Identification of genes and cellular response factors related to immunotherapy response in mismatch repair-proficient colorectal cancer: a bioinformatics analysis
Highlight box
Key findings
• The current study sought to identify the underlying molecular mechanisms, pathways, and cell landscapes that explain why early-to-mid stage mismatch repair-proficient (pMMR) colorectal cancers (CRCs) respond well to immune checkpoint inhibitors (ICIs).
What is known and what is new?
• ICI treatment has been shown in clinical trials to be ineffective in patients with metastatic pMMR CRC. Recent research suggests that patients with early-stage pMMR may be extremely susceptible to ICI therapy, but the underlying mechanisms remain unknown.
• We investigate the characteristics of the early pMMR CRC tumor microenvironment using The Cancer Genome Atlas (TCGA) CRC data sets and bioinformatics analysis. These characteristics include immune response activation, the existence of anti-tumor cell populations, the absence of pro-metastatic cell populations, and so on.
What is the implication, and what should change now?
• The current tumor microenvironment-based analysis could help identify patients who would benefit from immunotherapeutic treatments.
Introduction
In recent years, the availability of immune checkpoint inhibitors (ICIs) has revolutionized the treatment landscape of cancers, including colorectal cancer (CRC). Based on the outstanding outcomes of several studies, including KEYNOTE-016 (1), the US Food and Drug Administration (FDA) approved pembrolizumab in May 2017 for the treatment of metastatic mismatch repair (MMR)-deficient (dMMR)/microsatellite instability-high (MSI-H) CRCs; more recently, according to the studies of Overman et al. (2) and André et al. (3), pembrolizumab has further received approval for first-line dMMR/MSI-H metastatic CRC (mCRC) treatment. However, because KEYNOTE-016 demonstrated ICIs to be completely ineffective in patients with MMR-proficient (pMMR)/microsatellite-stable (MSS) disease, the use of ICIs in the treatment of pMMR/MSS disease has been limited. The MMR genes are a group of DNA mismatch repair genes. Immunohistochemistry identifies the expression of four common MMR proteins: MLH1, PMS2, MSH2, and MSH6, and normal expression indicates the presence of pMMR. Tumor tissue with pMMR generally has low frequency microsatellite instability (MSI-L) or stability (MSS). Previous studies have concluded that immunotherapy is ineffective against pMMR/MSS CRC. However, recently, some improvements have emerged. The Japanese REGONIVO (Regorafenib and Nivolumab Simultaneous Combination Therapy) study (4) showed that patients with pMMR/MSS disease had a 33% objective response rate (ORR) after combination treatment with regorafenib plus nivolumab. An ORR rate of 27.3% was also obtained with fruquintinib [a vascular endothelial growth factor receptor (VEGFR) inhibitor] in combination with sintilimab [an anti-programmed death 1 (PD-1) antibody] (5). A progression-free survival benefit was observed in patients with pMMR disease in a study of FOLFOXIRI (fluorouracil, leucovorin, oxaliplatin, and irinotecan) plus bevacizumab plus atezolizumab treatment (6). Furthermore, combination with radiotherapy results in ICI sensitization in MSS CRC (7). Importantly, a recent shift in treatment focus from metastatic CRC to early- and mid-stage CRC has provided new research directions. The nivolumab in early stage colorectal cancer (NICOLE) (8) and neoadjuvant immunotherapy in early stage colon cancers (NICHE) studies (9) elegantly demonstrated that at least a subset of early-to-mid stage pMMR colon cancers, previously thought to be resistant to monotherapy with ICIs, are susceptible to ICI-induced immune responses. After at least 2 cycles of preoperative nivolumab therapy in a 22-case group with resectable stage III colon cancer, according to the Response Evaluation Criteria in Solid Tumors (RECIST), the NICOLE study reported that a major pathological response (MPR; 10% viable tumor cells) was observed in 3 pMMR tumors, including 1 pathological complete response (pCR). Four patients with MSS had more than 30% tumor regression. In the NICHE trial, after receiving 1 dose of ipilimumab and 2 doses of nivolumab before surgery, 4 of the 15 (27%) evaluable patients with pMMR colon cancer showed pathological response, with 2 patients (with stage I and IIa disease) achieving pCR and 1 patient (with stage IIIa disease) having only 1% viable tumor cells remaining. Significant tumor regression was seen in 4 other patients (1 patient had stage IIa disease, and 3 patients had stage IIIb disease). These insights have shed new light on pMMR disease. It seems possible that the disease stage will have a significant impact on the efficacy of ICI monotherapy, especially given that ICI therapy use in the early- and mid-stage has achieved such a high ORR compared to late-stage pMMR CRCs.
On the other hand, previous observations in advanced cancer cases have identified that the number of immunosuppressive cells, such as regulatory T cells (Tregs) and myeloid-derived suppressor cells (MDSCs), typically increases according to the tumor volume while T cell function is strongly suppressed (10). Thus, a blunted immune status forms, which dramatically inhibits the ICI response. In addition, the biomarker found to predict ICI response in CRC tumor microenvironment (TME) was the presence of T cells with coexpression of CD8 and PD1 (9). Therefore, we hypothesize that a TME suitable for ICI treatment exists in early pMMR CRCs. A comparison of different factors within the TME in early- and late-stage disease may provide a reasonable explanation for the efficacy of ICIs and identify potential biomarkers to improve ICI therapy efficacy in pMMR CRC.
Mechanistic studies have shown that the TME within dMMR/MSI-H CRC tissues is mainly immune inflammatory, while in pMMR/MSS CRC, the TME is mainly of the immune-desert type or immune-excluded type (11). Briefly, dMMR is a state of “hyperimmunogenicity” that may be required for ICI effectiveness. Correspondingly, early-stage pMMR CRCs also have similar characteristics within the TME. T cell infiltration (TCI), a lower tumor burden, and a lower degree of systemic immune suppression have all been identified as characteristics possibly related to the better response rates in early disease compared to late disease (12). In the NICHE trial, the presence of T cells with CD8 and PD-1 coexpression was the biomarker that predicted an effective response in pMMR tumors, and strong COX or TGF-beta expression, which creates a suppressive TME, were identified as likely biomarkers of nonresponse (9). Moreover, patients with significantly increased immunoscores (13), defined by CD3+ and CD8+ T cell infiltration after ICI treatment, had better outcomes (8). However, because of the small sample sizes in both of these studies, these markers could not be quantified, limiting the potential for pretreatment clarification. Other published biomarkers for pMMR/MSS CRC immunotherapy include the combined positive score (CPS) (14) and tumor mutation burden (TMB) (15), but their thresholds and cutoff values are inconsistent across studies and platforms. POLE/POLD1 mutations have been shown to have potential predictive value for ICI treatment (16). However, only 1–3% of CRCs have been found to have POLE mutations (17). Overall, ICI response markers in pMMR/MSS CRC are still lacking.
