Identification and validation in a novel quantification system of the glutamine metabolism patterns for the prediction of prognosis and therapy response in hepatocellular carcinoma
Introduction
Hepatocellular carcinoma (HCC), the most frequent primary tumor of the liver, is a leading cause of cancer-associated mortality worldwide (1). The poor prognosis and a low 5-year survival rate of HCC patients are attributed to the absence of recognizable physical symptoms and the lack of sensitive screening methods. At present, surgical resection remains the primary option for HCC patients (2). However, as a result of tumor heterogeneity, late diagnosis, and high recurrence, the long-term survival rate of HCC patients remains low. Thus, we urgently need to develop a feasible and reliable predictive model to better evaluate the prognosis of HCC patients.
Glutamine is the most abundant amino acid in human plasma. It provides cells with carbon and nitrogen source energy and maintains fatty-acid synthesis (3). Aberrant nutrient metabolism is considered a hallmark characteristic of cancer (4). Recent studies have investigated metabolic reprogramming in cancer, among which glutamine metabolism (GM) has been widely investigated because of its criticality (5,6). It has been reported that the effective inhibition of tumor-specific glutaminase (GLS) reduces tumor initiation (7). The term “glutamine addiction” has been used to describe the strong dependence of most cancer cells on essential nitrogen substrates after metabolic reprogramming (8). An increased rate of glutaminolysis and glutamine uptake have been shown to be critical metabolic features in several human cancers, including HCC (9-11). For instance, Glutaminase 2 (GLS2) and carbamoyl phosphate synthetase 1 (CPS1), glutamine metabolism-related genes (GMRGs), are involved in glutamine metabolic reprogramming to promote HCC progression. Furthermore, GLS2 and CPS1 expression were associated with overall survival of HCC and may serve as a therapeutic and diagnostic target for HCC. Thus, GM-related genes (GMRGs) may be an important prognostic factor for HCC.
In this study, the expression of 23 GMRGs and their relationship with prognosis were analyzed based on gene expression and corresponding clinical information data. We sought to establish a risk model based on multiple GMRGs to predict the prognosis of HCC patients that can serve as a new tool for predicting the prognosis and guiding the treatment of HCC patients. We present the following article in accordance with the TRIPOD reporting checklist (available at https://jgo.amegroups.com/article/view/10.21037/jgo-22-895/rc).
Methods
The study was conducted in accordance with the Declaration of Helsinki (as revised in 2013). The present study sought to examine the predictive value of GM in HCC patient’s prognosis and therapy response. Our findings provide a GMRGs to predict the prognosis of HCC patients that can serve as a new tool for predicting the prognosis and guiding the treatment of HCC patients. The immune infiltration analysis, pathway enrichment analysis was also compared to each other in the 2 risk groups.
Data collection
We obtained 377 HCC samples with clinical information from The Cancer Genome Atlas (TCGA) database (https://cancergenome.nih.gov/). We also obtained 242 HCC samples (GSE14520) (12) with clinical information and ribonucleic acid (RNA)-sequencing data from the Gene Expression Omnibus (GEO) (https://www.ncbi.nlm.nih.gov/gds) for verification (Table 1). We acquired the GMRGs from the gene set “GO_GLUTAMINE_METABOLIC_PROCESS” of the Molecular Signatures Database v 7.5.1 for the gene set enrichment analysis (GSEA) (http://www.broadinstitute.org/gsea/msigdb/index.jsp). The copy number variation (CNV) analyses were performed using the “Rcircos” package. We used the GSE104580 and GSE109211 (13) chip in this study to analyze transcatheter arterial chemoembolization (TACE) and sorafenib sensitivity.
