Identification of a chemotherapy-associated gene signature for a risk model of prognosis in gastric adenocarcinoma through bioinformatics analysis
Introduction
Digestive tract-associated tumours account for over half of the morbidity and mortality of all tumours, among which gastric adenocarcinoma (GA) is frequently seen worldwide (1). Thanks to continuous improvement in early diagnosis and surgical methods, and the application of chemotherapy (2), radiotherapy and biological agents (3), the overall level of GA treatment has achieved a significant improvement over the past few years (4). Surgery is still the leading treatment, including distal gastrectomy (5). For a long time, due to the late diagnosis of GA, the surgical outcome was poor, with a postoperative 5-year survival rate around 30% (6). Compared with non-screening or imaging, endoscopic screening can lower the risk of death from GA, and will not affect the incidence in Asian countries (7).
In addition to surgery, neoadjuvant chemotherapy (NACT) is an important therapy for digestive tract-associated tumours (8). And palliative treatment is the standard treatment for incurable advanced GA (9). A meta-analysis of individual patient data and a Cochrane systematic review verified the benefits of NACT (10), and 5-year follow-up data confirmed that NACT with S-1 after operation can prolong the overall survival (OS) as well as recurrence-free survival (RFS) of patients with stage II or III GA who underwent D2 gastrectomy (11). Moreover, 3-year follow-up data confirmed that postoperative NACT of S-1 combined with docetaxel can improve RFS and OS, and can be recommended as the standard for patients with stage III GA treated with D2 sandwich therapy (12). However, there is no consensus on which chemotherapy regimen is most effective in improving the OS and disease-free survival rates (13). Therefore, risk models based on genetics, diet, nutrition, living habits or occupational characteristics are being widely used in the prediction and prognosis of GA.
A large number of studies have identified a series of risk models that can be used to forecast GA patients’ prognosis through bioinformatics methods. For instance, Zhou et al. (14) constructed a 4-genes-based prognosis prediction model by analysing and screening the differentially expressed genes (DEGs) of normal and GA tissues in The Cancer Genome Atlas (TCGA) as well as the Gene Expression Omnibus (GEO) and confirmed the validity of the model by data sets. However, the clinical heterogeneity of gastric cancer is mainly reflected in the inequality of treatment results. Its molecular mechanism remains unclear, including its molecular subtypes and related biomarkers have not been established, which cannot improve the prognosis and treatment of gastric cancer. Thus, in the presented study, we analyzed gene expression profiling data from gastric cancer patients through microarray technologies and uncovered potential prognostic subtypes, thereby identified the gene expression signature associated with prognosis and adjuvant chemotherapy response of gastric cancer. Meanwhile, the construction of a prognosis prediction model related to chemotherapy for GA has not been reported. Accordingly, we identified potential key chemotherapy-related genes of GA by bioinformatics methods, evaluated them and constructed a corresponding risk assessment model of GA and verified its prognostic value, with the goal of providing a reference for the treatment and improvement of prognosis of GA patients. We present the following article in accordance with the TRIPOD reporting checklist (available at https://jgo.amegroups.com/article/view/10.21037/jgo-22-872/rc).
Methods
Data source
From TCGA (https://portal.gdc.cancer.gov/), the clinicopathological parameters of stomach adenocarcinoma (STAD) samples and the RNA-seq of tumour tissue samples were downloaded. According to the integrity of the clinical sample data, as well as the matching with sequencing samples, duplicate and deleted samples and cases with missing clinical outcomes were screened. A total of 360 cancer tissue samples and 50 matched normal tissue samples were obtained. The GSE26901 data set was set as training set, downloaded from GEO (15), and was grouped according to the treatment with chemotherapy into 44 chemotherapy samples and 65 non-chemotherapy samples. In addition, the GSE84437 data set was downloaded as the verification set (16), and finally clinical phenotypic data on survival time and survival status of 110 tumour samples were obtained. The microarray data were normalized using the quantile normalization method in the Linear Models for Microarray Data package in the R language environment. The expression level of each gene was transformed into a log2 base before further analysis. The study was conducted in accordance with the Declaration of Helsinki (as revised in 2013).
