Identification of potential prognostic biomarkers for hepatocellular carcinoma
Introduction
Liver cancer is a common malignant tumor of the digestive tract and the Hepatocellular carcinoma (HCC) is one of the most common type of primary liver cancer (1). At present, there are multiple treatment modalities for HCC, among which liver transplantation, tumor resection, chemoembolization, ablation, etc. are widely utilized (2). However, the curative effects of these methods differ, and the prognosis of patients is poor. Therefore, exploring the molecular mechanism of HCC from a genetic perspective and finding new tumor markers are helpful for early diagnosis as well as the development of highly accurate targeted therapy and preventive treatment. Studying the pathogenesis and molecular mechanism has always been a hotspot in HCC research. Based on genomics research, scholars have studied the key genome changes in HCC by using The Cancer Genome Atlas (TCGA) and Gene Expression Omnibus (GEO) online datasets (3,4). At present, most investigations on potential prognostic biomarkers for hepatocellular carcinoma from TCGA are single or commination studies. In this study, we analyzed associations between HCC oncogenes expression and clinical prognosis of HCC patients, aim to find novel biomarkers and therapeutic target genes for HCC.
With the evolution of high-throughput sequencing technology, characterization of gene expression signature has been widely used in the study cancer researches, with this technique we can efficiently and inefficiently identify thess clinical biomarkers and therapeutic targets. Weighted gene co-expression network analysis (WGCNA) algorithm is a novel biological instrument used to analyze correlated gene expression patterns and key genes in samples. It can construct a co-expression network to identify clusters of genes with similar expression patterns for the investigation of clinical traits (5). In this research, WGCNA was performed to identify the gene modules that are correlated with HCC. Furthermore, gene function enrichment analysis and correlation analysis were conducted to investigate the mechanism of HCC progression and prognosis. We present the following article in accordance with the STREGA reporting checklist (available at https://jgo.amegroups.com/article/view/10.21037/jgo-22-303/rc).
Methods
Data collection
The gene expression data of HCC was downloaded from TCGA (http://portal.gdc.cancer.gov), and the DEGs of HCC and normal tissues in GSE60502 dataset was downloaded from the GEO database (https://www.ncbi.nlm.nih.gov/geo). The study was conducted in accordance with the Declaration of Helsinki (as revised in 2013).
Weighted gene co-expression network analysis (WGCNA)
WGCNA can be used to detect the co-expression gene modules. The pickSoftThreshold function was used to to calculate the soft threshold power β. The connectivity threshold power β was set to 3. A cluster tree was then generated to classify the gene modules. According to the dissimilarity measure of the topological overlap matrix (TOM), the gene modules were generated by average linkage hierarchical clustering. Each branch and color represented a different gene module. Pearson’s correlation analysis was performed to analyze the interaction of these modules and the clinical traits.
Differentially-expressed genes (DEGs) identification
An in-depth analysis the DEGs in HCC, we used the edgeR package in Bioconductor (http://bioconductor.org/) with P<0.05 & |log fold change (FC)| >1. Then a Venn diagram wa constructed to identify overlapping hub module target genes among different groups.
Gene function enrichment analysis
To identify the characteristic biological and functional properties of all DEGs, the sequences were mapped using the GO database. To identify candidate biomarkers in this process, we performed Gene Ontology analysis. Functional attributes was performed using the Kyoto Encyclopedia of Genes and Genomes (KEGG) enrichment analysis.
Protein-protein interaction (PPI) network analysis
The online Search Tool for the Retrieval of Interacting Genes (STRING) database (https://string-db.org/) and cytoHubba (version 0.1, https://cytoscape.org/), a cytoscape plugin were performed to construct a PPI network of the interaction between DEGs with criteria were confidence score >0.7. The top 10 genes were screened out as the hub genes by using the Maximal Clique Centrality (MCC) algorithm.
OS analysis
We chose the hub genes within the significant modules to validate their usage for the prediction of survival information. Next, we used the “survival” R package to calculate the correlation between each module and overall survival (OS), with a value of P<0.05 was considered as statistically significant. In addition, the prognostic value of the hub genes was analyzed by univariate and multivariate Cox regression, with a value of P<0.05 was treated as statistically significant.
Correlation analysis between clinical features and hub genes expression
The correlation between clinical features and hub genes by using the “cor” package in R (http://bioconductor.org/). In the present study, clinical features were downloaded from TCGA database. P<0.05 was considered as statistically significant.
