Constructing and validating of m7G-related genes prognostic signature for hepatocellular carcinoma and immune infiltration: potential biomarkers for predicting the overall survival
Highlight box
Key findings
• This study Constructing and Validating of m7G-Related Genes Prognostic Signature that discover 21 m7G-related DEGs in LIHC. Among them, the expressions of the
What is known and what is new?
• mRNA modification has gradually been proven to be related to the onset and progression of cancer, which provides an assessment approach to identity early effective biomarkers to achieve early detection, early prevention;
• The impact of m7G-related DEGs indicators in LIHC and identification of effective biomarkers. In the immune, the expressions of neutrophils and natural killer (NK) cells were low and that of regulatory T-cells (Tregs) was high in the high-risk group.
What is the implication, and what should change now?
• Early identification of effective biomarkers to intervene in the course of cancer.
Introduction
Globally, liver hepatocellular carcinoma (LIHC) ranks sixth among all cancers in terms of incidence and fourth in terms of cancer-related deaths. In 2020, there were 905,677 new LIHC cases worldwide, accounting for 4.69% of all cancers, and over 780,000 people die of LIHC each year (1). Currently, LIHC is predominantly managed by surgical resection, liver transplantation, transarterial chemoembolization (TACE), and ablation therapy; however, each of these methods has its limitations (2). Over 70% of LIHC patients relapse within 5 years after hepatectomy and/or tumor ablation (3,4). The 5-year survival rate is 60–70% following hepatectomy (5) and 38.3% after TACE (6). These figures highlight the need to better understand the molecular mechanisms that regulate the development of effective strategies for treating LIHC.
The mechanisms involved in the pathogenesis of LIHC are complicated. Epigenetics refers to the study of the regulation of gene expression in terms of the lack of variation in the nucleotide sequence of a gene. Recent studies have shown that misregulated RNA modifications often lead to abnormal gene expression and are strongly associated with developmental diseases and cancers (7,8). Over 150 forms of RNA alterations have been identified, and N7-methylguanosine (m7G), which is among the most prevalent RNA modifications, results from the methylation of RNA guanine at position N7 by methyltransferase (9) and is widely found within transfer RNAs (tRNAs), ribosomal RNAs (rRNAs), the 5' cap of messenger RNA (mRNA), and mRNAs.
Methylation modifications in RNAs are typically controlled by “writer”, “eraser”, and “reader” proteins. A writer is a protein that “writes” a specific chemical group into an RNA. An eraser is a protein that removes the modifying chemical group added by the writer. A reader is a protein that recognizes and binds to the modified RNA site and participates in transcription, translation, and other processes. Currently, the writers of m7G include METTL1, WBSCR22, and RNMT, and no eraser or reader of m7G has been found to date (10). m7G modifications predominantly exist at position 46 of the variable region of tRNAs and are catalyzed by the methyltransferase METTL1/WDR4 complex. m7G modification and C13-G22 form a tertiary base pair, which contributes to the stability of tRNAs (11).
A loss of m7G modification causes ribosomes to arrest at m7G tRNA-dependent codons, affecting the gene expression related to brain development and the cell cycle (12). In rRNAs, m7G is catalyzed by the WBSCR22/TRMT112 complex, in which the TRMT112 protein, as an activating enzyme of methyltransferase WBSCR22, mediates G1639 methylation in 18S rRNA and regulates the processing, export, and maturation of RNAs and pre-RNAs (13,14). A cap structure exists at the 5' end of mRNA, and this guanosine cap is methylated at position N-7 by the methyltransferase complex RNA guanine-7 methyltransferase (RNMT)-RNMT-activating miniprotein (RAM). RAM is a subunit of RNMT, which increases methyltransferase activity (15). The m7G cap protects mRNAs from decomposition at the 5' end, thereby playing an important role in regulating the export, stability, splicing, transcription, translation, and decay of mRNAs (16,17). Moreover, the RNMT-RAM complex influences the synthesis of rRNAs by regulating c-Myc (18). With the improvement of sequencing techniques, m7G modification catalyzed by METTL1 methyltransferase has been confirmed within mRNAs (19).
Taken together, m7G modifications are instrumental in regulating the processing, transcription, and metabolism of RNAs and intervening in the expression of related genes. Abnormal expression of RNA-modifying enzymes leads to either an increase or a decrease in RNA modifications, resulting in gene expression disorders and tumor cell proliferation (20). METTL1 over-expression results in an increase in the number of m7G-modified tRNAs, represented by Arg-TCT-4-1, and upregulates the translation of cell cycle-regulating mRNAs and the expression of growth-promoting proteins, which drives the development of cancer (21). m7G modifications of tRNAs are closely related to lung cancer and intrahepatic cholangiocarcinoma (ICC) (22,23). Let-7 is one of the most studied miRNAs; the let-7 gene is significantly downregulated in human breast, lung, and intestinal cancers. METTL1-mediated methylation augments let-7 miRNA processing by disrupting an inhibitory secondary structure within the primary miRNA transcript (pri-miRNA), regulates the expression of pri-let-7e and let-7, and affects the migration of lung cancer cells, highlighting that m7G methylation may serve as a new RNA modification to regulate the structure of miRNAs and cell migration (24).
