A bioinformatics analysis for diagnostic roles of the E2F family in esophageal cancer
Introduction
E2F family transcription factors are among the most important links in the cell cycle regulatory network, which regulate proliferation, cell differentiation, and apoptosis, and participate in a variety of physiological and pathological processes. The E2F family consists of eight members: E2F1, E2F2, E2F3, E2F4, E2F5, E2F6, E2F7, and E2F8. Previous studies have confirmed that the E2F family is related to multiple malignant tumors, including breast, colorectal, gastric, liver, and ovarian cancers, etc. These comprehensive studies have also revealed potential biomarkers and conducted prognostic evaluations. High expressions of E2F1, E2F3, and E2F4 have been shown to be significantly associated with poor overall survival (OS) in gastric cancer; however, increased expressions of E2F2, E2F5, E2F6, and E2F7 have been associated with favorable OS outcomes (1). Also, E2F2, E2F5, and E2F8 might serve as potential prognostic biomarkers in ovarian cancer (2). Moreover, E2F5, E2F3, and E2F6 have a poor effect on the OS and disease-free survival (DFS) in hepatocellular carcinoma (HCC) patients and can serve as a prognostic indicator for these patients (3). We have previously found that the E2F family plays an important role in the digestive tracts of many individuals. At present, there are numerous reports concerning E2Fs in digestive tracts; however, those in esophageal cancer are lacking.
Esophageal cancer is the eighth most commonly occurring cancer worldwide and the sixth leading cause of cancer-related deaths (4). Esophageal squamous cell carcinoma and esophageal adenocarcinoma are the two main histological types of esophageal cancer. Esophageal cancer is characterized by its insidious onset, poor clinical prognosis, and high mortality rates. Surgery, chemotherapy, and radiotherapy are the mainstay of treatment. With the development of targeted therapy and immunotherapy, the treatment of esophageal cancer has shifted toward a precision medicine age. According to the genetic and molecular typing, the trend toward combination therapy using appropriate drugs has been confirmed. Indeed, the same is true for the screening of esophageal cancer. It is essential to identify new gene targets in esophageal cancer to discover potential therapeutic targets as well as prognostic and predictive biomarkers. Targeted therapy research in esophageal cancer mainly focuses on EGFR, HER2, MET, VEGF, VEGFR, etc., and immunotherapy has only recently become a first-line therapy (5). Due to tumor heterogeneity, there are currently still limited biomarkers that predict prognosis. Common tumor markers for esophageal cancer are CYFRA21-1, P53, Caspase-3, COX-2, E-cadherin, SCC-Ag, VEGF and CA199, which are not specific for ESCA and cannot be therapeutic targets. Therefore, there is a pressing need for predictive biomarkers, which can guide individualized therapy and improve prognosis.
E2Fs have been shown to play an important role in the cell cycle. Among these, E2F1, which is the most studied, has demonstrated an increased expression that is associated with effective chemotherapy in esophageal squamous cell cancer. Up-regulated E2F1 can induce increased expression of microRNA (miR)-26b, miR-203, and miR-622, thereby inhibiting the G1/S phase transition (6-8). Furthermore, previous studies have shown that E2F2 is a target gene of miR-98 and that E2F2 and miR-98 may be used as biomarkers for esophageal cancer (9,10). By using siRNAs (small interfering RNA) to respectively knock down E2F1, E2F2, and E2F3, Zhao et al. demonstrated that only E2F3 inhibition could down-regulate the mRNA (messenger RNA) expression of RACGAP1, which predicts a better prognosis (11). Also, up-regulated E2F4 promotes autophagy and increases the chemoresistance of esophageal squamous cancer cells (12). Moreover, E2F5 is associated with a poor prognosis of esophageal squamous cancer (13). No clear correlation has been identified between E2F6 and esophageal cancer yet. Silencing E2F7 reduces the proliferation, migration, and invasion of esophageal cancer cells and induces apoptosis (14). Up-regulated E2F8 regulates important cell functions, including cell cycle progression and ESCC (esophageal squamous cell carcinoma) proliferation (15). In summary, E2F family play a key role in cell cycle progression and apoptosis, and when dysregulated can lead to cancer. Therefore, E2F family are closely related to tumor development and progression. The study about E2F family as biomarkers and drug targets is particularly important.
The role of the E2F family has only been partially established in the existing literature. We hypothesize that at least one E2F gene can predict the prognosis of esophageal cancer. In this study, we applied bioinformatics techniques to the integrative analysis of the E2F family to discover the potential prognostic and therapeutic targets against ESCA. Bioinformatics techniques can make large scale high-throughput screens combined with a larger number of clinical samples, which significantly improve the efficiency and reveal potential molecular mechanisms. It is highly desirable for clinical decision-making and individualized medical treatment.