Statistical analysis
All statistical analyses were performed using R software version 3.4.3. WGCNA were performed by using “WGCNA” package. P<0.05 was considered as statistically significant difference.
Results
Identification of significant gene modules
A WGCNA network was initially constructed using the HCC data from TCGA online database, the connectivity threshold power β was set to 3 to define the adjacency matrix based on calculated by applying the pickSoftThreshold function in WGCNA (Figure 1A). Totally of 12 co-expressed modules and hub genes were clustered, among which the black module (422 genes) was related to HCC (R=0.84, P<0.05, Figure 1B,1C). After correlation analysis, the results showed that black module was strong correlation with the module-related genes (R=0.96, Figure 1D, P<0.05).
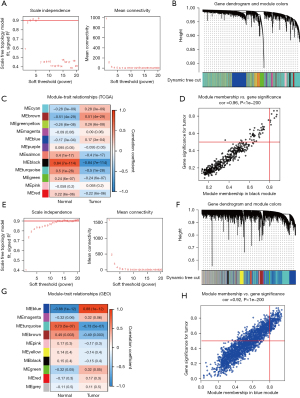
Similarly, WGCNA was conducted using the GSE60502 dataset from the GEO database. The scale-free network was constructed when β=13 (Figure 1E), and there were 10 co-expressed gene modules clustered (Figure 1F,1G). The results showed that the blue module (2,181 genes) exhibited the strongest correlation to HCC (R=0.88, P<0.05, Figure 1G). After correlation analysis, the results showed that blue module was strong correlation with the module-related genes (R=0.92, Figure 1H, P<0.05).
DEGs identification
Next, we used the “edgeR” package to screen out the DEGs in both TCGA and the GSE60502 dataset. In TCGA database, the volcano plot showed that 773 DEGs were obtained by applying a False Discovery Rate (FDR) value <0.05 and |logFC| >1 (Figure 2A), including 79 up-regulated genes and 694 down-regulated genes. In the GSE60502 dataset, 1,052 DEGs were screened of which 485 were up-regulated and 567 were down-regulated (Figure 2B). After compared analysis between brown and blue modules with the DEGs, we obtained 27 overlap genes (Figure 2C).
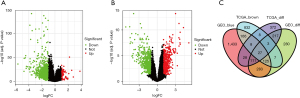
GO and KEGG analysis
We performed GO and KEGG analyses to investigate the potential function of the 27 shared genes (Figure 3A,3B). In biological process (BP), these shared genes were significantly enriched in mitotic nuclear division. As for cellular component (CC), chromosomal region was mainly enriched. Regarding molecular function (MF), tubulin binding was mainly enriched. Besides, the top three enriched KEGG pathways were “cell cycle”, “oocyte meiosis”, and the “p53 signaling pathway” (Figure 3B). The GO and KEGG results showed that these shared genes may affect the progression of HCC by regulating the cancer cell proliferation,
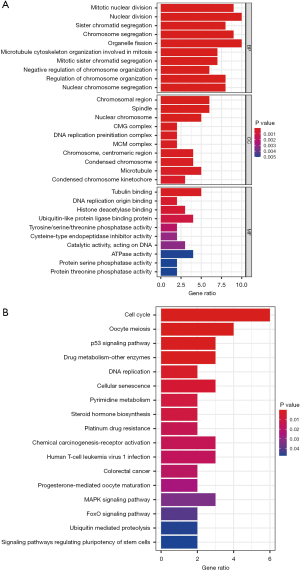
Since most of the mainly enriched GO and KEGG terms were involved in cell proliferation, we assumed that the 27 shared genes affected the progression of HCC by regulating the biological behavior of cancer cells.
Hub genes identification
String analysis was performed to identify the hub genes related to HCC progression (Figure 4A). According to the MCC algorithm, the top 10 candidate hub genes were MYC, MCM3, CDC20, CCNB1, BIRC5, UBE2C, TOP2A, RRM2, TK1, and PTTG1. Subsequently, Kaplan-Meier (KM) curves were drawn to analysis the correlation between vital genes and overall survival (OS) in HCC. Based on the KM curves, BIRC5, CDC20, and UBE2C showed a strong correlation with OS (Figure 4B-4D). Therefore, BIRC5, CDC20, and UBE2C were screened out as hub genes for further analysis.
