Construction and validation of a m6A RNA methylation and ferroptosis-related prognostic model for pancreatic cancer by integrated bioinformatics analysis
Introduction
The American Cancer Society estimates that 608,570 people will die of cancer and 1,898,160 people will be diagnosed with cancer in the United States in 2021. Due to continued advancements and developments in technology, economic standards, and the means of preventing and treating cancer worldwide, after a long period of sustained peaks, there was a downward trend in mortality rates from 1991 to 2018 (1). However, the number of patients diagnosed with pancreatic cancer has increased dramatically in recent years. Pancreatic cancer is one of the foremost malignant gastrointestinal tumors, with prognosis and postoperative prediction remaining challenging because of the lack of facile, sensitive diagnostic methods and a specific single biomarker. Tumor markers (such as CA199, CEA, etc.) are often used in the diagnosis of pancreatic cancer, but their sensitivity and specificity in early tumor diagnosis are poor. Combined-biomarker analysis which provides a promising strategy to conquer such dilemma still requires developments in methodologies to gain accurate and reliable outcomes (2). Further, more and more people are dying from various types of cancer. This is due to a variety of factors, including genetics, smoking, an unhealthy diet, obesity, excessive alcohol consumption, and an aging population (3).
Research has shown that genetic mutations, such as INK4A, KRAS, TP53 and SMAD4, drive the malignant transformation of normal pancreatic cells into cancer pancreatic cells, accelerating cell proliferation and metastasis, and thus exacerbating the malignancy of pancreatic cancer (4,5). However, the malignant transformation and recurrent genetic alterations in the cellular process of pancreatic cancer cells possess remarkable characteristics, such as a high malignancy, early diagnosis difficulties, a poor prognosis, and immunotherapy resistance, and have created issues in the whole treatment process of pancreatic cancer. Thus, research on pancreatic cancer needs to be conducted to establish more reliable and effective scientific methods for identifying prognosis indicators and therapeutic targets (6).
As a reversible post-transcription modification in eukaryotes, ribonucleic acid (RNA) methylation commonly occurs in N6-methyladenosine (m6A), 2’-O-methylation, and N1-methyladenosine (7). Notably, the m6A modification, which refers to a methylation modification formed by a nitrogen atom, is the most prevalent and significant epigenetic modification in messenger RNA (mRNA). M6A modification is mainly induced by 3 kinds of proteases. The first type of proteins are m6A methyltransferases encoded with writers, including METTL3, METTL16, and WTAP (8), which form complexes that collectively promote the writing of m6A methylated groups into RNA. The second type of proteins are m6A demethylases, whose encoding genes are called erasers. Typical erasers include ALKBH5 and FTO. Erasers can removed m6A modifications out of RNA and thus affect tumor biology. The third group of proteins is involved in the information reading function of m6A methylation. The most common readers include eIF3, YTHs, hnRNP A2/B1, and hnRNPC (9). The readers bind to m6A sites in the nuclei of cells, and thus perform specific biological functions. For example, they play a crucial role in manipulating normal biological processes, such as splicing, transport, transcription, and translation. Their dysregulation is closely linked to the development of malignant tumors. Research on the molecular biology mechanism of m6A modification in tumors is in the early stages, but scientists intend to explore how m6A regulates cancer progression by remodeling transcriptional products and to determine the potential value of m6A in human cancer diagnosis and treatment through the analysis and integration of a large number of existing basic data resources.
Ferroptosis differs to apoptosis. In essence, ferroptosis refers to programmed cell death, cell necrosis, and cell autophagy, and is iron-dependent. The main mechanism of ferroptosis is to catalyze a substance called lipid peroxidation on the cell membrane in the presence of divalent iron or ester oxygenase, which induces cell death (10). There is a prominent morphological characteristic of ferroptosis in the shrinkage of mitochondria and an increase in mitochondrial membrane density. Cysteine utilization, glutathione biosynthesis, polyunsaturated fatty acid metabolism, and the regulation of phospholipids are the key factors of ferroptosis (11,12). The cystine/glutamate retrotransporter and System Xc- (a cystine/glutamate antiporter system) mediate the production of glutathione (GSH), can be the promising targets in cancer cells for the induction of ferroptosis.