The relationship between m7G and LIHC has been marginally explored. Currently, four papers have used bioinformatics analysis to explore the relationship between m7G and LIHC (25-28). They constructed different models by bioinformatics to predict valid biomarkers. Their limitations including the limited prediction ability, and the potential strengths of m7G methylation-related genes, to support the clinical needs for new prognostic biomarkers or prognosis prediction model. In addition, TCGA is a continuously updated database and the sample is always changing. This is why it is essential to constantly update the algorithms and calculations. Based on the available data, it is known that m7G-related genes exert an important function in tumor formation, but their specific roles in LIHC have not yet been determined. The present research examined the differential expression of m7G-related genes in LIHC and healthy tissues as well as the prognostic significance of these differentially expressed genes (DEGs) and immune infiltration in LIHC. We present the following article in accordance with the TRIPOD reporting checklist (available at https://jgo.amegroups.com/article/view/10.21037/jgo-22-1134/rc).
Methods
Data acquisition
We retrieved the RNA sequencing (RNA-seq) data and relevant clinical features of 374 LIHCs and 50 normal data with LIHC from The Cancer Genome Atlas (TCGA) database (https://portal.gdc.cancer.gov/repository) on January 15, 2022. For the external validation cohort, the RNA-seq data and clinical information of 243 LIHC patients were obtained from the International Cancer Genome Consortium (ICGC) (https://icgc.org/) (Table 1). The study was conducted in accordance with the Declaration of Helsinki (as revised in 2013).
Table 1
Variables | Number of samples |
---|---|
TCGA | |
Gender (male/female) | 255/122 |
Age at diagnosis (≤65/>65 years) | 235/141 |
Grade (G1/G2/G3/G4/NA) | 180/55/124/13/5 |
Stage (I/II/III/IV/NA) | 2/131/141/136/2 |
T (T1/T2/T3/T4/NA) | 175/87/86/5/24 |
M (M0/M1/NA) | 272/4/101 |
N (N0/N1/NA) | 257/4/116 |
ICGC | |
Gender (male/female) | 97/135 |
Age at diagnosis (≤65/>65 years) | 51/181 |
Grade (high/low) | Unknown |
Stage (I–II/III–IV) | 142/90 |
T (T1/T2/T3/T4/NA) | Unknown |
M (M0/M1) | Unknown |
N (N0/N1/N2/N3/NA) | Unknown |
TCGA, The Cancer Genome Atlas; ICGC, International Cancer Genome Consortium.
Identification of m7G-related DEGs
Twenty-three m7G-related genes were extracted from a previous review (11) and the Gene Set Enrichment Analysis (GSEA) website (http://www.gsea-msigdb.org/gsea/login.jsp). Using the “limma” R package (version 4.1.2, New Zealand), DEGs with a P value of 0.05 were identified according to the following denominations: *P<0.05, **P<0.01, and ***P<0.001.
Development and validation of a predictive model according to the m7G-related genes
The prognostic significance of m7G-related genes was assessed by univariate Cox regression analysis to determine the relationship between every gene of TCGA cohort and the survival status, using a P value of 0.05. A total of 12 survival-related genes were detected for additional examination. By utilizing the “glmnet” R package (version 4.1.2, New Zealand), LASSO Cox regression analysis was carried out to narrow the scope of applicant genes and develop a predictive model. Finally, five genes and their coefficients were preserved, and the “λ” value represents the degree of overfitting of the model. TCGA expression data were centralized and standardized to calculate risk scores. The following equation was utilized to calculate the risk score: risk score = P7iXi'Yi (X: coefficient, Y: gene expression pattern).
LIHC patients in TCGA cohort were divided into low- and high-risk groups based on their average risk score. Kaplan-Meier estimation was utilized to assess the survival time between the two groups. The “survival” and “timeROC” R packages (version 4.1.2, New Zealand) were employed to analyze the 3-year receiver operating characteristic (ROC) curves. Validation was performed using a cohort of patients with LIHC from the ICGC database (https://icgc.org/). The same equation was utilized to measure the risk scores for TCGA cohort. The average risk score was also employed to classify the patients into low- or high-risk groups, and the genetic model was validated.
Independent prognostic analysis of the risk scores
We extracted the clinical data (age and grading) of patients from TCGA and ICGC cohorts and examined these variables along with risk scores obtained from our regression model. Univariate and multivariate Cox regression analyses were also carried out.
Functional enrichment analysis of DEGs in the two groups
LIHC patients from TCGA cohort were separated into two subgroups depending on their median risk scores. The DEGs of the two groups were examined according to the following specific criteria: false discovery rate (FDR), a more liberal criterion, was adopted together with absolute log2fold change (FC) to determine the significance criteria (FDR <0.05 and |log2FC| ≥1). Gene Ontology (GO) and Kyoto Encyclopedia of Genes and Genomes (KEGG) analyses of these DEGs were performed using the “clusterProfiler” R package (version 4.1.2, New Zealand). Also, the “gsva” program was employed to determine the infiltrative immune cells score and immune function was evaluated through single-sample GSEA (ssGSEA).