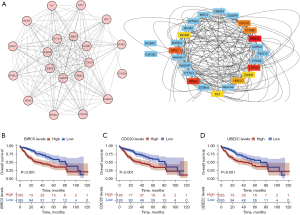
Prognostic value analysis of hub genes
Next, we analyzed the relationship between the expression of these hub genes and clinical features (age, stage, TNM categories) to explore their prognostic value, and the three hub genes showed similar results. As shown in Figure 5, the expression level of these three hub genes in patients younger than 65 years was higher than that in patients older than 65 years. Also, compared to stage I, the expression level of hub genes was significantly increased in stages II and III. As for T category, compared to T1, patients exhibited a higher expression level of hub genes in T2, T3, and T4. Taken together, the expression of hub genes was decreasingly associated with age, while increasingly associated with the HCC stages and T category.
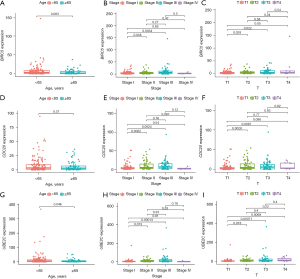
Discussion
In this study, we detected 12 co-expressed gene modules in TCGA database and 10 co-expressed gene modules in the GSE60502 dataset, among which brown and blue modules were most strongly associated with HCC, and the Venn diagram results showed that there were 27 shared genes. Furthermore, in biological process (BP), theses shared genes were significantly enriched in mitotic nuclear division, chromosomal region, and tubulin binding. KEGG analysis revealed that the 27 shared genes were significantly enriched in the cell cycle, oocyte meiosis, and the p53 signaling pathway.
Several previous studies have confirmed that these enriched GO and KEGG terms play critical roles in the progression of HCC (6,7). Obviously, abnormality during mitotic nuclear division is a crucial reason for the predisposition and progression of cancers such as HCC. It has been shown that mitotic errors in HCC progression will lead to Chk2 activation, which causes lagging chromosome/Deoxyribonucleic acid (DNA) damage (8). The genetic polymorphism site located in specific chromosomal regions, such as chromosome 6p21.3 and 6p21.33, is closely correlated to the susceptibility of HCC (9,10).
Microtubules, composed of tubulin, are widely prevalent in dividing cells, and have significant regulatory effects on mitosis, cytoskeletal shape, cell motility, intracellular protein, and organelle transport (11). Tubulin binding agent has been reported as a novel drug, which can inhibit mitosis, cell growth, migration, and vascularization (12,13). P53 is a famous tumor suppressor gene, which plays crucial roles in the cell cycle, apoptosis, and maintenance of genomic stability. P53 mutations have been observed in HCC pathogenesis (14,15). MDM2 negatively regulates p53 activity by inducing p53 protein degradation. Thus, the mechanism of steady state maintenance of the MDM2-p53 axis is an important factor in the initiation and progression of HCC (16,17).
Based on PPI network analysis and the MCC algorithm, we screened out 10 potential candidate hub genes. Subsequently, three hub genes (BIRC5, CDC20 and UBE2) were identified according to the KM curves, which were strongly correlated to the OS of HCC patients. Furthermore, the expression level of these hub genes was elevated in patients younger than 65 years than that in patients over the age of 65 years. However, the hub genes showed a positive correlation with the stages and T category of HCC. As for N and M categories, there was no significant correlation with the hub genes.
BIRC5, also known as Survivin, it lies at crossroads of a series of tumors cell signaling networks, particularly some upstream cellular signaling molecules regulate surviving and its functions, the survivin has been reported to affect the proliferation and division of tumor cells by regulating apoptosis during the progression of several cancers (18,19). It has been demonstrated that co-suppression of OCT4 and BIRC5 can efficiently inhibit the proliferative activity of cancer cells by inducing apoptosis and cell cycle arrest (19). Xu et al. revealed that highly-expressed BIRC5 is strongly correlated with a poor prognosis in HCC (20). Treatment targeting BIRC5 has been recognized as a potential therapy for HCC.
CDC20 (cell division cycle 20), a cell-cycle checkpoint control factor was first discovered by Lee Hartwell 40 years ago. It has been revealed that APC can be activated by the substrate-recruiting module CDC20, and the CDC20 can promote cancer progression as a carcinogen, the overexpression of CDC20 is correlated with the poor prognosis of several cancers (21-23). Compared with adjacent non-cancerous specimens, the expression of CDC20 in HCC specimens is increased (24). Consistent with our findings, Li et al. found that CDC20 is also positively correlated with TNM stages in HCC (25). De-activation of CDC20 may be effective in the treatment of HCC, and further researches should pay more attention to CDC20 inhibitors.