GSH, which is an important intracellular antioxidant, is a negative regulator of ferroptosis (13). GSH/glutathione peroxidase 4 inhibits lipoxygenase activity, scavenges the lipid peroxidation generated by iron aggregation, effectively counteracts lipid bilayer lipid peroxidation, and prevents cell membrane damage (14,15). Research on the ferroptosis mechanism has shown that the promotion of ferroptosis in cancer cells inhibits cancer cell differentiation and migration and improves tumor drug resistance, which has become a new strategy for cancer intervention therapy (16).
As a result of breakthroughs in high-throughput sequencing technology and extensive research in the field of oncology, combined analysis models of multiple phenotypes have become popular in predicting tumor treatment and have been proven to be better than analyses that rely on a single phenotype or a single histology of a tumor disease (17). Indeed, m6A and ferroptosis have become a popular area in the field of oncology research, and their important roles in both tumor development and treatment have been widely recognized by scientists. In this study, we sought to find the fit between the independent analysis of the 2 mechanisms to construct a visual bioinformatic model to explore possible prognostic diagnostic markers and future therapeutic targets of pancreatic cancer in a more comprehensive and multidimensional manner. We present the following article in accordance with the TRIPOD reporting checklist (available at https://jgo.amegroups.com/article/view/10.21037/jgo-22-941/rc).
Methods
Collection of the PAAD data set
To download the data set from The Cancer Genome Atlas (TCGA), gene expression RNA sequencing data were acquired from the authentic Genomic Data Commons (GDC) website (https://portal.gdc.cancer.gov/) using the R package TCGA biolink (18). We also acquired the relative clinical database and specific samples for TCGA–Pancreatic Adenocarcinoma (PAAD). We obtained RNA sequencing data from the Gene Expression Omnibus (GEO) database, including GSE107610 (19), GSE62165 (20) and GSE16515 (21). The study was conducted in accordance with the Declaration of Helsinki (as revised in 2013).
Identifying the DEGs between the PAAD tissues and normal adjacent tissues
Batch effects in the GEO data sets were eliminated using the R package SVA (22). We used the R package limma to identify the differentially expressed genes (DEGs) (23). To identify the DEGs among the tissues, moderated t-tests applying the Bayesian model were used to evaluate the changes in gene expression. The DEGs were selected based on the significance criteria (an adjusted P value <0.05 was considered statistically significant) with the R package limma. Volcano plots and heatmaps were constructed to display the gene expression profiles between the related genes.
Selection of m6A RNA methylation regulators
In total, 13 genes (i.e., FTO, METTL3, ZC3H13, KIAA1429, HNRNPC, METTL14, YTHDC1, ALKBH5, RBM15, YTHDC2, YTHDF1, YTHDF2, and WTAP) were considered the major m6A RNA methylation regulators. To ensure the specificity and comprehensiveness of this study, we also added 11 other m6A RNA methylation regulators that have been identified in recent years (i.e., LRPPRC, METTL16, IGF2BP3, RBM15B, YTHDF3, IGF2BP1, IGF2BP2, CBLL1, ELAVL1, FMR1, and HNRNPA2B1). All the clinical information for the genes were obtained from TCGA-PAAD cohort. To identify the m6A RNA methylation regulators in the PAAD cohort, a clustering consensus analysis was conducted using the R package ConsensusClusterPlus (24). We observed 2 subgroups in the PAAD cohort. Next, we used the heatmaps and volcano plots to visualize the expressions of the RNA methylation regulators in the 2 subgroups. We used the Kyoto Encyclopedia of Genes and Genomes (KEGG) and Gene Ontology (GO) databases to identify the pathways involved and explore the potential function of related genes (25).
