Role of epithelial cell-mesenchymal transition regulators in molecular typing and prognosis of colon cancer
Highlight box
Key findings
• PCOLCE2 and CXCL1 have good potential for clinical translational application in colon cancer.
What is known and what is new?
• Once distant metastasis or local recurrence of colon cancer occurs, the prognosis is still poor, and new markers are needed to predict the prognosis and treatment of colon cancer.
• In this study, PCOLCE2 and CXCL1 can be used as novel molecular markers for colon cancer.
What is the implication, and what should change now?
• In this study, 22 prognostic genes were screened out from 200 EMT-related genes, and then PCOLCE2 and CXCL1 molecules were finally focused on through the combination of the NMF molecular typing model and machine learning screening feature genes, suggesting that PCOLCE2 and CXCL1 may have good application potential. It provides a theoretical basis for the next clinical transformation.
Introduction
Colon cancer is the most common and devastating primary tumor in the digestive system (1,2); it mainly occurs at the junction of the rectum and sigmoid colon, and the age of onset is typically 40–50 years old (3). Currently, it is believed that colon cancer may be significantly related to genetics, the environment, diet, inflammatory bowel disease, gender, race, and other factors (4). Although the rapid development of molecular biology in the past few decades has enriched theories related to carcinogenesis, its pathogenic mechanism is still unclear, and there is a lack of reliable molecular markers for early diagnosis and guidance. At present, surgery remains the main treatment for colon cancer with local non-distant metastasis (5), and once distant metastasis occurs, chemotherapy combined with targeted therapy is often applied (6,7). Despite advances in screening, diagnosis, chemotherapy, and targeted therapy for colon cancer, the prognosis remains poor once distant metastasis or local recurrence occurs. To further improve outcomes for colon cancer patients, researchers or clinicians may need to identify new markers for predicting colon cancer prognosis and treatment (8,9).
In recent years, epithelial-mesenchymal transition (EMT) has been a hot spot in tumor metastasis research. When cells undergo EMT, the appearance of epithelial cells gradually assumes fibroblast morphology, the connection between cells is weakened, and the expression of E-cadherin in the upper cell marker decreases, but the expression of mesenchymal cell markers Vimentin, Fibronectin 1, Slug, alpha smooth muscle actin (α-SMA) increases (10-13). It has been reported that EMT plays an important role in the occurrence, development, and metastasis of human colon cancer. Downregulation of E-cadherin expression indicates poor tumor progression and prognosis in colon cancer patients (14). In turn, increased Vimentin expression also indicates poor tumor progression and prognosis in colon cancer patients (15). In recent years, many researchers have conducted in-depth studies on the role of EMT in tumor metastasis, which has greatly enriched the understanding of EMT (16). However, to date, there has been no instrument capable of monitoring the entire process of EMT in tumor cells. Therefore, the exact role and mechanism of EMT in various stages of metastasis are still unclear. Recent literature also reported that the decreased expression of EMT-related gene MFN2 in colon cancer tissues was negatively correlated with the prognosis of colon cancer patients, and further studies suggest that MFN2 is a promising predictive biomarker and therapeutic target for colon cancer (17). Additional findings also suggest that circulating tumor cells with a mesenchymal phenotype or mixed epithelial/mesenchymal phenotype may be potential biomarkers for monitoring tumor progression in lung or colon cancer, respectively (18).
The progress of bioinformatics technology has enabled researchers to systematically explore tumor pathogenic genes and their regulatory mechanisms (19), facilitating an understanding of the occurrence, development, and metastasis of tumors from different perspectives, which has profoundly impacted the direction of tumor research (20) and played a huge role in its promotion. In order to discover a new mechanism by which EMT regulates tumor progression and to find new targets for diagnosis, targeted therapy, and prognostic guidance, this study combined EMT-related genes with data from The Cancer Genome Atlas (TCGA) database and Gene Expression Omnibus (GEO) database. In this study, 22 factors were screened from 200 EMT-related genes with prognostic value in colon cancer, including 15 tumor-promoting factors and 5 tumor-suppressing factors. On the basis of 22 EMT-related genes, we divided colon cancer into 2 different molecular subtypes by non-negative matrix factorization (NMF) model using 14 differentially expressed genes (DEGs), and the DEGs were enriched in multiple signaling pathways related to tumor metastasis process. Using random forest algorithm (RFA) to further analyze EMT DEGs, our study revealed that PCOLCE2 and CXCL1 genes are characteristic genes for clinical prognosis of colon cancer, which means that this study focused on PCOLCE2 and CXCL1, characteristic genes of colon cancer, from 200 EMT genes, among which PCOLCE2 gene has not been studied in colon cancer, using multiple bioinformatics techniques, suggesting that functional studies targeting PCOLCE2 gene have the potential to unravel new mechanisms of EMT regulation of tumors. We present the following article in accordance with the REMARK reporting checklist (available at https://jgo.amegroups.com/article/view/10.21037/jgo-23-49/rc).
