and KEGG pathways
To further understand the molecular mechanisms of the circRNAs, cancer pathway and cancer-related miRNAs were predicted using DIANA-MiRpath v.3 and identified from the initial list of 221 MREs. Four miRNA axes were identified among the MREs [(I) hsa-miR-324-3p/hsa-miR-29a-5p/hsa-miR-485-3p/hsa-miR-149-5p; (II) hsa-miR-485-5p/hsa-miR-574-5p/hsa-miR-454-3p/hsa-let-7g-3p; (III) hsa-miR-26b-3p/hsa-miR-382-5p/hsa-miR-181a-3p/hsa-miR-330-5p and (IV) hsa-miR-138-5p/hsa-miR-338-3p/hsa-miR-135b-5p/hsa-miR-135a-5p). The circRNA-miRNA coexpression network showed that these MREs interacted with hsa_circRNA_100375, hsa_circRNA_400082, hsa_circRNA_102034, and hsa_circRNA_101319. The target genes of the interacting miRNAs axis were predicted using the miRTarBase software option included in CluePedia of Cytoscape. The KEGG pathway of the miRNA-mRNA network was constructed using ClueGo. The results showed that the axis of has_circRNA_100375/hsa-miR324-3p/hsa-miR29a-5p/hsa-miR-485-3p/hsa-miR-149-5p was involved in a cancer-related pathway (Figure 7A). The seed sequence of the combined sites of the circRNA and 4 miRNAs was obtained using the Arraystar in-house software (miRNA target prediction) (Figure 7B). Finally, we further analyzed the proteins in a cancer-related pathway with their two closely related neighborhoods. The results showed that fibroblast growth factor receptor 1 (FGFR1), prostaglandin E receptor 2 (PTGER2), Wnt family member 9B (WNT9B), Wnt family member 2B (WNT2B), Sp1transcription factor (SP1), CREB binding protein (CREBBP), interleukin 6 (IL-6), and disheveled segment polarity protein2 (DVL2) played key roles in the pathway (Figure 7C).
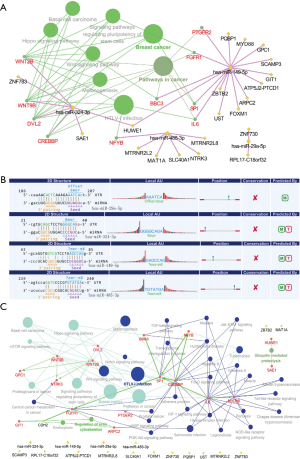
RT-PCR validation
The results showed that the expressions of hub genes hsa_circRNA_401955 and hsa_circRNA_100375 were significantly increased in the tumor samples compared to the normal adjacent tissues in the patients with EC (P<0.05; Figure 8A). The 3 most upregulated circRNAs (hsa_circRNA_043621, hsa_circRNA_087961, and hsa_circRNA_404474) and the 5 most downregulated circRNAs (hsa_circRNA_102034, hsa_circRNA_100191, hsa_circRNA_101009, hsa_circRNA_102459, and hsa_circRNA_037767) identified were also validated by RT-PCR. Compared to the controls, the results showed that the relative gene expressions of the upregulated circRNAs were all significantly increased in tumor samples, while the downregulated circRNAs were decreased in the tumor samples (P<0.05; Figure 8B).
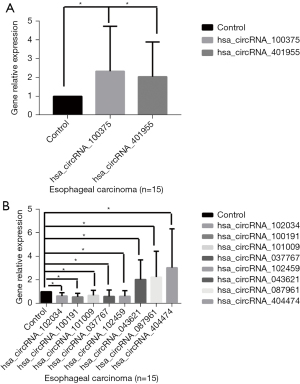
Discussion
In recent years, circRNA has become an important research topic because of its key role in the regulation of gene expression (23). After being found in RNA viruses, circRNAs were initially regarded as splicing errors (24). The development of sequencing technology has enabled more than 7,000 human diseases related to circRNAs to be identified, and reports continue to show that circRNAs promote the progression of some cancers (15,16,25,26) and the development of diseases such as nervous system diseases (27,28) and atherosclerosis diseases (29). Three types of circRNAs have been identified: exonic circRNA (the 5' end connects to the 3' end as a cyclic annular form), circular intronic RNA (the 5' end connects to the 2' end as a cyclic annular form), and exon-intron circular RNAs (induced by reverse splicing, these RNAs connect with exons and introns) (30,31). CircRNAs are widely expressed and have the properties of stability, conservation, and tissue specificity, which make them perfectly suited to be biomarkers that can be used in disease diagnosis (32).