Given the strong relationship between TNM stage and ICI response, we hypothesize that there may be a series of expression profiles of specific genes that can characterize the pMMR CRC disease stage and that dynamic changes in these expression profiles may provide insights into good ICI response in early- or mid-stage pMMR CRC. Using The Cancer Genome Atlas (TCGA) CRC [colon adenocarcinoma (COAD) and rectal adenocarcinoma (READ)] data sets, we first investigated the transcriptomic features that continuously changed (continuous upregulation or downregulation) with pMMR CRC disease-stage progression. We defined such gene sets as stage-associated genes (abbreviated as stage genes). Enrichment analysis revealed that the stage genes were primarily enriched in the immune response, implying that significant cancer immunoediting (18) occurs in the TME during disease progression. Through deconvolution analysis, we identified 2 main disease stage-associated alterations in the cellular composition of the pMMR CRCs, including alterations of cell types that are involved in host immune responses and tumor cell metastasis. The stage genes were then cross-referenced to current transcriptome profile data on the ICI treatment of pMMR CRC, which revealed the gene set specifying an effective pMMR tumor response. The current study sought to identify the underlying molecular mechanisms, pathways, and cell landscapes that explain why early- and mid-stage pMMR CRC responds well to ICI treatment. This kind of TME-based analysis might be valuable for the selection of patients who would benefit from immunotherapeutic strategies. We present the following article in accordance with the MDAR reporting checklist (available at https://jgo.amegroups.com/article/view/10.21037/jgo-22-1070/rc).
Methods
Data accession
The workflow of the present study is presented in Figure 1. RNA sequencing (RNA-seq) data for COAD and READ were downloaded from TCGA data portal (portal.gdc.cancer.gov/repository). We retained 398 pMMR samples that contained complete clinical information (including age, sex, status, survival time, T stage, N stage, M stage, and overall TNM stage). We also retained 65 dMMR samples that contained complete clinical information (including age, sex, status, survival time, T stage, N stage, M stage, and overall TNM stage; Table S1). MMR and MSS status were obtained by downloading clinical data from COAD and READ patients. Samples with incomplete information were excluded. GSE146771 was used to obtain single-cell RNA transcriptome sequences of immune and stromal cell types from the human CRC TME (19). Transcriptomic profiles of pMMR patients treated with ICIs were obtained from GSE179351 (7). In the Gene Expression Omnibus (GEO) database, we found only 1 patient with transcriptional profile data who achieved a pCR after ICI therapy (the patient was treated with 2 types of ICIs plus radiation; from the GSE179351 data set). Therefore, we used the R package “edgeR” (version 3.34.1; The R Foundation for Statistical Computing) (20) to compare the differential expression between the transcriptome profiles pre- and post-ICI treatment. This methodology can be used even with minimal levels of replication, provided that at least 1 phenotype or experimental condition is replicated. According to the suggestion of the “edgeR” package, we set the BCV (square-root-dispersion) to 0.4 and the significance threshold to P<0.05. We downloaded a table of differential gene expression in melanoma treated with ICIs from the online supplementary materials of the manuscript of Gide et al. (21). Transcriptomic profiles of non-small cell lung cancers (NSCLCs) treated with ICI therapy were obtained from GSE126044 (22). The study was conducted in accordance with the Declaration of Helsinki (as revised in 2013).
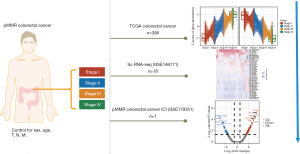
Identification of stage-associated genes in CRC
For visualization of adjusted expression levels, the raw counts were processed by variance-stabilizing transformation and a likelihood-ratio test (LRT) with DESeq2 (v. 1.32.0) (23), which was followed by a false discovery rate (FDR) adjustment for sex, age, T stage , N stage, and M stage using the remove batch effect function in “limma” (24) (v. 3.48.3). All boxplots were Tukey boxplots, with interquartile range (IQR) boxes and 1.5 × IQR whiskers. Stage-associated genes were determined at a significance threshold of P<0.05. The significant genes were scaled to Z scores and clustered using the “degPatterns” function of the R package “DEGreport” (v. 1.28.0). We retained the genes that changed in response to the TNM stages, which we termed stage-associated genes.
Immune and stromal cell population single-cell RNA-seq data analysis
We analyzed single-cell RNA-seq (scRNA-seq) data using the R package “Seurat” (v. 4.0.4) (25) in accordance with the methods proposed by Chow et al. (26). Of the 1,619 (774 genes with increasing expression and 845 genes with decreasing in expression with TNM stage) stage genes identified from the COAD and READ data set bulk transcriptomes, 901 genes were matched in the scRNA-seq data generated by Zhang et al. (19). The expression matrices were converted into binary matrices by setting a threshold of expression of >0 to determine the percentage of cells expressing a given gene. Specified cell type annotations (19) were then used to compute cell type-specific expression frequencies for each gene. We subsequently scaled the expression frequencies in R to obtain Z scores to identify the genes that were preferentially expressed in a given cell type. The R package “ComplexHeatmap” (v. 2.8.0) (27) was used to visualize cell type-specific expression frequencies.
Enrichment analysis
Gene ontology (GO) and Kyoto Encyclopedia of Genes and Genomes (KEGG) pathway analyses were conducted by using Metascape (database last update date: November 1, 2021) (28). The parameter settings were the following: P value cutoff =0.01, min overlap =3, and min enrichment =1.5.
