Network pharmacology prediction and molecular docking-based strategy to discover the potential pharmacological mechanism of action of Wang Bu Liu Xing (Semen vaccariae) for colorectal cancer
Highlight box
Key findings
• This study is the first to investigate the pharmacological effects of SV and its potential therapeutic effects on CRC.
What is known and what is new?
• CRC is one of the most malignant tumors worldwide. SV is a TCM with anti-angiogenic and anti-tumor effects;
• This study preliminarily investigated the pharmacological effects of SV, which appear to be mediated by multiple compounds, targets, and pathways, and its potential therapeutic effect on CRC.
What is the implication, and what should change now?
• Provide a theoretical basis for future use of SV in the treatment of CRC.
Introduction
Colorectal cancer (CRC) is the third most prevalent kind of cancer worldwide and the fourth most common cause of cancer-related death (1). In developing countries, CRC incidence and mortality rates have risen considerably in recent years (2). Currently, the mainstays of treatment for CRC include surgery, radiation, and chemotherapy with traditional Chinese medicine (TCM). TCM is largely regarded as a vital complementary therapy that has positive therapeutic effects for cancer patients (3). Chinese herbal medicine has been utilized as an adjunct therapy for cancer in the United States of America (4). Traditional herbal medicine as an adjuvant therapy, when used in conjunction with chemotherapy or radiation therapy, has been demonstrated to enhance therapeutic outcomes and quality of life, lessen side effects, and extend survival (5-9).
Carcinogenesis is a multi-step process that necessitates the accumulation of numerous genetic and epigenetic abnormalities in order to propel the progressive malignant transformation of healthy human cells. Angiogenesis and stem cells’ capacity for endless replication are 2 well-documented characteristics of carcinogenesis. The development of therapeutic treatments for CRC has focused on these characteristics throughout the past 10 years (10).
Semen vaccariae (SV) seeds of Vaccaria segetalis (Neck.) Garcke ex Asch. Medicinal properties: bitter cold and enters into the liver and stomach meridians, with the effect of promoting blood circulation, removing blood stasis, reducing breast swelling, promoting diuresis and passing urine. In recent years, numerous studies have shown that SV has anti-angiogenic and anti-tumor effects (11,12). Angiogenesis inhibitors can effectively inhibit tumor growth and metastasis by selectively inhibiting tumor neovascularization, which has the advantages of broad anti-tumor spectrum and less drug resistance. There are four main factors in TCM syndrome of CRC: deficiency, dampness, stasis and toxin (13). At present, in the clinical treatment of various malignant tumors, drugs to promote blood circulation and remove blood stasis are often used in the prescription of syndrome differentiation, and a lot of treatment experience has been obtained (14,15). There is therefore a need to investigate the role of SV in CRC, and the potential mechanisms.
A technique that shows promise is network pharmacology, which aims to find new medications as well as the scientific underpinnings and therapeutic mechanisms of TCM formulae (16,17). With the quick advancements in bioinformatics and pharmacology, network pharmacology now thoroughly examines the links between medications, targets, and diseases and visualizes the network of drug-targets-disease. This method, which is congruent with TCM theory and stresses the synergy of Chinese medicine, clearly observes the effects of medications on disease (18,19). Additionally, using network pharmacology, a novel significant bioactive component of the TCM recipe might be discovered.
Therefore, our research attempted to predict the SV target and signaling pathways against CRC from a network pharmacology aspect and to further analyze the anti-CRC potential and effector mechanism of SV. Additionally, we used molecular docking technology for verification in order to establish a theoretical framework for future research and practical therapeutic uses of SV against CRC. We present the following article in accordance with the MDAR reporting checklist (available at https://jgo.amegroups.com/article/view/10.21037/jgo-23-83/rc).
Methods
Active ingredient and target gene screening
With the help of Symptom Mapping (SymMap; http://www.symmap.org/) (20), using “Semen vaccariae” as the keyword, we searched the overview of the SymMap network for SMHB00410. From the SymMap database, the ingredients of SV were obtained, and the associated targets of the ingredients in SV were also retrieved. The Traditional Chinese Medicine Systems Pharmacology (TCMSP) database (https://old.tcmsp-e.com/) provided oral bioavailability (OB) scores and Chemical Abstracts Service (CAS) id for each ingredient. We were able to eventually obtain the gene symbol for the ingredients through retrieval and transformation. Ingredients and target genes were used as nodes, and the network relationship between compounds and target genes obtained by SymMap, and the Cytoscape v3.7.2 program (https://cytoscape.org/) was used to create an ingredient-target network (21,22).