Statistical analysis
One-way analysis of variance (ANOVA) was employed to assess the gene expression patterns in healthy and LIHC tissues. Pearson’s Chi-square test was employed to contrast the categorical variables. The survival status of patients was compared between groups using Kaplan-Meier estimation and a two-sided log-rank test. Univariate and multivariate Cox regression analyses were employed to study the independent predictive significance of the risk model. We utilized the Mann-Whitney U test to contrast the pattern of immune pathway activation and immune cell infiltration between the two groups. All data were examined using the R program.
Results
DEGs in normal and tumor tissues
Twenty-one DEGs were identified by examining the expression patterns of 23 m7G-related genes in 50 healthy and 374 LIHC samples (P<0.01). Among them, the expressions of the NUDT10 and EIF4E3 genes were downregulated, while those of the other 19 genes (EIF4E, NUDT16, NCBP1, NUDT4, CYFIP1, WDR4, EIF4E2, EIF4A1, NUDT3, SNUPN, LARP1, NUDT11, DCP2, NCBP2, EIF3D, METTL1, LARP1, WDR4, and NUDT11) were upregulated in the LIHC samples. Figure 1A depicts the expression patterns of the 23 m7G-related genes (green: low expression; red: high expression). An association network diagram was created to study the association between these m7G-related genes (Figure 1B; red and blue lines indicate positive and negative associations, respectively).
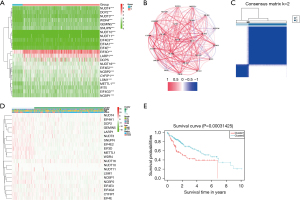
DEGs-based tumor classification
To study the relationship between the expression levels of 21 m7G-related DEGs and LIHC subtypes, consensus clustering was performed on all 374 LIHC patients in TCGA cohort. Elevating the cluster variable “κ” from 2 to 9 revealed the greatest intra-group correlation and low inter-group correlation at κ=2, suggesting that the 374 LIHC patients may be split into two clusters based on the 21 DEGs (Figure 1C). The heat map displays the gene expression levels and clinical data, including age (≤60 vs. >60 years), grade of tumor differentiation (G1–G4), and survival status (survival vs. death) (Figure 1D). Significant variations existed in the overall survival (OS) time between the two groups (P=0.0003, Figure 1E). Moreover, the clinical features also differed markedly between the two LIHC subgroups.
Constructing a predictive gene model based on TCGA data
We contrasted 366 LIHC samples to patients with complete corresponding survival data. The survival-related genes were initially examined by univariate Cox regression analysis. Twelve genes (NCBP2, GEMIN5, NCBP1, WDR4, EIF4E, EIF3D, LARP1, DCP2, EIF4E2, EIF4G3, METTL1, and LSM1) fulfilled the parameters of P<0.05 and were subjected to additional examination (Figure 2A). An NCBP1, NCBP2, WDR4, GEMIN5, and EIF4E gene-based prognostic model was developed using multivariate LASSO Cox regression (Figure 2B,2C).
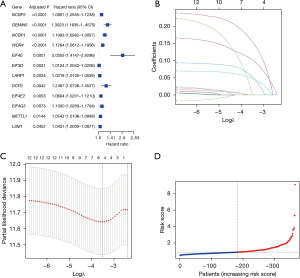
The risk score was measured as follows: risk score = (0.327*EIF4E exp.) + (0.036*PGEMIN5 exp.) + (0.088*NCBP1 exp.) + (0.026*NCBP2 exp.) + (0.057*WFR4 exp.).
The median risk score was determined using the risk score calculation equation, and the 366 patients were split equally between the low- and high-risk groups (Figure 2D).
Principal component analysis (PCA) revealed that patients with various risk levels were accurately categorized into two clusters (Figure 3A). The high-risk group exhibited greater mortality and lower survival times than the low-risk group. The OS differences between the high- and low-risk groups were statistically significant (P=0.001, Figure 3B). The sensitivity and specificity of the predictive model were evaluated through ROC analysis, with the area under the curve (AUC) employed as the scoring metric. According to the ROC curves, the AUC was 0.716 at 1 year, 0.64 at 2 years, and 0.631 at 3 years (Figure 3C). The threshold AUC value for a good predictive model is 0.7 and the P value<0.05 for Statistical significance. We examined the data and compared similar bioinformatics results. The lethality rate for liver cancer was found to be very high, with most patients dying in the first year. Our model made predictions based on the first-year results, resulting in an AUC of no more than 0.7 in years 2–3, which may have contributed to this result. However, after comparing similar studies, it was found that such a result was not influential.
