Identification and validation of a novel cuproptosis-related lncRNA signature for predicting colorectal cancer patients’ survival
Highlight box
Key findings
• This was the first study to develop a novel CRL signature that can predict the prognosis of CRC patients with high accuracy.
What is known and what is new?
• Cuproptosis is a novel form of cell death referred to as copper-dependent cytotoxicity. The regulation of proptosis is becoming an increasingly popular cancer treatment modality. To date, few studies have attempted to identify the CRLs in CRC.
• Using data from The Cancer Genome Atlas database, we first analyzed the CRLs and then identified the CRLs that showed good performance in predicting survival.
What is the implication, and what should change now?
• We constructed and validated a robust prognostic model that comprised 22 CRLs in CRC. Our model may be used to prognosticate the clinical outcomes and treatments responses in CRC patients.
Introduction
Colorectal cancer (CRC) is currently one of the most frequently diagnosed tumors in China (1). The standard treatment for operable CRC is radical surgery followed by adjuvant chemotherapy; however, the overall prognosis of advanced CRC patients remains poor (2). Consequently, research urgently needs to be conducted to identify more prognostic markers and elucidate the mechanisms underlying the progression of CRC.
As an important intracellular mineral nutrient, copper (Cu) plays a role in several essential cellular processes, including mitochondrial respiration, oxygen metabolism, and iron uptake, and is also a regulator of some biological pathways (3,4). Previous study has reported high levels of Cu in various malignant tumors and shown that the dysregulation of Cu homeostasis may trigger cytotoxicity, leading to alterations in the intracellular Cu levels and thus affecting the progression of cancer (5). Recently, Tsvetkov et al. identified a novel mechanism, which they termed cuproptosis, by which excessive intracellular concentrations of Cu lead to cell death (6), and identified an essential link between mitochondrial respiration and Cu-induced cell death, which provided a perspective direction for the possible combination of a number of therapeutic interventions.
Long non-coding RNA (lncRNA) refers to a type of non-coding RNA that is >200 nucleotides in length, and lncRNAs have been widely analyzed in CRC in recent decades (7-10). Numerous experiments have shown that lncRNAs are related to cell death (11-14); however, to date, no studies have attempted to identify the cuproptosis-related long non-coding RNAs (CRLs) in CRC. The prognostic signature based on CRLs have been developed and validated in several solid tumors including lung cancer, breast cancer and gastric cancer (15-17). Thus, we hypothesized that CRLs based prognostic signature will perform good in predicting CRC prognosis and research needs to be conducted to identify the key CRLs with prognostic significance.
In this study, the RNA-sequencing data of CRC patients with complete follow-up information were obtained from The Cancer Genome Atlas (TCGA) database, and a total of 22 CRLs were identified and used to construct a prognostic signature. A functional enrichment analysis was performed to investigate the effects of CRLs on CRC. Finally, we focused on the prognostic CRLs that were highly expressed in CRC tissues and tentatively validated their role in regulating cuproptosis in vitro. Our findings could help to predict the prognosis of CRC patients and provide new insights into cuproptosis regulation. We present the following article in accordance with the TRIPOD reporting checklist (available at https://jgo.amegroups.com/article/view/10.21037/jgo-23-228/rc).
Methods
RNA expression dataset
The RNA expression data of CRC patients from TCGA were downloaded from the Genomic Data Commons (GDC) database (https://portal.gdc.cancer.gov/). Patients with RNA-sequencing data and complete follow-up information were included in this analysis. The somatic mutation data were downloaded from the GDC database (https://portal.gdc.cancer.gov/repository). This study was conducted in accordance with the Declaration of Helsinki (as revised in 2013).
Somatic mutation analyses
The somatic mutation data [presented in the mutation annotation format (MAF)-file format] were downloaded from the GDC database (https://portal.gdc.cancer.gov/repository). The mutation distribution of the cuproptosis-related genes (CRGs) were plotted using the R “maftools” package (Bioconductor).
Differential expression analysis
To identify the differentially expressed lncRNAs between the normal and cancer tissues, the R “LIMMA” package (Bioconductor)was used and the threshold was set as a fold change >1 and a false discovery rate (FDR) <0.05.
Identification of the CRLs
Based on a previous report, 10 CRGs were identified in this study (6). A Pearson correlation analysis was performed based on the CRGs and lncRNA expression profiles to identify the CRLs that met the following criteria: |R| >0.3 and P<0.05. By further identifying the overlapping differentially expressed lncRNAs and CRLs, the differentially expressed CRLs were lastly determined.