In this study, we found 169 significantly DE circRNAs, including 102 upregulated and 67 downregulated circRNAs, in EC tumors compared to normal adjacent tissues based on a microarray analysis. The 3 most upregulated circRNAs and the 5 most downregulated circRNAs identified were validated by RT-PCR. The results showed that, compared to the normal adjacent tissues of EC patients, the relative gene expressions of the upregulated circRNAs were significantly increased in the tumor samples, while gene expression of the downregulated circRNAs were decreased in the tumor samples. These findings indicate that circRNA dysregulation may be associated with EC tumorigenesis and that certain key circRNAs may be biomarkers for EC.
Reports show that certain circRNAs, especially exonic circRNAs, can inhibit the miRNA-mediated regulation of gene expression by sequestering the relevant miRNAs (33). These circRNAs combine with miRNAs and inhibit the degradation of mRNAs through competitive integration with miRNAs (34). CircRNAs function as miRNA sponges (for example, ciRS-7, which adsorbs to miRNA-7 through its binding site and hence quenches normal miRNA-7 functions) (35-37). Our findings indicate that circRNA can directly suppress miRNAs and inhibit downstream miRNA target genes, thereby acting as a feedback loop in the circRNA-miRNA-mRNA network.
In our study, we identified circRNA-miRNA interactions using Arraystar in-house software (miRNA target prediction). In total, 221 MREs were predicted to combine with the DE circRNAs based on our predefined thresholds of FC >2.0 and P<0.05 thresholds. The Spearman correlation test results showed that hsa_circRNA_401955 was the most connected circRNA and was therefore regarded as the hub. This circRNA is an exonic circRNA and is located on chr18 from 32386182 to 32392077, which is annotated as hsa_circ_0108310 in circBase, and its gene symbol is dystrobrevin alpha (DTNA). This gene encodes a cytoskeleton-interacting membrane protein that is involved in the formation and stability of synapses in the permeability of the blood-brain barrier and may be related to congenital heart malformation (38,39). However, the role of hsa_circRNA_401955 in cancer has not been studied. Based on the MRE analysis, we observed that 5 MREs (hsa-miR-141-5p, hsa-miR-642a-5p, hsa-miR-1277-5p, hsa-miR-3064-5p and hsa-miR-6504-5p) were connected to this hub circRNA. Recent study shows that hsa-miR-141-5p is significantly upregulated in ovarian cancer tissue compared to normal tissue (40). Hsa-miR-642a-5p was identified as the competing endogenous RNA in regulating Linc00974 and KRT19 to affect the proliferation and invasion of hepatocellular carcinoma (41). Hsa-miR-1277-5p was determined to be a prognostic marker for colorectal cancer staging (42). Hsa-miR-3064-5p was found to be downregulated in aristolochic acid (AAN)-upper urinary tract carcinoma (UUC) and in non-AAN-UUC based on miRNA microarray profiling analysis (43). Hsa-miR-6504-5p was found to target the TRAF3 interacting protein 2 (TRAF3IP2), which plays a central role in the innate immune system response to pathogens, inflammatory signals, and stress (44). KEGG analysis showed that 4 primary pathways (mRNA surveillance, cytoskeleton actin regulation, the spliceosome, and the NOD-like receptor signaling pathway) were predicted within the hub circRNA-miRNA-mRNA network. These results indicate that the hub circRNA and the genes in the pathway are potential therapeutic targets and may help to advance our understanding of the mechanism underlying EC tumorigenesis.