DEG analysis
The gene expression matrix was analysed by limma software package in R software. Using the Benjamini-Hochberg method, the P value was adjusted to the false discovery rate (FDR). With FDR <0.05 and |log2(Fold Change) |> log2(1.2) as the standard, DEGs were screened out. Using the R package ggplot2, a volcano map was drawn, and a Venn map revealed the overlapping genes.
Key genes screening and prognosis model construction
The survival package in the R software was used for univariate Cox regression analysis, with P<0.05 as the screening criterion. With the survminer package, the key genes were assigned to high or low expression groups based on the optimal cut-off value, and the corresponding survival curve was drawn. The least absolute shrinkage and selection operator (LASSO) regression algorithm was used to select the characteristics of key genes, and 10-fold cross-validation for determining the parameters. Using the Akaike Information Criterion (AIC), the selected genes underwent stepwise regression analysis for constructing a prognosis model. Kaplan-Meier (K-M) survival curves of the high- and low-risk groups were drawn by the survival package, and the timeROC package was utilised for drawing corresponding receiver operating characteristic (ROC) curves. The accuracy of the model in predicting prognosis was verified internally and externally.
Univariate and multivariate Cox regression analyses
Cox regression analysis was performed with the survival package, and a forest map was drawn by the forestplot package to report the P value, hazard ratio (HR) and 95% confidence interval (CI) of every variable. Based on the multivariate Cox proportional risk analysis, the RMS package was used to construct a nomogram for predicting the 1-, 2- and 3-year survival rates.
Immune score evaluation
The TIMER database (http://timer.comp-genomics.org/) was used to analyse the correlations of the expression of key genes in STAD with immune cell infiltration levels and immune cell markers. Using the CIBERSORT algorithm, the immune score was evaluated, and the correlation of key genes with immune checkpoint genes was analysed (17). The ESTIMATE algorithm was used to infer the immune score of each sample. The characteristic genes of 29 immune cells were extracted from a previous study (18), and the single-sample gene set enrichment analysis (ssGSEA) method was used to calculate the scores of the 29 immune cells. TIDE (http://tide.dfci.harvard.edu/) software was used for the evaluation of immunotherapy.
Gene mutation analysis
The SNV mutation of key genes was extracted by the maftools package, and the collinearity and mutual exclusion were analysed by the somaticInteractions function according to the mutation.
Statistical analysis
Statistical processing was carried out by R software v4.0.3 (R Foundation for Statistical Computing, 2020). DEGs were analysed by the unpaired Wilcox test, and the K-M method was used to evaluate survival. In order to compare the survival curves, the HR and log-rank P value in the K-M Plotter were calculated by the time series test. Pearson’s correlation coefficient was used to analyse the correlation of genes with immune cells. We used the two-side test for all P values and P<0.05 suggested a notable difference.
Results
Screening of DEGs
Through differential expression analysis, 316 DEGs were obtained in the GSE26901 data set, including 222 differentially upregulated ones and 94 differentially down-regulated ones (Figure 1A). In the TCGA-STAD tumour tissues and normal tissues, 8,900 DEGs were screened, including 6694 differentially upregulated ones and 2206 differentially down-regulated ones (Figure 1B). Subsequently, 179 overlapping genes (Table S1) were obtained by intersecting the DEGs of the two data sets. We then screened out 36 genes associated with prognosis (Table S2) by conducting univariate Cox analysis of the 179 key genes.
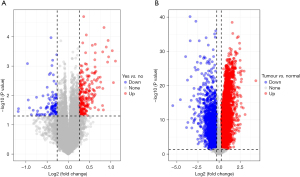
Key genes screened by LASSO regression analysis
The 36 genes were further screened via LASSO regression for reducing the number of genes in the risk model. Firstly, the change track of every independent variable was analysed, as shown in Figure 2A. Accordingly, as the lambda increased gradually, the number of independent variable coefficients close to zero also increased gradually. Next, 10-fold cross-validation was used to build the model and analyse the CI under each lambda (Figure 2B). It can be seen from the figure that the model is optimal if lambda =0.0194, so 12 genes with lambda =0.0194 were adopted as the target genes. To further screen the key genes, we performed stepwise regression based on the AIC and used the stepAIC algorithm to optimise the model. Finally, 8 key genes were selected, namely KCNJ2, GATA5, CLDN1, SERPINE1, FCER2, PMEPA1, TMEM37 and CRTAC1. Univariate Cox analysis showed the P value, risk coefficient, HR and CI of these 8 genes’ expression and prognostic characteristics (Figure 2C).