Methods
Differential expression analysis
The differential expression analysis data were obtained from TCGA, GTEx (Genotype-Tissue Expression Project), and GEPIA databases. In the TCGA database, there were 848 cases of ESCA admitted (RNA-sequencing data of HTSeq-FPKM formats), including squamous cell neoplasms, adenomas, and adenocarcinomas. Differential expression analysis was performed using the ggplot2 R package (https://www.r-project.org/). A heat map was constructed using the ComplexHeatmap R package. GEPIA contains the RNA sequencing data of 9,736 tumors and 8,587 normal tissues from e Genotype Tissue Expression (16). The global and differential stage expressions were analyzed using the GEPIA database. The study was conducted in accordance with the Declaration of Helsinki (as revised in 2013).
Molecular interactive networking
Enrichment analysis was conducted using the cluster Profiler R package and ID (IDentity) conversion was performed using the org.Hs.eg.db R package. Gene Ontology (GO) enrichment analysis was conducted to explore the functions of the targeted gene sets, including their molecular function (MF), biological process (BP), and cellular component (CC). Kyoto Encyclopedia of Genes and Genomes (KEGG) analysis was performed to explore one of the most commonly used metabolic pathway analyses. Visualization was displayed using the ggplot2 R package; the DESeq2 R package was used to screen molecules.
Functional clustering
The GeneMANIA database (http://genemania.org) was used to generate hypotheses about gene function, analyze gene lists, and prioritize genes for functional analysis. Additionally, it was also applied for gene function prediction; after searching for a specific gene, GeneMANIA identifies genes that are likely to share a function with it based on how the gene interacts with it.
A correlation heat map was constructed to demonstrate the connections between E2F family molecules using the ggplot2 R package. We drew an immune infiltration scatter plot using the GSVA R package by applying the ssGSEA (single-sample Gene Set Enrichment Analysis) algorithm.
Clinical significance
The pROC R package was used to perform data analysis, and the ggplot2 R package was used for data visualization. We estimated the area under the receiver operating characteristic (ROC) curve (AUC), sensitivity, and specificity to assess the diagnostic value of the E2F family genes for ESCA. The genes of AUC >0.7 was considered to be diagnosis-related genes for ESCA patients. Statistical analysis of the survival materials was performed using the Kaplan-Meier analysis and was achieved using the survival R package and survminer R package (P<0.05).The prognosis outcome included OS and PFI. We extracted the data including age, gender, race, pathological stage, histological type, smoking history, alcohol history, and E2F1-8, to perform univariate and multivariate analysis (P<0.05).
Results
Analysis of gene expression differences
We first analyzed the expression of E2Fs in different cancers and normal tissues using TCGA and GTEx databases. As is shown in Figure 1, significant differences were detected in E2Fs in numerous cancers. We used the GEPIA database to compare the mRNA transcription levels of E2Fs between ESCA tissues and normal esophageal tissues (Figure 2). The results suggested that E2F1, E2F3, E2F4, E2F5, E2F6, E2F7, and E2F8 were more highly expressed in ESCA tissues than in normal tissues. Low expression was only observed in E2F2.
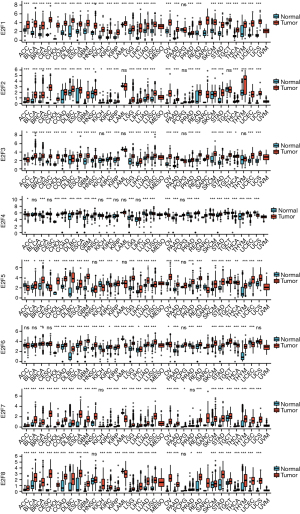
A more comprehensive comparison further highlighted the expression differences in ESCA (Figure 3). We also used the GEPIA database to analyze gene expressions that are correlated with the tumor stage. The result showed that only the expression E2F8 varied significantly [Pr(>F)=0.00856], while that of the other E2F family members did not (Figure 4). We also analyzed the gene sets of E2F1–8 using 848 gene probes, respectively, to export the heat map image (Figure 5); higher than average expression levels were marked in red, while lower than average levels were marked in blue.