Acquisition of CRC-related targets
Disease enrichment analysis was conducted using Harmonizome (https://maayanlab.cloud/Harmonizome/), the target genes imported the heatmap with input genes options, and dataset GAD Gene-Disease Associations, the heatmap of the disease enrichment analysis was obtained (23). Analysis of associations between genes and different cancers was performed using the Database for Annotation Visualization and Integrated Discovery (DAVID; https://david.ncifcrf.gov/). The CRC datasets GSE25070, GSE37182, GSE44076, GSE113513, and GSE10950 were searched and downloaded from the Gene Expression Omnibus (GEO) using the keyword “colorectal cancer”, and gene annotation was performed in Perl (https://www.perl.org/) and R (R Foundation for Statistical Computing, Vienna, Austria) to obtain CRC-related differential genes. The differentially expressed genes (DEGs) were screened by R software “limma” package for each of the 5 datasets (24,25). |log fold change (FC)| >1 and P<0.05 were selected as cutoff values (26). The study was conducted in accordance with the Declaration of Helsinki (as revised in 2013).
Network construction and enrichment analysis
A Venn diagram was plotted between the action targets of SV and the related gene targets of CRC in 5 datasets to obtain the potential action targets of SV against CRC (27,28). They were then imported into the Search Tool for the Retrieval of Interacting Genes/proteins (STRING; https://cn.string-db.org/) database to construct the protein-protein interaction (PPI) network of SV for CRC treatment (29), we set STRING parameter, medium confidence (0.4), homo sapiens, network nodes represent genes/proteins, edges represent protein-protein associations. Gene Ontology (GO) enrichment analysis and Kyoto Encyclopedia of Genes and Genomes (KEGG) pathway analysis were carried out using DAVID (https://david.ncifcrf.gov/summary.jsp), and the result visualization were performed using R software (v4.1.0, packages “clusterProfiler”), P<0.05 were selected (30). The biological process (BP), cellular component (CC), and molecular function (MF) of the targets were primarily examined using GO enrichment. KEGG pathway enrichment was used to examine the targets’ critical biological pathways (30).
Molecular docking
Using GO and KEGG pathway enrichment analysis, we identified the key genes targets by SV ingredients. These targets were confirmed by molecular docking with SV ingredients. The structural MOL2 formula of the ingredients was retrieved (PDB format) from the Research Collaboratory for Structural Bioinformatics (RCSB) database (https://www.rcsb.org) (31). The RCSB database was used to derive the crystal structures of the core genes, which were then edited using PyMOL software (https://pymol.org/2/), including solvent and organic removal. AutoDockTools software was used to incorporate hydrogen atoms prior to molecular docking. The active ingredients served as ligands and the core genes as receptors. Molecular docking was carried out using AutoDock Vina (32). The software automatically used default parameters during standard docking, the best-fit pose of docked molecules, the binding energy values, potential conformations, bond distances and types of interactions were predicted. PyMOL software was ultimately used to display the outcome. The final docking result was chosen to be the conformation with the highest affinity and the lowest binding energy.
Statistical analyses
Statistical analyses were performed using R software (v4.1.0, packages “limma”, and “clusterProfiler”). P<0.05 was considered statistically significant.
Results
Screening for SV ingredients and target genes
Figure 1A depicts our study’s procedure. SV and GSE25070, GSE37182, GSE44076, GSE113513, an GSE10950 from GEO datasets were analyzed. In this study, SymMap database was used to identify the SV; 16 ingredients of SV were finally obtained after screening (Table 1). The SymMap database predicted all of the ingredients’ targets. Eventually, 276 SV prospective targets were found after eliminating the repetitive ones acquired by the different ingredient. Using the Cytoscape v3.7.2 program, the ingredient-target interaction network was created (Figure 1B). The ingredients of SV were represented by the green nodes, and each edge between ingredients and target genes described their interaction. We then performed disease-associated enrichment analysis of these genes and displayed them in a heatmap from Harmonizome (Figure 1C). The results show that these genes are strongly associated with cancer. We then further analyzed their association with different cancers and found that the association with CRC was closer (Figure 1D). Therefore, we screened for CRC-related targets by performing a differential analysis of tumor and normal tissue in 5 CRC datasets in the GEO database. By searching for the point where the disease targets and the aforementioned medication targets converge, 20 overlapping genes were discovered (Figure 2A).
