A novel model based on protein post-translational modifications comprising the immune landscape and prediction of colorectal cancer prognosis
Highlight box
Key findings
• We constructed a nomogram model that incorporates clinical and pathological factors and post-translational modification data to predict patient outcomes of colorectal cancer (CRC), and verified by cell experiments.
What is known and what is new?
• Post-translational modification, as a regulation of most eukaryotic proteins, could modify specific amino acids to modulate the conformation, activity, interaction, stability, and spatial distribution of proteins. Phosphorylation is a common post-translational modification that is critical in cancer development.
• There are no relevant studies on prognostic phosphorylation factors in colon adenocarcinoma. We established and validated a novel nomogram model related to post-translational modification that can predict prognosis and guide the treatment of CRC.
What is the implication, and what should change now?
• Our post-translational modification model could predict clinical risks and help evaluate clinical decision-making. The model may provide individualized management and treatment for patients with CRC.
• Some relevant inhibitors of phosphorylated molecules may be considered and researched for the treatment of colon cancer.
Introduction
Colorectal cancer (CRC) is one of the most common cancers worldwide, and its incidence and mortality have been increasing among individuals younger than 50 years (1,2). Due to its metastasis propensity and gene heterogeneity, targeting CRC for effective prevention or therapeutic interventions remains challenging (3,4). This necessitates the development of prognostic and therapeutic indicators for CRC.
Recent technological advances in sequencing and bioinformatics analysis have led to the identification of various molecular markers as predictors for tumor diagnosis, prognosis, and treatment. Post-translational modification (PTM), such as phosphorylation, acetylation, ubiquitination, and glycosylation, can modulate the structure and function of proteins (5). Several studies have demonstrated an association between PTM and tumor development, progression, and therapeutic response, with phosphorylation being one of the most common PTM types, primarily phosphorylating serine, threonine, or tyrosine residues and participating in signaling pathways (6-10). Abnormal phosphorylation has been identified as closely correlated with tumorigenesis and the development of CRC, showing potential as valuable biomarkers for early diagnosis, chemoresistance, and individualized treatment (6-8).
Despite the potential of PTM as a prognostic marker, no prognostic models based on PTM have been reported in colon adenocarcinoma (COAD). Nomograms have been recognized as excellent predictive tools for survival prediction that combine biological variables with clinical factors simultaneously (11,12). In this study, we focused on the PTM differences in COAD and constructed a nomogram model to predict CRC patients’ 1-, 3-, and 5-year overall survival (OS), which could be beneficial for individual treatments and outcomes. We present this article in accordance with the TRIPOD and MDAR reporting checklists (available at https://jgo.amegroups.com/article/view/10.21037/jgo-24-45/rc).
Methods
Acquisition and processing of data
The Cancer Genome Atlas (TCGA) (https://portal.gdc.cancer.gov/repository) was retrieved to acquire the transcriptomic, proteomic, and relevant clinicopathological data comprising 316 CRC patients. Then, we randomly partitioned patients into training (discovery cohort, n=120) and test (validation cohort, n=196) groups. The data underwent background adjustment and normalization utilizing a style of fragments per kilobase million (FPKM) (13). The detailed clinical data are available at https://cdn.amegroups.cn/static/public/10.21037jgo-24-45-1.xlsx. In addition, we acquired information on 89 PTM sites and protein expression by retrieving the TCGA-COAD dataset and searching PubMed. The study was conducted in accordance with the Declaration of Helsinki (as revised in 2013).
