Molecular subtyping and IMScore based on immune-related pathways, oncogenic pathways, and DNA damage repair pathways for guiding immunotherapy in hepatocellular carcinoma patients
Highlight box
Key finding
• Classifier of HCC and risk model based on immune maybe useful for accurately predicting prognosis for HCC.
What is known and what is new?
• Immunotherapy has been proven to hope for HCC patient treatment.
• The impact of immune related classifier and risk model for HCC has studied a little.
What is the implication, and what should change now?
• Classifier of HCC and risk model based on immune maybe used for clinical application in HCC to help clinicians develop personalized treatment.
Introduction
Immunotherapy has been revealed as a promising therapeutic strategy for the treatment of advanced cancers (1). Compared with other traditional therapies such as chemotherapy and radiotherapy, immunotherapy targets the tumor cells in a more direct manner, with less side effects. Immune checkpoint blockade therapy is one of the most satisfying methods of cancer treatment, wherein programmed cell death protein 1/programmed cell death ligand 1 (PD-1/PD-L1) and cytotoxic T lymphocyte associated antigen-4 (CTLA4) inhibitors are 2 immune checkpoint inhibitors (ICIs) which have been successfully implemented in metastatic cancer patients (2). In human hepatocellular carcinoma (HCC), elevated expression of PD-1 was observed in CD8 T cells, which inhibited the proliferative ability and effector function with reduced granule and cytokine expression (3). Both CD8 T cells and Kupffer cells have been shown to express high levels of PD-1 and PD-L1 in human HCC tissues, respectively. An increased expression level of PD-L1 is associated with unfavorable prognosis in HCC patients (4,5).
A group of clinical trials on anti-PD-1/PD-L1 or anti-CTLA4 have been implemented in HCC patients. Sorafenib (CheckMate 040) and pembrolizumab (KEYNOTE-224) are 2 ICIs for HCC which have been approved by the United States Food and Drug Administration (FDA) (6,7). In the phase III clinical trials of KEYNOTE-224, median overall survival (OS) was 13.8 months for pembrolizumab and 10.6 months for the control group (8). Although pembrolizumab prolonged OS and progression-free survival (PFS) for advanced HCC patients, the outcomes did not meet the predetermined level of statistical significance. Also, the phase III study of sorafenib did not reach a favorable result in advanced HCC patients (9). In recent years, combination strategies such as ICIs + anti-VEGF/TKI have been shown to perform better than monotherapy in advanced HCC patients (10-12). In a phase Ib study (13) published at the American Society of Clinical Oncology in 2018, advanced HCC patients received both atezolizumab and bevacizumab, and the results showed that the combination of the two had good efficacy and safety, with an effective rate of up to 62%. A recently published phase III study (14) confirmed that the OS and PFS of atezolizumab combined with bevacizumab were superior to those of sorafenib group. Nevertheless, the efficiency of immunotherapy varies largely among individuals, and the tumor microenvironment (TME) has been demonstrated as a critical factor affecting the response to immunotherapy (15).
Spatial heterogeneity of the immune microenvironment in tumors is one of the important challenges of tumor immunotherapy and has attracted wide attention (16). The interaction between tumor cells and infiltrating immune cells regulates and influences each stage of tumorigenesis (17). Compared with paracancerous tissues, HCC immune microenvironment exhibits a more complex and inhibitory phenotype, which can induce tumor escape and promote disease progression (18,19). Immunosuppressant cells, including TAMs, MDSCs, TANs, CAFs and Tregs, are a key component of the TME that promotes the growth and invasion of HCC. The differentiation, maturation and function of immune cells require the involvement and regulation of cytokines and chemical factors, as well as the interaction of receptors and related ligands (20). These factors create a TME, inhibit the anti-tumor activity of immune cells and promote the development of HCC. In the regulation of the TME, in addition to immune-related pathways, several important pathways have also been suggested to be involved in the TME shaping, such as the Wnt signaling pathway (21), TGF-β signaling pathway (22), PI3K-Akt signaling pathway (23), and cell cycle (24). Tumorigenesis is initiated by the occurrence of abnormal mutagenesis and genomic variations known as DNA damage, and DNA repair plays an essential role in the malignant transformation of cells (25,26). Tumor mutation burden (TMB) and genomic instability have been revealed to be associated with the therapeutic response to immunotherapy (27,28). Bioinformatics contributes to the research of targeted therapies for diseases (29,30). Inspired by the previous study (31), we thought that we might be able to contribute to increasing the accuracy of immunotherapy by constructing novel molecular subtypes and a risk model based on the above TME-related or tumor-related pathways. Therefore, in this study, we constructed 3 novel subtypes (clusters) based on immune pathways, stromal pathways, oncogenic pathways, and DNA damage repair pathways. Moreover, we established an IMScore model based on these pathways. This study demonstrated the favorable performance of the IMScore model in predicting prognosis and response to immunotherapy or chemotherapeutic drugs in HCC patients. We present the following article in accordance with the TRIPOD reporting checklist (available at https://jgo.amegroups.com/article/view/10.21037/jgo-22-1101/rc).
Methods
Acquisition and preprocessing of HCC data
The data and information of HCC samples were accessed from the Genomic Data Commons (GDC) Data Portal (https://portal.gdc.cancer.gov/projects/TCGA-LIHC) and Hepatocellular Carcinoma Database (HCCDB; http://lifeome.net/database/hccdb/download.html) (32) on 23 April 2022, which we called The Cancer Genome Atlas liver hepatocellular carcinoma (TCGA-LIHC; abbreviated as TCGA) and HCCDB18 datasets, respectively. TCGA dataset contained RNA-sequencing (RNA-seq) data, clinical information, single nucleotide variation (SNV) data, copy number variation (CNV) data, and methylation array data. The HCCDB18 dataset contained RNA-seq data and survival information.