Table 1
Characteristic | TCGA database | GSE14520 |
---|---|---|
Age (years) | ||
≤53 | 111 (29.44%) | 93 (38.43%) |
>53 | 265 (70.29%) | 149 (61.57%) |
Unknown | 1 (0.265%) | 0 (0.00%) |
Gender | ||
Male | 255 (67.64%) | 211 (87.19%) |
Female | 122 (32.36%) | 31 (12.81%) |
Grade | ||
G1 | 55 (14.59%) | – |
G2 | 180 (47.75%) | – |
G3 | 124 (32.89%) | – |
G4 | 13 (3.45%) | – |
Unknown | 5 (1.33%) | – |
Stage | ||
Stage I | 175 (46.42%) | 96 (39.67%) |
Stage II | 87 (23.08%) | 78 (32.23%) |
Stage III | 86 (22.81%) | 51 (21.07%) |
Stage IV | 5 (1.33%) | 0 (0.00%) |
Unknown | 24 (6.37%) | 17 (7.02%) |
Survival status | ||
Live | 243 (64.46%) | 136 (56.20%) |
Dead | 123 (32.63%) | 85 (35.12%) |
Unknown | 11 (2.92%) | 21 (35.12%) |
HCC, hepatocellular carcinoma; TCGA, The Cancer Genome Atlas; GEO, Gene Expression Omnibus.
NMF clustering
To identify the potential features of the gene expression profile, the original matrix was subdivided into 2 non-negative matrices based on the NMF algorithm. A K value of 2 was the best cluster value, according to the cophenetic coefficient, sample size, and contour. Through the R package of “prcomp”, a principal component analysis (PCA) scoring system was constructed for all the selected GMRGs.
Evaluation of the immune cells in the TIME
Estimation of Stromal and Immune cells in Malignant Tumor tissues using Expression data (ESTIMATE), MCP-count, quanTIseq, Cell-type identification by estimating relative subsets of RNA transcripts (CIBERSORT), Tumor IMmune Estimation Resource (TIMER), single-sample gene set enrichment analysis (ssGSEA), xCell, and EPIC were used in R to assess the tumor immune microenvironment (TIME) status of each HCC sample (14).
Pathway enrichment analysis
To investigate the potential biological mechanism, we performed a Hall marker pathway analyses. The gene sets of “h.all.v7.5.1.symbols.gmt” were obtained from the MSigDB database for the GSEA. Through the R package of “GSVA,” we investigated the by gene set variation analysis (GSVA). The top 30 results with adjusted P values <0.05 were considered significant.
Establishment of a prognostic risk model based on the GMRGs
A univariate Cox proportional-hazard regression analysis (UCR) was conducted to identify the survival-related GMRGs using the survival package in R. Next, least absolute shrinkage and selection operator (LASSO)—penalized Cox regression analyses were conducted to further identify the genes screened by the UCR. The best prognostic-related genes were identified to establish a prognostic risk-score model for predicting prognosis. We stratified all the patients into high- and low-risk groups according to the median risk score. A Kaplan-Meier (K-M) analysis, log-rank test, and Cox regression were used for the survival analysis (the survival and survminer packages were used). The R package “timeROC” was used to test the time-dependent receiver operating characteristic (ROC) curve.
The efficacy evaluation of immunotherapy, chemotherapy, and targeted drug therapy
We employed the “oncoPredict” package in R to perform the prediction process; the half-maximal inhibitory concentrations (IC50s) of the samples were estimated by a ridge regression. Next, the IC50 differences between the 2 risk groups were compared.
To investigate immunotherapy, the sequencing data were uploaded to the Tumor Immune Dysfunction and Exclusion (TIDE) website (http://tide.dfci.harvard.edu/) (15).
Statistical analysis
We used R software 4.0.4 and the Perl language packages to perform all the statistical analysis and plot the results. The Wilcoxon rank-sum test was used to compare the continuous data between the 2 groups. The frequencies of the categorical data were compared using the chi-square test. The PCA was conducted using the “prcomp” function of the “stats” package. Threshold values for AUC between 0.5 and 1 are considered as better than random classifiers. In all the analyses, a P value <0.05 was considered statistically significant.
Results
The landscape of genetic variation of GMRGs in HCC
As Figure 1 shows, a total of 21 CNVs were identified in the GMRGs, including 14 CNV gains and 7 CNV losses (see Figure 1A,1B). In total, 19 genes differed significantly between the HCC and adjacent tissues (of which 9 were upregulated, and 10 were downregulated) (see Figure 1C). Our results indicated that the expression levels of the GMRGs differed significantly, which could potentially contribute to tumorigenesis.