UBE2C is one of the genes which used in molecular classification in many types of tumors, the expression level of UBE2C (Ubiquitin-conjugating enzyme E2C) is related to many types of solid tumors; however, UBE2C is nearly undetectable in normal tissues (26,27). Overexpression of UBE2C is associated with enhanced proliferation, migration, and invasion (28,29). Xiong et al. has demonstrated that UBE2C knockdown can attenuate the proliferation and invasion of HCC cells (30). A growing body of evidence has revealed that UBE2C can be identified as a potential candidate biomarker to predict the prognosis of HCC.
Taken together, combining the results of previous studies with our findings, we detected three hub genes (BIRC5, CDC20, and UBE2C), which were strongly correlated with the progression and prognosis of HCC, and targeting these hub genes may provide new therapeutics for HCC.
Acknowledgments
Funding: The present study was supported by Tianjin Health Science and Technology Project (No. TJWJ2021QN059).
Footnote
Reporting Checklist: The authors have completed the STREGA reporting checklist. (available at https://jgo.amegroups.com/article/view/10.21037/jgo-22-303/rc).
Conflicts of Interest: All authors have completed the ICMJE uniform disclosure form (available at https://jgo.amegroups.com/article/view/10.21037/jgo-22-303/coif). The authors have no conflicts of interest to declare.
Ethical Statement: The authors are accountable for all aspects of the work in ensuring that questions related to the accuracy or integrity of any part of the work are appropriately investigated and resolved. The study was conducted in accordance with the Declaration of Helsinki (as revised in 2013).
Open Access Statement: This is an Open Access article distributed in accordance with the Creative Commons Attribution-NonCommercial-NoDerivs 4.0 International License (CC BY-NC-ND 4.0), which permits the non-commercial replication and distribution of the article with the strict proviso that no changes or edits are made and the original work is properly cited (including links to both the formal publication through the relevant DOI and the license). See: https://creativecommons.org/licenses/by-nc-nd/4.0/.
References
- Llovet JM, Kelley RK, Villanueva A, et al. Hepatocellular carcinoma. Nat Rev Dis Primers 2021;7:6. [Crossref] [PubMed]
- Anwanwan D, Singh SK, Singh S, et al. Challenges in liver cancer and possible treatment approaches. Biochim Biophys Acta Rev Cancer 2020;1873:188314. [Crossref] [PubMed]
- Tomczak K, Czerwińska P, Wiznerowicz M. The Cancer Genome Atlas (TCGA): an immeasurable source of knowledge. Contemp Oncol (Pozn) 2015;19:A68-77. [Crossref] [PubMed]
- Edgar R, Domrachev M, Lash AE. Gene Expression Omnibus: NCBI gene expression and hybridization array data repository. Nucleic Acids Res 2002;30:207-10. [Crossref] [PubMed]
- Langfelder P, Horvath S. WGCNA: an R package for weighted correlation network analysis. BMC Bioinformatics 2008;9:559. [Crossref] [PubMed]
- Song X, Du R, Gui H, et al. Identification of potential hub genes related to the progression and prognosis of hepatocellular carcinoma through integrated bioinformatics analysis. Oncol Rep 2020;43:133-46. [PubMed]
- Cao J, Zhang C, Jiang GQ, et al. Identification of hepatocellular carcinoma-related genes associated with macrophage differentiation based on bioinformatics analyses. Bioengineered 2021;12:296-309. [Crossref] [PubMed]
- Carloni V, Lulli M, Madiai S, et al. CHK2 overexpression and mislocalisation within mitotic structures enhances chromosomal instability and hepatocellular carcinoma progression. Gut 2018;67:348-61. [Crossref] [PubMed]
- Coelho AV, Moura RR, Crovella S, et al. HLA-G genetic variants and hepatocellular carcinoma: a meta-analysis. Genet Mol Res 2016; [Crossref] [PubMed]