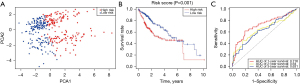
External validation of the predictive model
In total, 243 LIHC patients in the ICGC database were included in the validation set and were split into the low-risk group (n=122) and the high-risk group (n=121) as per the median risk score calculated using the above-mentioned formula. PCA showed that the patients could be appropriately split into two groups (Figure 4A). Patients in the low-risk group (Figure 4B, left side of the dashed line) exhibited longer survival times and a lower mortality rate compared with patients in the high-risk group. Furthermore, a significant variation in the survival rate between the low-risk and high-risk groups was determined through Kaplan-Meier estimation (P=0.0027, Figure 4C). The ROC curves of the ICGC cohort showed that the five-gene predictive model exhibited effective predictive performance (AUC =0.642 at 1 year, AUC =0.686 at 2 years, and AUC =0.667 at 3 years) (Figure 4D).
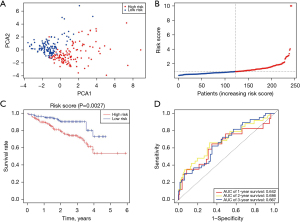
Independent predictive significance of the risk model
Univariate and multivariate Cox regression analyses were performed to evaluate whether the risk score of the predictive model might act as an independent predictive factor [hazard ratio (HR): 1.848, 95% confidence interval (CI): 1.286–2.656; HR: 2.597, 95% CI: 1.358–4.965] (Figure 5A,5B). Multivariate Cox analysis suggested that the risk score may be an independent predictor for patient survival status after correcting for other confounders. (HR: 1.889, 95% CI: 1.309–2.727; HRs: 2.348, 95% CI: 1.219–4.522) (Figure 5C,5D). The heat maps of gene expression and clinical features in TCGA and ICGC cohorts were also plotted (Figure 5E,5F).
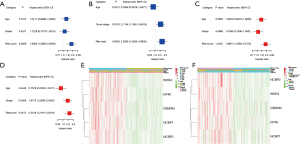
Functional analysis based on the risk model
To investigate the gene function and pathway variations between the two groups divided by the risk model, we obtained DEGs using the “limma” R package as well as the following parameters: FDR <0.05 and |log2FC| ≥1. Enrichment analysis was conducted using the “clusterProfiler” program (29). In TCGA cohort, 3,772 DEGs were determined between the low- and high-risk groups. The expression of 3,700 genes was elevated in the high-risk group, while that of the remaining 72 genes was downregulated. GO and KEGG analyses demonstrated that these DEGs were predominantly enriched in pathways such as the cell cycle, mitosis, neuroactive ligand-receptor regulation, and ECM-receptor interaction (Figures 6A,6B).
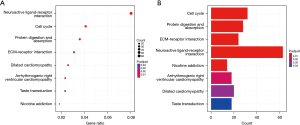
Comparison of immunoactivity between the high- and low-risk groups
We compared 16 immune cell enrichment scores and 13 immune functional activities using ssGSEA between the low- and high-risk groups in the TCGA and ICGC cohorts. Among the immune pathways, the expression of cytolytic activity and types I and II interferon (IFN) responses were low, while that of the major histocompatibility complex (MHC) was elevated in the high-risk group (P<0.05) (Figure 7A). The immuno-oncology scoring showed that of the 16 immune cells, the expression of neutrophils and natural killer (NK) cells was low, and that of regulatory T-cells (Tregs) was high in the high-risk group (Figure 7B). Similar conclusions were reached when analyzing the immunological state of the ICGC group (Figure 7C,7D).
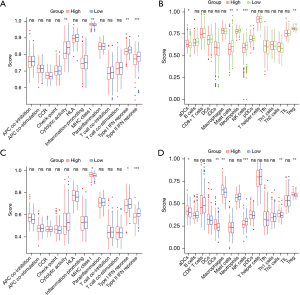
Discussion
LIHC clinical treatment is a critical clinical problem due to the disease’s fast development and dismal prognosis. A lack of potent tumor-killing initiators and selective tumor-targeting therapeutic medications limits the level of precision medicine for LIHC. A recent study discovered that changes in the process of programmed tumor cell death may enhance the focused therapeutic effect of LIHC (30). As a result, early detection and diagnosis of the condition are critical. m7G is the most prevalent internal mRNA change in all higher eukaryotes, and it is tightly controlled by a slew of “writers”, “erasers”, and “readers”. m7G mutations influence cancer processing. m7G regulates cellular proliferation and maturation, both of which have been associated to cancer development. A recent study discovered that changes in the process of programmed tumor cell death may enhance the focused therapeutic effect of LIHC (31). As a result, early detection and diagnosis of the condition are critical. m7G is the most prevalent internal mRNA change in all higher eukaryotes, and it is tightly controlled by a slew of “writers”, “erasers”, and “readers”. Evidence suggests that abnormal m7G methylation may promote cancer growth by upregulating oncogenes and inhibiting tumor suppressor genes, and that m7G RNA methylation can be influenced by m7G regulator expression and m7G enzyme activity, influencing tumor progression further (27,32). The interactions of m7G regulators in LIHC were used to determine the tendencies of m7G readers, writers, and erasers. This pattern suggests that LIHC was involved in m7G RNA methylation. These factors also have an impact on immune cell infiltration in LIHC. Therefore, exploring how m7G influences the onset, development of LIHC is of great importance for future effective target prediction and drug design (33). In this study, we constructed that a predictive model according to five m7G-related genes (EIF4E, GEMIN5, NCBP1, NCBP2, WDR4) could predict the survival of patients.