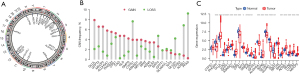
NMF clustering of GM-related patterns
According to the 23 GMRGs in the univariate Cox regression model, NMF clustering was used in TCGA-HCC cohort. K =2 was the best clustering result based on the cophenetic coefficients (see Figure 2A,2B). Next, we conducted a PCA to further examine the difference between C1 (N=254) and C2 (N=112) in the levels of GMRG transcription (see Figure 2C). Compared to C2, the K-M analysis suggested that the overall survival (OS) and progression-free survival (PFS) of the C1 patients were significantly longer than those of the C2 patients (see Figure 2D,2E; P<0.05). Finally, to examine the difference between C1 and C2 in terms of the clinicopathological characteristics, we used the chi-square test (see Figure 2F). As Figure 2F shows, the pathologic stage, T stages, N stages, and histologic grade distribution differed significantly between C1 and C2. The transcription profile heatmaps of the GMRGs in C1 and C2 are shown in Figure 2G.

The TIME of the GM-related patterns
We conducted a GSVA to examine the hallmark pathways, and confirmed that immune, metabolic, and carcinogenic-related pathways were associated with GM-related patterns (see Figure 3A). We found that the carcinogenic metabolic-related pathways, including in the regulation of fatty-acid metabolism and Kirsten rat sarcoma viral oncogene (KRAS) signaling, were more highly expressed in the C1 patients. Conversely, the immune and carcinogenic-related pathways, including the unfolded protein response, PI3K/AKT/mTOR signaling, and Wnt/beta-catenin signaling, were significantly more highly enriched in the C2 patients (see Figure 3A). Additionally, the expression of multiple HLA class II molecules, such as Major Histocompatibility Complex, Class II, DM Alpha (HLA-DMA), Major Histocompatibility Complex, Class II, DM Beta (HLA-DMB), and Major Histocompatibility Complex, Class II, DO Alpha (HLA-DOA), was higher in the C2 than the C1 patients (see Figure 3B). We also found that many of the immune checkpoint–related genes were overexpressed in the C2 group, such as Programmed Cell Death 1 (PDCD1), Lymphocyte Activating 3 (LAG3), and Cytotoxic T-Lymphocyte Associated Protein 4 (CTLA4) (see Figure 3C).
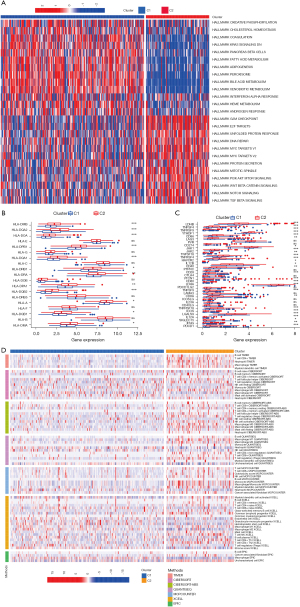
To determine the immune-related characteristics between C1 and C2, we quantified the TIME composition. Most of the immune cells, especially the tumor-killing cells, including cluster of differentiation CD8+ T cells, CD4+ T cells, M1 macrophages, and natural killer T (NKT) cells, were present in the C2 group. Conversely, the regulatory T cells (Tregs) and M2 macrophages were present in the C1 group (see Figure 3D). The above-mentioned results suggest that GM might affect the prognosis of the HCC patients through the potential regulation of these tumor-infiltrating immune cells (TIICs).
Identification of the prognosis-related GMRGs
First, based on the UCR model (P<0.05), a total of 9 GMRGs were found to be significantly related to OS (see Figure 4). Second, 5 genes [i.e., aldehyde dehydrogenase 5 family member A1 (ALDH5A1), ASNSD1, carbamoyl-phosphate synthetase 1 (CPS1), GMPS, and PPAT] were included in the LASSO-penalized Cox regression analyses. Based on the 5 identified prognostic GMRGs and their regression coefficients, a prognostic prediction system was established. The risk score was calculated using the following formula: risk score = (–0.0096 * ALDH5A1) + (0.0085 * ASNSD1) + (–0.0010 * CPS1) + (0.0619 * GMPS) + (0.3035 * PPAT).