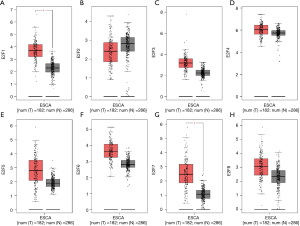
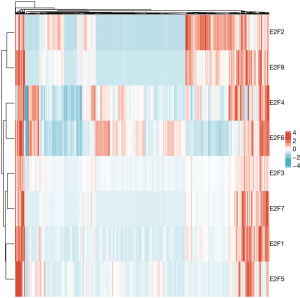
GO/KEGG enrichment analysis and gene set enrichment analysis (GSEA)
We used the GO and KEGG databases for enrichment analysis of the E2F family. As shown in Figure 5, the color of bubbles is relevant to significance; the size represents the number of enriched gene sets. It was predicted that E2F-related genes play a role in development and differentiation (Figure 6A). Furthermore, Figure 6B showed that E2F-related genes exist in several kinds of particles, such as the endoplasmic reticulum lumen, Golgi lumen, and lipoprotein particles. Translation activator activity, lipoprotein particle receptor binding, translation regulator activity, etc. may be catalyzed by these E2F-related genes (Figure 6C). Figure 6D was based on the KEGG database; we screened out seven pathways that were most closely related to E2F gene function, among which lipid metabolic signaling pathways accounted for the majority, especially the PPAR (peroxisome proliferators-activated receptor) signaling pathway.
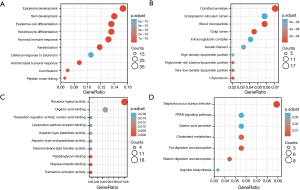
According to the NES (Normalized Enrichment Score) values in descending order (Figure 7), significant enrichment was observed in cell cycle checkpoint (NES =2.791, P. adj =0.016) (Figure 7A), DNA replication (NES =2.640, P. adj =0.016) (Figure 7B), mitotic metaphase and anaphase (NES =2.642, P. adj =0.016) (Figure 7C), and DNA repair (NES =2.506, P. adj =0.013) (Figure 7D). These gene sets were all enriched at the peak position and exhibited an up-regulated trend.
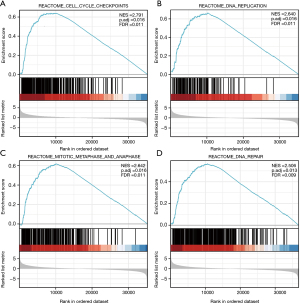
Gene correlation analysis and immune infiltration
As shown in Figure 8, red denoted a positive correlation and blue represented a negative correlation. The “*” filled-in color indicated a significant correlation between genes (P<0.05), and no fill symbolized P>0.05. The results demonstrated the following positive correlations: E2F1 with E2F2, E2F3, E2F4, E2F5, E2F7, and E2F8; E2F2 with E2F1, E2F3, E2F7, and E2F8; E2F3 with the other seven genes; E2F4 with E2F1, E2F3, E2F5, and E2F6; E2F5 with E2F1, E2F3, and E2F4; E2F6 with E2F3 and E2F4; E2F7 with E2F1, E2F2, E2F3, and E2F8; and E2F8 with E2F1, E2F2, E2F3, and E2F7. Weak negative correlations were observed between E2F6 and E2F2 and between E2F6 and E2F8.
We use the GeneMANIA database to establish the gene interaction networks. Figure 9 indicated that the network involved 502 total links between 8 E2Fs and other 20 genes. The links included shared protein domains (77.98%), physical interactions (6.35%), predicted interactions (5.27%), genetic interactions (0.02%), pathway interactions (2.13%), co-localization (6.42%), and co-expression (1.83). We identified six main genes (E2F1, E2F4, E2F7, E2F8, TFDP1, TFDP2) that are involved in functional relationships: signal transduction by the p53 class mediator (blue), signal transduction involved in the cell cycle checkpoint (pink), positive regulation of the cell cycle process (green), DNA damage response, signal transduction by the p53 class mediator (yellow), and cell cycle arrest (purple).
We compared the correlations between 24 kinds of tumor-infiltrating immune cells and the E2F family (Figure 10) and found that E2F1 is related to CD8 T cells, cytotoxic cells, mast cells, Th2 cells, etc.; E2F2 is related to CD8 T cells, iDC, macrophages, mast cells, neutrophils, Th2 cells, etc.; E2F3 is related to mast cells, Th2 cells, etc.; E2F4 is related to mast cells, pDC, Th2 cells, etc.; E2F5 is related to eosinophils, iDC, NK CD56bright cells, NK CD56dim cells, T helper cells, Tcm, Th17 cells, etc.; E2F6 is related to cytotoxic cells, Th2 cells, etc.; E2F7 is related to eosinophils, mast cells, pDC, Th17 cells, Th2 cells, etc.; E2F8 is related to NK CD56dim cells, T helper cells, Th17 cells, Th2 cells, etc. (all P<0.001). Moreover, some other tumor-infiltrating immune cells were also meaningful.