Selection of ferroptosis regulators
According to a previous study, 259 genes appear to be ferroptosis regulators. The ferroptosis markers were obtained from FerrDb (http://www.zhounan.org/ferrdb/). We also incorporated 77 newly acknowledged ferroptosis regulator genes and removed several non-human genes from the FerrDb (26). The specific expression of genes and clinical information were extracted from TCGA–PAAD data set. Consensus clustering analysis was performed to analyze the ferroptosis regulators in the PAAD cohort, and 2 subgroups were identified. Next, the R package limma was employed to compare the relevant DEGs in these 2 subgroups. Heatmaps and volcano plots were constructed to visualize the DEGs. Based on the DEGs in these 2 groups, KEGG and GO functional enrichment analyses were conducted to identify the related pathways and the potential function of the ferroptosis regulators.
Prognostic signature generation
The ferroptosis regulators and m6A RNA methylation regulators that had a significant effect on the prognosis of the PAAD patients were included in the prognostic signature. A univariate Cox regression model was used to determine the relevance between these genes and the prognosis of the PAAD patients. If a gene had a hazard ratio (HR) value >1, it was considered as a protective gene. In total, 3 protective genes and 14 risk genes met the criteria. Next, the risk score was calculated from the coefficients using the least absolute shrinkage and selection operator (LASSO) algorithm based on these genes. Consequently, the risk score was used to classify patients in TCGA-PAAD cohort into high- and low-risk groups based on the median value.
Evaluating the prognostic value of the gene signature for the pancreatic cancer patients
Age, gender, and lifestyle were the main clinicopathological features of pancreatic cancers. The distribution of the clinicopathological features (age, gender, grade stage, and survival state) was further evaluated for the high-and low-risk groups calculated by chi-square test and visualized with heatmaps. By applying the receiver operating characteristic (ROC) curve, the prognostic value of each characteristic in predicting the survival rate of the patients was assessed. A Kaplan-Meier analysis and logarithmic ranking measurement were used to compute the overall survival (OS) differences between the patients in the 2 groups using training sets and validation sets.
Statistical analysis
To standardize the data analysis, R Bioconductor and R (version 3.6.1) software were used for the statistical analysis. Fisher’s exact test and the chi-square (χ2) test were used to examine the differences between the 2 groups. We calculated the risk score based on the coefficients in the LASSO algorithm. The Kaplan-Meier method was used for the survival analysis. The subgroups survival curve of each data set was generated and the log-rank test was used to demonstrate the statistically significant differences. The quantitative real-time–polymerase chain reaction (qRT-PCR) data are presented as the mean ± standard deviation (SD), which were calculated by PRISM 6 software (GraphPad Software, Inc.) from 3 independent experiments. The student’s t-test was used to compare the 2 data sets. A P value <0.05 was considered statistically significant.
Cell cultures
Pancreatic cancer cell lines (i.e., PANC-1, BXPC-3, and CFPAC-1) were purchased from Wuhan, China (Procell Life Science and Technology Co., Ltd.). The normal pancreatic ductal esophageal cell line (hTERT-HPNE) was purchased from BeNa Culture Collection. The hTERT-HPNE and PANC-1 cell lines were cultured in Dulbecco’s Modified Eagle Medium (HyClone) supplemented with 10% fetal bovine serum (FBS) (Gibico) containing 1% penicillin-streptomycin (New Cell and Molecular Biotech Co., Ltd.) in an incubator at 37 ℃ with 5% carbon dioxide (CO2). The BXPC-3 cell line was cultured in Roswell Park Memorial Institute 1640 medium (HyClone) supplemented with 10% FBS (Gibico) containing 1% penicillin-streptomycin (New Cell and Molecular Biotech Co., Ltd.) in an incubator at 37 ℃ with 5% CO2. The CFPAC-1 cell line was cultured in Iscove’s Modified Dulbecco Medium (Gibico) supplemented with 10% FBS (Gibico) containing 1% penicillin-streptomycin (New Cell and Molecular Biotech Co., Ltd.) in an incubator at 37 ℃ with 5% CO2.