Inferring the cellular composition and dynamic changes in CRC
We used the CIBERSORTx algorithm (29) to infer the cellular composition of each CRC bulk transcriptome. A signature matrix file was built from scRNA-seq data as a reference (19). Since our single-cell data set contained smart-seq data, we used B model batch correction to calculate the proportions of cell types in each bulk sample. We filtered the cell types according to the percentage of samples with a nonzero proportion, and we retained at least 50% of the cell types with an estimated percentage greater than 0. By controlling for sex, age, and T stage, N stage, and M stage, we identified the changes in the proportions of several cell types in CRC related to TNM stage. We used the R package “MASS” (v. 7.3.54) to estimate an ordered logistic regression model.
Statistical analysis
All the statistics and figures in the paper were generated with R software (v. 4.1.0). LRT and FDR were used to identify stage-associated genes. The ordinal logistic regression model, a generalization of the nonparametric Kruskal-Wallis test that allows for multifactorial designs, was used to assess the statistical significance of TNM stage and cell types. A P value <0.05 indicated statistical significance.
Results
We focused our analysis on pMMR-subtype samples in the COAD and READ data sets from TCGA database. We investigated the RNA-seq results of tumor tissues from donors with diseases of different TNM stages because we hypothesized that a set of specific gene expression patterns could be used to characterize the stage of pMMR CRCs. A total of 398 COAD and READ RNA-seq profiles from 398 donors with pMMR disease were compiled, and complete clinical information was available (sex, age, and TNM stage; Table S1).
Identification of stage-associated genes in pMMR CRC
Previous studies have shown that sex (30) and age (31) may affect gene expression independent of cancer TNM stage. In addition, T, N, and M stage have been shown to be obviously directly associated to TNM stage. Therefore, we used LRT followed by statistical adjustment for sex, age, and T stage, N stage, and M stage to determine the genes whose expression was significantly affected by TNM stage (P value <0.05; https://cdn.amegroups.cn/static/public/jgo-22-1070-1.xlsx). We identified 2 clusters of genes whose expression progressively changed with TNM stage (Figure 2A). In total, 774 genes were found to increase in expression with increasing stage, while 845 genes decreased in expression with increasing stage (hereafter referred to as stage genes). Interestingly, GO and pathway analysis of the stage genes revealed significant enrichment of immune-related functions, including the terms immunoglobulin complex, antigen binding, adaptive immune response, and immunoglobulin receptor binding (Figure 2B). These data suggested that the development of tumors is associated with architectural immune editing in TME. These findings are consistent with those stating that the immune system plays a significant role in cancer progression (32). On the one hand, the immune system can suppress tumor growth by destroying cancer cells or inhibiting their outgrowth; on the other hand, it also promotes tumor progression either by selecting tumor cells that are more fit to survive in an immunocompetent host or by establishing conditions within the TME that facilitate tumor outgrowth (32).
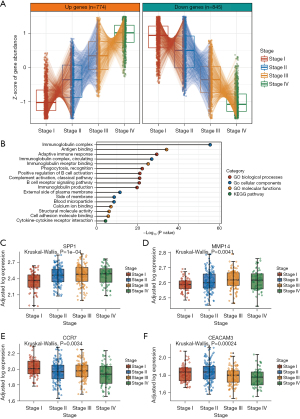
Among the increasing stage genes annotated, SPP1 and MMP14 showed strong stage-associated increases in expression (Figure 2C,2D). Myeloid and tumor cell-expressed SPP1 acts as an immune checkpoint to suppress T cell activation and confer host tumor immune tolerance (33), while MMP14 is involved in cell migration, invasion, metastasis, angiogenesis, and proliferation during tumor progression, with MMP14 blockade limiting tumor neoangiogenesis and hypoxia, in addition to increasing detection of cytotoxic immune cell markers (34). Of the decreasing stage genes annotated, CCR7 and CEACAM3 were among those with the strongest stage-associated decreases in expression (Figure 2E,2F). The CCL19/CCL21-CCR7 chemokine axis is significantly engaged in the trafficking of a number of effector cells involved in mounting an immune response to a growing tumor (35). CEACAM3 encodes a transmembrane protein and is thought to play an important role in controlling human-specific pathogens via the innate immune system (36).
Cell type-specific characterization of stage-associated genes
We then focused on analyzing the microenvironment of pMMR CRCs of different stages since the stage genes were mainly enriched in immune-related functions. These results implied that the genes that change most dramatically with disease progression are those related to the cross-talk between tumor cells and TME immune components. We used single-cell data of immune and stromal cell populations in CRC (19) to identify the cell types that normally express the stage genes. By examining the scaled percentage of expressing cells within each annotated cell subset, we identified that the stage genes were predominantly expressed in several cell types. Surprisingly, the cell types that were highly enriched for certain increasing stage genes and decreasing stage genes were practically identical. This result suggests that changes in gene expression profiles, whether upregulation or downregulation, are likely to reflect changes in the same cell types that accompany disease progression. Endothelial cells expressing CDH5, myofibroblasts expressing ACTA2, CD4+ T lymphocyte cells expressing FOXP3, natural killer (NK) cells expressing CD103, and several endothelial cell subtypes had highly enriched expression of stage genes (Figure 3A,3B, https://cdn.amegroups.cn/static/public/jgo-22-1070-2.xlsx).
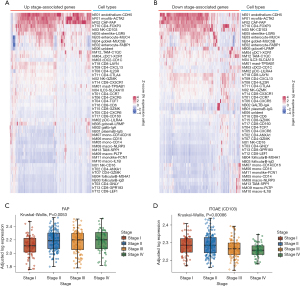
Fibroblast activation protein (FAP) showed particularly substantial stage-associated increases in expression among the genes associated with enriched FAP in cancer-associated fibroblasts (CAFs) that were annotated as increasing stage genes (Figure 3C). A previous study found that FAP activates a CAF subset with an inflammatory phenotype, which contributes to highly aggressive liver cancer with dense desmoplastic stroma, and that elevated levels of stromal FAP predict a poor survival outcome (37). In CRC, CAFs with high FAP expression have increased CCL2 secretion, recruit myeloid cells, and decrease T cell activity in the tumor immune microenvironment (38).