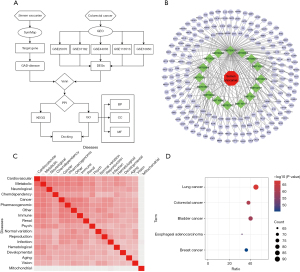
Table 1
SymMap id | Molecule name | Molecule formula | Molecule weight | OB score | PubChem id | TCMID id | TCMSP id | CAS id |
---|---|---|---|---|---|---|---|---|
SMIT00010 | Palmitic acid | C16H32O2 | 256.48 | 19.2966 | 985 | 23175 | MOL000069 | 67701-02-4 |
SMIT00013 | Quercetin | C15H10O7 | 302.25 | 46.4333 | 5280343 | 18302 | MOL000098 | 117-39-5 |
SMIT00044 | Linolenic acid | C18H30O2 | 278.48 | 45.0091 | 5282822 | 23046 | MOL000432 | 463-40-1 |
SMIT00065 | Lignoceric acid | C24H48O2 | 368.72 | 14.9036 | 11197 | 23239 | MOL000663 | 557-59-5 |
SMIT00066 | Oleic acid | C18H34O2 | 282.52 | 33.1284 | 445639 | 23306 | MOL000675 | 112-80-1 |
SMIT00079 | Stearic acid | C18H36O2 | 284.54 | 17.8254 | 5281 | 23171 | MOL000860 | 1957-11-4 |
SMIT00495 | Isovitexin | C21H20O10 | 432.41 | 31.2946 | 162350 | 38232 | MOL002322 | 61838-34-4 |
SMIT01507 | Menthol | C10H20O | 156.26 | 59.33 | 1254 | 13767 | MOL007330 | 1490-04-6 |
SMIT02702 | Arachic acid | C20H40O2 | 312.6 | 16.6564 | 10467 | 32880 | MOL000012 | 506-30-9 |
SMIT02797 | Eic | C18H32O2 | 280.5 | 41.9044 | 5280450 | – | MOL000131 | 2197-37-7 |
SMIT09297 | Swerchirin | C15H12O6 | 288.27 | 4.83817 | 5281660 | 20486 | MOL007956 | 521-65-3 |
SMIT10115 | 9H-xanthene-2-carboxylic acid | C16H12O8 | 226.24 | 42.263 | 12439784 | – | MOL008904 | 125850-40-0 |
SMIT10117 | (3S,6S,9S,12S)-6-(1H-indol-3-ylmethyl)-12-isopropyl-3,9-dimethyl-1,4,7,10,13-pentazacyclopentadecane-2,5,8,11,14-pentone | – | 484.62 | 33.269 | 10345235 | – | MOL008908 | – |
SMIT10120 | Isoschisandrin | C24H32O7 | 432.56 | 7.69729 | 10455507 | 31379 | MOL008912 | 114422-18-3 |
SMIT10121 | Meldenin | C28H38O5 | 440.63 | 15.9603 | 101289833 | 13654 | MOL008913 | – |
SMIT16886 | (E)-2-Nonenal | C9H16O | 140.25 | 19.18 | 5354833 | 33225 | MOL000716 | – |
OB, oral bioavailability; TCMID, Traditional Chinese Medicine integrative database; TCMSP, Traditional Chinese Medicine Systems Pharmacology; CAS, Chemical Abstracts Service.
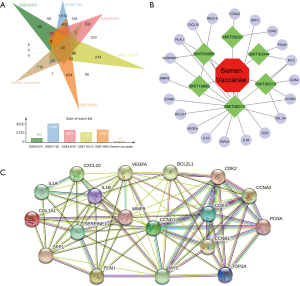
Component-target network construction and PPI analysis
To create a composite target network, the ingredients of SV and 20 probable action targets were loaded into the Cytoscape v3.7.2 program (Figure 2B). The results showed that these 20 potential targets were associated with 6 components including palmitic acid, quercetin, linolenic acid, oleic acid, swerchirin, and (E)-2-Nonenal. The 20 potential targets were uploaded to the STRING database to obtain potentially acting target interactions (Figure 2C). Proteins were represented by network nodes, whereas PPIs were represented by network edges. The strength of the predicted interaction was determined by the thickness of the lines connecting the nodes; the thicker the line, the greater the association between proteins that interact. It was found that these 20 targets were all interacting with each other.