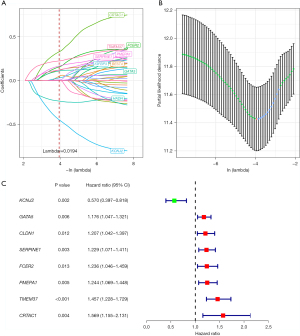
Prognosis of key genes and mutation analysis
The K-M method was used to analyse the survival curves of the 8 key genes. According to the results, high KCNJ2 expression was strongly associated with an unfavourable prognosis of STAD patients, while low expression of GATA5, CLDN1, SERPINE1, FCER2, PMEPA1, TMEM37 and CRTAC1 was strongly associated with a poor prognosis (Figure 3). Subsequently, the mutations of these 8 genes in STAD were evaluated (Figure 4A). The somatic interactions function analysis showed no collinearity and mutual exclusion among the 8 genes (Figure 4B). Additionally, only a few samples of the 8 genes were found to have copy number variation (CNV) mutations (Figure 4C). These results verified that these 8 key genes can stably help predict GA prognosis.
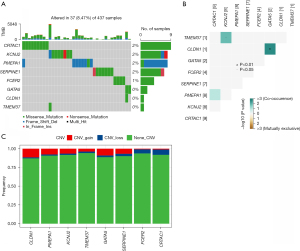
Correlation of key genes with immunity
For a deeper exploration of the correlation between key genes and immunity, we calculated the scores of 22 types of immune cells using the CIBERSORT algorithm, and then conducted an association analysis of immune cells and the 8 key genes by Pearson correlation analysis. According to the results, the expressions of KCNJ2, FCER2 and CRTAC1 were strongly associated with the scores of most immune cells (Figure 5A). Next, the immune score was calculated by the ESTIMATE method, and the correlation between immune score and the 8 key genes was also analysed by Pearson. According to the results, the expressions of SERPNE1, FCER2 and CRTAC1 were strongly linked to the immune score, matrix score and ESTIMATE score (Figure 5B). Additionally, we acquired the expression of immune checkpoint genes, and analysed their correlations with the 8 key genes by Pearson. According to the results, SERPINE1, FCER2 and TMEM37 were strongly linked to most immune checkpoint genes (Figure 5C). Moreover, we also calculated the immune cell score by the TIMER method, and found significant correlations between FCER2 expression and the scores of 6 types of immune cell (Figure 5D). Finally, we extracted the characteristic genes of 29 immune cells, and calculated their scores by the ssGSEA method. The results indicated a strong association between the expressions of SERPINE1 and FCER2 and the scores of most immune cells (Figure 5E).
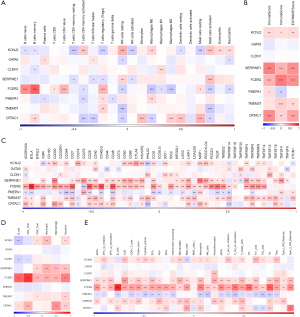
Construction of an 8-key-genes-based risk model for prognosis forecasting
With the TCGA data set as the training set, a risk model for prognosis forecasting based on the risk scores of the 8 key genes was constructed, and the samples were assigned to high- or low-risk group based on the median risk score. According to the results, the high-risk group had notably worse prognosis (Figure 6A). The ability of the model to predict the prognosis of patients in 1–5 years was calculated by the timeROC package, and the results showed that the model had an area under the curve (AUC) up to 0.7 (Figure 6B), indicating good ability in the TCGA data set for forecasting the patients’ prognosis. The independent data set GSE84437 was used for verification, and K-M survival analysis uncovered a strong correlation of the high-risk group with poor prognosis, and also revealed the good predictive ability of the model for 1–5-year prognosis forecast (Figure 6C,6D). By analysing the diagnostic value of the 8 key genes and the combined risk model for treatment on the basis of the GSE26901 data set, the AUC of the risk model for prediction was found to be up to 0.7 (Figure 6E), but the AUC values of these eight genes for diagnosis were all less than 0.7 (Figure 6F). These results indirectly showed that the effect of the combined 8 genes to distinguish patients before and after treatment was better than that of each gene alone.