One of the enriched genes among the NK cell genes that decreased in expression with increasing stage was CD103 [also known as integrin subunit alpha e (ITGAE); Figure 3D]. In the small intestine of infants, CD103+ NK cells are the most prevalent innate lymphoid cell population and have a robust effector phenotype, expression of perforin and granzyme B, and greater degranulation capabilities (39). High CD103 expression in CRCs is related to increased immune activity and can predict longer disease-free survival and overall survival (40).
Taken together, the integrative analysis of bulk and single-cell transcriptomes showed that many of the stage gene changes in pMMR CRC could be mapped to specific cell subpopulations, implying that the abundance of these cell types, their transcriptional statuses, or both may be altered with disease stage.
Dynamic changes in the cellular landscape related to pMMR CRC progression
Since tumor immunotherapy involves complex interactions between various cell types, dynamic changes in the cellular landscape as the disease advances could be important for understanding the poor immunotherapy response of late pMMR CRCs. Therefore, to assess the dynamic alterations in cell types, we deconvoluted pMMR CRC stage gene bulk transcriptomes with CIBERSORTx (29) using the scRNA-seq data from Zhang et al. as a reference (https://cdn.amegroups.cn/static/public/jgo-22-1070-3.xlsx) (19). We prefiltered and preserved those cell types with estimated proportions greater than 0 in at least 50% of the pMMR CRC samples (27 out of 48 total cell types; Figure S1) to gain confidence in subsequent analyses because some cell types may not reappear in 2 different samples due to tumor heterogeneity, and cell types can have overlapping gene expression profiles. We then identified changes in the proportions of several cell types in the CRCs related to disease stage (Figure 4).
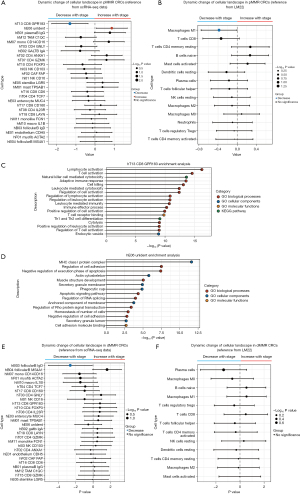
The proportions of the T cell population CD8_GPR183 decreased with increasing CRC stage, while the proportion of the endothelial cell population Unident increased. According to the annotation of Zhang et al. (19), CD8_GPR183 belongs to the central memory CD8+ T cell population, which is a precursor population of effector memory T cells. The latter have long been recognized as major mediators of tumor protection in peripheral tissues (41). Notably, memory CD8+ T cells are the main effectors of antitumor immunity because they can survive and function in host tissues and tumors for long periods of time (42). Functional enrichment analysis of CD8_GPR183 marker genes, for which the activated pathways were most related to lymphocyte activation and cell killing, emphasized the significance of this cell population in antitumor immunity (Figure 4C). On the other hand, we analyzed the enrichment of marker genes in hE06_Unident and discovered that they were largely enriched in cell adhesion regulation, actin cytoskeleton, negative regulation of execution phase of apoptosis, and other terms (Figure 4D). These characteristics are associated with tumor proliferation and metastasis. Similar results were found by using an independent leukocyte signature matrix (LM22) (43) from the CIBERSORT database (cibersortx.stanford.edu; Figure 4B, https://cdn.amegroups.cn/static/public/jgo-22-1070-4.xlsx). M1 macrophages, a type of antitumor immune cell, decreased with stage progression, as did CD8+ T cells, although not significantly (Figure 4B). In general, this type of cellular landscape reflects the dynamic decline of the population of cells that enhance the tumor immune response during the progression of the disease while the population of cells that promote metastasis gradually becomes dominant. These findings are in line with recent findings that the establishment of an immunologically cold TME over time in advanced disease inevitably reduces the efficacy of ICI therapy (44). Thus, stage-associated alterations in specific pMMR CRC immune and stromal cell populations may contribute to the relationship between disease stage and ICI therapy response.
Although we analyzed the dMMR CRCs, we did not find trends indicating the establishment of an immunologically cold TME with advancing disease (Figure 4E,4F). The evolving cell landscape of the disease stage in dMMR CRCs has little to do with immune function. The only population of cells that declined significantly as the disease progressed was hB03_FollicularB_IgD. Functional enrichment analysis of marker genes in the hB03_FollicularB_IgD cell population identified terms most related to ribosome function, such as cytosolic ribosome, cytoplasmic translation, and ribosomal subunit (Figure S1). Thus, stage-associated alterations in dMMR CRC immune and stromal cell populations may not significantly affect antitumor immune function in the TME. This finding explains why dMMR CRCs have been observed to have a significant therapeutic response to ICIs, at least in most cases, regardless of whether the disease is early stage (9) or late stage (1).
ICI response-associated factors for pMMR CRC
In tumors that respond well to ICI treatment, researchers (9,45) have found that immune activation in tumor tissues, such as increases in CD8+ T cell counts, T cell receptor clonality, interferon (IFN)-gamma score, and CXCL13 expression, can be seen after the start of ICI therapy. Therefore, we hypothesized that alterations in gene expression profiles in the TME can characterize such activation. We first compared the pretreatment and posttreatment differences in gene expression in patients carrying pMMR tumors who achieved an effective ICI response. We only found 1 such patient in the GEO database because pMMR patients with good ICI monotherapy outcomes are extremely rare. This patient was from a phase II trial (NCT03104439; GSE179351) that combined radiation, ipilimumab, and nivolumab to treat patients with metastatic pMMR CRC; this patient was the only patient out of 40 to achieve a metastatic lesion complete response (7). An analysis of expression profiles comparing pre- and post-ICI treatment revealed that 164 genes were significantly upregulated and 83 genes were significantly downregulated (Figure 5A, https://cdn.amegroups.cn/static/public/jgo-22-1070-5.xlsx). We assume that these genes are associated with the pMMR tumor ICI response. Enrichment analysis showed that the gene expression profile change induced by ICI treatment mainly affected immune response signatures (Figure 5B). In line with this result and similar to the enrichment of stage genes in specific cell types (Figure 3A,3B), the top 5 cell types that normally express these ICI response–associated genes were identified as CD4+ T cells expressing FOXP3, NK cells expressing CD103, CAFs expressing FAP, endothelial cells expressing CDH5, and myofibroblasts expressing ACTA2 (Figure 5C). These findings help to explain why ICIs are effective in treating a small subset of pMMR CRCs; that is, effective ICI therapy requires establishing or restoring antitumor immunity in the local microenvironment.