Functional enrichment analysis
We ran GO and KEGG functional enrichment analyses on the 20 genes using DAVID and R software to investigate the mechanism of SV in the therapy of CRC. The GO analysis included 3 levels: BP, CC, and MF. The results of BP, MF, and CC are, respectively, shown in Figure 3A-3F. BP mainly involved aspects of cell division, response to inorganic substance, and cellular response to lipid, response to oxygen levels, regeneration. MF was primarily related to the receptor ligand activity, protein kinase regulator activity, and kinase regulator activity, cytokine activity, cyclin-dependent protein serine/threonine kinase regulator activity. Only four terms of CC were statistically significant, they were mostly involved in cyclin-dependent protein kinase holoenzyme complex, serine/threonine protein kinase complex, protein kinase complex, transferase complex, and transferring phosphorus-containing groups. The relevant signaling pathways connected to the CRC of SV were found using KEGG pathway analysis. The 5 significant KEGG pathways (P<0.05) are shown in Figure 3G,3H, including cellular senescence, the advanced glycation end product (AGE)-receptor for AGE (RAGE) signaling pathway in diabetic complications, cell cycle, p53 signaling pathway, and bladder cancer. As an example, for the p53 signaling pathway, the potential target and mechanism of the SV in the treatment of prostate cancer (PCa) are shown in Figure 3.
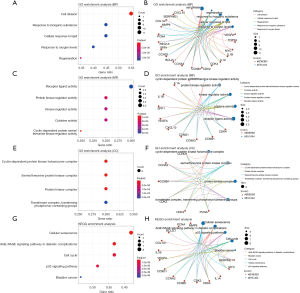
Molecular docking validation
By estimating the binding energy, molecular docking can model the interaction between the ligand and the receptor and forecast the affinity. The KEGG results showed that 6 genes, BCL2L1 (PDB:7jgw, 1.30Å), CDK2 (PDB:6q4g, 0.98Å), CCNB1 (PDB:2b9r, 2.90Å), SERPINE1 (PDB:7aqf, 1.77Å), CDK4 (PDB:3g33, 3.00Å), and CCND1 (PDB:2w96, 2.30Å), are involved in the p53 signaling pathway, so we selected them for subsequent analysis. We used the 6 genes and their corresponding ingredients obtained as receptors and ligands for molecular docking. The results showed strong affinity, five hydrogen bonds are formed between CDK2 and swerchirin, with a binding-energy value of −7.61 kcal/moL, swerchirin was able to bind with CDK2 at residues ASP-86, HJK-301, GLN-131 and ILE-10 (Figure 4A). Six hydrogen bonds are formed between BCL2L1 and quercetin, with a binding-energy value of −6.81 kcal/moL, Quercetin was able to bind with BCL2L1 at residues GLU-153, ILE-114, LEU-150, LYS-157 and THR-109 (Figure 4B). (E)-2-Nonenal interacted with SERPINE1 active participation of specific residues GLU-281 and HIS-261, the binding energy value of −5.13 kcal/moL, with three hydrogen bonds (Figure 4C). Four hydrogen bonds are formed between SERPINE1 and oleic acid, it was able to bind at residues ALA-24, GLN-375 and SER-27 with a binding-energy value of −4.99 kcal/mol (Figure 4D).
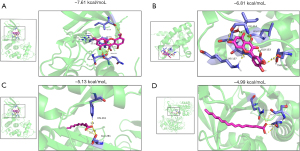
Discussion
In the present study, a network pharmacology-based approach and molecular docking were performed to identify bioactive substances and the molecular basis for SV’s ability to cure CRC. Correlation analysis showed that these target genes are strongly associated with CRC. CRC-associated genes were obtained by analysis of multiple GEO datasets. By looking for the point where SV targets and CRC targets overlap, 20 genes were found. The PPI network revealed a high correlation between any 2 genes, indicating that these genes may be crucial to the function of SV against CRC. We found that these 20 potential targets were associated with 6 components including palmitic acid, quercetin, linolenic acid, oleic acid, swerchirin and (E)-2-Nonenal.