For RNA-seq data in TCGA and HCCDB18 datasets, samples without survival time and survival status or expression data were excluded. Ensembl ID was converted to a gene symbol. The median values were selected when one gene had multiple gene symbols. After preprocessing, a total of 365 and 203 samples remained in the TCGA and HCCDB18 datasets, respectively (Table S1). The study was conducted in accordance with the Declaration of Helsinki (as revised in 2013).
Consensus clustering based on pathway scores
We obtained a total of 15 tumor-related pathways from a previous study (33), including 5 immune pathways (Fc-gamma R-mediated phagocytosis, natural killer cell mediated cytotoxicity, B cell receptor signaling, T cell receptor signaling, and antigen processing and presentation), 3 stromal pathways [extracellular matrix (ECM)-receptor interaction, focal adhesion, and tight junction], 4 oncogenic pathways (Wnt signaling, PI3K-Akt signaling, TGF-β signaling, and cell cycle) and 3 DNA damage repair pathways (mismatch repair, homologous recombination, and p53 signaling). Single sample gene set enrichment analysis (ssGSEA) was used to calculate the enrichment score of these pathways through GSVA R package (34). Then ConsensusClusterPlus R package (35) was applied to perform unsupervised consensus clustering based on the ssGSEA score of 15 pathways in TCGA and HCCDB18 datasets. Cumulative distribution function (CDF) was calculated when cluster number k=2 to 10 for determining the optimal cluster number.
Analysis of immune characteristics
The Estimation of STromal and Immune cells in MAlignant Tumor tissues using Expression data (ESTIMATE) algorithm was conducted to evaluate the immune infiltration, stromal infiltration, and tumor purity of HCC samples in different clusters. Gene sets of cytolytic activity and epithelial-mesenchymal transition (EMT) were obtained from Rooney et al. (36). Gene sets of 28 immune cells were accessed from Şenbabaoğlu et al. (37). Major histocompatibility complex (MHC)-related genes were downloaded from Tumor Immune System Interactions Database (TISIDB; http://cis.hku.hk/TISIDB/download.php) (38).
Analysis of driver genes and genomic variations
A total of 172 driver genes were downloaded from the cBioPortal for Cancer Genomics webpage (http://cbioportal.org) (39). Fisher’s exact test was performed to identify the driver genes that had different mutation frequencies in different clusters. A P value <0.05 was considered significantly different. GISTIC2 (40) was used to assess the CNVs, where ratio >0.2 was defined as gain of CNV, ratio <−0.2 was defined as loss of CNV, and others were defined as diploid. Homologous recombination deficiency (HRD) feature was evaluated by 3 aspects including number of telomeric regions with allelic imbalance (NtAI), large-scale transition (LST), and loss of heterozygosity (LOH). The scores of NtAI, LST, LOH, and HRD in TCGA dataset were obtained from a previous study (41).
The sensitivity to immunotherapy and chemotherapeutic drugs
The Tumor Immune Dysfunction and Exclusion (TIDE) tool (42) (http://tide.dfci.harvard.edu/) was used to estimate the sensitivity to ICIs. The TIDE score, T cell dysfunction, and T cell exclusion score were calculated for predicting the response to immunotherapy. A higher TIDE score indicated a higher possibility of immune escape from immunotherapy. The R package pRRophetic (43) was utilized to calculate the estimated IC50 of different clusters to 6 chemotherapeutic drugs including cisplatin, sunitinib, CGP-60474, A-770041, roscovitine, and bexarotene.
Weighted correlation network analysis (WGCNA)
The WGCNA R package (44) was used to identify the co-expression gene network. The co-expression network met the scale-free standard [negative correlation between log(k) and log(p(k)), |R| ≥0.85] by setting the suitable soft threshold. The expression matrix was transferred to topological overlap matrix (TOM), and average-link hierarchical clustering was implemented based on TOM and dynamics cutting tree method. For each gene module, at least 100 genes were contained. Then, eigengenes were calculated for each module, and modules were clustered and combined under conditions of height = 0.25, deepSplit = 2, minModuleSize = 100. WebGestaltR package (45) was used to analyze the Kyoto Encyclopedia of Genes and Genomes (KEGG) pathways in gene modules.
Construction of the IMScore model
The HCCDB18 dataset was used to construct the IMScore model. Firstly, univariate Cox regression analysis was performed to identify the pathways related to prognosis based on the ssGSEA score. Then, Pearson correlation analysis was implemented to determine the top 20 genes significantly correlated with pathway score. Univariate Cox regression was conducted on the screened genes to screen prognostic genes. Subsequently, least absolute shrinkage and selection operator (LASSO) regression in glmnet R package (46) was used to decrease the number of prognostic genes for constructing the optimal model. The IMScore model was defined as: IMScore = Σ(expi*βi), where exp represents gene expression levels, β represents LASSO coefficients, and i represents genes.
Validation of the IMScore model
The IMScore was calculated for each HCC sample in TCGA and HCCDB18 datasets. Survminer R package was employed to determine the optimal cut-off values of IMScore for classifying samples into high- and low-IMScore groups. Kaplan–Meier survival analysis was performed to assess the prognosis of 2 groups. Pan-cancer data containing 32 cancer types was downloaded from TCGA database. The IMvigor210 (47) and GSE91061 (48) datasets include immunotherapy data of cancer patients. Median cut-off values of IMScore and TIDE score were selected to classify the patients treated with immunotherapy into 2 risk groups, respectively. Receiver operation characteristic (ROC) curve analysis was used to evaluate the efficiency of IMScore and TIDE score in predicting the prognosis and response to immunotherapy.
Statistical analysis
For survival analysis in different groups, log-rank test was conducted to compare their differences. For comparison of the scores of molecular features, genomic features, and the expression of genes and methylation levels in 3 clusters, Kruskal-Wallis test was performed. Fisher’s exact test was conducted to screen the genes with significantly different mutation frequencies in different clusters. All statistical analysis was performed in R software (v4.0; The R Foundation for Statistical Computing, Vienna, Austria). The bioinformatics analysis in this study was supported by the Sangerbox platform (49).