Development of a risk model to analyze the risk score
Ten PTM sites closely associated with OS were selected through a univariate Cox regression analysis in the training set (P<0.10). Aided by the “glmnet” package in R software (version 4.1.3), a least absolute shrinkage and selection operator (LASSO) Cox regression analysis was performed (P<0.05). The risk computing formula is as follows: (14). We applied a 10-fold cross validation with minimum criteria to obtain an optimal λ (15). The final value of λ yielded a minimum cross validation error. Consequently, 8 PTM sites, namely ACC_pS79, ARAF_pS299, Aurora.ABC_p288/p232/p198, CMET_pY1235, EGFR_pY1173, SRC_pY527, H2AX_pS139, and PI3K_p110_b were identified. Univariate and multivariate Cox regression models confirmed the independent predictive ability of the risk score. The resulting median cut-off value was used to stratify patients into high-risk and low-risk groups. To evaluate the accuracy of our risk score, we analyzed the training and test groups, and constructed a Kaplan-Meier (K-M) survival curve, and the log-rank test was used to assess the survival differences between groups. The predictive ability of the risk score was evaluated by the receiver operating characteristic (ROC) curve and the area under curve (AUC).
Construction and validation of the nomogram
To improve the predictive ability of COAD patients, we established a prognostic nomogram to assess the survival probability in 1, 3, and 5 years via the “rms” R package. As independent parameters, age, gender, clinic stage, pathological stage, and risk score were all considered. The calibration curve displays the probability of the 1-, 3-, and 5-year OS predicted by the nomogram. Through the use of the “ggDCA” package, a decision curve analysis (DCA) was implemented to determine the superiority of the nomogram.
Exploration of signaling pathways
We generated enrichment plots in the TCGA COAD cohort by employing “gene set enrichment analysis (GSEA)” software with the filtering criteria of a P value less than 0.05 (16). To identify the differentially expressed proteins (DEPs), the “limma” packages were used for the differential expression analysis [log2 fold change (FC) >0.2, false discovery rate (FDR) <0.05] between the training and test sets (17). After that, we used the “org.Hs.eg.db” package in R to perform both Gene Ontology (GO) functional and Kyoto Encyclopedia of Genes and Genomes (KEGG) pathway enrichment analyses on the screened 26 DEPs (https://metascape.org/) (18).
Analysis of immune landscape and tumor microenvironment (TME)
Given the close relationship between immune status and prognosis, we used the TIMER, QUANTISEQ, and CIBERSORT algorithms to seek insight into any potential associations between the risk score and immune cell infiltration (19). A Mann-Whitney U-test was used to compare the surface markers of relevant infiltrating immune cells between the high- and low-risk groups in order to determine the immune alteration. We also investigated into the relationships between PTM risk score and microsatellite state.
Assessment of the therapeutic sensitivity
To compare drug sensitivity in different risk score levels, we calculated tumor immune dysfunction and exclusion (TIDE, https://tide.dfci.harvard.edu/) of two risk groups. TIDE could evaluate immunotherapy effectiveness and immune evasion (20). In addition, utilizing the R packages “pRRophetic” (21), we computed the IC50 of several chemotherapy-related medications through the Wilcoxon signed-rank test.
Experiment in vitro
DLD-1 and HCT116 (human CRC cells) were cultured at 37 ℃, 5% CO2 in complete medium (RPMI DMEM supplemented with 100 µg/mL streptomycin, 100 IU/mL penicillin, and 10% fetal bovine serum). Control plasmid, pCMV-CMETY1235E, and pCMV-CMETY1235F, purchased from Miaolinbio company (Wuhan, China), were transfected into the cells using lipofectamine 3000 transfection reagent (Invitrogen, USA) when the cell density reached 70–80%. CMETY1235E is an amino-acid substitution mutant that mimics the phosphorylated state of CMET, while CMETY1235F mimics the unphosphorylated form of CMET. The phosphorylation status of CMET in DLD1 and HCT116 was verified by western blotting results. Primary antibodies used in the western blotting assay were as follows: anti-β Tubulin (#M1305-2 HUABIO, Hangzhou, China), anti-CMET (#A0040), anti-pCMET (#AP1339) (ABclonal Technology, Wuhan, China). Cell viability assays were performed using the methyl thiazolyl tetrazolium (MTT) colorimetric assay (Topscience Biological Technology, Shanghai, China) at a wavelength of 490 nm by spectrophotometry. Colony formation experiments were conducted to assess cell proliferation. Approximately 500 cells per well were seeded into a 6-well culture plate and incubated for 2 weeks at 37 ℃. After 24 hours of transfection, cells were seeded into 8-µm pore inserts (Corning, France) for transwell migration assays. Cell counts were obtained by ImageJ software. Finally, apoptosis and cell cycle assays were performed by NovoCyte flow Cytometer (Agilent, USA) and analyzed by NovoExpress software.