RNA extraction and qRT-PCR
The total RNA from the cells was extracted by TRIzol reagent (Invitrogen) and converted into complementary deoxyribonucleic acid using a Reverse Transcription kit (Vazyme). The qRT-PCR was conducted in triplicate using the SYBR-Green PCR Master Mix kit (Vazyme). The follow primers were used: ENPP2 forward 5'- TCAGCCTGCCGACAAGTGT-3', and reverse, 5'- TTCTACCCATTTTGATTCGTCC-3'; IGF2BP2 forward 5'- CAGATGAGACCAAACTAGCCGAA-3', and reverse, 5'- TCAGTCTTCCAACCAAGCCATT-3'; ATP6V1G2 forward 5'- TTCCAAGTCATTCTCACCTAAACC-3', and reverse, 5'- CCAGACACTGAAGTCAGGATGTT-3'; ITGB4 forward 5'- GGCAACATCCATCTGAAACCT-3', and reverse, 5'- CACACTGTCCGCACACGAA-3'; PROM2 forward 5'- TGGTGTGAGCATTGGGAGC-3', and reverse, 5'- GCATTCAAGGTTTGCAGGTG-3'; IGF2BP3 forward 5'- CAGATGCCAAACCAAAGACAG-3', and reverse, 5'- CTCACAGAGACAGGAGTTCAAAAGT-3'; METTL16 forward 5'- TGGTCATCAGTAACAACAGAAAGC-3', and reverse, 5'- CACACTGTCCGCACACGAA'.
Results
The expression features of the PAAD tissues
Figure 1 shows the DEGs in the normal adjacent tissues and PAAD tissues. In total, 1,668 DEGs were found in the PAAD tissues (Figure 1A). Heatmaps were constructed to display the top 20 ferroptosis-related and the top 10 m6A-related DEGs (Figure 1B). The GO and KEGG functional enrichment analysis was carried out on the basis of the DEGs. The GO analysis showed that the DEGs were involved in a variety of processes, including cellular zinc ion homeostasis, and divalent inorganic cation homeostasis. The KEGG analysis revealed correlations between the cancer tissues and pancreatic secretion, focal adhesion, and glycine, serine, and threonine metabolism (Figure 1C). Further, the Gene Set Enrichment Analysis (GESA) showed that the function of DEGs was significantly enriched in the cell surface receptor signaling pathway, and the regulation of cell cycle process (Figure 1D).
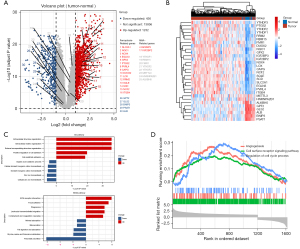
Correlation of m6A RNA methylation regulators with the prognosis of PAAD patients
To establish a prognostic signature of the characteristic m6A RNA methylation regulators, 177 patients were classified by a consensus clustering analysis. Through the cumulative distribution function (CDF) value, we found the most appropriate cluster number to classify the patients in the PAAD cohort into 2 groups. We also performed a principal component analysis (PCA) of the m6A RNA expression profile, and the results showed 2 significant clusters between group1 and group 2 differed (Figure 2A). We also assessed the associations between the groups and clinicopathological features. As Figure 2B shows, IGF2BP1, IGF2BP2 and IGF2BP3 had higher numerical values in group 2 than group, while the expression of METTL16 was lower. Notably, group 2 was significantly correlated with the survival outcome. The DEGs for the 2 groups are displayed in a volcano plot (Figure 2C). We conducted GO and KEGG analyses to examine the endowed functions and pathways in group 2. The GO analysis showed that the DEGs were involved in a number of different types of processes, including epidermis development, skin development, signal release, and the modulation of chemical synaptic transmission. Additionally, the KEGG analysis revealed an internal relationship between m6A RNA methylation and extracellular matrix (ECM)-receptor interaction, absorption, nicotine addiction, and insulin secretion (Figure 2D). A GSEA analysis was also conducted, and the results suggested that the gene expression profile was functionally enriched in epithelial cell migration, the immune response, and the regulation of RNA metabolic process (Figure 2E). However, the OS of patients in group 2 was lower than that of patients in group 1 (Figure 2F).