Results
Molecular subtyping for HCC based on pathway score
We obtained 4 types of pathways (a total of 15 pathways) including immune pathways, stromal pathways, oncogenic pathways, and DNA damage repair pathways from the previous research (33), and calculated the ssGSEA score of each pathway for HCC samples in TCGA and HCCDB18 datasets. Then, based on the ssGSEA of these pathways, molecular subtyping was performed for these samples in 2 datasets respectively by using consensus clustering. According to the CDF curve and consensus matrix, we determined cluster number k=3 as the optimal for classifying HCC samples into 3 clusters (molecular subtypes) (Figure S1). The ssGSEA score of each pathway was visualized and grouped by 3 clusters (Figure 1A). The 3 clusters showed evidently different enrichment patterns, which we subsequently named as immune-deprived (Immune-D), immune-enriched (Immune-E), and stromal-enriched (Stromal-E) based on their features. Immune-D showed derived immune infiltration and activated DNA damage repair pathways. Immune-E showed significantly activated immune pathways. Stromal-E showed a high enrichment score in stromal pathways but relatively low enrichment of immune pathways. Principle component analysis (PCA) displayed that the 3 clusters were relatively separated in both datasets, suggesting that the subtyping based on these pathways was effective and reasonable (Figure S2A,S2B).
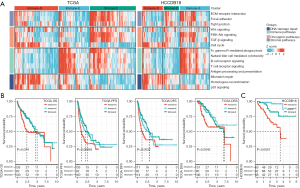
Subsequently, we compared the prognosis of 3 clusters in TCGA and HCCDB18 datasets. Immune-D had the worst prognosis compared with the other 2 clusters (Figure 1B,1C). The prognosis of Immune-E and Stromal-E was not significantly different in TCGA dataset but Immune-E had evidently longer survival than Stromal-E in HCCDB18 dataset. In addition, we compared the distribution of different clinical features in 3 clusters. There were significant differences on age, tumor (T) stage, metastasis (M) stage, and stage I to IV in the 3 clusters (Figure S2C).
Immune characteristics of 3 clusters
The 3 clusters showed different enrichment of immune-related pathways. We then analyzed their immune characteristics from different aspects including immune infiltration, cytolytic activity, EMT activity, immune checkpoints, and MHC expression. The ESTIMATE analysis supported the clustering results that stromal score, immune score, and ESTIMATE score were relatively higher in Immune-E but lower in Immune-D (Figure 2A-2C). There was also a significant difference in tumor purity in different clusters, where Immune-E had the lowest tumor purity score (Figure 2D). EMT is an important tumorigenic process in tumor development, which has a close crosstalk with the tumor immune microenvironment (TIME). We analyzed the enrichment of EMT pathways and observed that the EMT score was differentially distributed in different clusters. The cytolytic activity was also different among the 3 clusters, largely resulting from the distinct infiltration of immune cells (Figure 2E-2G). Immune-E had higher enrichment of most immune cells than the other 2 clusters, such as activated CD4 T cells and activated CD8 T cells (P<0.0001, Figure 2G).
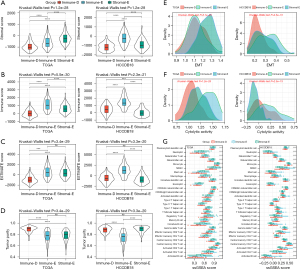
The expression of immune checkpoints was associated with the immune response to immunotherapy (50). We assessed 4 important immune checkpoints including PDCD1 (PD1), CTLA4, LAG3, and CD274. These 4 immune checkpoints were differentially expressed in the 3 clusters, and they were more highly expressed in Immune-E compared with Immune-D and Stromal-E (Figure 3A-3D). MHC is necessary for antigen presentation, which is associated with the tumor-specific immune response (51,52). We evaluated the expression of MHC-related genes in the 3 clusters and found that Immune-E had the highest expression of these genes (Figure 3E). The above results indicated that the 3 clusters had differential immune infiltration and different immune response to tumors.
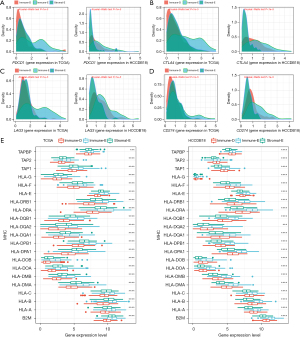
The alterations of tumor driver gene in the 3 clusters
The alteration of tumor driver genes is an important step in triggering tumorigenesis. We obtained a total of 172 tumor driver genes from a previous study (39), and evaluated the alteration features of these driver genes in the 3 clusters. We identified 4 genes as differentially mutated among the 3 clusters, including TP53, CIC, PTEN, and CDH1 (Figure 4A). TP53 was the top mutated gene in all 3 clusters with a high mutation frequency in Immune-D and Immune-E clusters (36% and 35% respectively). Immune-D and Immune-E also had higher TMB than Stromal-E (P<0.001, Figure 4B). To understand whether the mutation of driver genes was associated with HCC prognosis, we divided HCC samples into 2 groups (wild-type and mutant). For each driver gene, we performed the Kaplan–Meier survival analysis, and discovered 10 driver genes (TP53, CR1, B2M, ZBTB20, RPL10L, EPHA6, ZWINT, MAP2K1, TAS2R10, and PNMT) with possible effects on the prognosis (P<0.05, Figure 4C).