Construction of the CRL prognostic signature
First, a univariate Cox analysis was conducted to identify the prognostic differentially expressed CRLs. To construct and validate the CRL prognostic signature, the patients in TCGA cohort were randomly allocated to the training and validation sets at a ratio of 1:1. Next, a least absolute shrinkage and selection operator (LASSO) Cox regression analysis of the training set was performed to select the optimal candidates. Ultimately, 22 CRLs were identified and used to establish the gene signature for calculating the risk score, which was calculated as follows:
The median value of the risk_score calculated in the training was set as the threshold, and the patients in the training and validation sets were divide into the low- and high-risk groups based on this threshold. A Kaplan-Meier survival analysis was then conducted to compare the differences between the survival curves. Next, a survival receiver operating characteristic (ROC) curve analysis was conducted to assess the prognostic accuracy of the developed signature.
Functional enrichment analysis
The differentially expressed genes between the low- and high-risk groups were identified based on the method mentioned above. A P value <0.05 and a fold change >1.5 were set as the threshold for significance. A functional annotation of the enriched Kyoto Encyclopedia of Genes and Genomes (KEGG)/Gene Ontology (GO) biological processes was then performed using the clusterProfiler R package (Bioconductor).
Cell counting kit-8 (CCK-8) assays and the detection of ROS production
The CCK-8 assay kit (DOJINDO, Japan) was used to evaluate the cell proliferation ability of the CRC cells in accordance with the manufacturer’s instructions. Briefly, the procedure of CCK-8 assay involves seeding cells in a 96-well plate and then adding CCK-8 reagent to the plate. After incubation for 2 h, we measure the absorbance of the wells at 450 nm using a microplate reader. Lastly, we calculate the relative cell viability of the cells by comparing the absorbance of experimental wells with control wells containing untreated cells. The intracellular reactive oxygen species (ROS) level was detected by dichloro-dihydro-fluorescein diacetate assays using the Cellular ROS Assay Kit (Abcam, Cambridge, UK).
Statistical analyses
The Wilcoxon rank-sum test was employed for analyzing data with a skewed distribution, whereas the Student’s test was utilized for data that exhibited normal distribution, with an aim to ascertain the contrast in between the groups. Furthermore, a Spearman correlation analysis was conducted to investigate the correlation between CRGs and CRLs. A log-rank test was used to analyze the differences between the survival curves. All the statistical analyses were performed using R software (R version 3.4.2; https://www.r-project.org/).
Results
Genetic variations and expression profiles of the CRGs in CRC
In previous reports, 10 CRGs have been identified as involved in cancer (6). The mutation frequencies of the 10 CRGs are depicted in Figure 1A, and a low mutation rate was observed among these CRGs. To analyze the expression correlations among the CRGs, a correlation heat-map was plotted and a weak or negative correlation was observed (Figure 1B). We then compared the expression differences between the normal and cancer tissues among the CRGs and found that the expression of cyclin dependent kinase inhibitor 2A (CDKN2A) and glutaminase (GLS) were significantly upregulated in the cancer tissues, while dihydrolipoamide S-acetyltransferase (DLAT), dihydrolipoamide dehydrogenase (DLD), ferredoxin 1 (FDX1), lipoic acid synthetase (LIAS), metal regulatory transcription factor 1 (MTF1), and pyruvate dehydrogenase E1 subunit beta (PDHB) were downregulated (Figure 1C), which confirmed that the cuproptosis process was dysregulated in CRC.
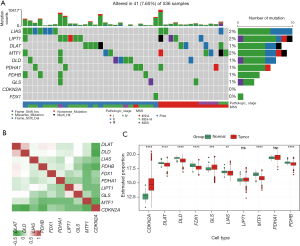
Identification of the differentially expressed and prognostic CRLs
First, we compared the lncRNA expression differences between the normal and cancer tissues and identified 4,094 differentially expressed lncRNAs (|fold change| >1, FDR <0.05), of which 2,791 were upregulated and 1303 were downregulated (Figure 2A, available online: https://cdn.amegroups.cn/static/public/jgo-23-228-1.xlsx). A Spearman correlation analysis was then conducted of the lncRNAs and CRGs, and 729 CRLs were identified (available online: https://cdn.amegroups.cn/static/public/jgo-23-228-2.xlsx). By identifying the overlapping differentially expressed lncRNAs and CRLs, 406 differentially expressed CRLs were identified (Figure 2B). We then performed a univariate Cox analysis and identified 31 prognostic CRLs, of which 8 CRLs were favorable for survival and 23 were unfavorable (Figure 2C). The expression correlations between the CRGs and the 31 differentially expressed and prognostic CRLs are depicted in Figure 2D.