Methods
Data sources
The data of GSE106582 were downloaded from the GEO database. GSE106582 contains 137 samples, including 77 primary colon cancer lesions and 60 paracancerous mucosal tissues.
Colon adenocarcinoma (COAD) samples were downloaded from TCGA database, including messenger RNA (mRNA) expression data and clinical data. Excluding data with incomplete clinical information and gene expression information, a total of 480 patients’ gene expression and corresponding clinical data were finally included. The study was conducted in accordance with the Declaration of Helsinki (as revised in 2013).
DEGs screening and survival analysis
In this study, R packages such as “ggplot2”, “limma”, and “pheatmap” were used to perform differential analysis of RNA-seq data in the database. The conditions for screening DEGs were as follows: |Log2(fold change)| >1.
The prognosis of genes was analyzed by Kaplan-Meier (KM) method using the “survival” package, where a P value of <0.05 was the screening condition.
Protein-protein interaction (PPI) network and signal pathway enrichment
Functional analysis of DEGs Gene Ontology (GO) functional analysis and Kyoto Encyclopedia of Genes and Genomes (KEGG) pathway enrichment analysis were performed using packages such as “org.Hs.eg.db”, “enrichplot”, and “clusterProfiler” in R language (The R Foundation for Statistical Computing, Vienna, Austria).
The differentially expressed protein interactions were predicted using the Search Tool for the Retrieval of INteracting Genes/Proteins (STRING) online database, the prediction results were displayed in a protein binding network diagram, and the effective binding score was set to >0.4.
Molecular typing of colon cancer patients
NMF represents a rapidly developing data analysis method in recent years, which has a wide application prospect in bioinformatics. NMF projects data into a low-dimensional space for analysis by decomposing a high-dimensional nonnegative data matrix into 2 low-dimensional nonnegative matrices and minimizing the distance between them (21), which has good interpretability and numerical results, and this method has been widely used in gene expression profiling data for cancer classification (22,23). In this study, the “Consensus ClusterPlus” analysis package in R language was used to construct the NMF molecular typing model, and the adjusted and unified data set was used for NMF hierarchical clustering. A value of k with good cluster stability was used for molecular typing (24).
RFA
RFA is a supervised classification method based on decision tree set, which estimates the importance of variables to subject classification (25). In this study, random forest classifier was used to rank the importance of target EMT genes, and genes that were relatively important for the establishment of classification models were obtained. The number of random forest decision trees (Ntree) =500. The analysis process was realized by R language programming. According to the result of the random forest classification model, mean decrease accuracy and gt was chosen, with decrease or decrease in gini and gt 2 as a condition for distinguishing important genes. The intersection of selected target genes from the dataset was taken, and the VennDiagram software package was used to make a Venn diagram.
Statistical analysis
The prognostic analysis of genes was performed using the K-M method. Differences in expression between genes were calculated using independent t-tests. Other statistical methods are described in the materials and methods above.
Results
Prognostic analysis of 200 EMT-related genes in colon cancer
A total of 200 EMT-related genes (website: https://cdn.amegroups.cn/static/public/jgo-23-49-01.pdf) were sourced from the Molecular Signatures Database (MSigDB) website and literature reports (26). In this study, the expression levels of 200 EMT-related genes were extracted from TCGA-COAD database. Combined with the survival data of colon cancer patients, we analyzed the effect of 200 EMT-related genes on the survival of colon cancer patients. Our study elucidated that 22 of the 200 EMT-related genes have prognostic value in colon cancer, of which 15 EMT-related genes (ENO2, FSTL3, MYL9, CRLF1, PFN2, MGP, PLOD3, GADD45B, VEGFC, SDC4, PCOLCE2, FBN2, VIM, VEGFA, and TPM2) have a tumor-promoting role in colon cancer (Figure 1A); the other 7 EMT-related genes (TFPI2, FUCA1, WNT5A, CXCL8, TPM4, CXCL1, and MMP3) exert a tumor suppressor effect in colon cancer (Figure 1B). Based on the above, we identified 22 factors from 200 EMT-related genes with prognostic value in colon cancer by bioinformatics technology, which laid a foundation for our subsequent clinical studies.