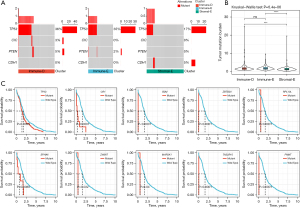
Furthermore, we parsed the CNVs of the driver genes in 3 clusters by using GISTIC2. As a result, we found that 159 of 172 driver genes had differential CNVs among the 3 clusters. The CNVs of partial genes were shown (Figure 5A). Immune-D had more CNVs than the other 2 clusters, and particularly the proportion of TP53 CNVs was significantly higher in Immune-D (74%) compared with Immune-E and Stromal-E (48% and 47%, respectively). DNA repair and DNA damage link to genome instability that increases the susceptibility of cancer. We accessed the HRD-related scores of TCGA dataset from a previous study (41). NtAL, LST and HRD-LOH were highest in Immune-D and lowest in Stromal-E (Figure 5B-5D). The HRD-related scores were the highest in Immune-E and the lowest in Stromal-E among the 3 clusters (Figure 5E). In addition, we compared the expression of gain and loss of CNVs. The gene expression of CNV loss was lower and the expression of CNV gain was higher than in the diploid group, which was evidently observed in TP53 (Figure 5F).
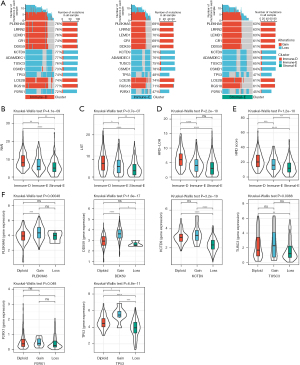
Methylation levels of the genes related to EMT and DNA repair
A total of 7 EMT-related genes (ZEB1, ZEB2, TWIST1, VIM, CDH2, CDH1, and CLDN1) and 2 DNA repair-related genes (MLH1 and MSH3) were included for the methylation analysis. The beta value of each gene in the 3 clusters was analyzed. The results revealed that Stromal-E had the highest mean beta values of 5 EMT-related genes including ZEB1, ZEB2, CDH2, CDH1, and CLDN1, as well as 2 DNA repair-related genes (Figure 6A). The methylation levels were negatively correlated with the expression of VIM, CDH2, CDH1, CLDN1, and MSH3, but a positive correlation was observed in ZEB1 and ZEB2 (Figure 6B). Moreover, we evaluated the distribution of beta values of specific cg probe sites in the 3 clusters. The beta values of most of cg cites, especially in CDH1, CDH2, CLDN1, and ZEB2 were differential in the 3 clusters (Figure 6C and Figure S3). The beta values of most of cg sites were negatively correlated with the expression CDH1, CDH2, VIM, and CLDN1, whereas ZEB2 expression was positively correlated with most of the cg sites in ZEB2 (Figure 6D and Figure S4).
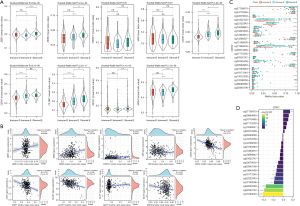
The sensitivity of the 3clusters to immunotherapy and chemotherapeutic drugs
Next, we applied the TIDE algorithm to estimate the potential response of the 3 clusters to ICIs. Immune-E had the lowest score of T cell exclusion, which was consistent with its clustering feature, however, T cell dysfunction was estimated to be the most severe in Immune-E (Figure 7A,7B). The results suggested that the cytolytic activity of T cells was inhibited possibly resulting from the overexpression of immune checkpoints (Figure 3A-3D). The TIDE score was relatively low in Immune-E, indicating that Immune-E was predicted to be more sensitive to ICIs. Of the response to chemotherapeutic drugs, Immune-E had lower estimated IC50 values of all 6 drugs including cisplatin, sunitinib, CGP-60474, A-770041, roscovitine, and bexarotene compared with the other 2 clusters (Figure 7C,7D), implying that Immune-E was more sensitive to these chemotherapeutic drugs.
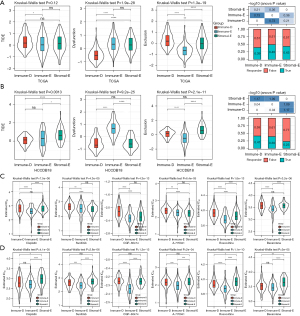
WGCNA for identifying gene modules related to the 3 clusters
To screen the key genes related to Immune-E, Immune-D and Stromal-E, we performed WGCNA to cluster genes in TCGA dataset (Figure S5A). The power of soft threshold (β) was set as 12 to meet a scale-free network (Figure S5B,S5C). Then, genes were further clustered based on average-linkage hierarchical clustering method with each clustering containing at least 100 genes. The clusters were combined according to their eigengenes to generate gene modules, and finally 8 gene modules were outputted (Figure S5D,S5E). We then analyzed the correlation of these gene modules with the 3 clusters (Figure 8A). the pink module was positively correlated with Stromal-E (R=0.48), and negatively correlated with Immune-D (R=−0.26) and Immune-E (R=−0.25). The yellow module was negatively correlated with Immune-D (R=−0.24) but positively correlated with Immune-E (R=−0.27). Stromal-E was also negatively correlated with the brown module (R=−0.35). Furthermore, we assessed the functional pathways of the pink module and yellow module. The results showed that stromal-related pathways such as ECM-receptor interaction and focal adhesion pathways were significantly enriched in the pink module (Figure S6A). Immune-related pathways such as B cell receptor signaling, T cell receptor signaling, natural killer cell mediated cytotoxicity, and chemokine signaling pathways were enriched in the yellow module (Figure S6B). The enriched pathways were consistent with the above correlation results.
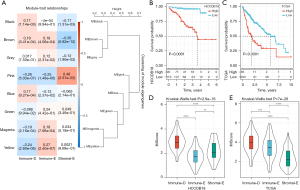
Construction of IMScore for predicting HCC prognosis
In the previous sections, we demonstrated that the 3 clusters had different prognosis, immune microenvironment, genomic characteristics, and response to immunotherapy and chemotherapeutic drugs, and these results supported the important role of the 15 pathways in HCC development. To identify key genes within these pathways that related to the prognosis, we firstly used univariate Cox regression analysis to determine the key pathways in HCCDB18 dataset. We determined 6 to be associated with the prognosis, including antigen processing and presentation, B cell receptor signaling, focal adhesion, mismatch repair, homologous recombination, PI3K-Akt signaling, and cell cycle (Table S2). Then, Pearson correlation analysis was conducted to screen the genes significantly associated the enrichment score of the 6 pathways, and the top 20 genes in each pathway were screened. Subsequently, we identified 70 genes associated with the prognosis through univariate Cox regression. To construct an optimal prognostic model, we used LASSO regression to deduct the number of genes. The model reached the optimal when lambda = 0.0841 (Figure S7). Ultimately, 3 genes were retained to construct a prognostic model: IMScore = 0.3×CCNB1 + 0.213×MCM2 + 0.565×CDC25C.