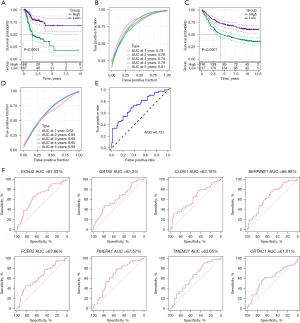
Clinicopathological characteristics and immune characteristics of different risk score groups
To test the relationship between risk scores and the clinical features of GA, we analysed risk scores between different TNM stages and clinical grades in the TCGA-STAD data set. The results showed that with increasing clinical grade, the risk score increased notably. In short, samples with a higher clinical grade had a higher risk score (Figure 7), and there was no notable difference in risk score between different sexes (P>0.05). For the purpose of clarifying differences in the immune microenvironment between different risk score groups, we compared the relative abundance of 22 immune cells in the high- and low-risk groups, and found notable differences between the risk score groups (Figure 8A). In addition, we also used ESTIMATE to evaluate immune cell infiltration, and found a notably lower immune score in the low-risk group, suggesting less immune cell infiltration in the low-risk group (Figure 8B). Moreover, we found different expressions of immune checkpoint genes between the groups (Figure 8C), and discovered strong correlations with the expression of most immune checkpoint genes. Further, we analysed the differences in immunotherapy among different molecular subtypes, and evaluated the potential clinical effects of immunotherapy in defined molecular subtypes using TIDE software. According to the results, the high-risk group had a notably higher TIDE score than the low-risk group in the TCGA cohort, suggesting that the high-risk group is more likely to escape immunity and less likely to benefit from immunotherapy (Figure 8D). We also analysed the response of different molecular subtypes in the TCGA cohort to cisplatin-based chemotherapy drugs, and found the high-risk group was more sensitive to these drugs than the low-risk group (Figure 8E). Overall, the results revealed differences in clinical phenotype and abnormalities in immune characteristics in the risk model based on the 8 key genes.
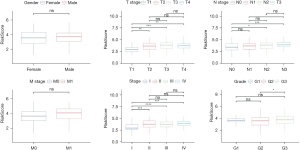
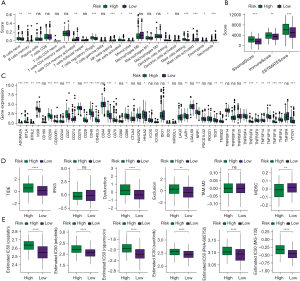
Univariate and multivariate Cox regression analyses
We analysed whether risk score was an independent prognostic factor of GA. Univariate and multivariate Cox regression analyses showed that sex and risk score may be independent prognostic factors of GA (Figure 9A,9B). We then generated a nomogram and used Cox regression to construct a model for forecasting 1-, 2- and 3-year OS (Figure 9C). The calibration results showed that in contrast to the ideal model, the 1, 2 and 3 years OS model had good predictability (Figure 9D), and the decision curve analysis showed that the nomogram was the optimal model (Figure 9E).
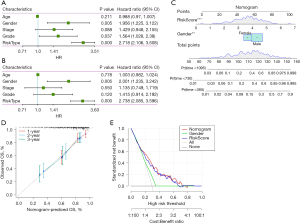
Discussion
NACT is a frequently used treatment for GA, which is often accompanied by tumour immune signals and microenvironment remodelling (19). However, clinical practice shows that more than a few GA patients gain no benefit from NACT because of the lack of biomarkers for patient selection and prognosis prediction (20). Therefore, to personalise the treatment it is essential to identify the patients who will benefit from NACT based on reliable prognostic and predictive factors. Although some clinical and tumour features can help identify patients with poor prognosis, they are not related to the treatment received (21-23). Therefore, it is imperative to identify the gene signature associated with treatment for predicting prognosis or the therapeutic effect.
In our study, TGCA-STAD data and GSE26901 data set were chosen, and 178 genes were identified using DEG analysis. A prediction model composed of 8 signature genes related to chemotherapy for GA was constructed via univariate Cox analysis and LASSO regression analysis. The model demonstrated high efficacy in forecasting the GA patients’ prognosis within 1–5 years and could be used to effectively distinguish the curative effect in patients before and after chemotherapy.