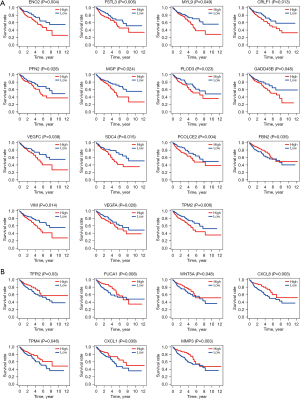
Differential expression analysis of 22 EMT-related genes with a prognostic role in colon cancer tissue samples
Differential expression analysis of 22 EMT-related genes with prognostic value in the previous study was performed using the sequencing data of normal tissues and primary lesions in GEO-GSE106582. The GSE106582 dataset included 137 tissue samples, and our study found that 14 of the 22 EMT-related genes were differentially expressed. Compared with the adjacent normal mucosa tissue, the expression of 10 genes (MMP3, ENO2, FSTL3, SDC4, PLOD3, VEGFA, TFPI2, CXCL1, TPM4, WNT5A) in colon cancer tissue was increased in colon cancer tissue (Figure 2A,2B); at the same time, the expression levels of 4 genes (MGP, MYL9, PUCA1, PCOLCE2) were decreased (Figure 2A,2B). Moreover, PPI network analysis using the STRING website predicted that MMP3 and VEGFA are at the core node, and both may bind to CXCL1 and WNT5A proteins (Figure 2C). In addition, Pearson analysis also found a certain correlation between these 14 genes (Figure 2D), suggesting that these genes may have a role in mutual regulation. Based on the above, our results suggest that these 14 EMT-related genes may have a complex relationship, and may play a role in combination, regulation, and modification in the occurrence, development, and metastasis of colon cancer.

Molecular typing of colon cancer
The 14 DEGs obtained from the above studies were used to study the molecular typing of colon cancer using the data of colon cancer tissue specimens in the GSE106582 dataset. According to the enrichment scores of 14 genes, we used K-means consistent clustering to analyze 77 colon cancer samples. Consistency matrix, and cumulative distribution function showed that stable clustering results can be obtained when k=2 (Figure 3A-3C). Therefore, the cluster was chosen as k=2 in this study, which means that there were 2 different EMT subtypes in 77 colon cancer samples. In addition, principal component analysis (PCA) also suggested that 77 colon cancer samples could be divided into 2 groups (A and B) according to the expression levels of the 14 related EMT genes (Figure 3D). Further studies also evaluated the expression differences of 14 EMT-related genes in 2 different molecular types (Figure 3E). Our results suggested that ENO2, PCOLCE2, FSTL3, MGP, VEGFA, CXCL1, TPM4, and MYL9 gene expressions were different in molecular typing B compared with molecular typing A (Figure 3F). Based on the above, our research data suggest that colon cancer can be well divided into 2 different types (A and B) based on these 14 EMT-related genes, which suggests that our study has a good potential for clinical translation.
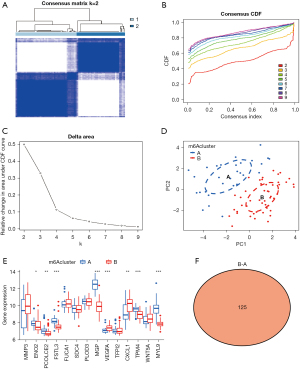
GO and KEGG enrichment analysis
Firstly, we analyzed the DEGs in 2 different molecular subtypes A and B of colon cancer. We did this to compare the differences in gene expression between different molecular subtypes and to determine whether there are factors with clinical translational potential. Compared with molecular typing A, we found 125 DEGs in molecular typing B (LogFC >2 or LogFC <−2). Next, GO function annotation was performed on 125 DEGs, and the relevant data could be divided into 3 categories: biological process (BP), cellular components (CC), and molecular functions (MF). P<0.05 was selected as the condition of significance (Figure 4A-4C). There were 16 KEGG pathways screened at P<0.05, including vascular smooth muscle contraction, focal adhesion, ECM-receptor interaction, malaria, protein digestion and absorption, tight junction, the Wnt signaling pathway, and so on (Figure 4D).