We calculated the IMScore for each HCC sample in the HCCDB18 dataset, and classified samples into high- and low-IMScore groups using survminer R package. The high-IMScore group had significantly shorter OS than low-IMScore group (P<0.0001, Figure 8B). The same result was observed in TCGA dataset (Figure 8C). In addition, we analyzed the distribution of IMScore in clusters and found that Immune-D had the higher IMScore than the other 2 clusters (Figure 8D,8E), which was consistent with the previous results that Immune-D had the worst prognosis (Figure 8B,8C).
The performance of the IMScore model in pan-cancer and the patients treated with immunotherapy
We accessed the expression data and survival information of 32 cancer types from TCGA database, and validated the performance of IMScore in pan-cancer data by using the same calculation method. The results displayed that high- and low-IMScore groups had significantly different OS in 27 cancer types except for cholangiocarcinoma (CHOL), glioblastoma (GBM), ovarian serous cystadenocarcinoma (OV), testicular germ cell tumors (TGCT), and thyroid carcinoma (THCA) (Figure S8). The IMScore was also effective to classify samples into different risk groups in most of cancer types.
Furthermore, we evaluated the performance of the IMScore model in the patients treated with immunotherapy (IMvigor210 and GSE91061 datasets). The IMScore and TIDE score were calculated for each patient. The IMScore showed a superior performance in dividing the patients into 2 risk groups than the TIDE score in 2 datasets (Figure 9A-9D). The areas under the ROC curve (AUC) of 0.5-, 1-, and 1.5-year for IMScore in predicting the prognosis in IMvigor210 dataset were 0.58, 0.60, and 0.60 respectively, which were higher than the AUCs of TIDE prediction with 0.54, 0.57, and 0.57 of 0.5-, 1-, and 1.5-year, respectively (Figure 9A,9B). In the GSE91061 dataset, the IMScore also had higher AUC of 1-, 2-, and 2.5-year than the TIDE score (Figure 9C,9D). Next, we compared the sensitivity of the IMScore and TIDE score in predicting the response of the patients to immunotherapy. IMScore (AUC =0.68) outperformed TIDE score (AUC =0.58) in the IMvigor210 dataset but both scores had the same AUC in the GSE91061 dataset (Figure 9E,9F). Overall, the IMScore was more advantageous in predicting the prognosis or the sensitivity to immunotherapy than the TIDE score.

Discussion
Multiple clinical trials have shown the potential of immunotherapy in advanced cancers, however, only a fraction of patients can benefit from the immunotherapy. The efficiency of immunotherapy is affected by various aspects and depends on the individual patient. Therefore, it is necessary to distinguish the cancer patients who are suitable to receive immunotherapy. Previous studies have explored a series of signatures or subtypes for predicting the response to immunotherapy for different cancer types such as bladder cancer (53), melanoma (54), pancreatic ductal adenocarcinoma (55), and HCC (56,57). These signatures or subtypes have been based on 1 type or group of genes. It is recognized that the TME contributes to the destiny of tumor development and prognosis. The crosstalk of TME with immune cell infiltration, stromal infiltration, activation or suppression of oncogenic pathways, and DNA damage pathways results in the complicated mechanism of tumor development and the different immune response.
In the present study, we constructed 3 molecular subtypes (Immune-E, Immune-D, and Stromal-E) based on the enrichment score of immune pathways, stromal pathways, oncogenic pathways, and DNA damage repair pathways. We compared the 3 subtypes in terms of prognosis, immune infiltration, genomic characteristics, methylation levels, and response to immunotherapy and chemotherapeutic drugs. The results showed significant differences of the above aspects, which may be responsible for their different responses to immunotherapy.
The 15 pathways were differentially enriched in 3 subtypes, where immune pathways were the most enriched in Immune-E but relatively barren in Immune-D and Stromal-E. Stromal pathways and oncogenic pathways were enriched in Stromal-E, whereas DNA damage repair pathways were evidently enriched in Immune-D. Immune-E had higher immune infiltration and higher enrichment of activated CD4 T cells and activated CD8 T cells compared with the other 2 subtypes, which contributed to higher cytolytic activity and better prognosis. Immune escape of tumor cells is related to loss of antigen presentation by MHC (52), suggesting that low expression of MHC-related genes can lead to the immune escape and promote tumor progression. We found that Immune-E had the highest expression on 21 MHC-related genes than Immune-D and Stromal-E. However, the expression of 4 important immune checkpoints (PD1, PDL1, CTLA4, and LAG3) were also much expressed in Immune-E, where high expression of these immune checkpoints was associated with poor prognosis (58,59). TIDE analysis also supported the above findings that T cell dysfunction was severe in Immune-E, which resulted from the high expression of immune checkpoints that blocking the functional effects of CD8 T cells.
The variations of tumor driver genes can rewrite the fate of cells by activating or suppressing important pathways. P53 signaling is a well-known oncogenic pathway, and TP53 is a tumor suppressive gene involved in the p53 signaling. We detected that TP53 was the most frequently mutated gene in HCC and the mutation frequency was higher in Immune-D and Immune-E (36% and 35%, respectively) than Stromal-E (17%). However, TP53 CNVs varied greatly in Immune-D and Immune-E, with frequencies of 74% and 48%, respectively. In HCC patients, TP53 alteration was associated with poor prognosis (60). In our results, mutant TP53 also had a worse prognosis than the wild-type group. Therefore, we speculated that the frequent alterations of TP53 in Immune-D was responsible for its worse prognosis than Immune-E.