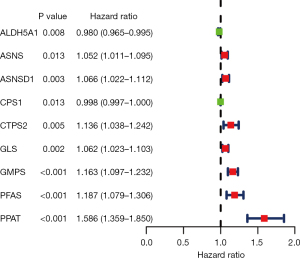
We next divided the HCC cases into high- and low-risk groups according to the median cut-off value of the scores. The K-M survival curves showed that the patients in the low-risk group generally had a better OS than those in the high-risk group (P<0.001; see Figure 5A). The areas under the curve (AUCs) were 0.753, 0.687, and 0.661 at 1, 3, and 5 years, respectively, in TCGA cohort (see Figure 5B). The scatter plot indicated that the mortality rate of HCC increases with the increase of risk score (see Figure 5C,5D). We further investigated the expression levels of the 5 genes and found that the risk scores were positively correlated with the expression levels of ALDH5A1, ASNSD1, and GMPS (see Figure 5E). The efficacy of the prognostic model was validated by GEO cohort. The results are consistent with the results from the TCGA datasets (see Figure 6A-6E).
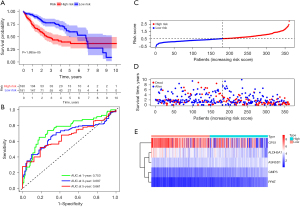
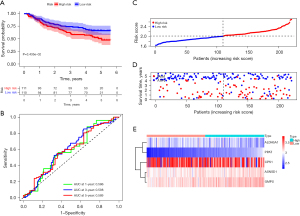
Independent prediction of HCC prognosis by the risk score
We then sought to further analyze the relevance of the risk score and clinicopathological characteristics of HCC. In TCGA cohort, the distribution of histologic grade, pathologic stage, and T stages differed significantly between the low- and high-risk groups (see Figure 7A). In the GEO cohort, the distribution of pathologic stage and tumor size differed significantly between the low- and high-risk groups (see Figure 7B). In summary, patients in the high-risk group had a tendency for poor prognosis results and advanced pathological characteristics. We the performed a UCR and MCR to further explore the prognostic value of the GM signature and various clinicopathological parameters. The results of the UCR showed that the GM signature (P<0.001) and stage (P<0.001) were significantly correlated with OS in both data sets (see Figure 7C,7D). The subsequent MCR analysis indicated that the GM signature and stage could be used as a robust independent prognostic indicator for the HCC patients in both the data sets (see Figure 7E,7F). Thus, the GM signature was an independent prognostic factor for HCC.
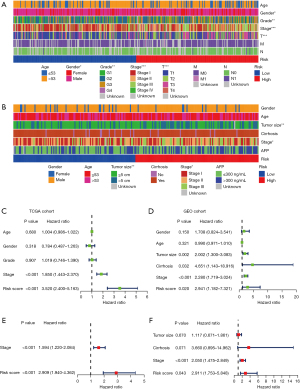
Correlation between the GM signature and the TIME
The above results revealed the role of GM-related patterns in the inflammatory response and the distinction in immunophenotypes, and the correlation between the GM signature and TIME were further analyzed. Our results indicated that the expression of HLA-DOA in the high-risk group was significantly higher than that in the low-risk group (see Figure 8A,8B). We also found that the levels of 38 immune checkpoint–related genes, such as YTH N6-Methyladenosine RNA Binding Protein 1 (YTHDF1), TNF Superfamily Member 4 (TNFSF4), and CD40 Molecule (CD40), were overexpressed in the high-risk group (see Figure 8C,8D). There was a tendency for the high-risk patients to have higher immune infiltration in levels, including CD8+ T cells, CD4+ T cells, macrophage, neutrophils, B memory cells, and NKT cells (see Figure 8E). The above results suggested that the GM signature promotes HCC progress through the potential regulation of these TIICs.