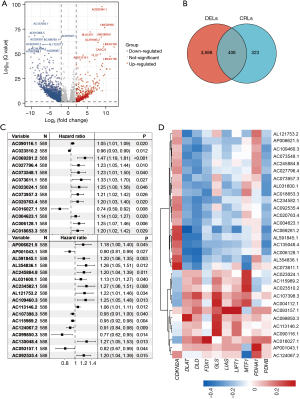
Construction and validation of the CRL prognostic signature
A LASSO regression model was applied to the training set, and the minimize λ method resulted in 22 CRLs (Figure 3A,3B). The risk score of each patient was then calculated in the training set based on the expression levels of the 22 CRLs and their corresponding LASSO Cox regression coefficients. The coefficients of the selected 22 CRLs are shown in website: https://cdn.amegroups.cn/static/public/jgo-23-228-3.xlsx. Based on the median risk score, patients in the training set were separated into the low-risk group (N=147) and the high-risk group (N=147). The distribution of the risk scores and survival status of patients are shown in Figure 3C. Remarkably, there was a progressive escalation in mortality risk concomitant with rising risk scores. The identical assessments were applied to the validation cohort, and a commensurate distribution was identified (Figure 3D).
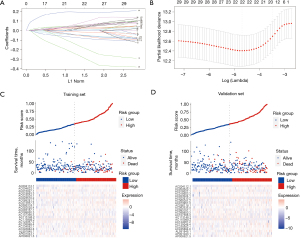
The survival analysis results further confirmed that the patients with higher risk scores had significantly worse survival outcomes than those with lower risk scores in both the training (Figure 4A) and validation sets (Figure 4B). Time-dependent ROC curve analyses at 3, 5, and 7 years were conducted, and the lncRNA signature had an outstanding ability to predict the overall survival of the patients. The 5-year AUC of the lncRNA signature reached 0.820 and 0.810 in the training (Figure 4C) and validation (Figure 4D) sets, respectively.
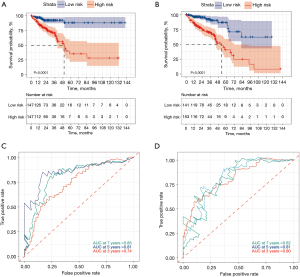
Independence and prognostic accuracy of the CRL prognostic signature
Univariate and multivariate Cox analyses were then conducted to test the independence of the CRL prognostic signature in predicting survival. After adjusting the other clinicopathological variables, the CRL signature remained a powerful and independent factor in the whole TCGA cohort (Figure 5A,5B). To facilitate the clinical use of the CRL signature, a nomogram was developed based on the multivariate analysis results (Figure 5C). The CRL signature, age, tumor stage were integrated into the nomogram. The combined nomogram had an excellent predictive ability and performed better than any other prognostic factors alone in predicting survival (Figures 5D,5E).
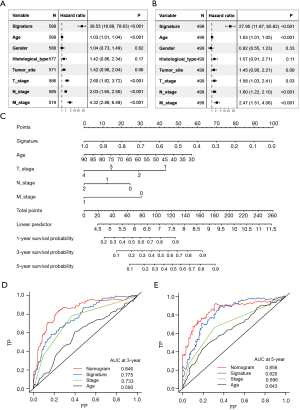
Functional enrichment analysis of the risk scores
To characterize the functional enrichment of the risk groups, we compared the messenger RNA expression differences between the low- and high-risk groups to identify the differentially expressed genes and found that 1,108 genes were upregulated in the high-risk group (available online: https://cdn.amegroups.cn/static/public/jgo-23-228-4.xlsx). We then performed a functional enrichment analysis of these upregulated genes and found that these genes mainly participated in the tumor invasion and metastasis-related biological processes, including axonogenesis, extracellular matrix (ECM) organization, extracellular structure organization, and collagen fibril organization (Figure 6A). Further, the KEGG pathway enrichment analysis confirmed that several critical oncogenic and metastatic pathways, including the Ras signaling pathway, mitogen-activated protein kinase (MAPK) signaling pathway, phosphatidylinositol 3-kinase (PI3K)/protein kinase B (AKT) signaling pathway, focal adhesion, and ECM-receptor interaction, were significantly enriched (Figure 6B).