To further analyze the role of the circRNA-miRNA in EC patients, we used the DIANA-MiRpath v.3 platform to predict the cancer-related miRNAs. Interestingly, 4 miRNA axes were identified from the MREs. From the circRNA-miRNA coexpression network, we observed that the 4 axes were combined with the 4 circRNAs (hsa_circRNA_100375, hsa_circRNA_400082, hsa_circRNA_102034 and hsa_circRNA_101319, respectively). MiRNAs always negatively regulate their target genes (45-47). To identify the key pathway in the 4 miRNA axes, we used the miRTarBase software implemented in CluePedia of Cytoscape to predict the miRNA target genes and used ClueGo to predict the KEGG pathway (48). Results showed that the hsa_circRNA_100375/hsa-miR-324-3p/hsa-miR-29a-5p/hsa-miR-485-3p/hsa-miR-149-5p axis was involved in a cancer-related pathway. Hsa_circRNA_100375 is also exonic and is located on chr1 from165859440 to 165860559, which is annotated as hsa_circ_0006758 in circBase, and its gene symbol is uridine-cytidine kinase 2 (UCK2). UCK2 has been reported to encode a pyrimidine ribonucleoside kinase [the functions of which include the phosphorylation of uridine and cytidine to uridine monophosphate (UMP) and cytidine monophosphate (CMP), respectively] and has been implicated in the uncontrolled proliferation of abnormal cells, which is a hallmark of cancer (49). Reports show that hsa-miR-324-3p is related to kidney cancer (50). Hsa-miR-29a-5p is significantly downregulated in laryngeal carcinoma (51). Hsa-miR-485-3p was found to be significantly downregulated in stage II colorectal cancer (CRC) tumors compared to normal tissues (52). Hsa-miR-149-5p contains a binding site for the long noncoding RNA MALAT1, which promotes the development of triple-negative breast cancer (53). KEGG pathway analysis in the cancer-related axis showed that certain hub proteins, such as FGFR1, PTGER2, WNT9B, WNT2B, SP1, CREBBP, IL-6 and DVL2, may play a key role in this pathway. Thus, these hub proteins maybe provide candidate targets for the treatment of EC. These hub proteins and hsa_circRNA_100375 may be the key genes regulating EC tumorigenesis.
Finally, the expression of hsa_circRNA_100375 and hsa_circRNA_401955 was detected in 15 EC patients by PCR. The results showed that these 2 hub circRNAs were significantly increased in the tumor samples compared to the normal adjacent tissues. These results indicate that the hsa_circRNA_100375 and hsa_circRNA_401955 play a key role in EC. As the stability and sensibility of circRNAs, they may be as potential biomarkers of therapeutic targets for the treatment EC, while there still some are the challenges in the clinical application of circRNAs such as the large-scale production and preparation technology, delivery technology.
Conclusions
circRNA dysregulation is involved in EC tumorigenesis. Hsa_circRNA_401955 was found to be the hub circRNA, and 4 primary pathways were predicted to occur in this hub circRNA-miRNA-mRNA network. Hsa_circRNA_100375 and its associated MRE axis are involved in a cancer-related pathway. Several key circRNAs and proteins may serve as potential biomarkers and therapeutic targets for EC. Furthermore, our findings improve our understanding of the EC tumorigenesis mechanism. However, this study is limited by the relatively small sample size. Our results showed that circRNAs have an effect on EC tumorigenesis, but the precise mechanism remains to be elucidated.
Acknowledgments
Funding: This work was supported by the grants from the Technical NewStar of Zhujiang, Pan Yu District, Guangzhou (No. 2013-special-15-6.10) and the Science and Technology Program of Guangzhou (No. 201804010012).
Footnote
Reporting Checklist: The authors have completed the MDAR reporting checklist. Available at https://jgo.amegroups.com/article/view/10.21037/jgo-22-137/rc
Data Sharing Statement: Available at https://jgo.amegroups.com/article/view/10.21037/jgo-22-137/dss
Conflicts of Interest: All authors have completed the ICMJE uniform disclosure form (available at https://jgo.amegroups.com/article/view/10.21037/jgo-22-137/coif). The authors have no conflicts of interest to declare.