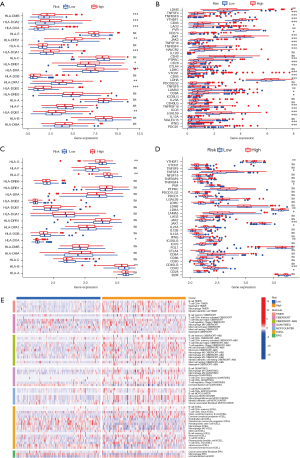
Correlation between the GM signature and drug sensitivity
Compared to the low-risk group, the IC50 values of axitinib (P=4*10-9; see Figure 9A), sunitinib (P=0.0018; see Figure 9B), sorafenib (P=6.2×10-6; see Figure 9C), AKT.inhibitor.VIII (P=2×10-7; see Figure 9D), and gefitinib (P=9.1×10-15; see Figure 9E) were significantly higher in the high-risk group than the low-risk group; thus, patients with lower GM scores were more sensitive to these drugs. However, the IC50 value of gemcitabine (P<0.0001; see Figure 9F) was significantly higher in the low-risk group than the high-risk group. The results showed that the high-risk group was more sensitive to gemcitabine. To further verify the above results, 2 GEO data sets (i.e., GSE109211 and GSE104580) were used as the test set. We then used this same risk-score formula to analyze the patients in the verification cohorts. The results indicated that the sorafenib-response group had lower risk scores than the sorafenib-non-response group (P=0.0016; see Figure 9G). Further, the low-risk group had higher response rates than the high-risk group (85% vs. 53%) (see Figure 9G). Similarly, the TACE-response group had lower risk scores than the TACE-non-response group (P=0.013; see Figure 9H). The low-risk group patients had higher response rates than the high-risk group patients (64% vs. 46%) (see Figure 9H). Finally, the TIDE algorithm was applied to predict the immunotherapy responses of the HCC patients. The low-risk group had a significantly higher TIDE score and dysfunction score (P<0.05; see Figure 9I,9J). Further, the high-risk group had a significantly higher Microsatellite Instability (MSI) score and exclusion score, which suggests that the high-risk group was potentially more sensitive to immunotherapy than the low-risk group (P<0.05; see Figure 9K,9L).
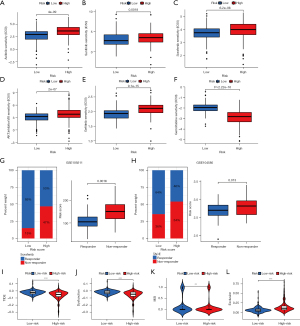
The GSEA revealed that the activated pathways in the high-risk group were mainly associated with the cancer and immune response, including the unfolded protein response, mTOR signaling, and PI3K/AKT/mTOR signaling. The pathways activated in the low-risk group were more involved in metabolic correlation functions, including fatty-acid metabolism, and KRAS signaling (see Figure 10).
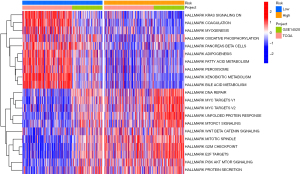
Discussion
Despite advances in diagnostic methods and treatment, the prognosis of HCC patients remains poor (2). The causes of HCC are heterogeneous, and metabolic, genetic and epigenetic alterations are associated with tumor progression. However, gene mutations and changes in the signaling pathways, which support the energy requirements of tumor cells, have been shown to promote the progression and metastasis of tumors (16). Metabolism reprogramming in tumors has recently been shown to be another common feature of cancer (8,17). Thus, research on the abnormal metabolism of HCC has attracted more attention.
As a non-essential amino acid, glutamine can be used as a nitrogen source to provide energy and antagonize reactive oxygen species, which can facilitate tumor progression (8). In the GM pathway, 2 types of GLS (i.e., GLS1 and GLS2) are critical enzymes (18). The expression of GLS1 has been reported to be elevated in HCC tissues, and the overexpression of GLS1 is associated with the poor prognosis of HCC patients; thus, GLS1 might be a potential target in HCC therapy (19). The evidence has suggested that GM plays an important role in anti-cancer immunity. A glutamine blockade has been shown to suppress cancer cells and to be a highly activated phenotype for effector T cells (20). Targeting GM renders immune-checkpoint inhibitor (ICI)–resistant tumors susceptible to immunotherapy in a breast cancer.