By inputting common targets for GO and KEGG analyses, the main significantly enriched BP term was mainly related to cell division, and relevant study has confirmed that CDK family and cell division plays an important role in cancers (33). The cell cycle ensures correct cell division, the loss of cell cycle is a hallmark of cancer for CRC (34). Less of inorganic substance may be associated with and increase the risk of CRC (35). The targets involved CDK4, COL1A1, VEGFA, REG1A mainly included which are mainly involved response to oxygen levels, and AGE-RAGE signaling pathway augments oxidative stress and inflammation that collectively promotes tumorigenesis (36), it is of great significance that SV inhibits AGE-RAGE signaling pathway for cancer prevention and therapeutics. Regeneration term is of importance for proliferation, angiogenesis and tumorigenesis in cancers (37), it indicates SV has a potential effect of preventing angiogenesis. MF and CC enrichment terms include the receptor ligand activity, protein kinase regulator activity, and kinase regulator activity, cytokine activity, cyclin-dependent protein serine/threonine kinase regulator activity, cyclin-dependent protein kinase holoenzyme complex, serine/threonine protein kinase complex, protein kinase complex, transferase complex, and transferring phosphorus-containing groups, these terms illustrate the complexity of the mechanism of SV inhibits CRC by protein kinase and receptor ligand activity. The protein kinases contribute to the onset and progression of almost all types of cancer, therefore the possibility of blocking them with targeted treatment could have major clinical therapeutic utility (38,39), maybe SV plays the role of kinase inhibitor in the treatment of CRC. To further explore the potential mechanism of SV in treating CRC, we found that the KEGG terms are closely related to p53 signaling pathway, p53 is an essential tumor suppressor that controls a variety of cellular responses to guard against the growth of cancer (40). In human CRC, p53 signaling is typically deactivated through p53 regulator changes or mutations (41). Some 43% of CRCs have p53 mutations, which decrease the tumor-suppressor activity of wild-type p53 and frequently result in neo-morphic functions that promote carcinogenesis. Meanwhile, p53 is a promising therapeutic target (42). Therefore, SV may inhibit tumor cell proliferation, angiogenesis, and metastasis by acting on the P53 pathway. Further investigate needed how SV regulates the p53 pathway to inhibit CRC development. In addition, the study found that SV extract significantly inhibits angiogenesis and has the potential to be an effective angiogenesis inhibitor (43). Matrix metalloproteinase 9 (MMP9) and vascular endothelial growth factor A (VEGFA) as key molecules promoting angiogenesis (44,45). Our analysis showed that SV inhibited angiogenesis related molecules MMP9 and VEGFA, and further experiments were required to verify that SV inhibit angiogenesis in CRC.
We then performed molecular docking of 6 target genes (BCL2L1, CDK2, CCNB1, SERPINE1, CDK4, and CCND1) involved in the p53 signaling pathway. When the binding energy is less than zero, the small-molecule ligand can spontaneously bind to the macromolecular receptor (46). The 2 displayed greater binding activity when the binding energy was less than −5.0 kcal/mol (47). Results of molecular docking indicated showed the lowest energy value between BCL2L1 and quercetin, CDK2 and swerchirin, SERPINE1 and (E)-2-Nonenal, SERPINE1 and oleic acid. Quercetin, swerchirin, (E)-2-Nonenal and oleic acid, as the main ingredients, selectively acts on the p53 signaling pathway, which may be an important molecular mechanism of the SV in the treatment of CRC in this study. CDK2 plays a critical role in both the malignant transformation of cells and tumorigenesis, the inhibitors exhibit an encouragingly anti-tumor effect (48). At present, there are few reports about swerchirin, and the potential role of swerchirin and CDK2 in CRC still needs to be further explored.
Conclusions
This study used network pharmacology to undertake a preliminary investigation of SV’s superior substances, superior targets, and efficient routes. SV exerts pharmacological effects in CRC, including regeneration, cell cycle, protein kinase regulator activity, p53 signaling pathway and others. The main molecular docking is BCL2L1 and quercetin, CDK2 and swerchirin, SERPINE1 and (E)-2-Nonenal, SERPINE1 and oleic acid. Superior monomer components in SV can be further uncovered with the use of network pharmacology, which may serve as the foundation for the creation of novel medications, although this still needs to be tested. Our findings offer a reference for further investigation of the mechanism underlying the therapeutic effect of SV in CRC.