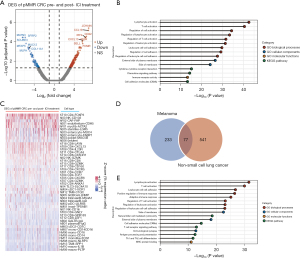
Next, we sought to determine whether similar establishment or rescue of antitumor immunity could be observed in other solid tumor types. We investigated ICI treatment data for melanoma (21) and NSCLC (GSE126044) (22). An analysis of the transcriptional expression profiles of pretreatment melanoma patients comparing 22 responders to 19 nonresponders revealed 229 genes with significantly high expression and 81 genes with significantly low expression in responders (https://cdn.amegroups.cn/static/public/jgo-22-1070-6.xlsx). When comparing 5 responders to 11 nonresponders in the case of NSCLC, it was discovered that 499 genes were expressed at high levels and 119 genes were expressed at low levels in responders (https://cdn.amegroups.cn/static/public/jgo-22-1070-7.xlsx). We performed an intersection analysis of these 2 groups of differentially expressed genes (DEGs) to rule out disease-specific genes, with the genes in the intersection assumed to be genes associated to ICI response (Figure 5D). Ultimately, the Wayne diagram depicted 77 genes associated with ICI response (Figure 5D, https://cdn.amegroups.cn/static/public/jgo-22-1070-8.xlsx). Further enrichment analysis (Figure 5E) revealed that the enriched pathways and annotations of these ICI response genes nearly entirely overlapped with those of the pMMR CRC ICI response genes (Figure 5B). This finding, along with the findings presented in previous sections, suggests that the ICI response is dependent on immune-related processes activated in the TME in early-to-midstage pMMR CRCs, metastatic pMMR CRCs, or other solid tumors. Our findings support previous findings from microenvironment-based analyses of immune infiltration signatures in solid tumors (46), indicating that such an assessment could be useful for identifying those pMMR patients who could benefit from immunotherapeutic strategies.
Identification of the pMMR CRC ICI response gene set
Finally, because disease stage has a significant impact on immunotherapy efficacy and the stage genes obviously include a collection of genes that only affect stage and not immunotherapy, we used the intersection of stage genes and pMMR CRC ICI response genes to narrow the scope of biomarkers even further. We identified 27 genes at the intersection (Figure 6A, https://cdn.amegroups.cn/static/public/jgo-22-1070-9.xlsx). Further GO and pathway analyses of these overlapping genes revealed significant enrichment of immune response terms, such as leukocyte differentiation, lymphocyte activation, T cell selection, and immune receptor activity (Figure 6B). The cell types that express these overlapping genes in the TME were identified as primarily suppressive cells, such as CD4+ T cells expressing FOXP3 (Figure 6C). Next, the 27 overlapping genes in the pMMR CRC pre-ICI treatment cohort of Parikh et al. (7) were assessed to determine whether their expression patterns could predict the efficacy of immunotherapy. As indicated in the unsupervised clustering heatmap in Figure 6D, these expression patterns are indeed predictive. The expression patterns of the 27 genes in the pretreatment transcriptional profiles were found to be completely different from those of patients who did not respond to ICI therapy or had pCR. Furthermore, the fact that 20 genes were upregulated and 7 genes were downregulated after ICI treatment (Figure 6D) suggests that a salvageable TME has predictive value for the response to ICI treatment. On the other hand, 8 out of the 27 genes were found to overlap with previously identified immune cell gene signatures (47), and 4 of these overlapping genes (CCR7, CD27, SASH3, and UBASH3A) were found to belong to T cell gene signatures. These findings highlight the significance of T cell activity in ICI efficacy and suggest that alteration of the expression pattern of the stage genes of pMMR CRC may significantly regulate the ICI response.
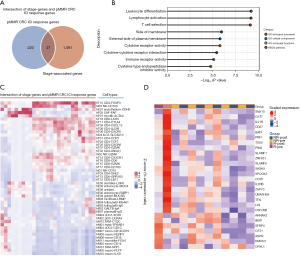
Discussion
dMMR/MSI-H CRC can now be treated with ICIs to induce long-lasting tumor responses even after progression following administration of standard chemotherapy agents (11). However, patients with dMMR/MSI-H make up only 15% of all patients with CRC (3). To overcome immunotherapy resistance in the remaining 85% of those with pMMR/MSS, it is necessary to analyze the immunological makeup in-depth and in the context of the tumor genetic makeup. In this study, based on impressive findings from patients with early-stage pMMR in the NICOLE and NICHE trials, we linked TNM stage with ICI response. Via analysis of transcriptomic profiles and deconvolution methods, we found that the central memory CD8+ T cell population decreased with increasing pMMR CRC disease stage. When using another cell type reference data (LM22), we discovered that M1 macrophages and CD8+ T cells decreased in the TME as the disease progressed. Conversely, such a decrease was absent in patients with dMMR CRC. By investigating the TME components, we provided an explanation for why ICIs exhibit some efficacy in early- and mid-stage pMMR CRC: ICI efficacy requires pre-existing strong immune infiltration or rescue of an efficient antitumor immune response. Furthermore, by cross-referencing our stage gene set and ICI response gene set, we identified 27 signature genes that can be used to assess ICI efficacy in treatment-naïve pMMR CRC patients. Although the results are significant, the 27 signature genes need to be further validated in larger pMMR CRC samples.