ROC diagnostic curve, survival analysis and cox analysis
ROC curves were used to assess the diagnostic accuracy for esophageal cancer based on the E2Fs. AUC values range from 0.5 to 1; the closer the AUC value is to 1, the better the diagnostic effect. However, AUCs ranging from 0.5 to 0.7 represented lower accuracy, while AUCs ranging from 0.7 to 0.9 denoted moderate accuracy. The accuracy of AUC greater than 0.9 is highest. Using the pROC and ggplot2 R packages, it was observed that in the predicted outcomes of the tumor and normal groups, E2F1 (AUC =0.945, CI: 0.890–1.000) and E2F7 (AUC =0.958, CI: 0.920–0.996) displayed higher predictive power accuracy. Also, E2F2, E2F3, E2F4, E2F5, E2F6, and E2F8 exhibited moderate accuracy (Figure 11).

The prognostic analyses are shown in Figure 12 (for OS) and Figure 13 (for PFI). We found that only E2F7 was closely related to OS [HR =1.91 (1.16–3.16), P=0.011], while the other seven genes were not. High E2F7 expression was associated with worse OS; conversely, low expression was related to better OS. None of these genes exhibited significant differences in PFI, but it was observed that the P value was the smallest and showed a trend towards statistical significance.
Based on 162 clinical cases from the TCGA, the univariate and multivariate analysis about ESCA are performed in Table 1. The cox analysis reveal that Black or African American [HR =4.286 (1.249–14.717), P=0.021], stage III [HR =4.730 (1.365–16.391), P=0.014], stage IV [HR =13.716 (3.479–54.079), P<0.001], high expression of E2F7 [HR =1.912 (1.157–3.161), P=0.011] are risk factors.
Table 1
Characteristics | Total (N) | Univariate analysis | Multivariate analysis | |||
---|---|---|---|---|---|---|
Hazard ratio (95% CI) | P value | Hazard ratio (95% CI) | P value | |||
Age (years) | 162 | |||||
≤60 | 83 | Reference | ||||
>60 | 79 | 0.831 (0.506–1.365) | 0.466 | |||
Gender | 162 | |||||
Female | 23 | Reference | ||||
Male | 139 | 2.306 (0.922–5.770) | 0.074 | 2.124 (0.601–7.506) | 0.242 | |
Race | 144 | |||||
Asian | 38 | Reference | ||||
Black or African American | 6 | 4.286 (1.249–14.717) | 0.021 | 1.240 (0.146–10.537) | 0.844 | |
White | 100 | 1.408 (0.616–3.217) | 0.417 | 1.023 (0.402–2.604) | 0.962 | |
Pathologic stage | 142 | |||||
Stage I | 16 | Reference | ||||
Stage II | 69 | 1.969 (0.581–6.676) | 0.277 | 1.653 (0.364–7.501) | 0.515 | |
Stage III | 49 | 4.730 (1.365–16.391) | 0.014 | 2.893 (0.620–13.503) | 0.177 | |
Stage IV | 8 | 13.716 (3.479–54.079) | <0.001 | 16.947 (3.036–94.583) | 0.001 | |
Histological type | 162 | |||||
Adenocarcinoma | 80 | Reference | ||||
Squamous cell carcinoma | 82 | 0.875 (0.526–1.455) | 0.607 | |||
Smoker | 144 | |||||
No | 47 | Reference | ||||
Yes | 97 | 1.539 (0.799–2.966) | 0.197 | |||
Alcohol history | 159 | |||||
No | 46 | Reference | ||||
Yes | 113 | 0.738 (0.442–1.231) | 0.245 | |||
E2F1 | 162 | |||||
Low | 81 | Reference | ||||
High | 81 | 1.178 (0.720–1.928) | 0.514 | |||
E2F2 | 162 | |||||
Low | 81 | Reference | ||||
High | 81 | 1.046 (0.640–1.711) | 0.857 | |||
E2F3 | 162 | |||||
Low | 81 | Reference | ||||
High | 81 | 0.958 (0.586–1.567) | 0.865 | |||
E2F4 | 162 | |||||
Low | 81 | Reference | ||||
High | 81 | 1.119 (0.683–1.833) | 0.656 | |||
E2F5 | 162 | |||||
Low | 81 | Reference | ||||
High | 81 | 1.082 (0.660–1.773) | 0.755 | |||
E2F6 | 162 | |||||
Low | 81 | Reference | ||||
High | 81 | 0.947 (0.577–1.555) | 0.831 | |||
E2F7 | 162 | |||||
Low | 81 | Reference | ||||
High | 81 | 1.912 (1.157–3.161) | 0.011 | 1.611 (0.782–3.317) | 0.196 | |
E2F8 | 162 | |||||
Low | 81 | Reference | ||||
High | 81 | 1.228 (0.740–2.039) | 0.426 |
ESCA, esophageal carcinoma.