Acknowledgments
Funding: This study was supported by the Hebei Provincial Administration of Traditional Chinese Medicine Project (No. 2022147).
Footnote
Reporting Checklist: The authors have completed the MDAR reporting checklist. Available at https://jgo.amegroups.com/article/view/10.21037/jgo-23-83/rc
Peer Review File: Available at https://jgo.amegroups.com/article/view/10.21037/jgo-23-83/prf
Conflicts of Interest: All authors have completed the ICMJE uniform disclosure form (available at https://jgo.amegroups.com/article/view/10.21037/jgo-23-83/coif). The authors have no conflicts of interest to declare.
Ethical Statement: The authors are accountable for all aspects of the work in ensuring that questions related to the accuracy or integrity of any part of the work are appropriately investigated and resolved. The study was conducted in accordance with the Declaration of Helsinki (as revised in 2013)
Open Access Statement: This is an Open Access article distributed in accordance with the Creative Commons Attribution-NonCommercial-NoDerivs 4.0 International License (CC BY-NC-ND 4.0), which permits the non-commercial replication and distribution of the article with the strict proviso that no changes or edits are made and the original work is properly cited (including links to both the formal publication through the relevant DOI and the license). See: https://creativecommons.org/licenses/by-nc-nd/4.0/.
References
- Bray F, Ferlay J, Soerjomataram I, et al. Global cancer statistics 2018: GLOBOCAN estimates of incidence and mortality worldwide for 36 cancers in 185 countries. CA Cancer J Clin 2018;68:394-424. [Crossref] [PubMed]
- Arnold M, Sierra MS, Laversanne M, et al. Global patterns and trends in colorectal cancer incidence and mortality. Gut 2017;66:683-91. [Crossref] [PubMed]
- Xiang Y, Guo Z, Zhu P, et al. Traditional Chinese medicine as a cancer treatment: Modern perspectives of ancient but advanced science. Cancer Med 2019;8:1958-75. [Crossref] [PubMed]
- Wang CZ, Calway T, Yuan CS. Herbal medicines as adjuvants for cancer therapeutics. Am J Chin Med 2012;40:657-69. [Crossref] [PubMed]
- Mao D, Feng L, Huang S, et al. Meta-Analysis of Xihuang Pill Efficacy When Combined with Chemotherapy for Treatment of Breast Cancer. Evid Based Complement Alternat Med 2019;2019:3502460. [Crossref] [PubMed]
- Wang Q, Jiao L, Wang S, et al. Maintenance Chemotherapy With Chinese Herb Medicine Formulas vs. With Placebo in Patients With Advanced Non-small Cell Lung Cancer After First-Line Chemotherapy: A Multicenter, Randomized, Double-Blind Trial. Front Pharmacol 2018;9:1233. [Crossref] [PubMed]
- Tang YC, Zhang Y, Zhou J, et al. Ginsenoside Rg3 targets cancer stem cells and tumor angiogenesis to inhibit colorectal cancer progression in vivo. Int J Oncol 2018;52:127-38. [PubMed]
- Zhang Q, Chen X, Luo Y, et al. Fuzi Enhances Anti-Tumor Efficacy of Radiotherapy on Lung Cancer. J Cancer 2017;8:3945-51. [Crossref] [PubMed]
- Liu X, Tian S, Liu M, et al. Wogonin inhibits the proliferation and invasion, and induces the apoptosis of HepG2 and Bel7402 HCC cells through NF-κB/Bcl-2, EGFR and EGFR downstream ERK/AKT signaling. Int J Mol Med 2016;38:1250-6. [Crossref] [PubMed]
- Mathonnet M, Perraud A, Christou N, et al. Hallmarks in colorectal cancer: angiogenesis and cancer stem-like cells. World J Gastroenterol 2014;20:4189-96. [Crossref] [PubMed]
- Tian Y, Xin D, Gao D. Research progress of Vaccaria segetalis. China Continuing Medical Education 2015;201-2.