EIF4E, a member of the translation initiation factors family in eukaryotic cells, is located at chromosome 4q21–4q25. It binds specifically to the m7G cap structure at the 5' end of mRNAs, and as a component of the EIF4F complex, participates in initiation site regulation and translation localization. As the most important regulatory factor discovered to date, EIF4E mediates the recruitment of mRNA on the 40S ribosomal subunits and participates in mRNA transport and processing (34). The EIF4E expression pattern is relatively reduced in normal tissues; however, it can selectively enhance the translation of mRNAs, resulting in increased synthesis of tumor-related proteins (such as VEGF, vascular endothelial growth factor) and promotion of tumor occurrence and development (35). The enrichment of EIF4E in cancer tissues and the high-risk group observed herein indicates that EIF4E promotes the development of LIHC, which is consistent with the literature.
GEMIN5, a part of the macromolecular complex in the cytoplasm and nucleus, has recently been identified as a novel m7G cap-binding protein. A study has shown that GEMIN5 can bind specifically to the m7G cap structure via its WD repeat domain and affect the small nuclear ribonucleic protein (snRNP) assembly process, thereby regulating the level of post-transcriptional gene expression (36). Functional mutations in GEMIN5 lead to developmental disorders of the nervous system, resulting in neurodevelopmental retardation and ataxia syndrome (37). No association between GEMIN5 and carcinogenesis has been reported, but GEMIN5 was identified as a risk factor for LIHC in the present study.
NCBP1 and NCBP2 are components of the nuclear cap-binding complex and protein complexes in the nucleus. The cap-binding complexes, first found in HeLa cells, can bind to the m7G caps of newly transcribed mRNAs and coordinate downstream RNA biogenesis (38). NCBP1 and NCBP2 are key target genes of lung cancer (39,40). NCBP1 mediates the aggressiveness of lung cancer cells by regulating the expression of the epithelial-stromal transition protein via CUL48 (41).
WDR4 is a gene that encodes the non-catalytic subunit of methyltransferase, and WDR4 and METTL1 co-modify the m7G of tRNAs. Modifying tRNAs is essential for efficient framework maintenance and the fidelity of translation in protein synthesis (42). WDR4 abnormalities are associated with dwarfism (43). Dai et al. reported that expression of the complex comprising METTLE and WDR4 was significantly upregulated in ICC, and was correlated with a poor prognosis. Experimental study showed that the METTLE/WDR4 complex selectively upregulates the expression of genes involved in pathways such as the cell cycle (23). These reports are consistent with our results, in which the WDR4 expression pattern was elevated in the LIHC group, and the DEGs in the high- and low-risk groups of LIHC were mainly enriched in pathways such as the cell cycle.
The present study demonstrated that the DEGs of the two groups were predominantly enriched in pathways regulating the cell cycle, mitosis, neuroactive ligand-receptor regulation, and ECM-receptor interplay. New research indicates that abnormal activity of the growth cycle mechanism of core cells is the driving force of tumorigenesis, and occurs in almost all tumors. Thus, targeting specific cyclins is a new direction in cancer treatment (44). The interplay between cancer cells and immune cells constitutes the microenvironment of tumors, and enhanced cyclin activity in cancer cells inhibits anti-tumor immunity and affects the tumor microenvironment. A previous study showed that cyclin-dependent kinase 4/6 (CDK4/6) inhibitors suppressed tumor growth by downregulating the expression of DNA methyltransferase-1 (DNMT1) in a transgenic mouse model for breast cancer (45). The genome and transcriptome profiles of human tumors also support the presumption that the cell cycle disorders of cancer cells promote immune evasion (46). The immunological scoring of the LIHC two groups in this study revealed that of the 16 immune cells, neutrophils and NK cells were expressed at low levels, and Tregs were expressed at high levels in the high-risk group tissues. Also, among the immune pathways in the high-risk group, the expression of cytolytic activity and types I and II IFN responses were low, while that of MHC was high.
MHC, a key component of the adaptive immune system, binds peptides derived from cells expressing genes, transporting and presenting these antigens on the cell surface. This allows CD8 T cells to recognize and destroy pathological cells synthesizing abnormal proteins. Hence, one mechanism through which cancer evades immune surveillance is via the loss of MHC-I (MHC class I molecules) antigen presentation. This not only impairs the ability of the natural immune response to control cancer but also hinders immunotherapy that involves reactivating anti-tumor CD8 T cells (47).