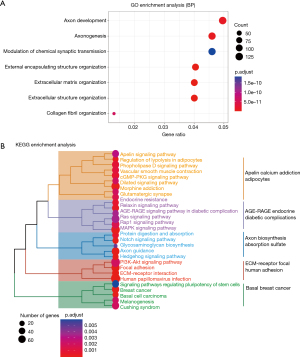
AC090116.1 prevents elesclomol-induced cuproptosis
To identify the lncRNAs that regulate cuproptosis, we focused on the upregulated CRL AC090116.1 (ENSG00000277223) in the cancer tissues with the highest fold change (Figure 7A). We then silenced AC090116.1 in the CRC cells (Figure 7B), and the cell proliferation assays showed that AC090116.1 attenuation significantly inhibited cell growth (Figure 7C,7D). The Cu chelator elesclomol-mediated Cu overload has been established to have considerable anti-cancer efficacy by inducing cell cuproptosis. Under elesclomol treatment, we found that AC090116.1 silencing significantly increased elesclomol-induced ROS production (Figure 7E) and inhibited cell viability (Figure 7F), which suggests that AC090116.1 has a potential role in preventing cell cuproptosis.
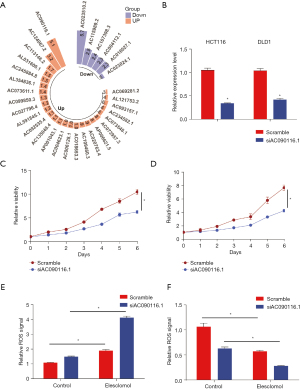
Discussion
A number of studies have attempted to investigate the factors regulating cell cuproptosis to identify promising therapeutic targets; however, few studies have focused on the lncRNAs involved in the cuproptosis of cancer. The CRLs in CRC need to be identified to determine the potential regulators of cuproptosis.
In this study, we comprehensively analyzed the expression profiles of 10 experimentally validated CRGs and found that the majority of the CRGs were differentially expressed between the normal and cancer tissues, which confirmed that the process of cuproptosis is dysregulated in CRC. Our correlation analysis identified 406 differentially expressed CRLs, and our subsequent Cox analysis identified 31 prognostic CRLs. Further, based on a LASSO regression analysis, a novel prognostic signature comprising 22 CRLs was developed. By applying the CRL signature to the training set patients, a significant difference was observed in the survival curves between the low- and high-risk patients. The result in training set was successfully internally validated in TCGA database, which indicated that the CRL signature had good reproducibility in CRC. The multivariate Cox analysis revealed the independent prognostic role of the prognostic signature. Further, the time-dependent ROC curve suggested that this signature had considerable prognostic accuracy in predicting survival. The prognostic signature and other clinical factors were then combined in a nomogram that showed an outstanding predictive ability and performed better than any other parameter alone. Thus, this study identified a novel CRL signature that could help to improve survival prediction and guide the individualized treatment and follow-up approaches adopted for CRC patients.
To further evaluate the mechanism by which this signature regulates the biological processes or signaling pathway activities in CRC, a functional enrichment analysis was performed, and the results showed that the processes of axonogenesis, ECM organization, extracellular structure organization, and collagen fibril organization, which are associated with tumor invasion, were also significantly enriched in the high-risk patients. Cancer metastasis pathways, such as the Ras signaling pathway, MAPK signaling pathway, PI3K-Akt signaling pathway, focal adhesion, and ECM-receptor interaction, were also enriched. These results suggest that cuproptosis may be inhibited in the high-risk group, and the CRLs might promote tumor metastasis through multiple pathways.
To date, few studies have investigated the function of the 22 prognostic CRLs identified in this signature in cancer. A previous study of CRC based on TCGA data confirmed that AL591845.1 and AC245884.8 are key survival-related lncRNAs (18). AC124067.2 is considered an autophagy-related lncRNA and is thought to be associated with the tumor immune microenvironment in non-small cell lung cancer (19). A prognostic analysis of the prognostic lncRNAs in hepatocellular carcinoma reveled that AC107398.3 effectively predicted survival in hepatocellular carcinoma patients with or without fibrosis (20). LncRNA AC099850.3 has been widely studied in cancer. The overexpression of AC099850.3 has been shown to be correlated with tumor progression and a poor prognosis in lung adenocarcinoma (21) and to promote hepatocellular carcinoma proliferation and invasion through the phosphatidylinositol-4,5-bisphosphate 3-kinase signaling (22). In addition, AC099850.3/NCAPG Axis was found associated with Resistance to EGFR Tyrosine-Kinase Inhibitors in Lung Adenocarcinoma (23). AC092535.4 has been proven to be an immune-related lncRNA and has been used to improve survival prediction in clear cell renal cell carcinoma (24).