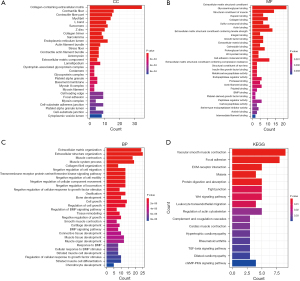
PCOLCE2 and CXCL1 are characteristic genes for clinical prognosis of colon cancer
Through bioinformatics analysis of biological data, we focused on 14 EMT-related genes. In order to further improve the efficiency and economization of clinical transformation and to screen the factors with the most potential for clinical transformation, the 14 EMT-related genes were further analyzed in this study.
Firstly, we used the DEGs generated by the first molecular typing combined with GSE106582 data to perform a second molecular typing analysis. According to the enrichment scores of 125 genes, we used K-means consistent clustering to analyze 77 colon cancer samples. Consistency matrix and cumulative distribution function showed that stable clustering results can be obtained when k=2 (Figure 5A-5C). Here, we again analyzed the expression of EMT-related genes among molecular typing based on 125 DEGs. Our results suggested that ENO2, PCOLCE2, FSTL3, MGP, VEGFA, CXCL1, TPM4, and MYL9 were differentially expressed (Figure 5D), which was consistent with the above results (Figure 3E).
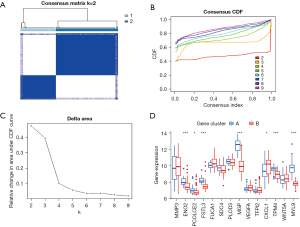
Next, we used RFA to perform screening feature calculation on the 14 EMT-related genes. After calculation, we screened 9 characteristic genes (FUCA1, PLOD3, MMP3, VEGFA, SDC4, PCOLCE2, WNT5A, CXCL1, FSTL3) from 14 EMT-related genes (Figure 6A,6B).
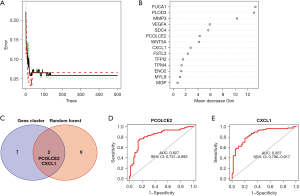
Finally, we intersected the 7 EMT-related DEGs based on the second molecular typing comparison and the feature genes generated by random forest calculation, and our research finally focused on the PCOLCE2 and CXCL1 genes (Figure 6C). Receiver operating characteristic (ROC) analysis found that the area under the curve (AUC) values of PCOLCE2 and CXCL1 were 0.827 (Figure 6D) and 0.857 (Figure 6E), respectively, which clearly indicated that the PCOLCE2 and CXCL1 genes screened in this study well represented the characteristics of the 14 EMT genes.
Based on the above, to facilitate further clinical translation, we combined molecular typing of 14 EMT genes to find DEGs and machine learning screening of characteristic genes. This study finally focused on the PCOLCE2 and CXCL1 genes, which suggested that PCOLCE2 and CXCL1 may have good potential for clinical translation and application.
Discussion
EMT is a developmental process that promotes the transition of epithelial cells to migratory mesenchymal cells (27). Previous articles have reported that EMT is involved in the occurrence, development, and metastasis of tumors, in which it plays an important role (28,29), with a great correlation with cancer metastasis (30). Due to the different driving factors of EMT in different tumor types or in different stages of the occurrence and development of the same tumor, its role in tumor metastasis is not the same (31-34). Secondly, there are few studies on the changes of cell metabolism of tumor cells after EMT and the effects and mechanisms on tumor drug resistance, radioresistance, and tumor microenvironment, which will comprise the focus and challenge of studying EMT-mediated related cancer biology.
In recent years, with the development of high-throughput sequencing technology, the reduction of the cost of gene chips, and the improvement of bioinformatics algorithms, researchers can easily obtain a large amount of biological data and become able to further analyze these data. At present, researchers can use these technologies to predict the core factors involved in tumor progression, and some of these factors may be used as biomarkers. By detecting the expression changes of these factors in the tumor, we can understand the information inside the tumor, which in turn can guide the diagnosis and treatment of tumor (35,36). However, there are still some problems with bioinformatics, such as that large amounts of data are often analyzed incorrectly, resulting in conflicting results. In addition, the target genes or biomarkers screened by bioinformatics technology lack the verification of basic research, which hinders further clinical translation. Therefore, from the perspective of clinical application, the use of bioinformatics technology, the combination of basic experiments, and the combination of clinical patient data will be an important trend in molecular biology research in the future.