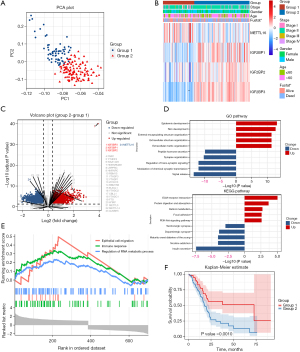
Correlation between the ferroptosis regulators and the prognosis of the PAAD patients
To establish a prognostic signature comprising the characteristic m6A RNA methylation regulators, 177 patient samples were stratified for a consensus clustering analysis. Based on the CDF value, we found that a k value of 2 was the most appropriate cluster number for classifying the patients in the PAAD cohort into groups 1 and 2. To further demonstrate the classification, a PCA based on gene expression was conducted, and the result showed significant clusters between group 1 and group 2 (Figure 3A). Next, we evaluated the relationship between the groups and the clinicopathological features in the TCGA data set. As Figure 3B shows, the 29 ferroptosis-related genes differed significantly between the 2 groups (Figure 3B). Further, group 2 had a close correlation with survival state and advanced stage. The DEGs in the 2 groups were visualized by a volcano plot (Figure 3C). We conducted GO and KEGG analyses of the DEGs to identify the functions and pathways enriched in group 2. The GO analysis showed that the DEGs were involved in different kinds of processes, including epidermis development, skin development, signal release, and the modulation of chemical synaptic transmission. The KEGG analysis showed that clustering was internally related to neuroactive ligand-receptor interaction, cell adhesion molecules, retinol metabolism, and xenobiotic metabolism (Figure 3D). The GSEA analysis showed that several pathways were significantly enriched, including cellular metal ion homeostasis, lipid catabolic process, and tumor necrosis factor production (Figure 3E). However, the OS of the patients in group 2 was lower than that of the patients in group 1 (see Figure 3F).
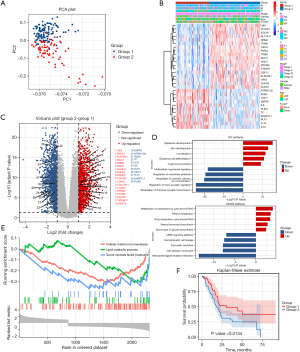
Prognostic significance of the signature based on the m6a RNA methylation and ferroptosis regulators
As shown above, 115 of the 177 samples were clustered into the same group by the M6A- and ferroptosis-related DEGs. We merged these genes to predict patient prognosis and survival. As Figure S1 shows, the DEGs related to m6A and ferroptosis differed between the normal and tumor tissues in the GEO data sets, which supported the selection of the genes. The potential performance of the prediction model based on the m6A RNA methylation and ferroptosis regulators was estimated in the PAAD patients. Both Kaplan-Meier curve and the univariate Cox regression analyses showed that 3 of the regulators were related to good survival (see Figure 4A); that is, METTL16, ATP6V1G2, and ENPP2. Conversely, IGF2BP3, IGF2BP2, CA9, PVRL4, ALDH3A1, MUC1, ITGB4, SLC2A1, IL6, PROM2, DUOX2, CAPG, RRM2, and ITGA6 were found to be risk factors for the PAAD patients.
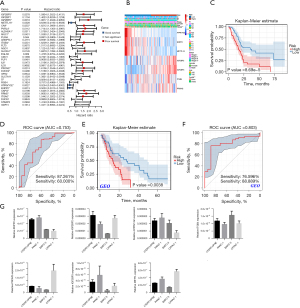
Next, the LASSO algorithm was used to construct the prognostic signature. A coefficient profile plot was constructed, with the best log2 transformed lambda value determined by the smallest likelihood deviance. In the PAAD cohort, 7 regulators (i.e., ENPP2, IGF2BP2, ATP6V1G2, ITGB4, PROM2, IGF2BP3, and METTL16) and related coefficients were calculated using the 10-fold cross-validated method. The risk factor of the patients was calculated as follows: sum of gene expression × coefficient. Based on the median risk score, we classified the patients in the PAAD cohort into low- and high-risk groups. A high-risk score was positively correlated with an aggressive pathology of T stage, and survival status (see Figure 4B and Table S1). The high-risk patients had a shorter OS than the low-risk patients (P<0.001; see Figure 4C). As Figure 4D shows, the signature’s risk score could generally predict the survival rates of the PAAD patients (area under the curve =0.753). We also estimated the risk elements in the GEO data sets. Notably, a high-risk score indicated poor survival among the PAAD patients in the GEO data sets (Figure 4E,4F).