To identify the lncRNAs that may regulate cuproptosis, we focused on the CRL of AC090116.1 which is the most highly expressed in cancer tissues. No previous studies have investigated the prognostic role and molecular function of AC090116.1 in cancer. In this study, we found that AC090116.1 was highly expressed in the cancer tissues, and the high expression of AC090116.1 was associated with poor survival in CRC. Further in vitro experiments showed that silencing AC090116.1 significantly increased elesclomol-induced ROS production and inhibited cell viability, which suggests that AC090116.1 has a potential role in preventing cell cuproptosis. Besides the CRLs identified in this study, lots of CRLs have been detected in other tumors. A Recent study found that lncRNA XIST/miR-125a-5p/CDKN2A regulatory axis may be involved in the progression of uterine corpus endometrial carcinoma (25).
To the best of our knowledge, this was the first study to identify CRLs and establish a prognostic CRL-based signature in CRC. However, this study still had several limitations. First, the performance of this signature needs to be externally validated. Second, information about several other important clinicopathological features, such as differentiation and the number of lymph nodes, was not available in the TCGA database. Finally, the mechanism by which AC090116.1 regulates cuproptosis requires further research.
Conclusions
In conclusion, our study constructed and validated a robust prognostic model that comprised 22 CRLs in CRC. This model has the potential to prognosticate the outcomes of patients with colon cancer and may even augment their therapeutic management in a clinical setting.
Acknowledgments
Funding: None.
Footnote
Reporting Checklist: The authors have completed the TRIPOD reporting checklist. Available at https://jgo.amegroups.com/article/view/10.21037/jgo-23-228/rc
Peer Review File: Available at https://jgo.amegroups.com/article/view/10.21037/jgo-23-228/prf
Conflicts of Interest: Both authors have completed the ICMJE uniform disclosure form (available at https://jgo.amegroups.com/article/view/10.21037/jgo-23-228/coif). The authors have no conflicts of interest to declare.
Ethical Statement:
Open Access Statement: This is an Open Access article distributed in accordance with the Creative Commons Attribution-NonCommercial-NoDerivs 4.0 International License (CC BY-NC-ND 4.0), which permits the non-commercial replication and distribution of the article with the strict proviso that no changes or edits are made and the original work is properly cited (including links to both the formal publication through the relevant DOI and the license). See: https://creativecommons.org/licenses/by-nc-nd/4.0/.
References
- Siegel RL, Miller KD, Goding Sauer A, et al. Colorectal cancer statistics, 2020. CA Cancer J Clin 2020;70:145-64. [Crossref] [PubMed]
- Dekker E, Tanis PJ, Vleugels JLA, et al. Colorectal cancer. Lancet 2019;394:1467-80. [Crossref] [PubMed]
- Oliveri V. Selective Targeting of Cancer Cells by Copper Ionophores: An Overview. Front Mol Biosci 2022;9:841814. [Crossref] [PubMed]
- Cobine PA, Moore SA, Leary SC. Getting out what you put in: Copper in mitochondria and its impacts on human disease. Biochim Biophys Acta Mol Cell Res 2021;1868:118867. [Crossref] [PubMed]
- Ruiz LM, Libedinsky A, Elorza AA. Role of Copper on Mitochondrial Function and Metabolism. Front Mol Biosci 2021;8:711227. [Crossref] [PubMed]
- Tsvetkov P, Coy S, Petrova B, et al. Copper induces cell death by targeting lipoylated TCA cycle proteins. Science 2022;375:1254-61. [Crossref] [PubMed]
- Wang L, Cho KB, Li Y, et al. Long Noncoding RNA (lncRNA)-Mediated Competing Endogenous RNA Networks Provide Novel Potential Biomarkers and Therapeutic Targets for Colorectal Cancer. Int J Mol Sci 2019;20:5758. [Crossref] [PubMed]