This study focused on colon cancer (not limited to colon cancer) to investigate the effect of EMT on colon cancer and to screen colon cancer biomarkers that can be used for clinical transformation. By using bioinformatics technology and the clinical data of TCGA-COAD database, we found that 22 genes were associated with colon cancer prognosis from 200 EMT-related genes. Using GSE106582 data, we compared the DEGs associated with EMT in paracancerous mucosal tissue and colon cancer primary foci. Our study identified 14 DEGs. These data indicate that although EMT plays an important role in the occurrence, development, and metastasis of colon cancer (37,38), the key genes involved in tumor progression are still relatively few (10%). It is difficult for researchers to find and verify these key genes and how to carry out clinical transformation.
The occurrence, development, and metastasis of tumors are not determined by a single factor (39); they involve very complex processes, such as the imbalance of tumor suppressor genes and promoting genes, immune microenvironment, and changes in cell metabolism (40). At present, the diagnosis and staging of tumors depend on pathology, but it is difficult to reflect the biological nature of tumors. New tumor typing methods based on molecular typing can provide global characteristics of tumor genes, enrich molecular pathological information of tumor occurrence and progression, and provide support for clinical diagnosis, staging, individualized treatment, and prognosis prediction (41). In this study, through the construction of NMF molecular typing model (42,43), colon cancer patients were divided into 2 categories based on the expression levels of 14 core EMT-related genes. Although there was no prognostic assessment between the 2 different molecular subtypes of colon cancer, we performed functional enrichment analysis of DEGs between the 2 subtypes. Our results suggest that 125 DEGs are closely associated with signaling pathways related to tumor metastasis. These results indicate that colon cancer can be classified into high- and low-risk groups by typing, which represent 2 different biological functions, and molecular typing of core genes can make up for the deficiency of traditional pathological diagnosis. Since the ENO2, PCOLCE2, FSTL3, MGP, VEGFA, CXCL1, TPM4, and MYL9 genes were significantly different in the comparison of 2 different molecular typing, we suggest that these genes may have more important roles, and we speculate that these genes are involved in colon cancer progression, which may be closely related to the poor prognosis of colon cancer patients.
However, molecular typing involves many genes, ranging from a few to more than a dozen, which is not convenient for direct clinical translation. Based on this, to discover the factors with the most potential for clinical translation, we employed the RFA to screen the characteristic genes and molecular typing of DEGs to further analyze the EMT-related genes. The RFA can handle various types of data, can handle a large number of variables, evaluate the importance of variables, has high classification accuracy, and has a fast process (44-46). Using this method, we found that 9 EMT-related genes were ranked at the top. Further, 2 genes, CXCL1 and PCOLCE2, were obtained by intersecting the EMT-related DEGs with molecular typing. It has been confirmed in a variety of tumor tissues that the expression of CXCL1 is related to tumor progression, invasion, and metastasis. Studies have shown that CXCL1 has a role in promoting tumorigenesis, development, and metastasis in colon cancer (47,48), which contradicts the tumor suppressor effect of CXCL1 in this study, suggesting that CXCL1 may have a complex mechanism, and further use of real-world patient data for research and clinical trials. PCOLCE2 is rarely studied in colon cancer, and it is more common in bioinformatics analysis (49-51). There has been no exact functional study of PCOLCE2 in colon cancer, which needs to be further confirmed by specific experiments. In addition, we speculate that the key genes PCOLCE2 and CXCL1 may have a role in regulating the immune microenvironment of colon cancer, however, we need further data analysis and experimental validation. For drug development, we believe that not only antagonistic drugs should be developed for the “star genes”, but also for individual multi-targeted coverage based on the genotype of the patient’s disease, which requires full consideration of the safety of the drug. Finally, because EMT is one of the important phenotypes of tumor cells, and although the causal relationship between EMT and tumor metastasis is not fully understood, yet the role of EMT in drug resistance has been repeatedly confirmed, this study suggests that treatment targeting EMT has the potential to reverse drug resistance, possibly by a mechanism that alters signaling pathways or affects cell proliferation to reverse tumor drug resistance.