The stage-associated genes identified in our study were mainly enriched in immune-related functions. Interestingly, Mlecnik et al. (46) reported that the DEGs of MSI-H and MSS CRC samples were also associated with immune response functions. They further identified a good prognostic subgroup of MSS patients with high intratumoral immune gene expression whose transcriptional profiles were similar to those of their MSI-H counterparts (46). Due to immunogenic neoepitopes generated by hypermutation, MSI-H tumors are presumably highly antigenic, thus attracting a large amount of infiltrating lymphocytes. The mechanism underlying good prognosis in this MSS subgroup is thought to be similar to MSI-H tumors (46). In contrast, as identified in our study, changes leading to an immunosuppressive TME as a result of disease stage progression should help to explain the poor prognosis and why ICIs are ineffective in advanced pMMR CRC. Our study extends the findings of Mlecnik et al. at the level of disease stage. To increase clinical benefit, researchers have sought to reverse the blunted immune status and reinvigorate immunity. These immune-activating strategies specific for pMMR CRC mainly include combinations with COX-2 inhibitors (9), radiotherapy (7), or depletion of protumorigenic or immunosuppressive cells (19) to reduce tumor load and increase immunogenicity. Our observation of dynamic changes in the cellular landscape when comparing the TME of patients with dMMR and pMMR tumors supports these strategies. Even with stage progression of dMMR CRC, there was no significant decline in antitumor immune cell populations within the TME, suggesting that an “immune-hot” microenvironment always exists, and thus, a favorable immunotherapy outcome is likely. Additionally, we identified that the proportions of endothelial cells that regulate cell adhesion and proliferation were increased within the intratumoral TME with increasing disease stage. Clinical trials with strategies targeting VEGFR in combination with ICIs are now ongoing. According to the REGONIVO study (4) and Guo et al. (5), patients with pMMR/MSS had 33% and 27.3% ORRs after the combination treatment regimens, respectively. These clinical data confirm that targeting VEFGR on endothelial cells is a very promising ICI sensitization strategy.
Current cancer classification is provided by the American Joint Committee on Cancer/Union for International Cancer Control (AJCC/UICC) and strictly relies on tumor characteristics, such as the extent of the primary tumor (T stage), the involvement of regional lymph nodes (N stage), and the presence of distant metastases (M stage; collectively, TNM stage). An extensive and comprehensive review previously indicated that the TNM staging system does not provide insight into the immune status of a tumor and therefore may not predict the response to immunotherapy (13). However, the NICHE study clearly shows that there is a window of opportunity for ICIs as neoadjuvant treatment for patients with early-to-midstage MSS/pMMR colon cancer (9). These insights have provided a new perspective, and the evaluation of ICI response markers, which has been typically limited to MMR, MSI, and TMB, may need to be updated. The analysis of the TME provides valuable new information for identifying patients who might benefit from immunotherapeutic treatments. For example, in TME of ICI-treated effective patients, there was a significant increase in CD8+ CD3+ T-cell infiltration as well as in IFN-γ scores after ICI treatment compared to pretreatment biopsies in surgical specimens (9). The current study further provided an explanation of the global transcriptome expression profile and cell type dynamic changes in the TME underlying stage-associated ICI responses. The TNM staging system once again proved useful. However, why ICIs are so important in early- or mid-stage patients with limited disease when they may be cured with surgery alone remains to be determined. Currently available evidence indicates that 30% of stage I–III patients who initially receive curative treatment by surgery develop recurrent disease (48). Nonsurgical intervention could be beneficial given that the immune suppression associated with surgical wound repair can enable the revival and dissemination of occult metastases (49). Importantly, long-term follow-up data showed that a subset of patients survived for ≥10 years after ICI treatment (50). Considering the potential of immunotherapy to achieve long-term durable responses, the need for biomarkers that can distinguish tumors that will and will not respond to immunotherapy is apparent. However, to date, reliable biomarkers for guiding immunotherapy remain to be established. The present study may provide a further step in that direction.
We emphasize that the analyses presented here have some limitations that must be considered before interpreting the results. First, there is currently an insufficient amount of available data to directly assess the microenvironment of ICI treatment-naïve pMMR CRC. In the GEO database, we found only 1 patient with transcriptional profile data who achieved pCR. Therefore, although we excluded differences between individuals, we could only directly compare the characteristics of transcriptional spectrum changes in the TME of this patient pre- and post-ICI treatment, providing some clues for the establishment or rescue of an efficient antitumor immune response. Second, immune cell transcriptome signatures are made up of lists of marker genes that indicate the presence of a specific immune cell population (29). However, in any given tissue, a gene may be expressed by multiple cell types present therein, or a cell type may not be present. In fact, there are currently few consensus lists of immune or stromal markers available (47). To increase the confidence, we used the CRC reference TME cell set from Zhang et al., which was generated from the single-cell sequencing of immune and stromal cell types in CRC tissues (19), but the current findings require more clinical and experimental data for further validation.
Conclusions
The current study identified disease stage-associated dynamic changes in the genomic and cellular landscape associated with pMMR CRC progression. As the pMMR CRC disease stage increased, the central memory CD8+ T cell population decreased, but endothelial cell populations associated with proliferation and metastasis increased. Using a different cell type annotation set (LM22), we were able to replicate these findings. In contrast, this trend was not observed in patients with dMMR CRC. We provided an explanation for why ICIs have some efficacy in early- and mid-stage pMMR CRC by investigating the TME components: ICI efficacy is dependent on pre-existing strong immune infiltration or the rescue of an effective antitumor immune response. Furthermore, by cross-referencing our stage gene set to the ICI response gene set, we discovered 27 signature genes that can be used to assess ICI efficacy in treatment-naive pMMR CRC patients. Despite the fact that additional large-scale experimental verification is required, our analysis might be valuable for the selection of patients who might benefit from immunotherapeutic strategies.
Acknowledgments
We would like to thank the contributions of the GEO and TCGA for providing free access to online data.
Funding: None.
Footnote
Reporting Checklist: The authors have completed the MDAR reporting checklist. Available at https://jgo.amegroups.com/article/view/10.21037/jgo-22-1070/rc
Conflicts of interest: Both authors have completed the ICMJE uniform disclosure form (available at https://jgo.amegroups.com/article/view/10.21037/jgo-22-1070/coif). The authors have not conflict of interest to declare.