Further, we observed the mRNA levels of these 7 regulators (i.e., ENPP2, IGF2BP2, ATP6V1G2, ITGB4, PROM2, IGF2BP3, and METTL16) in the PANC-1, BXPC-3, CFPAC-1, and hTERT-HPNE cell lines. We found these 7 regulators were differently expressed in the pancreatic cancer cells and normal pancreatic ductal esophageal cells (Figure 4G). The question of how regulators affect the progression and prognosis of pancreatic cancer patients and by what mechanism requires further study.
Discussion
Pancreatic cancer is difficult to diagnose, highly malignant, and has poor overall survival rates. Many studies and analyses have found differences in the survival rates of pancreatic cancer patients. For example, research has shown that ferroptosis and methylation-related gene alterations have a significant effect on cancer diagnosis, prognosis, and treatment. They can be regarded as molecular biomarkers for the diagnosis, prognosis, and treatment of pancreatic cancer. A growing body of evidence has established effective prognostic models for the core genes and how they function in pancreatic cancer, such as the molecules related to oxidative stress, autophagy, and immune infiltration; however, most of these models are based on a single set of histological data. Tumors are complex diseases that plague human health, and involve very sophisticated life science mysteries. A single functional phenotype or a single level of data mining cannot accurately analyze tumor prognostic assessments. Thus, this study sought to combine 2 popular research areas in the field of tumor research (i.e., methylation and ferroptosis) to establish a joint prognostic assessment model and achieved good results. Compared to previous prognostic models established by a single functional phenotype, this joint model assesses patients’ prognostic risk more comprehensively. Further, this model was shown to have high stability and reproducibility and thus provides a good sight for the diagnosis and treatment of pancreatic cancer. Our findings also provide novel ideas for the treatment and prognosis assessment of pancreatic cancer, which is known to be a difficult tumor with highly malignant.
Methylation and ferroptosis are also research hotspots in studies related to gastrointestinal (GI) tumors. Methylation has a significant effect on the development of pancreatic cancer. Wang et al. found that the upregulation of MetT14 affects Perp mRNA n adenosine methylation, and also contributes to the metastasis of pancreatic cancer (27). Researchers have also explored the relationship between methylation and different subtypes of pancreatic cancer to develop a classification model to determine the Overall survival (OS) rate of pancreatic cancer patients (28). Methylation-mediated LINC00261 inhibits pancreatic cancer progression by repressing c-MYC transcription (29). Ferroptosis leads to a state of oxidative stress and can also be driven by the ferroptosis under the GOT1 pathway. Thus, ferroptosis functions to improve the outcome of pancreatic cancer drug therapies and patients’ prognoses. Some studies have shown that pancreatic cancers are highly resistant to chemical and radiation therapy. For example resistance to gemcitabine tend to depend on the intracellular iron and lipid reactive oxygen species (30). Additionally, key metabolites in ferroptosis-related pathways can also overlap with major circulating metabolites in pancreatic cancer patients, thereby affecting the survival rate of pancreatic cancer patients (31).
This study mainly focused on the pancreatic cancer-related data and was conducted with the help of TCGA and the GEO databases. We selected microarray sets, such as GSE107610, GSE62165 and GSE16515, to identify the DEGs in normal tissues and tumor tissues and screened them to identify the molecules associated with methylation and ferroptosis, and then constructed a joint prognostic monitoring model using these selected molecules.
Conclusions
An analysis that combines a variety of epigenetic characteristics provides a practical solution to analyzing the prognosis of patients with tumors. It is better than any single histological analysis or single epigenetic analysis. This study provides a broader perspective for the subsequent establishment of prognostic models for “difficult” diseases.