- Feng L, Hua H, Qiu L, et al. Pharmacodynamic study on the inhibition of angiogenesis by extracts from Wang Bu Liu Xing. Chinese Traditional and Herbal Drugs 2009;40:1949-52.
- Li D, Wang W, Xiang L, et al. The type of Traditional Chinese Medicine syndrome predicts prognosis and chemotherapeutic outcomes in colorectal cancer. European Journal of Integrative Medicine 2020;33:101026. [Crossref]
- Li Q, Chen JX, Wu Y, et al. The mechanism of FZXJJZ decoction suppresses colorectal liver metastasis via the VDR/TGF-β/Snail1 signaling pathways based on network pharmacology-TCGA data-transcriptomics analysis. J Ethnopharmacol 2022;287:114904. [Crossref] [PubMed]
- Chu XD, Zhang YR, Lin ZB, et al. A network pharmacology approach for investigating the multi-target mechanisms of Huangqi in the treatment of colorectal cancer. Transl Cancer Res 2021;10:681-93. [Crossref] [PubMed]
- Zuo H, Zhang Q, Su S, et al. A network pharmacology-based approach to analyse potential targets of traditional herbal formulas: An example of Yu Ping Feng decoction. Sci Rep 2018;8:11418. [Crossref] [PubMed]
- Li X, Wei S, Niu S, et al. Network pharmacology prediction and molecular docking-based strategy to explore the potential mechanism of Huanglian Jiedu Decoction against sepsis. Comput Biol Med 2022;144:105389. [Crossref] [PubMed]
- Zeng L, Yang K. Exploring the pharmacological mechanism of Yanghe Decoction on HER2-positive breast cancer by a network pharmacology approach. J Ethnopharmacol 2017;199:68-85. [Crossref] [PubMed]
- Jing C, Sun Z, Xie X, et al. Network pharmacology-based identification of the key mechanism of Qinghuo Rougan Formula acting on uveitis. Biomed Pharmacother 2019;120:109381. [Crossref] [PubMed]
- Ru J, Li P, Wang J, et al. TCMSP: a database of systems pharmacology for drug discovery from herbal medicines. J Cheminform 2014;6:13. [Crossref] [PubMed]
- Shannon P, Markiel A, Ozier O, et al. Cytoscape: a software environment for integrated models of biomolecular interaction networks. Genome Res 2003;13:2498-504. [Crossref] [PubMed]
- Sun G, Li X, Wei J, et al. Pharmacodynamic substances in Salvia miltiorrhiza for prevention and treatment of hyperlipidemia and coronary heart disease based on lipidomics technology and network pharmacology analysis. Biomed Pharmacother 2021;141:111846. [Crossref] [PubMed]
- Rouillard AD, Gundersen GW, Fernandez NF, et al. The harmonizome: a collection of processed datasets gathered to serve and mine knowledge about genes and proteins. Database (Oxford) 2016;2016:baw100. [Crossref] [PubMed]
- Wang J, Ren J, Liu J, et al. Identification and verification of the ferroptosis- and pyroptosis-associated prognostic signature for low-grade glioma. Bosn J Basic Med Sci 2022;22:728-50. [Crossref] [PubMed]
- Chen X, Yuan Q, Liu J, et al. Comprehensive characterization of extracellular matrix-related genes in PAAD identified a novel prognostic panel related to clinical outcomes and immune microenvironment: A silico analysis with in vivo and vitro validation. Front Immunol 2022;13:985911. [Crossref] [PubMed]
- Zhao J, Guo C, Ma Z, et al. Identification of a novel gene expression signature associated with overall survival in patients with lung adenocarcinoma: A comprehensive analysis based on TCGA and GEO databases. Lung Cancer 2020;149:90-6. [Crossref] [PubMed]
- Ren J, Yuan Q, Liu J, et al. Identifying the role of transient receptor potential channels (TRPs) in kidney renal clear cell carcinoma and their potential therapeutic significances using genomic and transcriptome analyses. BMC Med Genomics 2022;15:156. [Crossref] [PubMed]
- Bardou P, Mariette J, Escudié F, et al. jvenn: an interactive Venn diagram viewer. BMC Bioinformatics 2014;15:293. [Crossref] [PubMed]