Ethical Statement: The authors are accountable for all aspects of the work in ensuring that questions related to the accuracy or integrity of any part of the work are appropriately investigated and resolved. The study was conducted in accordance with the Declaration of Helsinki (as revised in 2013). The study was approved by institutional ethics board of Panyu Central Hospital (No. K20170003) and informed consent was taken from all the patients. The Third Affiliated Hospital of Southern Medical University is also informed and agreed with the study.
Open Access Statement: This is an Open Access article distributed in accordance with the Creative Commons Attribution-NonCommercial-NoDerivs 4.0 International License (CC BY-NC-ND 4.0), which permits the non-commercial replication and distribution of the article with the strict proviso that no changes or edits are made and the original work is properly cited (including links to both the formal publication through the relevant DOI and the license). See: https://creativecommons.org/licenses/by-nc-nd/4.0/.
References
- Siegel RL, Miller KD, Jemal A. Cancer Statistics, 2017. CA Cancer J Clin 2017;67:7-30. [Crossref] [PubMed]
- Hikami S, Shiozaki A, Fujiwara H, et al. Outcome of Patients with Pathological Complete Response after Neoadjuvant Chemotherapy in Advanced Esophageal Cancer. Gan To Kagaku Ryoho 2017;44:1796-8. [PubMed]
- Saddoughi SA, Reinersman JM, Zhukov YO, et al. Survival After Surgical Resection of Stage IV Esophageal Cancer. Ann Thorac Surg 2017;103:261-6. [Crossref] [PubMed]
- Zhang Y, Xue W, Li X, et al. The Biogenesis of Nascent Circular RNAs. Cell Rep 2016;15:611-24. [Crossref] [PubMed]
- Liang D, Wilusz JE. Short intronic repeat sequences facilitate circular RNA production. Genes Dev 2014;28:2233-47. [Crossref] [PubMed]
- Greene J, Baird AM, Brady L, et al. Circular RNAs: Biogenesis, Function and Role in Human Diseases. Front Mol Biosci 2017;4:38. [Crossref] [PubMed]
- Beermann J, Piccoli MT, Viereck J, et al. Non-coding RNAs in Development and Disease: Background, Mechanisms, and Therapeutic Approaches. Physiol Rev 2016;96:1297-325. [Crossref] [PubMed]
- Zhang HD, Jiang LH, Sun DW, et al. CircRNA: a novel type of biomarker for cancer. Breast Cancer 2018;25:1-7. [Crossref] [PubMed]
- Meng S, Zhou H, Feng Z, et al. CircRNA: functions and properties of a novel potential biomarker for cancer. Mol Cancer 2017;16:94. [Crossref] [PubMed]
- Guo XY, He CX, Wang YQ, et al. Circular RNA Profiling and Bioinformatic Modeling Identify Its Regulatory Role in Hepatic Steatosis. Biomed Res Int 2017;2017:5936171. [Crossref] [PubMed]
- Miao R, Wang Y, Wan J, et al. Microarray expression profile of circular RNAs in chronic thromboembolic pulmonary hypertension. Medicine (Baltimore) 2017;96:e7354. [Crossref] [PubMed]
- Rong D, Sun H, Li Z, et al. An emerging function of circRNA-miRNAs-mRNA axis in human diseases. Oncotarget 2017;8:73271-81. [Crossref] [PubMed]
- Bure IV, Nemtsova MV. Methylation and Noncoding RNAs in Gastric Cancer: Everything Is Connected. Int J Mol Sci 2021;22:5683. [Crossref] [PubMed]
- Yi YC, Chen XY, Zhang J, et al. Novel insights into the interplay between m6A modification and noncoding RNAs in cancer. Mol Cancer 2020;19:121. [Crossref] [PubMed]
- Liang HF, Zhang XZ, Liu BG, et al. Circular RNA circ-ABCB10 promotes breast cancer proliferation and progression through sponging miR-1271. Am J Cancer Res 2017;7:1566-76. [PubMed]