Discussion
Most E2F family genes have been studied in esophageal cancer. The mechanisms and pathways of cell cycle regulation have also been reported in previous studies. This paper is the first comprehensive analysis of the expression and prognosis of the entire E2F family in esophageal cancer and aims to provide a further basis for the accurate prognostic prediction of esophageal cancer patients and explore possible target pathways, thereby providing some new ideas concerning diagnosis and treatment.
A maximum of E2F1 has been studied in previous studies. It has been confirmed that E2F1 exerts a suppressive effect in esophageal adenocarcinomas (17) and a cancer-promoting effect in esophageal squamous cell cancer (18). In recent years, E2F1-related pathways have been studied extensively. Zhang et al. reported that E2F1 binding to miR-26b could increase the expression of miR-26b, thereby inhibiting the proliferation of ESCC (6). A 2020 result from TCGA showed that E2F1 is a potential regulator with good diagnostic and prognostic values in ESCA (19). Song et al. suggested that E2F1 is the direct target gene of miR-622, which plays a functional tumor suppressor role in ESCC (8). A recent result demonstrated that E2F1 inhibits miR-375 expression in ESCC and promotes SESN3 expression, thereby activating the PI3K/AKT pathway (20). Another paper reported that the abnormal expression of NSUN2 is positively regulated by E2F1; higher NSUN2 levels predict poorer survival in ESCC patients (21). However, the overexpression of miR-25 promotes the invasion and metastasis of ESCC cells (22). In our study, E2F1 was found to be differentially expressed but had no prognostic significance for OS and PFI. Compared to the previous different conclusions, maybe different pathological types and samples cause the opposite results.
At present, research about E2F2 has rarely been reported. A 2020’s study points out that the miR-17-92a cluster and E2Fs (E2F1, E2F2, E2F3) create a cellular balance between apoptosis and proliferation. MiR-18a-5p, which belongs to the miR-17-92a cluster, is a poor prognostic biomarker in ESCA (23). MiR-98 and miR-363 are associated with esophageal cancer and regulate a sequence of cell cycle-related genes, including E2F1 and E2F2 (10). In ESCC patients, a previous RT-PCR analysis revealed that expression of E2F2, which is a known miR-31 target oncogene, was negatively connected with the expression of miR-31 in a p21-dependent manner, and overexpression of miR-31 resulted in a better OS in ESCC patients (24). In our study, low expression was only observed in E2F2, but no significant differences were found in OS and PFI.
The overexpression of E2F3 is associated with worse esophageal cancer outcomes. RACGAP1 is a transcription target of E2F3; E2F3 inhibition can down-regulate the mRNA expression of RACGAP1, leading to extensive apoptosis (11). In addition to the miR-17-92a cluster mentioned above, high levels of β-catenin have been found to activate the expression of E2F3. Moreover, DHODH (dihydroorotate dehydrogenase) is positively correlated to β-catenin, and high DHODH expression induces a worse prognosis (25). The new circ_0087378 in ESCC can bind to miR-140-3p to up-regulate the expression of E2F3 and eventually induces poorer outcomes in ESCC (26). In a 2019 study, Lv et al. showed that E2F3 is up-regulated in esophageal adenocarcinoma but was not related to prognosis (27). Another study conducted in 2021 reported new crosstalk of the oncogenic role of circ_0000654/mir-375/E2F3 ceRNA (competing endogenous RNA) in ESCC and established the concept that targeting circ_0000654 and its pathways may improve the prognosis of ESCC (28). Another report found that silencing circFIG 4 can mitigate EC malignant progression by mediating the miR-493-5p/E2F3 pathway and may become a new biomarker and therapeutic target for EC treatment (29). Our results illustrated that there were no statistical differences in the OS and PFI of E2F3. However, E2F3 was differentially expressed in both tumor and normal tissues.