In conclusion, some of the “star EMT genes” selected by this analysis have been confirmed to be related to the occurrence, development, and metastasis of colon cancer, and these indicators will be further studied in clinical practice.
Conclusions
In this study, 22 prognostic genes were screened out from 200 EMT-related genes, and then the PCOLCE2 and CXCL1 molecules were finally focused on through the combination of NMF molecular typing model and machine learning screening feature genes, suggesting that PCOLCE2 and CXCL1 may have good application potential. Our findings provide a theoretical basis for the next clinical transformation.
Acknowledgments
Funding: None.
Footnote
Reporting Checklist: The authors have completed the REMARK reporting checklist. Available at https://jgo.amegroups.com/article/view/10.21037/jgo-23-49/rc
Peer Review File: Available at https://jgo.amegroups.com/article/view/10.21037/jgo-23-49/prf
Conflicts of Interest: All authors have completed the ICMJE uniform disclosure form (available at https://jgo.amegroups.com/article/view/10.21037/jgo-23-49/coif). The authors have no conflicts of interest to declare.
Ethical Statement: The authors are accountable for all aspects of the work in ensuring that questions related to the accuracy or integrity of any part of the work are appropriately investigated and resolved. The study was conducted in accordance with the Declaration of Helsinki (as revised in 2013).
Open Access Statement: This is an Open Access article distributed in accordance with the Creative Commons Attribution-NonCommercial-NoDerivs 4.0 International License (CC BY-NC-ND 4.0), which permits the non-commercial replication and distribution of the article with the strict proviso that no changes or edits are made and the original work is properly cited (including links to both the formal publication through the relevant DOI and the license). See: https://creativecommons.org/licenses/by-nc-nd/4.0/.
References
- Wang Y, Lu Z, Wang N, et al. MicroRNA-1299 is a negative regulator of STAT3 in colon cancer. Oncol Rep 2017;37:3227-34. [Crossref] [PubMed]
- Katsaounou K, Nicolaou E, Vogazianos P, et al. Colon Cancer: From Epidemiology to Prevention. Metabolites 2022;12:499. [Crossref] [PubMed]
- Dou C, Sun L, Jin X, et al. Long non-coding RNA colon cancer-associated transcript 1 functions as a competing endogenous RNA to regulate cyclin-dependent kinase 1 expression by sponging miR-490-3p in hepatocellular carcinoma progression. Tumour Biol 2017;39:1010428317697572. [Crossref] [PubMed]
- Dekker E, Tanis PJ, Vleugels JLA, et al. Colorectal cancer. Lancet 2019;394:1467-80. [Crossref] [PubMed]
- van de Velde CJ, Boelens PG, Borras JM, et al. EURECCA colorectal: multidisciplinary management: European consensus conference colon & rectum. Eur J Cancer 2014;50:1.e1-1.e34. [Crossref] [PubMed]
- Kuipers EJ, Grady WM, Lieberman D, et al. Colorectal cancer. Nat Rev Dis Primers 2015;1:15065. [Crossref] [PubMed]
- Shinji S, Yamada T, Matsuda A, et al. Recent Advances in the Treatment of Colorectal Cancer: A Review. J Nippon Med Sch 2022;89:246-54. [Crossref] [PubMed]
- Zhu J, Kong W, Xie Z. Expression and Prognostic Characteristics of Ferroptosis-Related Genes in Colon Cancer. Int J Mol Sci 2021;22:5652. [Crossref] [PubMed]
- Yuan Y, Chen J, Wang J, et al. Development and Clinical Validation of a Novel 4-Gene Prognostic Signature Predicting Survival in Colorectal Cancer. Front Oncol 2020;10:595. [Crossref] [PubMed]
- Singh AB, Sharma A, Smith JJ, et al. Claudin-1 up-regulates the repressor ZEB-1 to inhibit E-cadherin expression in colon cancer cells. Gastroenterology 2011;141:2140-53. [Crossref] [PubMed]