In this study, we identified 2 GM-related patterns based on the expression of the GMRGs through NMF clustering. The C2 group had a poor OS probability and significantly elevated advanced clinicopathological stages. Further, the GM-related patterns not only showed significant differences in biological pathway enrichment, but also displayed significant TIME cell infiltration. Additionally, we found that several immune checkpoints were highly expressed in the C2 group. These results indicate that GM is significantly related to the immune landscape of HCC.
We then united the gene set of GMRGs with HCC and investigated the underlying prognostic value of the GMRGs in HCC. A risk model based on 5 prognostic GMRGs (i.e., ALDH5A1, ASNSD1, CPS1, GMPS, and PPAT) was constructed. Using this model, every HCC patient was assigned a risk score. In TCGA dataset, the survival difference between the low- and high-score HCC patients was significant. The ROC curves and AUCs indicated that the models performed well. Further, the risk score could be used as an independent prognostic marker. The conclusion was verified with the GEO data. This prognostic system might help HCC patients to evaluate their prognosis by enabling the realization of individualized survival predictions, which reveal better treatment options.
Among the 5 model genes included in the prognostic signature, ALDH5A1 is essential for the synthesis of various molecules (21). In several tumors, such as ovarian cancer, the expression of ALDH5A1 is downregulated. ALDH5A1 could reprogram gamma-aminobutyric acid metabolism and acquire tumor stem cell-like properties (21). CPS1 plays crucial functions in the progression of cancer (22). Previous studies have shown that CPS1 is downregulated in HCC and is associated with a poor prognosis (22). GMPS is involved in cell proliferation and deoxyribonucleic acid replication (23). GMPS is a potential target for ICI therapy (24). A high expression of GMPS accompanied by high levels of TIICs was associated with a poor prognosis in esophageal squamous cell carcinoma (25). PPAT catalysis is the first committed step of de novo purine nucleotide biosynthesis (26); thus, targeting PPAT might be a promising cancer strategy (27). PPAT could serve as a prognostic biomarker in HCC (28).
Immunotherapy is an active and promising area of clinical oncology that has transformed the care of diagnosed patients at unresectable stages. However, for most patients, ICI treatments are not effective enough (29). In our study, patients in the high-risk group had higher levels of immune-checkpoint molecules, a greater infiltration of anti-tumor cells (e.g., CD8+ T cells and B cells), and lower TIDE scores. TACE and molecular-targeted drugs are other effective strategies for treating advanced HCC. Drug sensitivity is influenced by numerous genes (30). Based on the IC50, the comparison results revealed that the high-risk patients were more resistant to axitinib, sunitinib, sorafenib, AKT.inhibitor.VIII, TACE, and gefitinib. The mechanism might result from the activation of the unfolded protein response, mTOR, and PI3K/AKT/mTOR signaling pathways. For example, sorafenib induced an unfolded protein response, and sorafenib resistance could be promoted by protein kinase RNA-like ER kinase (PERK)/activating transcription factor 4 (ATF4) activation in HCC (31). Mutations of the PI3K-AKT-mTOR pathway could be predictors of immune cell infiltration and immunotherapy efficacy in gastric adenocarcinoma (32). Thus, the GM-related signature may optimize the treatment of patients.
Conclusions
To sum up, the GM score can individualize and quantify the GM phenotype of patients and indicate the clinical characteristics, prognosis, and TIME in HCC. The GM score is an independent prognostic marker for HCC patients and can be used as a guiding indicator in the formulation of immunotherapy, TACE, and targeted drugs. However, this study had a number of limitations. For example, as a retrospective study, this research may be associated with some biases, and large, multicenter, prospective studies need to be conducted to further confirm our results.
Acknowledgments
We would like to thank TCGA, GEO, and TIDE databases for the provision of the data.
Funding: None.
Footnote
Reporting Checklist: The authors have completed the TRIPOD reporting checklist. Available at https://jgo.amegroups.com/article/view/10.21037/jgo-22-895/rc
Conflicts of Interest: All authors have completed the ICMJE uniform disclosure form (available at https://jgo.amegroups.com/article/view/10.21037/jgo-22-895/coif). The authors have no conflicts of interest to declare.