E2F4 has been reported to play a role in the functional proliferation of gastric cancers. LINC00337 can recruit E2F4 to enhance the transcription of TPX2(a microtubule nucleation factor), thus promoting cell autophagy. Silencing of LINC00337 enhances apoptosis in ESCC (12). A previous study indicated that the E2F4 methylation exists in the promoter regions of EAC (esophageal adenocarcinoma) and ESCC, which leads to the inhibition of LTBP4 (latent transforming growth factor beta binding protein 4) and promotes migration (30). In this paper, the expression of E2F4 was higher in tumor tissues compared to normal tissues, but there were no differences in the stages and survival analysis.
E2F5 enables increased cell proliferation and migration and inhibits apoptosis. In ESCC, miR-34a has a direct effect on E2F5, and low miR-34a expression promotes apoptosis in ESCC (31). In contrast, miR-544 is negatively correlated with E2F5; its overexpression induces ESCC proliferation (32). A previous study pointed out that E2F5 was a biomarker for poor prognosis in ESCC patients (13). Our results showed that E2F5 was not related to the prognosis of EC.
At present, there are no studies about E2F6 and esophageal cancer. Yang et al. demonstrated that E2F6 inhibits the trans-activation and apoptosis of E2F1 by competing with E2F1 for DNA binding sites, and E2F6 plays a role in hypoxia-induced apoptosis by regulating E2F1 (33). Silencing E2F7 reduces the proliferation, migration, and invasion of EC cells, and induces apoptosis. The effects of lncRNA (long non-coding RNA) DLEU2 on the proliferation, migration, and invasion of EC cells are reversed by miR-30E-5P inhibitors or the up-regulation of E2F7 (14). E2F8 is up-regulated to promote cell proliferation and affect cyclin D1 (CCND1)/P21 expression in ESCC. Down-regulation of E2F8 expression inhibits cell proliferation in vivo (15). Our study confirmed that E2F7 is correlated with OS and PFI (P<0.05). E2F7 was the only transcription factor that we validated as statistically significant.
Our results also illustrated that esophageal cancer is closely related to lipid metabolism, especially the PPAR signaling pathway. According to the studies mentioned above, E2Fs are related to esophageal cancer through various miRNA signaling pathways. MiRNA is a key regulator of lipid metabolism (34), which is involved in the regulation of numerous cellular processes, including cell growth, proliferation, differentiation, survival, apoptosis, inflammation, motility, membrane homeostasis, chemotherapy response, and drug resistance (35). A previous animal experiment showed that cholesterol stimulates the proliferation of ECA109 cells both in vivo and in vitro (36). Several reports have confirmed that the PPAR-γ pathway is correlated with the development of esophageal cancer. A 2020 study demonstrated that miRNA-mediated PPAR-γ pathway inhibition enhances the proliferation of ESCC (37). Nevertheless, the increased expression of PPAR-γ may play an important role in the development and progression of normal cell transformation to Barrett's esophagus and esophageal adenocarcinoma (38). Combined with the above studies, we speculate that lipid metabolism plays an important role in the generation of EC.
In summary, our study provides some possible prognostic biomarkers and mechanisms, which could lead to the development of novel targeted drugs. Through molecular typing of esophageal cancer patients, accurate prognostic prediction in individual patients will gain further improvement. Therefore, more meaningful approaches will be explored.
Conclusions
This study demonstrated that E2F7 is a prognostic biomarker. Also, the E2F family plays a role in esophageal cancer formation through lipid metabolism pathways.
Acknowledgments
The authors are grateful for the invaluable support and useful discussions with other members of the Department of Medical Oncology.
Funding: None.
Footnote
Conflicts of Interest: All authors have completed the ICMJE uniform disclosure form (available at https://jgo.amegroups.com/article/view/10.21037/jgo-22-855/coif). The authors have no conflicts of interest to declare.
Ethical Statement: The authors are accountable for all aspects of the work in ensuring that questions related to the accuracy or integrity of any part of the work are appropriately investigated and resolved. The study was conducted in accordance with the Declaration of Helsinki (as revised in 2013).
Open Access Statement: This is an Open Access article distributed in accordance with the Creative Commons Attribution-NonCommercial-NoDerivs 4.0 International License (CC BY-NC-ND 4.0), which permits the non-commercial replication and distribution of the article with the strict proviso that no changes or edits are made and the original work is properly cited (including links to both the formal publication through the relevant DOI and the license). See: https://creativecommons.org/licenses/by-nc-nd/4.0/.