Homologous recombination is an essential process in repairing DNA breaks and protect cells from being tumor-prone. Previous research has revealed that HRD frequently occurs in human cancers (41). Lin et al. identified molecular subtypes based on HRD-related genes and confirmed the association between HRD and HCC prognosis (61). In our study, we evaluated the HRD signatures of 3 subtypes, and revealed that Immune-D had the highest score of NtAI, LST, LOH, and HRD compared with the other subtypes. The results indicated that the severe deficiency of homologous recombination in Immune-D was also an important factor contributing to its poor survival.
In the response of the 3 subtypes to ICIs, TIDE analysis showed that Immune-E had a relative favorable response to immunotherapy in 2 TCGA and HCCDB18 datasets, but there was no significant difference of the responsive rates in the 3 subtypes. In addition, the estimated IC50 prediction uncovered that the performance of 6 chemotherapeutic drugs was also better in Immune-E than the other 2 subtypes. Furthermore, we established an IMScore model containing CCNB1, MCM2, and CDC25C for predicting HCC prognosis and response to immunotherapy. These 3 genes were all reported to be involved in tumor progression, where CCNB1 and MCM2 were suggested as biomarkers in cancer (62,63). The IMScore model was effective and stable to divide HCC patients into 2 risk groups with distinct OS. In addition, the IMScore outperformed the TIDE score in predicting HCC prognosis and response to immunotherapy. Multiple gene-constructed prognostic models are more reflective of patient status than single prognostic genes, gene signatures have the disadvantage of currently appearing to have limited transferability between tumour types.
However, this study had some limitations, such as it is necessary for polymerase chain reaction (PCR) and immunohistochemical verification. Other considerations were not assimilated on our end because the samples lacked essential clinical follow-up information, most notably diagnostic specifics.
Conclusions
In conclusion, this study identified 3 novel molecular subtypes based on immune pathways, stromal pathways, oncogenic pathways, and DNA damage repair pathways. The different TME and genomic features allowed us to identify 3 subtypes with different responses to immunotherapy and chemotherapeutic drugs. Besides, the IMScore model showed potential to provide a guidance in immunotherapy of HCC patients. The above analysis can guide clinicians in the diagnosis and prognostic prediction of HCC patients with different immunophenotypes.
Acknowledgments
Funding: None.
Footnote
Reporting Checklist: The authors have completed the TRIPOD reporting checklist. Available at https://jgo.amegroups.com/article/view/10.21037/jgo-22-1101/rc
Conflicts of Interest: All authors have completed the ICMJE uniform disclosure form (available at https://jgo.amegroups.com/article/view/10.21037/jgo-22-1101/coif). The authors have no conflicts of interest to declare.
Ethical Statement: The authors are accountable for all aspects of the work in ensuring that questions related to the accuracy or integrity of any part of the work are appropriately investigated and resolved. The study was conducted in accordance with the Declaration of Helsinki (as revised in 2013).
Open Access Statement: This is an Open Access article distributed in accordance with the Creative Commons Attribution-NonCommercial-NoDerivs 4.0 International License (CC BY-NC-ND 4.0), which permits the non-commercial replication and distribution of the article with the strict proviso that no changes or edits are made and the original work is properly cited (including links to both the formal publication through the relevant DOI and the license). See: https://creativecommons.org/licenses/by-nc-nd/4.0/.
References
- Esfahani K, Roudaia L, Buhlaiga N, et al. A review of cancer immunotherapy: from the past, to the present, to the future. Curr Oncol 2020;27:S87-97. [Crossref] [PubMed]
- Buchbinder EI, Desai A. CTLA-4 and PD-1 Pathways: Similarities, Differences, and Implications of Their Inhibition. Am J Clin Oncol 2016;39:98-106. [Crossref] [PubMed]
- Wu K, Kryczek I, Chen L, et al. Kupffer cell suppression of CD8+ T cells in human hepatocellular carcinoma is mediated by B7-H1/programmed death-1 interactions. Cancer Res 2009;69:8067-75. [Crossref] [PubMed]
- Dai X, Xue J, Hu J, et al. Positive Expression of Programmed Death Ligand 1 in Peritumoral Liver Tissue is Associated with Poor Survival after Curative Resection of Hepatocellular Carcinoma. Transl Oncol 2017;10:511-7. [Crossref] [PubMed]
- Semaan A, Dietrich D, Bergheim D, et al. CXCL12 expression and PD-L1 expression serve as prognostic biomarkers in HCC and are induced by hypoxia. Virchows Arch 2017;470:185-96. [Crossref] [PubMed]
- El-Khoueiry AB, Sangro B, Yau T, et al. Nivolumab in patients with advanced hepatocellular carcinoma (CheckMate 040): an open-label, non-comparative, phase 1/2 dose escalation and expansion trial. Lancet 2017;389:2492-502. [Crossref] [PubMed]