Ethical Statement: The authors are accountable for all aspects of the work in ensuring that questions related to the accuracy or integrity of any part of the work are appropriately investigated and resolved. The study was conducted in accordance with the Declaration of Helsinki (as revised in 2013).
Open Access Statement: This is an Open Access article distributed in accordance with the Creative Commons Attribution-NonCommercial-NoDerivs 4.0 International License (CC BY-NC-ND 4.0), which permits the non-commercial replication and distribution of the article with the strict proviso that no changes or edits are made and the original work is properly cited (including links to both the formal publication through the relevant DOI and the license). See: https://creativecommons.org/licenses/by-nc-nd/4.0/.
References
- Siegel RL, Miller KD, Fuchs HE, et al. Cancer Statistics, 2021. CA Cancer J Clin 2021;71:7-33. [Crossref] [PubMed]
- Grandhi MS, Kim AK, Ronnekleiv-Kelly SM, et al. Hepatocellular carcinoma: From diagnosis to treatment. Surg Oncol 2016;25:74-85. [Crossref] [PubMed]
- Altman BJ, Stine ZE, Dang CV. From Krebs to clinic: glutamine metabolism to cancer therapy. Nat Rev Cancer 2016;16:619-34. [Crossref] [PubMed]
- Ward PS, Thompson CB. Metabolic reprogramming: a cancer hallmark even warburg did not anticipate. Cancer Cell 2012;21:297-308. [Crossref] [PubMed]
- Osanai-Sasakawa A, Hosomi K, Sumitomo Y, et al. An anti-ASCT2 monoclonal antibody suppresses gastric cancer growth by inducing oxidative stress and antibody dependent cellular toxicity in preclinical models. Am J Cancer Res 2018;8:1499-513. [PubMed]
- Jacque N, Ronchetti AM, Larrue C, et al. Targeting glutaminolysis has antileukemic activity in acute myeloid leukemia and synergizes with BCL-2 inhibition. Blood 2015;126:1346-56. [Crossref] [PubMed]
- Xiang Y, Stine ZE, Xia J, et al. Targeted inhibition of tumor-specific glutaminase diminishes cell-autonomous tumorigenesis. J Clin Invest 2015;125:2293-306. [Crossref] [PubMed]
- Yang L, Venneti S, Nagrath D. Glutaminolysis: A Hallmark of Cancer Metabolism. Annu Rev Biomed Eng 2017;19:163-94. [Crossref] [PubMed]
- Pan T, Gao L, Wu G, et al. Elevated expression of glutaminase confers glucose utilization via glutaminolysis in prostate cancer. Biochem Biophys Res Commun 2015;456:452-8. [Crossref] [PubMed]
- Suzuki S, Venkatesh D, Kanda H, et al. GLS2 Is a Tumor Suppressor and a Regulator of Ferroptosis in Hepatocellular Carcinoma. Cancer Res 2022;82:3209-22. [Crossref] [PubMed]
- Wu T, Luo G, Lian Q, et al. Discovery of a Carbamoyl Phosphate Synthetase 1-Deficient HCC Subtype With Therapeutic Potential Through Integrative Genomic and Experimental Analysis. Hepatology 2021;74:3249-68. [Crossref] [PubMed]
- Roessler S, Jia HL, Budhu A, et al. A unique metastasis gene signature enables prediction of tumor relapse in early-stage hepatocellular carcinoma patients. Cancer Res 2010;70:10202-12. [Crossref] [PubMed]
- Pinyol R, Montal R, Bassaganyas L, et al. Molecular predictors of prevention of recurrence in HCC with sorafenib as adjuvant treatment and prognostic factors in the phase 3 STORM trial. Gut 2019;68:1065-75. [Crossref] [PubMed]
- Li T, Fu J, Zeng Z, et al. TIMER2.0 for analysis of tumor-infiltrating immune cells. Nucleic Acids Res 2020;48:W509-14. [Crossref] [PubMed]