Acknowledgments
Funding: This work was supported by grants from the National Natural Science Foundation of China (No. 82272624), the Natural Science Foundation of Jiangsu Province (No. BK20211105), the Key Research and Development Plan of Jiangsu Province (No. BE2019692), the Health Project of Jiangsu Province (grant No. H2019072), the Social Development Foundation of Nantong City (grant Nos. MS22020005, JCZ21061, JC22022001 and JCZ2022027), the Postgraduate Research and Practice Innovation Program of Jiangsu Province (Nos. KYCX20_2673, KYCX20_2681, and 518 KYCX21_3112), and the Jiangsu Elderly Health Research Project (Nos. LD2021008 and LR2021012).
Footnote
Reporting Checklist: The authors have completed the TRIPOD reporting checklist. Available at https://jgo.amegroups.com/article/view/10.21037/jgo-22-941/rc
Conflicts of Interest: All authors have completed the ICMJE uniform disclosure form (available at https://jgo.amegroups.com/article/view/10.21037/jgo-22-941/coif). The authors have no conflicts of interest to declare.
Ethical Statement: The authors are accountable for all aspects of the work in ensuring that questions related to the accuracy or integrity of any part of the work are appropriately investigated and resolved. The study was conducted in accordance with the Declaration of Helsinki (as revised in 2013).
Open Access Statement: This is an Open Access article distributed in accordance with the Creative Commons Attribution-NonCommercial-NoDerivs 4.0 International License (CC BY-NC-ND 4.0), which permits the non-commercial replication and distribution of the article with the strict proviso that no changes or edits are made and the original work is properly cited (including links to both the formal publication through the relevant DOI and the license). See: https://creativecommons.org/licenses/by-nc-nd/4.0/.
References
- Mizrahi JD, Surana R, Valle JW, et al. Pancreatic cancer. Lancet 2020;395:2008-20. [Crossref] [PubMed]
- Huang Z, Li Z, Jiang M, et al. Homogeneous Multiplex Immunoassay for One-Step Pancreatic Cancer Biomarker Evaluation. Anal Chem 2020;92:16105-12. [Crossref] [PubMed]
- Siegel RL, Miller KD, Fuchs HE, et al. Cancer Statistics, 2021. CA Cancer J Clin 2021;71:7-33. [Crossref] [PubMed]
- Schizas D, Charalampakis N, Kole C, et al. Immunotherapy for pancreatic cancer: A 2020 update. Cancer Treat Rev 2020;86:102016. [Crossref] [PubMed]
- Jin D, Jiao Y, Ji J, et al. Identification of prognostic risk factors for pancreatic cancer using bioinformatics analysis. PeerJ 2020;8:e9301. [Crossref] [PubMed]
- Ho WJ, Jaffee EM, Zheng L. The tumour microenvironment in pancreatic cancer - clinical challenges and opportunities. Nat Rev Clin Oncol 2020;17:527-40. [Crossref] [PubMed]
- Han X, Wang M, Zhao YL, et al. RNA methylations in human cancers. Semin Cancer Biol 2021;75:97-115. [Crossref] [PubMed]
- He L, Li H, Wu A, et al. Functions of N6-methyladenosine and its role in cancer. Mol Cancer 2019;18:176. [Crossref] [PubMed]
- Huang H, Weng H, Chen J. m6A Modification in Coding and Non-coding RNAs: Roles and Therapeutic Implications in Cancer. Cancer Cell 2020;37:270-88. [Crossref] [PubMed]
- Zhou F, Chen B. Prognostic significance of ferroptosis-related genes and their methylation in AML. Hematology 2021;26:919-30. [Crossref] [PubMed]
- Mou Y, Wang J, Wu J, et al. Ferroptosis, a new form of cell death: opportunities and challenges in cancer. J Hematol Oncol 2019;12:34. [Crossref] [PubMed]
- Wang Y, Wei Z, Pan K, et al. The function and mechanism of ferroptosis in cancer. Apoptosis 2020;25:786-98. [Crossref] [PubMed]