- Zhong Z, Huang M, Lv M, et al. Circular RNA MYLK as a competing endogenous RNA promotes bladder cancer progression through modulating VEGFA/VEGFR2 signaling pathway. Cancer Lett 2017;403:305-17. [Crossref] [PubMed]
- Zhang P, Wu W, Chen Q, et al. Non-Coding RNAs and their Integrated Networks. J Integr Bioinform 2019;16:20190027. [Crossref] [PubMed]
- Liu YC, Li JR, Sun CH, et al. CircNet: a database of circular RNAs derived from transcriptome sequencing data. Nucleic Acids Res 2016;44:D209-15. [Crossref] [PubMed]
- Ghosal S, Das S, Sen R, et al. Circ2Traits: a comprehensive database for circular RNA potentially associated with disease and traits. Front Genet 2013;4:283. [Crossref] [PubMed]
- Enright AJ, John B, Gaul U, et al. MicroRNA targets in Drosophila. Genome Biol 2003;5:R1. [Crossref] [PubMed]
- Pasquinelli AE. MicroRNAs and their targets: recognition, regulation and an emerging reciprocal relationship. Nat Rev Genet 2012;13:271-82. [Crossref] [PubMed]
- Vlachos IS, Zagganas K, Paraskevopoulou MD, et al. DIANA-miRPath v3.0: deciphering microRNA function with experimental support. Nucleic Acids Res 2015;43:W460-6. [Crossref] [PubMed]
- Jeck WR, Sorrentino JA, Wang K, et al. Circular RNAs are abundant, conserved, and associated with ALU repeats. RNA 2013;19:141-57. [Crossref] [PubMed]
- Sanger HL, Klotz G, Riesner D, et al. Viroids are single-stranded covalently closed circular RNA molecules existing as highly base-paired rod-like structures. Proc Natl Acad Sci U S A 1976;73:3852-6. [Crossref] [PubMed]
- Memczak S, Jens M, Elefsinioti A, et al. Circular RNAs are a large class of animal RNAs with regulatory potency. Nature 2013;495:333-8. [Crossref] [PubMed]
- Huang YS, Jie N, Zou KJ, Weng Y. Expression profile of circular RNAs in human gastric cancer tissues. Mol Med Rep 2017;16:2469-76. [Crossref] [PubMed]
- Huang G, Li S, Yang N, et al. Recent progress in circular RNAs in human cancers. Cancer Lett 2017;404:8-18. [Crossref] [PubMed]
- Kumar L. Circular RNAs: the Emerging Class of Non-coding RNAs and Their Potential Role in Human Neurodegenerative Diseases. Mol Neurobiol 2017;54:7224-34. [Crossref] [PubMed]
- Burd CE, Jeck WR, Liu Y, et al. Expression of linear and novel circular forms of an INK4/ARF-associated non-coding RNA correlates with atherosclerosis risk. PLoS Genet 2010;6:e1001233. [Crossref] [PubMed]
- Zhang XO, Wang HB, Zhang Y, et al. Complementary sequence-mediated exon circularization. Cell 2014;159:134-47. [Crossref] [PubMed]
- Li Z, Huang C, Bao C, et al. Exon-intron circular RNAs regulate transcription in the nucleus. Nat Struct Mol Biol 2015;22:256-64. [Crossref] [PubMed]
- Schwanhäusser B, Busse D, Li N, et al. Global quantification of mammalian gene expression control. Nature 2011;473:337-42. Erratum in: Nature 2013;495:126-7. [Crossref] [PubMed]
- Hansen TB, Jensen TI, Clausen BH, et al. Natural RNA circles function as efficient microRNA sponges. Nature 2013;495:384-8. [Crossref] [PubMed]
- Hansen TB, Wiklund ED, Bramsen JB, et al. miRNA-dependent gene silencing involving Ago2-mediated cleavage of a circular antisense RNA. EMBO J 2011;30:4414-22. [Crossref] [PubMed]
- Peng L, Yuan XQ, Li GC. The emerging landscape of circular RNA ciRS-7 in cancer Oncol Rep 2015;33:2669-74. (Review). [Crossref] [PubMed]