- Jiang P, Gu S, Pan D, et al. Signatures of T cell dysfunction and exclusion predict cancer immunotherapy response. Nat Med 2018;24:1550-8. [Crossref] [PubMed]
- Cairns RA, Harris IS, Mak TW. Regulation of cancer cell metabolism. Nat Rev Cancer 2011;11:85-95. [Crossref] [PubMed]
- Galai G, Ben-David H, Levin L, et al. Pan-Cancer Analysis of Mitochondria Chaperone-Client Co-Expression Reveals Chaperone Functional Partitioning. Cancers (Basel) 2020;12:825. [Crossref] [PubMed]
- Zhang C, Liu J, Zhao Y, et al. Glutaminase 2 is a novel negative regulator of small GTPase Rac1 and mediates p53 function in suppressing metastasis. Elife 2016;5:e10727. [Crossref] [PubMed]
- Xi J, Sun Y, Zhang M, et al. GLS1 promotes proliferation in hepatocellular carcinoma cells via AKT/GSK3β/CyclinD1 pathway. Exp Cell Res 2019;381:1-9. [Crossref] [PubMed]
- Dai W, Xu L, Yu X, et al. OGDHL silencing promotes hepatocellular carcinoma by reprogramming glutamine metabolism. J Hepatol 2020;72:909-23. [Crossref] [PubMed]
- Jackson B, Brocker C, Thompson DC, et al. Update on the aldehyde dehydrogenase gene (ALDH) superfamily. Hum Genomics 2011;5:283-303. [Crossref] [PubMed]
- Ridder DA, Schindeldecker M, Weinmann A, et al. Key Enzymes in Pyrimidine Synthesis, CAD and CPS1, Predict Prognosis in Hepatocellular Carcinoma. Cancers (Basel) 2021;13:744. [Crossref] [PubMed]
- Welin M, Lehtiö L, Johansson A, et al. Substrate specificity and oligomerization of human GMP synthetase. J Mol Biol 2013;425:4323-33. [Crossref] [PubMed]
- Nakamura J, Lou L. Biochemical characterization of human GMP synthetase. J Biol Chem 1995;270:7347-53. [Crossref] [PubMed]
- Wang J, Luo FF, Huang TJ, et al. The upregulated expression of RFC4 and GMPS mediated by DNA copy number alteration is associated with the early diagnosis and immune escape of ESCC based on a bioinformatic analysis. Aging (Albany NY) 2021;13:21758-77. [Crossref] [PubMed]
- Iwahana H, Oka J, Mizusawa N, et al. Molecular cloning of human amidophosphoribosyltransferase. Biochem Biophys Res Commun 1993;190:192-200. [Crossref] [PubMed]
- Bibi N, Parveen Z, Nawaz MS, et al. In Silico Structure Modeling and Molecular Docking Analysis of Phosphoribosyl Pyrophosphate Amidotransferase (PPAT) with Antifolate Inhibitors. Curr Cancer Drug Targets 2019;19:408-16. [Crossref] [PubMed]
- Hu X, Bao M, Huang J, et al. Identification and Validation of Novel Biomarkers for Diagnosis and Prognosis of Hepatocellular Carcinoma. Front Oncol 2020;10:541479. [Crossref] [PubMed]
- Hegde PS, Chen DS. Top 10 Challenges in Cancer Immunotherapy. Immunity 2020;52:17-35. [Crossref] [PubMed]
- Ma B, Sheng J, Wang P, et al. Combinational phototherapy and hypoxia-activated chemotherapy favoring antitumor immune responses. Int J Nanomedicine 2019;14:4541-58. [Crossref] [PubMed]
- Lin JC, Yang PM, Liu TP. PERK/ATF4-Dependent ZFAS1 Upregulation Is Associated with Sorafenib Resistance in Hepatocellular Carcinoma Cells. Int J Mol Sci 2021;22:5848. [Crossref] [PubMed]
- Wang Z, Wang X, Xu Y, et al. Mutations of PI3K-AKT-mTOR pathway as predictors for immune cell infiltration and immunotherapy efficacy in dMMR/MSI-H gastric adenocarcinoma. BMC Med 2022;20:133. [Crossref] [PubMed]