References
- Manicum T, Ni F, Ye Y, et al. Prognostic values of E2F mRNA expression in human gastric cancer. Biosci Rep 2018;38:BSR20181264. [Crossref] [PubMed]
- Zhou Q, Zhang F, He Z, et al. E2F2/5/8 Serve as Potential Prognostic Biomarkers and Targets for Human Ovarian Cancer. Front Oncol 2019;9:161. [Crossref] [PubMed]
- Huang YL, Ning G, Chen LB, et al. Promising diagnostic and prognostic value of E2Fs in human hepatocellular carcinoma. Cancer Manag Res 2019;11:1725-40. [Crossref] [PubMed]
- Secrier M, Li X, de Silva N, et al. Mutational signatures in esophageal adenocarcinoma define etiologically distinct subgroups with therapeutic relevance. Nat Genet 2016;48:1131-41. [Crossref] [PubMed]
- NCCN Clinical Practice Guidelines in Oncology-Esophageal and Esophagogastric Junction Cancers (Version 1.2022) [DB/OL]. Available online: http://www.nccn.org
- Zhang K, Zhang B, Bai Y, et al. E2F1 promotes cancer cell sensitivity to cisplatin by regulating the cellular DNA damage response through miR-26b in esophageal squamous cell carcinoma. J Cancer 2020;11:301-10. [Crossref] [PubMed]
- Zhang K, Dai L, Zhang B, et al. miR-203 is a direct transcriptional target of E2F1 and causes G1 arrest in esophageal cancer cells. J Cell Physiol 2015;230:903-10. [Crossref] [PubMed]
- Song C, Lu P, Shi W, et al. MiR-622 functions as a tumor suppressor and directly targets E2F1 in human esophageal squamous cell carcinoma. Biomed Pharmacother 2016;83:843-9. [Crossref] [PubMed]
- Li M, Zhao J, Li X, et al. HiFreSP: A novel high-frequency sub-pathway mining approach to identify robust prognostic gene signatures. Brief Bioinform 2020;21:1411-24. [Crossref] [PubMed]
- Du J, Zhang L. Analysis of salivary microRNA expression profiles and identification of novel biomarkers in esophageal cancer. Oncol Lett 2017;14:1387-94. [Crossref] [PubMed]
- Zhao W, Wang M, Wang C, et al. RACGAP1 is transcriptionally regulated by E2F3, and its depletion leads to mitotic catastrophe in esophageal squamous cell carcinoma. Ann Transl Med 2020;8:950. [Crossref] [PubMed]
- Yang C, Shen S, Zheng X, et al. Long non-coding RNA LINC00337 induces autophagy and chemoresistance to cisplatin in esophageal squamous cell carcinoma cells via upregulation of TPX2 by recruiting E2F4. FASEB J 2020;34:6055-69. [Crossref] [PubMed]
- Ishimoto T, Shiozaki A, Ichikawa D, et al. E2F5 as an independent prognostic factor in esophageal squamous cell carcinoma. Anticancer Res 2013;33:5415-20. [PubMed]
- Lu T, Wang R, Cai H, et al. Long non-coding RNA DLEU2 promotes the progression of esophageal cancer through miR-30e-5p/E2F7 axis. Biomed Pharmacother 2020;123:109650. [Crossref] [PubMed]
- Chang H, Song J, Wu J, et al. E2F transcription factor 8 promotes cell proliferation via CCND1/p21 in esophageal squamous cell carcinoma. Onco Targets Ther 2018;11:8165-73. [Crossref] [PubMed]
- Tang Z, Li C, Kang B, et al. GEPIA: a web server for cancer and normal gene expression profiling and interactive analyses. Nucleic Acids Res 2017;45:W98-W102. [Crossref] [PubMed]
- Evangelou K, Kotsinas A, Mariolis-Sapsakos T, et al. E2F-1 overexpression correlates with decreased proliferation and better prognosis in adenocarcinomas of Barrett oesophagus. J Clin Pathol 2008;61:601-5. [Crossref] [PubMed]
- Fujita Y, Sakakura C, Shimomura K, et al. Chromosome arm 20q gains and other genomic alterations in esophageal squamous cell carcinoma, as analyzed by comparative genomic hybridization and fluorescence in situ hybridization. Hepatogastroenterology 2003;50:1857-63. [PubMed]
- Xu Z, Wu Z, Zhang J, et al. Development of Multiscale Transcriptional Regulatory Network in Esophageal Cancer Based on Integrated Analysis. Biomed Res Int 2020;2020:5603958. [Crossref] [PubMed]