Ethical Statement: The authors are accountable for all aspects of the work in ensuring that questions related to the accuracy or integrity of any part of the work are appropriately investigated and resolved. The study was conducted in accordance with the Declaration of Helsinki (as revised in 2013).
Open Access Statement: This is an Open Access article distributed in accordance with the Creative Commons Attribution-NonCommercial-NoDerivs 4.0 International License (CC BY-NC-ND 4.0), which permits the non-commercial replication and distribution of the article with the strict proviso that no changes or edits are made and the original work is properly cited (including links to both the formal publication through the relevant DOI and the license). See: https://creativecommons.org/licenses/by-nc-nd/4.0/.
References
- Le DT, Uram JN, Wang H, et al. PD-1 Blockade in Tumors with Mismatch-Repair Deficiency. N Engl J Med 2015;372:2509-20. [Crossref] [PubMed]
- Overman MJ, Lonardi S, Wong KYM, et al. Durable Clinical Benefit With Nivolumab Plus Ipilimumab in DNA Mismatch Repair-Deficient/Microsatellite Instability-High Metastatic Colorectal Cancer. J Clin Oncol 2018;36:773-9. [Crossref] [PubMed]
- André T, Shiu KK, Kim TW, et al. Pembrolizumab in Microsatellite-Instability-High Advanced Colorectal Cancer. N Engl J Med 2020;383:2207-18. [Crossref] [PubMed]
- Fukuoka S, Hara H, Takahashi N, et al. Regorafenib Plus Nivolumab in Patients With Advanced Gastric or Colorectal Cancer: An Open-Label, Dose-Escalation, and Dose-Expansion Phase Ib Trial (REGONIVO, EPOC1603). J Clin Oncol 2020;38:2053-61. [Crossref] [PubMed]
- Guo Y, Zhang W, Ying J, et al. Preliminary results of a phase 1b study of fruquintinib plus sintilimab in advanced colorectal cancer. J Clin Oncol 2021;39:2514. [Crossref]
- Cremolini C, Rossini D, Antoniotti C, et al. LBA20 FOLFOXIRI plus bevacizumab (bev) plus atezolizumab (atezo) versus FOLFOXIRI plus bev as first-line treatment of unresectable metastatic colorectal cancer (mCRC) patients: Results of the phase II randomized AtezoTRIBE study by GONO. Ann Oncol 2021;32:S1294-5. [Crossref]
- Parikh AR, Szabolcs A, Allen JN, et al. Radiation therapy enhances immunotherapy response in microsatellite stable colorectal and pancreatic adenocarcinoma in a phase II trial. Nat Cancer 2021;2:1124-35. [Crossref] [PubMed]
- Avallone A, De Stefano A, Pace U, et al. 491P Neoadjuvant nivolumab in early stage colorectal cancer. Ann Oncol 2020;31:S449. [Crossref]
- Chalabi M, Fanchi LF, Dijkstra KK, et al. Neoadjuvant immunotherapy leads to pathological responses in MMR-proficient and MMR-deficient early-stage colon cancers. Nat Med 2020;26:566-76. [Crossref] [PubMed]
- Tcyganov E, Mastio J, Chen E, et al. Plasticity of myeloid-derived suppressor cells in cancer. Curr Opin Immunol 2018;51:76-82. [Crossref] [PubMed]
- Breakstone R. Colon cancer and immunotherapy-can we go beyond microsatellite instability? Transl Gastroenterol Hepatol 2021;6:12. [Crossref] [PubMed]
- Forde PM, Chaft JE, Smith KN, et al. Neoadjuvant PD-1 Blockade in Resectable Lung Cancer. N Engl J Med 2018;378:1976-86. [Crossref] [PubMed]
- Angell HK, Bruni D, Barrett JC, et al. The Immunoscore: Colon Cancer and Beyond. Clin Cancer Res 2020;26:332-9. [Crossref] [PubMed]
- Alexander PG, McMillan DC, Park JH. A meta-analysis of CD274 (PD-L1) assessment and prognosis in colorectal cancer and its role in predicting response to anti-PD-1 therapy. Crit Rev Oncol Hematol 2021;157:103147. [Crossref] [PubMed]
- Meiri E, Garrett-Mayer E, Halabi S, et al. Pembrolizumab (P) in patients (Pts) with colorectal cancer (CRC) with high tumor mutational burden (HTMB): Results from the Targeted Agent and Profiling Utilization Registry (TAPUR) Study. J Clin Oncol 2020;38:133. [Crossref]
- Gong J, Wang C, Lee PP, et al. Response to PD-1 Blockade in Microsatellite Stable Metastatic Colorectal Cancer Harboring a POLE Mutation. J Natl Compr Canc Netw 2017;15:142-7. [Crossref] [PubMed]
- He J, Ouyang W, Zhao W, et al. Distinctive genomic characteristics in POLE/POLD1-mutant cancers can potentially predict beneficial clinical outcomes in patients who receive immune checkpoint inhibitor. Ann Transl Med 2021;9:129. [Crossref] [PubMed]
- Dunn GP, Bruce AT, Ikeda H, et al. Cancer immunoediting: from immunosurveillance to tumor escape. Nat Immunol 2002;3:991-8. [Crossref] [PubMed]
- Zhang L, Li Z, Skrzypczynska KM, et al. Single-Cell Analyses Inform Mechanisms of Myeloid-Targeted Therapies in Colon Cancer. Cell 2020;181:442-459.e29. [Crossref] [PubMed]
- Robinson MD, McCarthy DJ, Smyth GK. edgeR: a Bioconductor package for differential expression analysis of digital gene expression data. Bioinformatics 2010;26:139-40. [Crossref] [PubMed]
- Gide TN, Quek C, Menzies AM, et al. Distinct Immune Cell Populations Define Response to Anti-PD-1 Monotherapy and Anti-PD-1/Anti-CTLA-4 Combined Therapy. Cancer Cell 2019;35:238-255.e6. [Crossref] [PubMed]
- Cho JW, Hong MH, Ha SJ, et al. Genome-wide identification of differentially methylated promoters and enhancers associated with response to anti-PD-1 therapy in non-small cell lung cancer. Exp Mol Med 2020;52:1550-63. [Crossref] [PubMed]