- Zhang X, Yu K, Ma L, et al. Endogenous glutamate determines ferroptosis sensitivity via ADCY10-dependent YAP suppression in lung adenocarcinoma. Theranostics 2021;11:5650-74. [Crossref] [PubMed]
- Ding Y, Chen X, Liu C, et al. Identification of a small molecule as inducer of ferroptosis and apoptosis through ubiquitination of GPX4 in triple negative breast cancer cells. J Hematol Oncol 2021;14:19. [Crossref] [PubMed]
- Xu C, Sun S, Johnson T, et al. The glutathione peroxidase Gpx4 prevents lipid peroxidation and ferroptosis to sustain Treg cell activation and suppression of antitumor immunity. Cell Rep 2021;35:109235. [Crossref] [PubMed]
- Ajoolabady A, Aslkhodapasandhokmabad H, Libby P, et al. Ferritinophagy and ferroptosis in the management of metabolic diseases. Trends Endocrinol Metab 2021;32:444-62. [Crossref] [PubMed]
- Bailey P, Chang DK, Nones K, et al. Genomic analyses identify molecular subtypes of pancreatic cancer. Nature 2016;531:47-52. [Crossref] [PubMed]
- Colaprico A, Silva TC, Olsen C, et al. TCGAbiolinks: an R/Bioconductor package for integrative analysis of TCGA data. Nucleic Acids Res 2016;44:e71. [Crossref] [PubMed]
- Seino T, Kawasaki S, Shimokawa M, et al. Human Pancreatic Tumor Organoids Reveal Loss of Stem Cell Niche Factor Dependence during Disease Progression. Cell Stem Cell 2018;22:454-467.e6. [Crossref] [PubMed]
- Janky R, Binda MM, Allemeersch J, et al. Prognostic relevance of molecular subtypes and master regulators in pancreatic ductal adenocarcinoma. BMC Cancer 2016;16:632. [Crossref] [PubMed]
- Pei H, Li L, Fridley BL, et al. FKBP51 affects cancer cell response to chemotherapy by negatively regulating Akt. Cancer Cell 2009;16:259-66. [Crossref] [PubMed]
- Leek JT, Johnson WE, Parker HS, et al. The sva package for removing batch effects and other unwanted variation in high-throughput experiments. Bioinformatics 2012;28:882-3. [Crossref] [PubMed]
- Smyth GK. limma: Linear Models For Microarray Data. In: Gentleman R, Carey VJ, Huber W, et al. editors. Bioinformatics and computational biology solutions using R and Bioconductor. New York, NY: Springer, 2005:397-420.
- Wilkerson MD, Hayes DN. ConsensusClusterPlus: a class discovery tool with confidence assessments and item tracking. Bioinformatics 2010;26:1572-3. [Crossref] [PubMed]
- Yu G, Wang LG, Han Y, et al. clusterProfiler: an R package for comparing biological themes among gene clusters. OMICS 2012;16:284-7. [Crossref] [PubMed]
- Zhou N, Bao J. FerrDb: a manually curated resource for regulators and markers of ferroptosis and ferroptosis-disease associations. Database (Oxford) 2020;2020:baaa021. [Crossref] [PubMed]
- Wang M, Liu J, Zhao Y, et al. Upregulation of METTL14 mediates the elevation of PERP mRNA N6 adenosine methylation promoting the growth and metastasis of pancreatic cancer. Mol Cancer 2020;19:130. [Crossref] [PubMed]
- Liu S, Zheng Y, Zhang Y, et al. Methylation-mediated LINC00261 suppresses pancreatic cancer progression by epigenetically inhibiting c-Myc transcription. Theranostics 2020;10:10634-51. [Crossref] [PubMed]
- Yamaguchi Y, Kasukabe T, Kumakura S. Piperlongumine rapidly induces the death of human pancreatic cancer cells mainly through the induction of ferroptosis. Int J Oncol 2018;52:1011-22. [Crossref] [PubMed]
- Yang J, Xu J, Zhang B, et al. Ferroptosis: At the Crossroad of Gemcitabine Resistance and Tumorigenesis in Pancreatic Cancer. Int J Mol Sci 2021;22:10944. [Crossref] [PubMed]
- Yuan C, Clish CB, Wu C, et al. Circulating Metabolites and Survival Among Patients With Pancreatic Cancer. J Natl Cancer Inst 2016;108:djv409. [Crossref] [PubMed]