- Ogunwobi OO, Mahmood F, Akingboye A. Biomarkers in Colorectal Cancer: Current Research and Future Prospects. Int J Mol Sci 2020;21:5311. [Crossref] [PubMed]
- Luo XJ, Zhao Q, Liu J, et al. Novel Genetic and Epigenetic Biomarkers of Prognostic and Predictive Significance in Stage II/III Colorectal Cancer. Mol Ther 2021;29:587-96. [Crossref] [PubMed]
- Chen S, Shen X. Long noncoding RNAs: functions and mechanisms in colon cancer. Mol Cancer 2020;19:167. [Crossref] [PubMed]
- Zhang X, Yao J, Shi H, et al. LncRNA TINCR/microRNA-107/CD36 regulates cell proliferation and apoptosis in colorectal cancer via PPAR signaling pathway based on bioinformatics analysis. Biol Chem 2019;400:663-75. [Crossref] [PubMed]
- Ren J, Ding L, Zhang D, et al. Carcinoma-associated fibroblasts promote the stemness and chemoresistance of colorectal cancer by transferring exosomal lncRNA H19. Theranostics 2018;8:3932-48. [Crossref] [PubMed]
- Jiang L, Zhao XH, Mao YL, et al. Long non-coding RNA RP11-468E2.5 curtails colorectal cancer cell proliferation and stimulates apoptosis via the JAK/STAT signaling pathway by targeting STAT5 and STAT6. J Exp Clin Cancer Res 2019;38:465. [Crossref] [PubMed]
- Huan L, Guo T, Wu Y, et al. Hypoxia induced LUCAT1/PTBP1 axis modulates cancer cell viability and chemotherapy response. Mol Cancer 2020;19:11. [Crossref] [PubMed]
- Feng A, He L, Chen T, et al. A novel cuproptosis-related lncRNA nomogram to improve the prognosis prediction of gastric cancer. Front Oncol 2022;12:957966. [Crossref] [PubMed]
- Jiang ZR, Yang LH, Jin LZ, et al. Identification of novel cuproptosis-related lncRNA signatures to predict the prognosis and immune microenvironment of breast cancer patients. Front Oncol 2022;12:988680. [Crossref] [PubMed]
- Wang X, Jing H, Li H. A novel cuproptosis-related lncRNA signature to predict prognosis and immune landscape of lung adenocarcinoma. Transl Lung Cancer Res 2023;12:230-46. [Crossref] [PubMed]
- Chen J, Song Y, Li M, et al. Comprehensive analysis of ceRNA networks reveals prognostic lncRNAs related to immune infiltration in colorectal cancer. BMC Cancer 2021;21:255. [Crossref] [PubMed]
- Jiang H, Xu A, Li M, et al. Seven autophagy-related lncRNAs are associated with the tumor immune microenvironment in predicting survival risk of nonsmall cell lung cancer. Brief Funct Genomics 2022;21:177-87. [Crossref] [PubMed]
- Ye J, Wu S, Pan S, et al. Risk scoring based on expression of long non-coding RNAs can effectively predict survival in hepatocellular carcinoma patients with or without fibrosis. Oncol Rep 2020;43:1451-66. [Crossref] [PubMed]
- Chen X, Guo J, Zhou F, et al. Over-Expression of Long Non-Coding RNA-AC099850.3 Correlates With Tumor Progression and Poor Prognosis in Lung Adenocarcinoma. Front Oncol 2022;12:895708. [Crossref] [PubMed]
- Zhong F, Liu S, Hu D, et al. LncRNA AC099850.3 promotes hepatocellular carcinoma proliferation and invasion through PRR11/PI3K/AKT axis and is associated with patients prognosis. J Cancer 2022;13:1048-60. [Crossref] [PubMed]
- Bao J, Wu Y, Zhang K, et al. AC099850.3/NCAPG Axis Predicts Poor Prognosis and is Associated with Resistance to EGFR Tyrosine-Kinase Inhibitors in Lung Adenocarcinoma. Int J Gen Med 2022;15:6917-30. [Crossref] [PubMed]
- Zhang Z, Tang Y, Liu Y, et al. A Novel Immune-Related lncRNA-Based Model for Survival Prediction in Clear Cell Renal Cell Carcinoma. J Immunol Res 2021;2021:9921466. [Crossref] [PubMed]
- Chen Y. Identification and Validation of Cuproptosis-Related Prognostic Signature and Associated Regulatory Axis in Uterine Corpus Endometrial Carcinoma. Front Genet 2022;13:912037. [Crossref] [PubMed]
(English Language Editor: L. Huleatt)