- Szklarczyk D, Gable AL, Lyon D, et al. STRING v11: protein-protein association networks with increased coverage, supporting functional discovery in genome-wide experimental datasets. Nucleic Acids Res 2019;47:D607-13. [Crossref] [PubMed]
- Yu G, Wang LG, Han Y, et al. clusterProfiler: an R package for comparing biological themes among gene clusters. OMICS 2012;16:284-7. [Crossref] [PubMed]
- Azfaralariff A, Farahfaiqah F, Shahid M, et al. Marantodes pumilum: Systematic computational approach to identify their therapeutic potential and effectiveness. J Ethnopharmacol 2022;283:114751. [Crossref] [PubMed]
- Li C, Du X, Liu Y, et al. A Systems Pharmacology Approach for Identifying the Multiple Mechanisms of Action for the Rougui-Fuzi Herb Pair in the Treatment of Cardiocerebral Vascular Diseases. Evid Based Complement Alternat Med 2020;2020:5196302. [Crossref] [PubMed]
- Long ZJ, Wang JD, Xu JQ, et al. cGAS/STING cross-talks with cell cycle and potentiates cancer immunotherapy. Mol Ther 2022;30:1006-17. [Crossref] [PubMed]
- Yu C, Chen F, Jiang J, et al. Screening key genes and signaling pathways in colorectal cancer by integrated bioinformatics analysis. Mol Med Rep 2019;20:1259-69. [Crossref] [PubMed]
- Takada I, Makishima M. Control of Inflammatory Bowel Disease and Colorectal Cancer by Synthetic Vitamin D Receptor Ligands. Curr Med Chem 2017;24:868-75. [Crossref] [PubMed]
- Waghela BN, Vaidya FU, Ranjan K, et al. AGE-RAGE synergy influences programmed cell death signaling to promote cancer. Mol Cell Biochem 2021;476:585-98. [Crossref] [PubMed]
- Li P, Gong Z, Shultz LD, et al. Mesenchymal stem cells: From regeneration to cancer. Pharmacol Ther 2019;200:42-54. [Crossref] [PubMed]
- Kannaiyan R, Mahadevan D. A comprehensive review of protein kinase inhibitors for cancer therapy. Expert Rev Anticancer Ther 2018;18:1249-70. [Crossref] [PubMed]
- Hainaut P, Plymoth A. Targeting the hallmarks of cancer: towards a rational approach to next-generation cancer therapy. Curr Opin Oncol 2013;25:50-1. [Crossref] [PubMed]
- Hernández Borrero LJ, El-Deiry WS. Tumor suppressor p53: Biology, signaling pathways, and therapeutic targeting. Biochim Biophys Acta Rev Cancer 2021;1876:188556. [Crossref] [PubMed]
- Liebl MC, Hofmann TG. The Role of p53 Signaling in Colorectal Cancer. Cancers (Basel) 2021;13:2125. [Crossref] [PubMed]
- Soussi T, Wiman KG. Shaping genetic alterations in human cancer: the p53 mutation paradigm. Cancer Cell 2007;12:303-12. [Crossref] [PubMed]
- Feng L, Hua H, Qiu L, et al. Study on antiangiogenesis effect of Vaccaria segetalis. Journal of Chinese Medicinal Materials 2009;32:1256-9. [PubMed]
- Huang H. Matrix Metalloproteinase-9 (MMP-9) as a Cancer Biomarker and MMP-9 Biosensors: Recent Advances. Sensors (Basel) 2018;18:3249. [Crossref] [PubMed]
- Zou G, Zhang X, Wang L, et al. Herb-sourced emodin inhibits angiogenesis of breast cancer by targeting VEGFA transcription. Theranostics 2020;10:6839-53. [Crossref] [PubMed]
- Trott O, Olson AJ. AutoDock Vina: improving the speed and accuracy of docking with a new scoring function, efficient optimization, and multithreading. J Comput Chem 2010;31:455-61. [PubMed]
- Wei M, Li H, Li Q, et al. Based on Network Pharmacology to Explore the Molecular Targets and Mechanisms of Gegen Qinlian Decoction for the Treatment of Ulcerative Colitis. Biomed Res Int 2020;2020:5217405. [Crossref] [PubMed]
- Zhang J, Gan Y, Li H, et al. Inhibition of the CDK2 and Cyclin A complex leads to autophagic degradation of CDK2 in cancer cells. Nat Commun 2022;13:2835. [Crossref] [PubMed]
(English Language Editor: J. Jones)