Statistical analysis
With the support of Pearson analysis, correlation coefficients were calculated. The K-M method, Cox regression model, and log-rank tests were employed to analyze the prognostic significance. The level of statistical significance was set at a P value of 0.05 or P value of 0.10 for all statistical data analyses, which were all conducted in a two-sided manner. All statistical analyses were carried out throughout the procedure using R software (version 4.1.3). Each experiment was a composite of three independent studies.
Results
Construction of a PTM prognostic signatures in TCGA COAD cohort
The flow diagram is provided in Figure 1. This study included 316 CRC patients. Demographic and clinical information of the patients are described in available at https://cdn.amegroups.cn/static/public/10.21037jgo-24-45-1.xlsx. We randomly selected 120 samples as the training cohort from TCGA-COAD in R, while the other samples were defined as the validation group. Baseline data were not statistically different between the two groups (Table S1). Proteomic results of the patients are listed in available at https://cdn.amegroups.cn/static/public/10.21037jgo-24-45-2.xlsx, including 89 PTM sites, which were all phosphorylation sites. Incorporation of 89 PTM sites into a univariate Cox regression analysis, 10 PTM were discovered to be significantly linked to OS (P<0.10) (Figure 2A). Then, LASSO regression was conducted to obtain an optimal λ (Figure 2B), selecting 8 PTM sites to generate the risk score model (Figure 2C). Each patient was assigned a separate risk score according to the formula. The functions of the prognostic PTM signatures were involved in fatty acid metabolism, DNA replication, chromosomal stability, and other vital cell biological processes (Table S2). Patients were classified into low- and high-risk score groups according to the calculated median value of the PTM score. The phosphorylation level profiles of the prognostic risk protein between the high-risk group and low-risk group were compared using a heatmap (Figure 2D). Meanwhile, we discovered that PI3K_p110_b was at a high phosphorylation level in the high-risk score group, whereas the opposite is true for ACC_pS79, ARAF_pS299, Aurora.ABC_p288/p232/p198, CMET_pY1235, EGFR_pY1173, SRC_pY527, and H2AX_pS139.
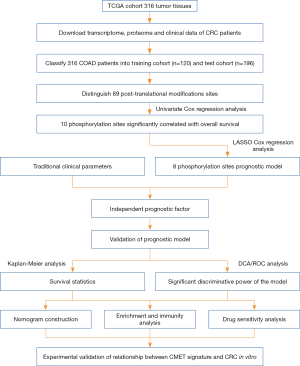
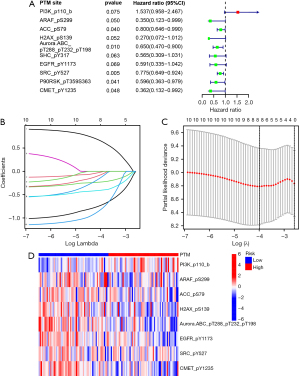
Verification of the PTM signatures and assessment of survival prediction
Univariate and multivariate Cox regression analyses were performed to evaluate the prognostic implication of the PTM score. In both training and test cohorts, univariate Cox regression analysis revealed that the PTM score, clinicopathologic stage, and age were significantly associated with OS (Figure 3A,3B). Multivariate Cox regression analysis proved the PTM score to be an independent prognostic variable (Figure 3C,3D). Additionally, in the test cohort, K-M analysis indicated that high phosphorylation levels of ACC_pS79 (P=0.01), ARAF_pS299 (P=0.03), SRC_pY527 (P=0.001), CMET_pY1235 (P=0.007) and H2AX_pS139 (P=0.03) were significantly related to high survival rates (Figure 3E-3I). On the contrary, the lower phosphorylation levels of EGFR_pY1173 (P=0.049) was associated with a higher chance of survival (Figure 3J). Furthermore, independent phosphorylation levels of Aurora.ABC_p288/p232/p198 (P=0.10) and PI3K_p110_b (P=0.11) were not associated with survival rates (Figure 3K,3L).