The 8 signature genes related to chemotherapy comprise KCNJ2, GATA5, CLDN1, SERPINE1, FCER2, PMEPA1, TMEM37 and CRTAC1. First, the correlation between these 8 genes and survival was analysed. According to the results, high KCNJ2 expression was strongly linked with an unfavourable prognosis, and patients with lower expression of the other 7 genes also had an unfavourable prognosis. The association of KCNJ2 with tumour progression and prognosis has been confirmed by a previous study (24). Reportedly, in cases of small cell lung cancer, inhibiting the expression of KCNJ2 promoted cell apoptosis, inhibited the cell cycle and enhanced the sensitivity of cancer cells to chemotherapeutic drugs (25). In addition, silencing KCNJ2 expression can greatly weaken the invasion and metastasis of GA cells as well as epithelial–mesenchymal transformation (26). The correlations of these 8 key genes with immunity were also evaluated, and immune cells in GA cases were scored by different algorithms for correlation analysis of gene expression with immune score. Notably, the expression of FCER2 was strongly linked to immune cell scores in the various algorithms. FCER2 encodes the key molecule of B cell activation and growth. It is the receptor of immunoglobulin (Ig)E and is considered to be an ideal candidate gene for producing traits closely related to the IgE-mediated immune response (27,28). Moreover, FCER2 in urine has been identified as a biomarker that can be used to improve eligibility for prostate biopsy and detect high-grade prostate cancer (29). The results of our study provide a starting point for its role in GA and immunity.
Finally, the relationship between the value of the 8-signature gene-based risk model for prognosis prediction of GA and clinical related characteristics was analysed. The risk model was found to have excellent 1–5-year prognostic efficacy, in both the training and validation sets. The risk model also demonstrated good diagnostic efficiency for predicting the chemotherapeutic effect of GA. In addition, the risk scores were grouped based on this risk model and their correlation with immune characteristics were analysed. The results revealed notable differences in some immune cell scores among the different risk score groups, and also revealed lower immune cell infiltration and a lower possibility of immune escape in the low-risk group. More and more evidences show that the tumour microenvironment, including tumour-infiltrating immune cells, supports the growth and development of cancer, and further promotes invasion, metastasis and sensitivity to drug therapy (30,31). Immune escape surveillance, in which the host immune system recognises and destroys cancer cells, is a sign of biological ability (32) and has been reported in both animal and human cancer patients, which supports the hypothesis that the immune escape of cancer cells is a crucial step in tumour development (33). There is an immunogenic interaction between tumour and host, and tumour immune escape recognition often determines the clinical course of the disease (34). Therefore, we believe that our risk model will be helpful in identifying tumour immune escape and can be used to determine the clinical course of the disease. Moreover, the results showed that as clinical grade increases, the risk score increases notably. Therefore, this risk model can be used to predict the chemotherapeutic effect and prognosis of GA patients, and can be used to study changes in the tumour microenvironment during the development of GA.
Conclusions
We constructed an effective and accurate STAD prognosis prediction model based on 8 chemotherapy-related signature genes, which demonstrated good predictability. According to the risk score, it is able to strongly distinguish high-risk from low-risk patients, and can be used to identify the clinical course and chemotherapeutic effect of GA patients. However, this study also had some limitations. It mainly depended on bioinformatics analysis, so the results need to be verified by a series of biological experiments, and the potential biological mechanisms and pathways associated with these 8 genes need further study.
Acknowledgments
Funding: This study was supported by Fund of Ningbo Yinzhou Science and Technology Bureau (No. 2017-110).
Footnote
Reporting Checklist: The authors have completed the TRIPOD reporting checklist. Available at https://jgo.amegroups.com/article/view/10.21037/jgo-22-872/rc
Conflicts of Interest: All authors have completed the ICMJE uniform disclosure form (available at https://jgo.amegroups.com/article/view/10.21037/jgo-22-872/coif). The authors have no conflicts of interest to declare.
Ethical Statement: The authors are accountable for all aspects of the work in ensuring that questions related to the accuracy or integrity of any part of the work are appropriately investigated and resolved. The study was conducted in accordance with the Declaration of Helsinki (as revised in 2013).
Open Access Statement: This is an Open Access article distributed in accordance with the Creative Commons Attribution-NonCommercial-NoDerivs 4.0 International License (CC BY-NC-ND 4.0), which permits the non-commercial replication and distribution of the article with the strict proviso that no changes or edits are made and the original work is properly cited (including links to both the formal publication through the relevant DOI and the license). See: https://creativecommons.org/licenses/by-nc-nd/4.0/.