- Zhu AX, Finn RS, Edeline J, et al. Pembrolizumab in patients with advanced hepatocellular carcinoma previously treated with sorafenib (KEYNOTE-224): a non-randomised, open-label phase 2 trial. Lancet Oncol 2018;19:940-52. [Crossref] [PubMed]
- Finn RS, Ryoo BY, Merle P, et al. Pembrolizumab As Second-Line Therapy in Patients With Advanced Hepatocellular Carcinoma in KEYNOTE-240: A Randomized, Double-Blind, Phase III Trial. J Clin Oncol 2020;38:193-202. [Crossref] [PubMed]
- Yau T, Park JW, Finn RS, et al. CheckMate 459: A randomized, multi-center phase III study of nivolumab (NIVO) vs sorafenib (SOR) as first-line (1L) treatment in patients (pts) with advanced hepatocellular carcinoma (aHCC). Ann Oncol 2019;30:v874-5. [Crossref]
- Finn RS, Ikeda M, Zhu AX, et al. Phase Ib Study of Lenvatinib Plus Pembrolizumab in Patients With Unresectable Hepatocellular Carcinoma. J Clin Oncol 2020;38:2960-70. [Crossref] [PubMed]
- Lee MS, Ryoo BY, Hsu CH, et al. Atezolizumab with or without bevacizumab in unresectable hepatocellular carcinoma (GO30140): an open-label, multicentre, phase 1b study. Lancet Oncol 2020;21:808-20. [Crossref] [PubMed]
- Kudo M. Combination immunotherapy with anti-VEGF/TKI for hepatocellular carcinoma: present and future perspective. Hepatobiliary Surg Nutr 2021;10:241-5. [Crossref] [PubMed]
- Stein S, Pishvaian MJ, Lee MS, et al. Safety and clinical activity of 1L atezolizumab + bevacizumab in a phase Ib study in hepatocellular carcinoma (HCC). J Clin Oncol 2018;36:4074. [Crossref]
- Finn RS, Qin S, Ikeda M, et al. Atezolizumab plus Bevacizumab in Unresectable Hepatocellular Carcinoma. N Engl J Med 2020;382:1894-905. [Crossref] [PubMed]
- Binnewies M, Roberts EW, Kersten K, et al. Understanding the tumor immune microenvironment (TIME) for effective therapy. Nat Med 2018;24:541-50. [Crossref] [PubMed]
- Kurebayashi Y, Ojima H, Tsujikawa H, et al. Landscape of immune microenvironment in hepatocellular carcinoma and its additional impact on histological and molecular classification. Hepatology 2018;68:1025-41. [Crossref] [PubMed]
- Tlsty TD, Coussens LM. Tumor stroma and regulation of cancer development. Annu Rev Pathol 2006;1:119-50. [Crossref] [PubMed]
- Chew V, Lai L, Pan L, et al. Delineation of an immunosuppressive gradient in hepatocellular carcinoma using high-dimensional proteomic and transcriptomic analyses. Proc Natl Acad Sci U S A 2017;114:E5900-9. [Crossref] [PubMed]
- Li S, Yang F, Ren X. Immunotherapy for hepatocellular carcinoma. Drug Discov Ther 2015;9:363-71. [Crossref] [PubMed]
- Zhou Z, Hu Y, Wu Y, et al. The immunosuppressive tumor microenvironment in hepatocellular carcinoma-current situation and outlook. Mol Immunol 2022;151:218-30. [Crossref] [PubMed]
- Patel S, Alam A, Pant R, et al. Wnt Signaling and Its Significance Within the Tumor Microenvironment: Novel Therapeutic Insights. Front Immunol 2019;10:2872. [Crossref] [PubMed]
- Chung JY, Chan MK, Li JS, et al. TGF-β Signaling: From Tissue Fibrosis to Tumor Microenvironment. Int J Mol Sci 2021;22:7575. [Crossref] [PubMed]
- Caforio M, de Billy E, De Angelis B, et al. PI3K/Akt Pathway: The Indestructible Role of a Vintage Target as a Support to the Most Recent Immunotherapeutic Approaches. Cancers (Basel) 2021.
- Li J, Stanger BZ. Cell Cycle Regulation Meets Tumor Immunosuppression. Trends Immunol 2020;41:859-63. [Crossref] [PubMed]
- Torgovnick A, Schumacher B. DNA repair mechanisms in cancer development and therapy. Front Genet 2015;6:157. [Crossref] [PubMed]
- Jeggo PA, Pearl LH, Carr AM. DNA repair, genome stability and cancer: a historical perspective. Nat Rev Cancer 2016;16:35-42. [Crossref] [PubMed]
- Goodman AM, Kato S, Bazhenova L, et al. Tumor Mutational Burden as an Independent Predictor of Response to Immunotherapy in Diverse Cancers. Mol Cancer Ther 2017;16:2598-608. [Crossref] [PubMed]
- Mardis ER. Neoantigens and genome instability: impact on immunogenomic phenotypes and immunotherapy response. Genome Med 2019;11:71. [Crossref] [PubMed]
- Ning W, Li S, Tsering J, et al. Protective Effect of Triphala against Oxidative Stress-Induced Neurotoxicity. Biomed Res Int 2021;2021:6674988. [Crossref] [PubMed]
- Ning W, Jiang X, Sun Z, et al. Identification of the Potential Biomarkers Involved in the Human Oral Mucosal Wound Healing: A Bioinformatic Study. Biomed Res Int 2021; [Crossref]
- Wu Y, Liu Z, Xu X. Molecular subtyping of hepatocellular carcinoma: A step toward precision medicine. Cancer Commun (Lond) 2020;40:681-93. [Crossref] [PubMed]
- Lian Q, Wang S, Zhang G, et al. HCCDB: A Database of Hepatocellular Carcinoma Expression Atlas. Genomics Proteomics Bioinformatics 2018;16:269-75. [Crossref] [PubMed]
- Li L, Wang X. Identification of gastric cancer subtypes based on pathway clustering. NPJ Precis Oncol 2021;5:46. [Crossref] [PubMed]
- Hänzelmann S, Castelo R, Guinney J. GSVA: gene set variation analysis for microarray and RNA-seq data. BMC Bioinformatics 2013;14:7. [Crossref] [PubMed]
- Wilkerson MD, Hayes DN. ConsensusClusterPlus: a class discovery tool with confidence assessments and item tracking. Bioinformatics 2010;26:1572-3. [Crossref] [PubMed]
- Rooney MS, Shukla SA, Wu CJ, et al. Molecular and genetic properties of tumors associated with local immune cytolytic activity. Cell 2015;160:48-61. [Crossref] [PubMed]