However, the present study discovered that the MHC-I expression pattern increased in the high-risk group, which was explained in Barkal et al.’s study (48). The authors found that the expression of MHC-I did not decrease in some cancers. MHC-I on the surface of cancer cells contains β2 microglobulin, which can bind to the LILRB1 protein on the surface of macrophages. Experimental studies have illustrated that such binding can inhibit the phagocytosis of macrophages and kill cancer cells (48,49). These findings may explain the enrichment of MHC-I discovered in the high-risk group and provide a novel approach for treating LIHC.
Although the mechanism through which RNA modification controls cancer progression remains unclear, intervention in RNA modification and RNA-modified proteins has become a new anticancer strategy (50,51). For example, ribavirin, an artificial guanosine nucleoside analog with a broad spectrum of antiviral properties, has recently been shown to inhibit the activity of EIF4E, and clinical trials have demonstrated that it has good efficacy in leukemia and other cancers (52,53).
Conclusions
This research demonstrated that m7G is strongly linked to LIHC and that the risk scores based on a five m7G-related gene predictive model were independent risk factors for estimating patient outcomes in TCGA and ICGC cohorts. This study also identified a novel genetic marker for estimating the prognoses of LIHC patients. We speculate that m7G-related genes can regulate tumor development and influence the immunologic microenvironment of tumors by regulating the cell cycle and other pathways. Findings from this study provide a basis for further exploration of the occurrence and treatment of LIHC as well as the relationship between LIHC and m7G.
The following restrictions apply to our analysis: (I) The current study did not collect enough alternative data sources from other publicly available sites to evaluate the model’s dependability. (II) While the functional enrichment processes at work in the regulatory networks of diverse risk groups were investigated, additional research is needed to corroborate the current findings. (III) The prediction model developed in this work must be evaluated both externally and practically.
Acknowledgments
Funding: This research was supported by the National Natural Science Foundation of China (Nos. 81874420 and 82274388) and the Shanxi Province focuses on essential regional innovation cooperation tasks in lookup and improvement (international scientific and technological cooperation) (No. 201903D421018).
Footnote
Reporting Checklist: The authors have completed the TRIPOD reporting checklist. Available at https://jgo.amegroups.com/article/view/10.21037/jgo-22-1134/rc
Conflicts of Interest: All authors have completed the ICMJE uniform disclosure form (available at https://jgo.amegroups.com/article/view/10.21037/jgo-22-1134/coif). The authors have no conflicts of interest to declare.
Ethical Statement: The authors are accountable for all aspects of the work in ensuring that questions related to the accuracy or integrity of any part of the work are appropriately investigated and resolved. The study was conducted in accordance with the Declaration of Helsinki (as revised in 2013).
Open Access Statement: This is an Open Access article distributed in accordance with the Creative Commons Attribution-NonCommercial-NoDerivs 4.0 International License (CC BY-NC-ND 4.0), which permits the non-commercial replication and distribution of the article with the strict proviso that no changes or edits are made and the original work is properly cited (including links to both the formal publication through the relevant DOI and the license). See: https://creativecommons.org/licenses/by-nc-nd/4.0/.
References
- Cao M, Li H, Sun D, et al. Global epidemiological status of liver cancer in 2020. Chinese Journal of Cancer Prevention and Treatment 222,29:322-8.
- Chen Z, Xie H, Hu M, et al. Recent progress in treatment of hepatocellular carcinoma. Am J Cancer Res 2020;10:2993-3036. [PubMed]
- Marasco G, Colecchia A, Colli A, et al. Role of liver and spleen stiffness in predicting the recurrence of hepatocellular carcinoma after resection. J Hepatol 2019;70:440-8. [Crossref] [PubMed]
- Lee DH, Lee JM, Lee JY, et al. Radiofrequency ablation of hepatocellular carcinoma as first-line treatment: long-term results and prognostic factors in 162 patients with cirrhosis. Radiology 2014;270:900-9. [Crossref] [PubMed]