- Li P, Lv H, Wu Y, et al. E2F transcription factor 1 is involved in the phenotypic modulation of esophageal squamous cell carcinoma cells via microRNA-375. Bioengineered 2021;12:10047-62. [Crossref] [PubMed]
- Su J, Wu G, Ye Y, et al. NSUN2-mediated RNA 5-methylcytosine promotes esophageal squamous cell carcinoma progression via LIN28B-dependent GRB2 mRNA stabilization. Oncogene 2021;40:5814-28. [Crossref] [PubMed]
- Wang M, Yang YO, Jin Q, et al. Function of miR-25 in the invasion and metastasis of esophageal squamous carcinoma cells and bioinformatical analysis of the miR-106b-25 cluster. Exp Ther Med 2018;15:440-6. [PubMed]
- Kolenda T, Guglas K, Kopczyńska M, et al. Good or not good: Role of miR-18a in cancer biology. Rep Pract Oncol Radiother 2020;25:808-19. [Crossref] [PubMed]
- Ning Z, Zhu H, Li F, et al. Tumor suppression by miR-31 in esophageal carcinoma is p21-dependent. Genes Cancer 2014;5:436-44. [Crossref] [PubMed]
- Qian Y, Liang X, Kong P, et al. Elevated DHODH expression promotes cell proliferation via stabilizing β-catenin in esophageal squamous cell carcinoma. Cell Death Dis 2020;11:862. [Crossref] [PubMed]
- Wang J, Wang Q, Gong Y, et al. Knockdown of circRNA circ_0087378 Represses the Tumorigenesis and Progression of Esophageal Squamous Cell Carcinoma Through Modulating the miR-140-3p/E2F3 Axis. Front Oncol 2020;10:607231. [Crossref] [PubMed]
- Lv J, Guo L, Wang JH, et al. Biomarker identification and trans-regulatory network analyses in esophageal adenocarcinoma and Barrett's esophagus. World J Gastroenterol 2019;25:233-44. [Crossref] [PubMed]
- Luo C, Zhao X, Wang Y, et al. A novel circ_0000654/miR-375/E2F3 ceRNA network in esophageal squamous cell carcinoma. Thorac Cancer 2022;13:2223-34. [Crossref] [PubMed]
- Huang Z, Wang C, Zhao X. circFIG 4 drives the carcinogenesis and metastasis of esophagus cancer via the miR-493-5p/E2F3 axis. Thorac Cancer 2022;13:783-94. [Crossref] [PubMed]
- Bultmann I, Conradi A, Kretschmer C, et al. Latent transforming growth factor β-binding protein 4 is downregulated in esophageal cancer via promoter methylation. PLoS One 2013;8:e65614. [Crossref] [PubMed]
- Jiang H, Guo Y, Huang K, et al. MicroRNA-34a inhibits esophageal squamous cell carcinoma progression by targeting E2F5. J BUON 2019;24:2514-22. [PubMed]
- Sun F, Zhang C, Ma D, et al. MicroRNA-544 inhibits esophageal squamous cell carcinoma cell proliferation and enhances sensitivity to cisplatin by repressing E2F transcription factor 5. Oncol Lett 2019;18:4203-9. [Crossref] [PubMed]
- Yang WW, Shu B, Zhu Y, et al. E2F6 inhibits cobalt chloride-mimetic hypoxia-induced apoptosis through E2F1. Mol Biol Cell 2008;19:3691-700. [Crossref] [PubMed]
- Aryal B, Singh AK, Rotllan N, et al. MicroRNAs and lipid metabolism. Curr Opin Lipidol 2017;28:273-80. [Crossref] [PubMed]
- Huang C, Freter C. Lipid metabolism, apoptosis and cancer therapy. Int J Mol Sci 2015;16:924-49. [Crossref] [PubMed]
- Li C, Shi Y, Lin Y. Effects of 27-hydroxycholesterol and cholesterol on the proliferation of esophageal squamous cell carcinoma in nude mice and human esophageal carcinoma cells (ECA109). Journal of Shandong University (Health Sciences) 2020;58:45-52.
- Wu K, Hu Y, Yan K, et al. microRNA-10b confers cisplatin resistance by activating AKT/mTOR/P70S6K signaling via targeting PPARγ in esophageal cancer. J Cell Physiol 2020;235:1247-58. [Crossref] [PubMed]
- Wang W, Wang R, Zhang Z, et al. Enhanced PPAR-gamma expression may correlate with the development of Barrett's esophagus and esophageal adenocarcinoma. Oncol Res 2011;19:141-7. [Crossref] [PubMed]
(English Language Editor: A. Kassem)