References
- Deng W, Jin L, Zhuo H, et al. Alcohol consumption and risk of stomach cancer: A meta-analysis. Chem Biol Interact 2021;336:109365. [Crossref] [PubMed]
- Clark PI, Slevin ML. Chemotherapy for stomach cancer. Br Med J (Clin Res Ed) 1987;295:870-1. [Crossref] [PubMed]
- Tey J, Soon YY, Koh WY, et al. Palliative radiotherapy for gastric cancer: a systematic review and meta-analysis. Oncotarget 2017;8:25797-805. [Crossref] [PubMed]
- Kelly RJ, Ajani JA, Kuzdzal J, et al. Adjuvant Nivolumab in Resected Esophageal or Gastroesophageal Junction Cancer. N Engl J Med 2021;384:1191-203. [Crossref] [PubMed]
- Lu J, Zheng CH, Xu BB, et al. Assessment of Robotic Versus Laparoscopic Distal Gastrectomy for Gastric Cancer: A Randomized Controlled Trial. Ann Surg 2021;273:858-67. [Crossref] [PubMed]
- Yu J, Huang C, Sun Y, et al. Effect of Laparoscopic vs Open Distal Gastrectomy on 3-Year Disease-Free Survival in Patients With Locally Advanced Gastric Cancer: The CLASS-01 Randomized Clinical Trial. JAMA 2019;321:1983-92. [Crossref] [PubMed]
- Zhang X, Li M, Chen S, et al. Endoscopic Screening in Asian Countries Is Associated With Reduced Gastric Cancer Mortality: A Meta-analysis and Systematic Review. Gastroenterology 2018;155:347-354.e9. [Crossref] [PubMed]
- Park SH, Lim DH, Sohn TS, et al. A randomized phase III trial comparing adjuvant single-agent S1, S-1 with oxaliplatin, and postoperative chemoradiation with S-1 and oxaliplatin in patients with node-positive gastric cancer after D2 resection: the ARTIST 2 trial☆. Ann Oncol 2021;32:368-74. [Crossref] [PubMed]
- Fujitani K, Yang HK, Mizusawa J, et al. Gastrectomy plus chemotherapy versus chemotherapy alone for advanced gastric cancer with a single non-curable factor (REGATTA): a phase 3, randomised controlled trial. Lancet Oncol 2016;17:309-18. [Crossref] [PubMed]
- Ge L, Hou L, Yang Q, et al. A systematic review and network meta-analysis protocol of adjuvant chemotherapy regimens for resected gastric cancer. Medicine (Baltimore) 2019;98:e14478. [Crossref] [PubMed]
- Sasako M, Sakuramoto S, Katai H, et al. Five-year outcomes of a randomized phase III trial comparing adjuvant chemotherapy with S-1 versus surgery alone in stage II or III gastric cancer. J Clin Oncol 2011;29:4387-93. [Crossref] [PubMed]
- Kakeji Y, Yoshida K, Kodera Y, et al. Three-year outcomes of a randomized phase III trial comparing adjuvant chemotherapy with S-1 plus docetaxel versus S-1 alone in stage III gastric cancer: JACCRO GC-07. Gastric Cancer 2022;25:188-96. [Crossref] [PubMed]
- Zhang YW, Zhang YL, Pan H, et al. Chemotherapy for patients with gastric cancer after complete resection: a network meta-analysis. World J Gastroenterol 2014;20:584-92. [Crossref] [PubMed]
- Zhou L, Li SH, Wu Y, et al. Establishment of a prognostic model of four genes in gastric cancer based on multiple data sets. Cancer Med 2021;10:3309-22. [Crossref] [PubMed]
- Oh SC, Sohn BH, Cheong JH, et al. Clinical and genomic landscape of gastric cancer with a mesenchymal phenotype. Nat Commun 2018;9:1777. [Crossref] [PubMed]
- Yoon SJ, Park J, Shin Y, et al. Deconvolution of diffuse gastric cancer and the suppression of CD34 on the BALB/c nude mice model. BMC Cancer 2020;20:314. [Crossref] [PubMed]
- Danilova L, Ho WJ, Zhu Q, et al. Programmed Cell Death Ligand-1 (PD-L1) and CD8 Expression Profiling Identify an Immunologic Subtype of Pancreatic Ductal Adenocarcinomas with Favorable Survival. Cancer Immunol Res 2019;7:886-95. [Crossref] [PubMed]