- Love MI, Huber W, Anders S. Moderated estimation of fold change and dispersion for RNA-seq data with DESeq2. Genome Biol 2014;15:550. [Crossref] [PubMed]
- Ritchie ME, Phipson B, Wu D, et al. limma powers differential expression analyses for RNA-sequencing and microarray studies. Nucleic Acids Res 2015;43:e47. [Crossref] [PubMed]
- Hao Y, Hao S, Andersen-Nissen E, et al. Integrated analysis of multimodal single-cell data. Cell 2021;184:3573-3587.e29. [Crossref] [PubMed]
- Chow RD, Majety M, Chen S. The aging transcriptome and cellular landscape of the human lung in relation to SARS-CoV-2. Nat Commun 2021;12:4. [Crossref] [PubMed]
- Gu Z, Eils R, Schlesner M. Complex heatmaps reveal patterns and correlations in multidimensional genomic data. Bioinformatics 2016;32:2847-9. [Crossref] [PubMed]
- Zhou Y, Zhou B, Pache L, et al. Metascape provides a biologist-oriented resource for the analysis of systems-level datasets. Nat Commun 2019;10:1523. [Crossref] [PubMed]
- Newman AM, Steen CB, Liu CL, et al. Determining cell type abundance and expression from bulk tissues with digital cytometry. Nat Biotechnol 2019;37:773-82. [Crossref] [PubMed]
- Haupt S, Caramia F, Klein SL, et al. Sex disparities matter in cancer development and therapy. Nat Rev Cancer 2021;21:393-407. [Crossref] [PubMed]
- Cook MB, Hurwitz LM, Geczik AM, et al. An Up-to-date Assessment of US Prostate Cancer Incidence Rates by Stage and Race: A Novel Approach Combining Multiple Imputation with Age and Delay Adjustment. Eur Urol 2021;79:33-41. [Crossref] [PubMed]
- Schreiber RD, Old LJ, Smyth MJ. Cancer immunoediting: integrating immunity's roles in cancer suppression and promotion. Science 2011;331:1565-70. [Crossref] [PubMed]
- Klement JD, Paschall AV, Redd PS, et al. An osteopontin/CD44 immune checkpoint controls CD8+ T cell activation and tumor immune evasion. J Clin Invest 2018;128:5549-60. [Crossref] [PubMed]
- Ling B, Watt K, Banerjee S, et al. A novel immunotherapy targeting MMP-14 limits hypoxia, immune suppression and metastasis in triple-negative breast cancer models. Oncotarget 2017;8:58372-85. [Crossref] [PubMed]
- Salem A, Alotaibi M, Mroueh R, et al. CCR7 as a therapeutic target in Cancer. Biochim Biophys Acta Rev Cancer 2021;1875:188499. [Crossref] [PubMed]
- Sintsova A, Guo CX, Sarantis H, et al. Bcl10 synergistically links CEACAM3 and TLR-dependent inflammatory signalling. Cell Microbiol 2018; [Crossref] [PubMed]
- Yang X, Lin Y, Shi Y, et al. FAP Promotes Immunosuppression by Cancer-Associated Fibroblasts in the Tumor Microenvironment via STAT3-CCL2 Signaling. Cancer Res 2016;76:4124-35. [Crossref] [PubMed]
- Chen L, Qiu X, Wang X, et al. FAP positive fibroblasts induce immune checkpoint blockade resistance in colorectal cancer via promoting immunosuppression. Biochem Biophys Res Commun 2017;487:8-14. [Crossref] [PubMed]
- Sagebiel AF, Steinert F, Lunemann S, et al. Tissue-resident Eomes+ NK cells are the major innate lymphoid cell population in human infant intestine. Nat Commun 2019;10:975. [Crossref] [PubMed]
- Hu X, Li YQ, Li QG, et al. ITGAE Defines CD8+ Tumor-Infiltrating Lymphocytes Predicting a better Prognostic Survival in Colorectal Cancer. EBioMedicine 2018;35:178-88. [Crossref] [PubMed]
- Molodtsov A, Turk MJ. Tissue Resident CD8 Memory T Cell Responses in Cancer and Autoimmunity. Front Immunol 2018;9:2810. [Crossref] [PubMed]
- Han J, Khatwani N, Searles TG, et al. Memory CD8+ T cell responses to cancer. Semin Immunol 2020;49:101435. [Crossref] [PubMed]
- Newman AM, Liu CL, Green MR, et al. Robust enumeration of cell subsets from tissue expression profiles. Nat Methods 2015;12:453-7. [Crossref] [PubMed]
- Galon J, Bruni D. Approaches to treat immune hot, altered and cold tumours with combination immunotherapies. Nat Rev Drug Discov 2019;18:197-218. [Crossref] [PubMed]
- Huang AC, Orlowski RJ, Xu X, et al. A single dose of neoadjuvant PD-1 blockade predicts clinical outcomes in resectable melanoma. Nat Med 2019;25:454-61. [Crossref] [PubMed]
- Mlecnik B, Bindea G, Angell HK, et al. Integrative Analyses of Colorectal Cancer Show Immunoscore Is a Stronger Predictor of Patient Survival Than Microsatellite Instability. Immunity 2016;44:698-711. [Crossref] [PubMed]
- Nirmal AJ, Regan T, Shih BB, et al. Immune Cell Gene Signatures for Profiling the Microenvironment of Solid Tumors. Cancer Immunol Res 2018;6:1388-400. [Crossref] [PubMed]
- van der Stok EP, Spaander MCW, Grünhagen DJ, et al. Surveillance after curative treatment for colorectal cancer. Nat Rev Clin Oncol 2017;14:297-315. [Crossref] [PubMed]
- Dąbrowska AM, Słotwiński R. The immune response to surgery and infection. Cent Eur J Immunol 2014;39:532-7. [Crossref] [PubMed]
- Schadendorf D, Hodi FS, Robert C, et al. Pooled Analysis of Long-Term Survival Data From Phase II and Phase III Trials of Ipilimumab in Unresectable or Metastatic Melanoma. J Clin Oncol 2015;33:1889-94. [Crossref] [PubMed]
(English Language Editor: J. Gray)