- Fang X, Cai Y, Liu J, et al. Twist2 contributes to breast cancer progression by promoting an epithelial-mesenchymal transition and cancer stem-like cell self-renewal. Oncogene 2011;30:4707-20. [Crossref] [PubMed]
- Hanahan D, Weinberg RA. Hallmarks of cancer: the next generation. Cell 2011;144:646-74. [Crossref] [PubMed]
- Duan H, Liu Y, Gao Z, et al. Recent advances in drug delivery systems for targeting cancer stem cells. Acta Pharm Sin B 2021;11:55-70. [Crossref] [PubMed]
- He X, Chen Z, Jia M, et al. Downregulated E-cadherin expression indicates worse prognosis in Asian patients with colorectal cancer: evidence from meta-analysis. PLoS One 2013;8:e70858. [Crossref] [PubMed]
- Toiyama Y, Yasuda H, Saigusa S, et al. Increased expression of Slug and Vimentin as novel predictive biomarkers for lymph node metastasis and poor prognosis in colorectal cancer. Carcinogenesis 2013;34:2548-57. [Crossref] [PubMed]
- Tan Z, Sun W, Li Y, et al. Current Progress of EMT: A New Direction of Targeted Therapy for Colorectal Cancer with Invasion and Metastasis. Biomolecules 2022;12:1723. [Crossref] [PubMed]
- Cheng X, Li Y, Liu F. Prognostic impact of mitofusin 2 expression in colon cancer. Transl Cancer Res 2022;11:3610-9. [Crossref] [PubMed]
- Fang J, Wang W, Fang J, et al. Epithelial-mesenchymal transition classification of circulating tumor cells in lung and colon cancer patients: potential role in clinical practice. Transl Cancer Res 2020;9:6639-51. [Crossref] [PubMed]
- Liu M, Yang F, Xu Y. Identification of Potential Drug Therapy for Dermatofibrosarcoma Protuberans with Bioinformatics and Deep Learning Technology. Curr Comput Aided Drug Des 2022;18:393-405. [Crossref] [PubMed]
- Gong HB, Wu XJ, Pu XM, et al. Bioinformatics analysis of key biomarkers and pathways in KSHV infected endothelial cells. Medicine (Baltimore) 2019;98:e16277. [Crossref] [PubMed]
- Lee DD, Seung HS. Learning the parts of objects by non-negative matrix factorization. Nature 1999;401:788-91. [Crossref] [PubMed]
- Bayar B, Bouaynaya N, Shterenberg R. Probabilistic non-negative matrix factorization: theory and application to microarray data analysis. J Bioinform Comput Biol 2014;12:1450001. [Crossref] [PubMed]
- Ching T, Peplowska K, Huang S, et al. Pan-Cancer Analyses Reveal Long Intergenic Non-Coding RNAs Relevant to Tumor Diagnosis, Subtyping and Prognosis. EBioMedicine 2016;7:62-72. [Crossref] [PubMed]
- Lancichinetti A, Fortunato S. Consensus clustering in complex networks. Sci Rep 2012;2:336. [Crossref] [PubMed]
- Kimura R, Nakata M, Funabiki Y, et al. An epigenetic biomarker for adult high-functioning autism spectrum disorder. Sci Rep 2019;9:13662. [Crossref] [PubMed]
- Ouyang W, Jiang Y, Bu S, et al. A Prognostic Risk Score Based on Hypoxia-, Immunity-, and Epithelialto-Mesenchymal Transition-Related Genes for the Prognosis and Immunotherapy Response of Lung Adenocarcinoma. Front Cell Dev Biol 2021;9:758777. [Crossref] [PubMed]
- Pastushenko I, Brisebarre A, Sifrim A, et al. Identification of the tumour transition states occurring during EMT. Nature 2018;556:463-8. [Crossref] [PubMed]
- Yen CH, Young TH, Hsieh MC, et al. Increased Cell Detachment Ratio of Mesenchymal-Type Lung Cancer Cells on pH-Responsive Chitosan through the β3 Integrin. Mar Drugs 2019;17:659. [Crossref] [PubMed]
- Thiery JP, Acloque H, Huang RY, et al. Epithelial-mesenchymal transitions in development and disease. Cell 2009;139:871-90. [Crossref] [PubMed]
- Yu X, Zheng Y, Zhu X, et al. Osteopontin promotes hepatocellular carcinoma progression via the PI3K/AKT/Twist signaling pathway. Oncol Lett 2018;16:5299-308. [Crossref] [PubMed]