- Bindea G, Mlecnik B, Tosolini M, et al. Spatiotemporal dynamics of intratumoral immune cells reveal the immune landscape in human cancer. Immunity 2013;39:782-95. [Crossref] [PubMed]
- Li Z, Gao X, Peng X, et al. Multi-omics characterization of molecular features of gastric cancer correlated with response to neoadjuvant chemotherapy. Sci Adv 2020;6:eaay4211. [Crossref] [PubMed]
- Li Z, Jia Y, Zhu H, et al. Tumor mutation burden is correlated with response and prognosis in microsatellite-stable (MSS) gastric cancer patients undergoing neoadjuvant chemotherapy. Gastric Cancer 2021;24:1342-54. [Crossref] [PubMed]
- van den Boorn HG, Engelhardt EG, van Kleef J, et al. Prediction models for patients with esophageal or gastric cancer: A systematic review and meta-analysis. PLoS One 2018;13:e0192310. [Crossref] [PubMed]
- Sohn BH, Hwang JE, Jang HJ, et al. Clinical Significance of Four Molecular Subtypes of Gastric Cancer Identified by The Cancer Genome Atlas Project. Clin Cancer Res 2017;23:4441-9. [Crossref] [PubMed]
- Shao W, Yang Z, Fu Y, et al. The Pyroptosis-Related Signature Predicts Prognosis and Indicates Immune Microenvironment Infiltration in Gastric Cancer. Front Cell Dev Biol 2021;9:676485. [Crossref] [PubMed]
- Valdora FF, Freier C, Seyler N, et al. Kcnj2 comprises a marker of poor prognosis and a therapeutic target in non-wnt/non-shh medulloblastoma. Cancer Res 2012;72:1424-24. [Crossref]
- Liu H, Huang J, Peng J, et al. Upregulation of the inwardly rectifying potassium channel Kir2.1 (KCNJ2) modulates multidrug resistance of small-cell lung cancer under the regulation of miR-7 and the Ras/MAPK pathway. Mol Cancer 2015;14:59. [Crossref] [PubMed]
- Ji CD, Wang YX, Xiang DF, et al. Kir2.1 Interaction with Stk38 Promotes Invasion and Metastasis of Human Gastric Cancer by Enhancing MEKK2-MEK1/2-ERK1/2 Signaling. Cancer Res 2018;78:3041-53. [Crossref] [PubMed]
- Laitinen T, Ollikainen V, Lázaro C, et al. Association study of the chromosomal region containing the FCER2 gene suggests it has a regulatory role in atopic disorders. Am J Respir Crit Care Med 2000;161:700-6. [Crossref] [PubMed]
- Shen M, Vermeulen R, Rajaraman P, et al. Polymorphisms in innate immunity genes and lung cancer risk in Xuanwei, China. Environ Mol Mutagen 2009;50:285-90. [Crossref] [PubMed]
- Alijaj N, Pavlovic B, Martel P, et al. Identification of Urine Biomarkers to Improve Eligibility for Prostate Biopsy and Detect High-Grade Prostate Cancer. Cancers (Basel) 2022;14:1135. [Crossref] [PubMed]
- Steven A, Seliger B. The Role of Immune Escape and Immune Cell Infiltration in Breast Cancer. Breast Care (Basel) 2018;13:16-21. [Crossref] [PubMed]
- Lin Y, Pan X, Zhao L, et al. Immune cell infiltration signatures identified molecular subtypes and underlying mechanisms in gastric cancer. NPJ Genom Med 2021;6:83. [Crossref] [PubMed]
- Hanahan D, Weinberg RA. Hallmarks of cancer: the next generation. Cell 2011;144:646-74. [Crossref] [PubMed]
- Klein G, Klein E. Surveillance against tumors--is it mainly immunological? Immunol Lett 2005;100:29-33. [Crossref] [PubMed]
- Hoenicke L, Zender L. Immune surveillance of senescent cells--biological significance in cancer- and non-cancer pathologies. Carcinogenesis 2012;33:1123-6. [Crossref] [PubMed]
(English Language Editor: K. Brown)