- Şenbabaoğlu Y, Gejman RS, Winer AG, et al. Tumor immune microenvironment characterization in clear cell renal cell carcinoma identifies prognostic and immunotherapeutically relevant messenger RNA signatures. Genome Biol 2016;17:231. [Crossref] [PubMed]
- Ru B, Wong CN, Tong Y, et al. TISIDB: an integrated repository portal for tumor-immune system interactions. Bioinformatics 2019;35:4200-2. [Crossref] [PubMed]
- Gao J, Aksoy BA, Dogrusoz U, et al. Integrative analysis of complex cancer genomics and clinical profiles using the cBioPortal. Sci Signal 2013;6:pl1. [Crossref] [PubMed]
- Mermel CH, Schumacher SE, Hill B, et al. GISTIC2.0 facilitates sensitive and confident localization of the targets of focal somatic copy-number alteration in human cancers. Genome Biol 2011;12:R41. [Crossref] [PubMed]
- Knijnenburg TA, Wang L, Zimmermann MT, et al. Genomic and Molecular Landscape of DNA Damage Repair Deficiency across The Cancer Genome Atlas. Cell Rep 2018;23:239-254.e6. [Crossref] [PubMed]
- Jiang P, Gu S, Pan D, et al. Signatures of T cell dysfunction and exclusion predict cancer immunotherapy response. Nat Med 2018;24:1550-8. [Crossref] [PubMed]
- Geeleher P, Cox N, Huang RS. pRRophetic: an R package for prediction of clinical chemotherapeutic response from tumor gene expression levels. PLoS One 2014;9:e107468. [Crossref] [PubMed]
- Langfelder P, Horvath S. WGCNA: an R package for weighted correlation network analysis. BMC Bioinformatics 2008;9:559. [Crossref] [PubMed]
- Liao Y, Wang J, Jaehnig EJ, et al. WebGestalt 2019: gene set analysis toolkit with revamped UIs and APIs. Nucleic Acids Res 2019;47:W199-205. [Crossref] [PubMed]
- Friedman J, Hastie T, Tibshirani R. Regularization Paths for Generalized Linear Models via Coordinate Descent. J Stat Softw 2010;33:1-22. [Crossref] [PubMed]
- Balar AV, Galsky MD, Rosenberg JE, et al. Atezolizumab as first-line treatment in cisplatin-ineligible patients with locally advanced and metastatic urothelial carcinoma: a single-arm, multicentre, phase 2 trial. Lancet 2017;389:67-76. [Crossref] [PubMed]
- Riaz N, Havel JJ, Makarov V, et al. Tumor and Microenvironment Evolution during Immunotherapy with Nivolumab. Cell 2017;171:934-949.e16. [Crossref] [PubMed]
- Shen W, Song Z, Zhong X, et al. Sangerbox: A comprehensive, interaction-friendly clinical bioinformatics analysis platform. iMeta 2022; [Crossref]
- He X, Xu C. Immune checkpoint signaling and cancer immunotherapy. Cell Res 2020;30:660-9. [Crossref] [PubMed]
- Martini M, Testi MG, Pasetto M, et al. IFN-gamma-mediated upmodulation of MHC class I expression activates tumor-specific immune response in a mouse model of prostate cancer. Vaccine 2010;28:3548-57. [Crossref] [PubMed]
- Dhatchinamoorthy K, Colbert JD, Rock KL. Cancer Immune Evasion Through Loss of MHC Class I Antigen Presentation. Front Immunol 2021;12:636568. [Crossref] [PubMed]
- Song BN, Kim SK, Mun JY, et al. Identification of an immunotherapy-responsive molecular subtype of bladder cancer. EBioMedicine 2019;50:238-45. [Crossref] [PubMed]
- Yu Y, Zhang W, Li A, et al. Association of Long Noncoding RNA Biomarkers With Clinical Immune Subtype and Prediction of Immunotherapy Response in Patients With Cancer. JAMA Netw Open 2020;3:e202149. [Crossref] [PubMed]
- Wang Y, Liang Y, Xu H, et al. Single-cell analysis of pancreatic ductal adenocarcinoma identifies a novel fibroblast subtype associated with poor prognosis but better immunotherapy response. Cell Discov 2021;7:36. [Crossref] [PubMed]
- Peng Y, Liu C, Li M, et al. Identification of a prognostic and therapeutic immune signature associated with hepatocellular carcinoma. Cancer Cell Int 2021;21:98. [Crossref] [PubMed]
- Dai Y, Qiang W, Lin K, et al. An immune-related gene signature for predicting survival and immunotherapy efficacy in hepatocellular carcinoma. Cancer Immunol Immunother 2021;70:967-79. [Crossref] [PubMed]
- Zhang Y, Kang S, Shen J, et al. Prognostic significance of programmed cell death 1 (PD-1) or PD-1 ligand 1 (PD-L1) Expression in epithelial-originated cancer: a meta-analysis. Medicine (Baltimore) 2015;94:e515. [Crossref] [PubMed]
- Long L, Zhang X, Chen F, et al. The promising immune checkpoint LAG-3: from tumor microenvironment to cancer immunotherapy. Genes Cancer 2018;9:176-89. [Crossref] [PubMed]
- Liu J, Ma Q, Zhang M, et al. Alterations of TP53 are associated with a poor outcome for patients with hepatocellular carcinoma: evidence from a systematic review and meta-analysis. Eur J Cancer 2012;48:2328-38. [Crossref] [PubMed]
- Lin H, Xie Y, Kong Y, et al. Identification of molecular subtypes and prognostic signature for hepatocellular carcinoma based on genes associated with homologous recombination deficiency. Sci Rep 2021;11:24022. [Crossref] [PubMed]
- Deng Y, Ma H, Hao J, et al. MCM2 and NUSAP1 Are Potential Biomarkers for the Diagnosis and Prognosis of Pancreatic Cancer. Biomed Res Int 2020;2020:8604340. [Crossref] [PubMed]
- Ding K, Li W, Zou Z, et al. CCNB1 is a prognostic biomarker for ER+ breast cancer. Med Hypotheses 2014;83:359-64. [Crossref] [PubMed]
(English Language Editor: J. Jones)