- Zheng X, Carstens JL, Kim J, et al. Epithelial-to-mesenchymal transition is dispensable for metastasis but induces chemoresistance in pancreatic cancer. Nature 2015;527:525-30. [Crossref] [PubMed]
- Tran HD, Luitel K, Kim M, et al. Transient SNAIL1 expression is necessary for metastatic competence in breast cancer. Cancer Res 2014;74:6330-40. [Crossref] [PubMed]
- Krebs AM, Mitschke J, Lasierra Losada M, et al. The EMT-activator Zeb1 is a key factor for cell plasticity and promotes metastasis in pancreatic cancer. Nat Cell Biol 2017;19:518-29. [Crossref] [PubMed]
- Gundamaraju R, Lu W, Paul MK, et al. Autophagy and EMT in cancer and metastasis: Who controls whom? Biochim Biophys Acta Mol Basis Dis 2022;1868:166431. [Crossref] [PubMed]
- Wang J, Chu Y, Li J, et al. Development of a prediction model with serum tumor markers to assess tumor metastasis in lung cancer. Cancer Med 2020;9:5436-45. [Crossref] [PubMed]
- Zhao L, Chi W, Cao H, et al. Screening and clinical significance of tumor markers in head and neck squamous cell carcinoma through bioinformatics analysis. Mol Med Rep 2019;19:143-54. [PubMed]
- Zhang J, Miller Z, Musich PR, et al. DSTYK Promotes Metastasis and Chemoresistance via EMT in Colorectal Cancer. Front Pharmacol 2020;11:1250. [Crossref] [PubMed]
- Zhang D, Bi J, Liang Q, et al. VCAM1 Promotes Tumor Cell Invasion and Metastasis by Inducing EMT and Transendothelial Migration in Colorectal Cancer. Front Oncol 2020;10:1066. [Crossref] [PubMed]
- Weiss F, Lauffenburger D, Friedl P. Towards targeting of shared mechanisms of cancer metastasis and therapy resistance. Nat Rev Cancer 2022;22:157-73. [Crossref] [PubMed]
- Tian L, Guo N, Zhang N, et al. Association of ZEB1 and FOXO3a protein with invasion/metastasis of non-small cell lung cancer. Int J Clin Exp Pathol 2017;10:11308-16. [PubMed]
- Liotta L, Petricoin E. Molecular profiling of human cancer. Nat Rev Genet 2000;1:48-56. [Crossref] [PubMed]
- Mirzal A. Nonparametric Tikhonov Regularized NMF and Its Application in Cancer Clustering. IEEE/ACM Trans Comput Biol Bioinform 2014;11:1208-17.
- Gao Y, Church G. Improving molecular cancer class discovery through sparse non-negative matrix factorization. Bioinformatics 2005;21:3970-5. [Crossref] [PubMed]
- Crist AM, Hinkle KM, Wang X, et al. Transcriptomic analysis to identify genes associated with selective hippocampal vulnerability in Alzheimer's disease. Nat Commun 2021;12:2311. [Crossref] [PubMed]
- Alhusain L, Hafez AM. Cluster ensemble based on Random Forests for genetic data. BioData Min 2017;10:37. [Crossref] [PubMed]
- Liu Y, Wang P, Li Y, et al. Air quality prediction models based on meteorological factors and real-time data of industrial waste gas. Sci Rep 2022;12:9253. [Crossref] [PubMed]
- Zhuo C, Ruan Q, Zhao X, et al. CXCL1 promotes colon cancer progression through activation of NF-κB/P300 signaling pathway. Biol Direct 2022;17:34. [Crossref] [PubMed]
- Jia SN, Han YB, Yang R, et al. Chemokines in colon cancer progression. Semin Cancer Biol 2022;86:400-7. [Crossref] [PubMed]
- Chen L, Lu D, Sun K, et al. Identification of biomarkers associated with diagnosis and prognosis of colorectal cancer patients based on integrated bioinformatics analysis. Gene 2019;692:119-25. [Crossref] [PubMed]
- Yao H, Li C, Tan X. An age stratified analysis of the biomarkers in patients with colorectal cancer. Sci Rep 2021;11:22464. [Crossref] [PubMed]
- Thutkawkorapin J, Picelli S, Kontham V, et al. Exome sequencing in one family with gastric- and rectal cancer. BMC Genet 2016;17:41. [Crossref] [PubMed]
(English Language Editor: J. Jones)