- Marrero JA, Kulik LM, Sirlin CB, et al. Diagnosis, Staging, and Management of Hepatocellular Carcinoma: 2018 Practice Guidance by the American Association for the Study of Liver Diseases. Hepatology 2018;68:723-50. [Crossref] [PubMed]
- Burrel M, Reig M, Forner A, et al. Survival of patients with hepatocellular carcinoma treated by transarterial chemoembolisation (TACE) using Drug Eluting Beads. Implications for clinical practice and trial design. J Hepatol 2012;56:1330-5. [Crossref] [PubMed]
- Suzuki T. The expanding world of tRNA modifications and their disease relevance. Nat Rev Mol Cell Biol 2021;22:375-92. [Crossref] [PubMed]
- Wood S, Willbanks A, Cheng JX. The Role of RNA Modifications and RNA-modifying Proteins in Cancer Therapy and Drug Resistance. Curr Cancer Drug Targets 2021;21:326-52. [Crossref] [PubMed]
- Enroth C, Poulsen LD, Iversen S, et al. Detection of internal N7-methylguanosine (m7G) RNA modifications by mutational profiling sequencing. Nucleic Acids Res 2019;47:e126. [Crossref] [PubMed]
- Chen L, Wang P, Bahal R, et al. Ontogenic mRNA expression of RNA modification writers, erasers, and readers in mouse liver. PLoS One 2019;14:e0227102. [Crossref] [PubMed]
- Tomikawa C. 7-Methylguanosine Modifications in Transfer RNA (tRNA). Int J Mol Sci 2018;19:4080. [Crossref] [PubMed]
- Lin S, Liu Q, Lelyveld VS, et al. Mettl1/Wdr4-Mediated m(7)G tRNA Methylome Is Required for Normal mRNA Translation and Embryonic Stem Cell Self-Renewal and Differentiation. Mol Cell 2018;71:244-255.e5. [Crossref] [PubMed]
- Haag S, Kretschmer J, Bohnsack MT. WBSCR22/Merm1 is required for late nuclear pre-ribosomal RNA processing and mediates N7-methylation of G1639 in human 18S rRNA. RNA 2015;21:180-7. [Crossref] [PubMed]
- Figaro S, Wacheul L, Schillewaert S, et al. Trm112 is required for Bud23-mediated methylation of the 18S rRNA at position G1575. Mol Cell Biol 2012;32:2254-67. [Crossref] [PubMed]
- Varshney D, Petit AP, Bueren-Calabuig JA, et al. Molecular basis of RNA guanine-7 methyltransferase (RNMT) activation by RAM. Nucleic Acids Res 2016;44:10423-36. [Crossref] [PubMed]
- Mandal SS, Chu C, Wada T, et al. Functional interactions of RNA-capping enzyme with factors that positively and negatively regulate promoter escape by RNA polymerase II. Proc Natl Acad Sci U S A 2004;101:7572-7. [Crossref] [PubMed]
- Furuichi Y, LaFiandra A, Shatkin AJ. 5'-Terminal structure and mRNA stability. Nature 1977;266:235-9. [Crossref] [PubMed]
- Dunn S, Lombardi O, Cowling VH. c-Myc co-ordinates mRNA cap methylation and ribosomal RNA production. Biochem J 2017;474:377-84. [Crossref] [PubMed]
- Zhang LS, Liu C, Ma H, et al. Transcriptome-wide Mapping of Internal N(7)-Methylguanosine Methylome in Mammalian mRNA. Mol Cell 2019;74:1304-1316.e8. [Crossref] [PubMed]
- Katsara O, Schneider RJ. m(7)G tRNA modification reveals new secrets in the translational regulation of cancer development. Mol Cell 2021;81:3243-5. [Crossref] [PubMed]
- Orellana EA, Liu Q, Yankova E, et al. METTL1-mediated m(7)G modification of Arg-TCT tRNA drives oncogenic transformation. Mol Cell 2021;81:3323-3338.e14. [Crossref] [PubMed]
- Ma J, Han H, Huang Y, et al. METTL1/WDR4-mediated m(7)G tRNA modifications and m(7)G codon usage promote mRNA translation and lung cancer progression. Mol Ther 2021;29:3422-35. [Crossref] [PubMed]
- Dai Z, Liu H, Liao J, et al. N(7)-Methylguanosine tRNA modification enhances oncogenic mRNA translation and promotes intrahepatic cholangiocarcinoma progression. Mol Cell 2021;81:3339-3355.e8. [Crossref] [PubMed]
- Pandolfini L, Barbieri I, Bannister AJ, et al. METTL1 Promotes let-7 MicroRNA Processing via m7G Methylation. Mol Cell 2019;74:1278-1290.e9. [Crossref] [PubMed]
- Li XY, Wang SL, Chen DH, et al. Construction and Validation of a m7G-Related Gene-Based Prognostic Model for Gastric Cancer. Front Oncol 2022;12:861412. [Crossref] [PubMed]
- Regmi P, He ZQ, Lia T, et al. N7-Methylguanosine Genes Related Prognostic Biomarker in Hepatocellular Carcinoma. Front Genet 2022;13:918983. [Crossref] [PubMed]
- Li XY, Zhao ZJ, Wang JB, et al. m7G Methylation-Related Genes as Biomarkers for Predicting Overall Survival Outcomes for Hepatocellular Carcinoma. Front Bioeng Biotechnol 2022;10:849756. [Crossref] [PubMed]
- Sun J, Li L, Chen H, et al. Identification and Validation of an m7G-Related lncRNAs Signature for Prognostic Prediction and Immune Function Analysis in Endometrial Cancer. Genes (Basel) 2022.