- Pan H, Li T, Jiang Y, et al. Overexpression of Circular RNA ciRS-7 Abrogates the Tumor Suppressive Effect of miR-7 on Gastric Cancer via PTEN/PI3K/AKT Signaling Pathway. J Cell Biochem 2018;119:440-6. [Crossref] [PubMed]
- Zhao Y, Alexandrov PN, Jaber V, et al. Deficiency in the Ubiquitin Conjugating Enzyme UBE2A in Alzheimer’s Disease (AD) is Linked to Deficits in a Natural Circular miRNA-7 Sponge (circRNA; ciRS-7). Genes (Basel) 2016;7:116. [Crossref] [PubMed]
- Requena T, Cabrera S, Martín-Sierra C, et al. Identification of two novel mutations in FAM136A and DTNA genes in autosomal-dominant familial Meniere’s disease . Hum Mol Genet 2015;24:1119-26. [Crossref] [PubMed]
- Zlotina A, Nikulina T, Yany N, et al. Ring chromosome 18 in combination with 18q12.1 (DTNA) interstitial microdeletion in a patient with multiple congenital defects. Mol Cytogenet 2016;9:18. [Crossref] [PubMed]
- Wang L, Zhu MJ, Ren AM, et al. A ten-microRNA signature identified from a genome-wide microRNA expression profiling in human epithelial ovarian cancer. PLoS One 2014;9:e96472. [Crossref] [PubMed]
- Tang J, Zhuo H, Zhang X, et al. A novel biomarker Linc00974 interacting with KRT19 promotes proliferation and metastasis in hepatocellular carcinoma. Cell Death Dis 2014;5:e1549. [Crossref] [PubMed]
- Motieghader H, Kouhsar M, Najafi A, et al. mRNA-miRNA bipartite network reconstruction to predict prognostic module biomarkers in colorectal cancer stage differentiation. Mol Biosyst 2017;13:2168-80. [Crossref] [PubMed]
- Tao L, Zeng Y, Wang J, et al. Differential microRNA expression in aristolochic acid-induced upper urothelial tract cancers ex vivo. Mol Med Rep 2015;12:6533-46. [Crossref] [PubMed]
- Anandaram H. A computational approach to identify microRNA (miRNA) based biomarker from the regulation of disease pathology. Biol Med Case Rep 2018;2:12-25. [Crossref]
- Fujiwara T, Yada T. miRNA-target prediction based on transcriptional regulation. BMC Genomics 2013;14:S3. [Crossref] [PubMed]
- Liu B, Li J, Cairns MJ. Identifying miRNAs, targets and functions. Brief Bioinform 2014;15:1-19. [Crossref] [PubMed]
- Guo L, Zhao Y, Yang S, et al. Integrative analysis of miRNA-mRNA and miRNA-miRNA interactions. Biomed Res Int 2014;2014:907420. [Crossref] [PubMed]
- Bindea G, Galon J, Mlecnik B. CluePedia Cytoscape plugin: pathway insights using integrated experimental and in silico data. Bioinformatics 2013;29:661-3. [Crossref] [PubMed]
- Malami I, Abdul AB, Abdullah R, et al. In Silico Discovery of Potential Uridine-Cytidine Kinase 2 Inhibitors from the Rhizome of Alpinia mutica. Molecules 2016;21:417. [Crossref] [PubMed]
- Petillo D, Kort EJ, Anema J, et al. MicroRNA profiling of human kidney cancer subtypes. Int J Oncol 2009;35:109-14. [Crossref] [PubMed]
- Lu ZM, Lin YF, Jiang L, et al. Micro-ribonucleic acid expression profiling and bioinformatic target gene analyses in laryngeal carcinoma. Onco Targets Ther 2014;7:525-33. [Crossref] [PubMed]
- Chang KH, Miller N, Kheirelseid EA, et al. MicroRNA signature analysis in colorectal cancer: identification of expression profiles in stage II tumors associated with aggressive disease. Int J Colorectal Dis 2011;26:1415-22. [Crossref] [PubMed]
- Jin C, Yan B, Lu Q, et al. Reciprocal regulation of Hsa-miR-1 and long noncoding RNA MALAT1 promotes triple-negative breast cancer development. Tumour Biol 2016;37:7383-94. [Crossref] [PubMed]
(English Language Editor: C. Mullens)