- Wang H, Wang B, Wang T, et al. A genetic variant in the promoter region of miR-877 is associated with an increased risk of hepatocellular carcinoma. Clin Res Hepatol Gastroenterol 2020;44:692-8. [Crossref] [PubMed]
- Loong HH, Yeo W. Microtubule-targeting agents in oncology and therapeutic potential in hepatocellular carcinoma. Onco Targets Ther 2014;7:575-85. [PubMed]
- Bennani YL, Gu W, Canales A, et al. Tubulin binding, protein-bound conformation in solution, and antimitotic cellular profiling of noscapine and its derivatives. J Med Chem 2012;55:1920-5. [Crossref] [PubMed]
- Moser C, Lang SA, Mori A, et al. ENMD-1198, a novel tubulin-binding agent reduces HIF-1alpha and STAT3 activity in human hepatocellular carcinoma(HCC) cells, and inhibits growth and vascularization in vivo. BMC Cancer 2008;8:206. [Crossref] [PubMed]
- Azer SA. MDM2-p53 Interactions in Human Hepatocellular Carcinoma: What Is the Role of Nutlins and New Therapeutic Options? J Clin Med 2018;7:64. [Crossref] [PubMed]
- Amaral JD, Castro RE, Steer CJ, et al. p53 and the regulation of hepatocyte apoptosis: implications for disease pathogenesis. Trends Mol Med 2009;15:531-41. [Crossref] [PubMed]
- Cao H, Chen X, Wang Z, et al. The role of MDM2-p53 axis dysfunction in the hepatocellular carcinoma transformation. Cell Death Discov 2020;6:53. [Crossref] [PubMed]
- Wang L, Huang J, Jiang M, et al. Survivin (BIRC5) cell cycle computational network in human no-tumor hepatitis/cirrhosis and hepatocellular carcinoma transformation. J Cell Biochem 2011;112:1286-94. [Crossref] [PubMed]
- Yamamoto H, Ngan CY, Monden M. Cancer cells survive with survivin. Cancer Sci 2008;99:1709-14. [Crossref] [PubMed]
- Cao L, Li C, Shen S, et al. OCT4 increases BIRC5 and CCND1 expression and promotes cancer progression in hepatocellular carcinoma. BMC Cancer 2013;13:82. [Crossref] [PubMed]
- Xu R, Lin L, Zhang B, et al. Identification of prognostic markers for hepatocellular carcinoma based on the epithelial-mesenchymal transition-related gene BIRC5. BMC Cancer 2021;21:687. [Crossref] [PubMed]
- Zhuang L, Yang Z, Meng Z. Upregulation of BUB1B, CCNB1, CDC7, CDC20, and MCM3 in Tumor Tissues Predicted Worse Overall Survival and Disease-Free Survival in Hepatocellular Carcinoma Patients. Biomed Res Int 2018;2018:7897346. [Crossref] [PubMed]
- Wu WJ, Hu KS, Wang DS, et al. CDC20 overexpression predicts a poor prognosis for patients with colorectal cancer. J Transl Med 2013;11:142. [Crossref] [PubMed]
- Zhang Q, Huang H, Liu A, et al. Cell division cycle 20 (CDC20) drives prostate cancer progression via stabilization of β-catenin in cancer stem-like cells. EBioMedicine 2019;42:397-407. [Crossref] [PubMed]
- Zhang X, Zhang X, Li X, et al. Connection Between CDC20 Expression and Hepatocellular Carcinoma Prognosis. Med Sci Monit 2021;27:e926760. [Crossref] [PubMed]
- Li J, Gao JZ, Du JL, et al. Increased CDC20 expression is associated with development and progression of hepatocellular carcinoma. Int J Oncol 2014;45:1547-55. [Crossref] [PubMed]
- Guo J, Wu Y, Du J, et al. Deregulation of UBE2C-mediated autophagy repression aggravates NSCLC progression. Oncogenesis 2018;7:49. [Crossref] [PubMed]
- Li L, Li X, Wang W, et al. UBE2C is involved in the functions of ECRG4 on esophageal squamous cell carcinoma. Biomed Pharmacother 2018;98:201-6. [Crossref] [PubMed]
- Wang R, Song Y, Liu X, et al. UBE2C induces EMT through Wnt/β-catenin and PI3K/Akt signaling pathways by regulating phosphorylation levels of Aurora-A. Int J Oncol 2017;50:1116-26. [Crossref] [PubMed]
- Zhang Y, Tian S, Li X, et al. UBE2C promotes rectal carcinoma via miR-381. Cancer Biol Ther 2018;19:230-8. [Crossref] [PubMed]
- Xiong Y, Lu J, Fang Q, et al. UBE2C functions as a potential oncogene by enhancing cell proliferation, migration, invasion, and drug resistance in hepatocellular carcinoma cells. Biosci Rep 2019;39:BSR20182384. [Crossref] [PubMed]