- Yu G, Wang LG, Han Y, et al. clusterProfiler: an R package for comparing biological themes among gene clusters. OMICS 2012;16:284-7. [Crossref] [PubMed]
- Zongyi Y, Xiaowu L. Immunotherapy for hepatocellular carcinoma. Cancer Lett 2020;470:8-17. [Crossref] [PubMed]
- Chen J, Yao S, Sun Z, et al. The pattern of expression and prognostic value of key regulators for m(7)G RNA methylation in hepatocellular carcinoma. Front Genet 2022;13:894325. [Crossref] [PubMed]
- Wang T, Zhou Z, Wang X, et al. Comprehensive analysis of nine m7G-related lncRNAs as prognosis factors in tumor immune microenvironment of hepatocellular carcinoma and experimental validation. Front Genet 2022;13:929035. [Crossref] [PubMed]
- Huang M, Long J, Yao Z, et al. METTL1-mediated m7G tRNA modification promotes lenvatinib resistance in hepatocellular carcinoma. Cancer Res 2022. [Epub ahead of print]. pii: CAN-22-0963. doi:
10.1158/0008-5472.CAN-22-0963 .10.1158/0008-5472.CAN-22-0963 - Jackson RJ, Hellen CU, Pestova TV. The mechanism of eukaryotic translation initiation and principles of its regulation. Nat Rev Mol Cell Biol 2010;11:113-27. [Crossref] [PubMed]
- Truitt ML, Conn CS, Shi Z, et al. Differential Requirements for eIF4E Dose in Normal Development and Cancer. Cell 2015;162:59-71. [Crossref] [PubMed]
- Bradrick SS, Gromeier M. Identification of gemin5 as a novel 7-methylguanosine cap-binding protein. PLoS One 2009;4:e7030. [Crossref] [PubMed]
- Kour S, Rajan DS, Fortuna TR, et al. Loss of function mutations in GEMIN5 cause a neurodevelopmental disorder. Nat Commun 2021;12:2558. [Crossref] [PubMed]
- Miura P, Coriati A, Bélanger G, et al. The utrophin A 5'-UTR drives cap-independent translation exclusively in skeletal muscles of transgenic mice and interacts with eEF1A2. Hum Mol Genet 2010;19:1211-20. [Crossref] [PubMed]
- Wei S, Wang Y, Xu H, et al. Screening of potential biomarkers for chemoresistant ovarian carcinoma with miRNA expression profiling data by bioinformatics approach. Oncol Lett 2015;10:2427-31. [Crossref] [PubMed]
- Xie ZC, Tang RX, Gao X, et al. A meta-analysis and bioinformatics exploration of the diagnostic value and molecular mechanism of miR-193a-5p in lung cancer. Oncol Lett 2018;16:4114-28. [Crossref] [PubMed]
- Zhang H, Wang A, Tan Y, et al. NCBP1 promotes the development of lung adenocarcinoma through up-regulation of CUL4B. J Cell Mol Med 2019;23:6965-77. [Crossref] [PubMed]
- Alexandrov A, Martzen MR, Phizicky EM. Two proteins that form a complex are required for 7-methylguanosine modification of yeast tRNA. RNA 2002;8:1253-66. [Crossref] [PubMed]
- Shaheen R, Abdel-Salam GM, Guy MP, et al. Mutation in WDR4 impairs tRNA m(7)G46 methylation and causes a distinct form of microcephalic primordial dwarfism. Genome Biol 2015;16:210. [Crossref] [PubMed]
- Suski JM, Braun M, Strmiska V, et al. Targeting cell-cycle machinery in cancer. Cancer Cell 2021;39:759-78. [Crossref] [PubMed]
- Goel S, DeCristo MJ, Watt AC, et al. CDK4/6 inhibition triggers anti-tumour immunity. Nature 2017;548:471-5. [Crossref] [PubMed]
- Miao D, Margolis CA, Vokes NI, et al. Genomic correlates of response to immune checkpoint blockade in microsatellite-stable solid tumors. Nat Genet 2018;50:1271-81. [Crossref] [PubMed]
- Dersh D, Hollý J, Yewdell JW. A few good peptides: MHC class I-based cancer immunosurveillance and immunoevasion. Nat Rev Immunol 2021;21:116-28. [Crossref] [PubMed]
- Barkal AA, Weiskopf K, Kao KS, et al. Engagement of MHC class I by the inhibitory receptor LILRB1 suppresses macrophages and is a target of cancer immunotherapy. Nat Immunol 2018;19:76-84. [Crossref] [PubMed]
- Zhang CC, Fu YX. Another way to not get eaten. Nat Immunol 2018;19:6-7. [Crossref] [PubMed]
- Jonkhout N, Tran J, Smith MA, et al. The RNA modification landscape in human disease. RNA 2017;23:1754-69. [Crossref] [PubMed]
- Huang H, Weng H, Chen J. m(6)A Modification in Coding and Non-coding RNAs: Roles and Therapeutic Implications in Cancer. Cancer Cell 2020;37:270-88. [Crossref] [PubMed]
- Assouline S, Culjkovic B, Cocolakis E, et al. Molecular targeting of the oncogene eIF4E in acute myeloid leukemia (AML): a proof-of-principle clinical trial with ribavirin. Blood 2009;114:257-60. [Crossref] [PubMed]
- Tan H, He L, Cheng Z. Inhibition of eIF4E signaling by ribavirin selectively targets lung cancer and angiogenesis. Biochem Biophys Res Commun 2020;529:519-25. [Crossref] [PubMed]
(English Language Editor: A. Kassem)