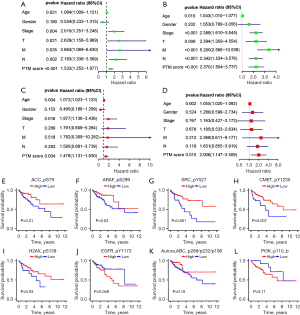
Validation of the PTM signatures and assessment of survival prediction
The K-M curves of the training, test, and whole groups revealed that higher risk score denoted worse prognosis, validating the applicability and reliability of the PTM score (Figure 4A-4C). Meanwhile, the risk lines and scatterplots displayed the risk score distribution together with the correlation between risk score and survival among all patients (Figure 4D-4F). The risk score was proportional to the mortality and the proportion of patients who were at high risk. Besides, we performed ROC to estimate the accuracy of the PTM predictive model in each subset. The AUC of the risk score for the survival probability at 1, 3, and 5 years respectively, were 0.602, 0.700, 0.752 in the training set, 0.635, 0.631, 0.706 in the test set, and 0.611, 0.574, 0.627 in the whole set (Figure 4G-4I). These findings verified that our PTM signatures was an optimal prognostic indicator.
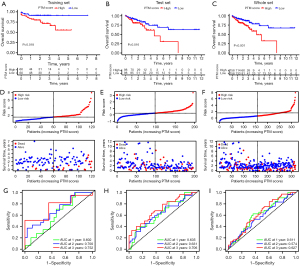
Construction of the nomogram and its link to clinical characteristics
Based on the clinicopathological characteristics, there were significant differences of PTM score in gender (P=0.02) and survival state (P=0.002) (Figure 5A,5B). After that, we generated a nomogram combining age, gender, clinical stage, T stage, M stage, N stage, and PTM signatures for improving the accuracy of the prognostic prediction model (Figure 5C). Each point of the factors represents their corresponding contributions to the probability of survival. In addition, the calibration curve suggested the consistence of our nomogram (Figure 5D). We also conducted DCA, showing that our nomogram was the most optimal predictor (Figure 5E).
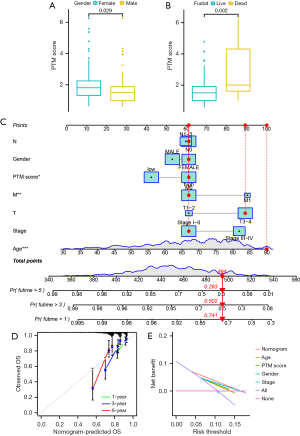
Exploration of function and signaling pathways of PTM signatures
In tumor development, various regulatory factors changed. First, we chose the “limma” package to analyze the DEPs related to PTM score between training and test groups. Based on FDR <0.05 and |log2FC| >0.2, there were 19 up-regulated DEPs and 33 down-regulated DEPs in the training group, and 39 up-regulated DEPs and 43 down-regulated DEPs in the test group (Table S3). The intersection of DEPs is visualized in a Venn diagram (Figure 6A,6B). Enrichment analysis of 26 overlapped DEPs revealed a striking enrichment in the cell cycle process, TP53 transcriptional regulation, and apoptotic signaling pathway (Figure 6C,6D). According to the different levels of related cell cycle protein and histone H3 phosphorylation, high-risk score group had a higher level of cyclin D1, cyclin B1, cyclin E1, and phosphorylated H3 (Figure 6E,6F). GSEA results showed that the high-risk score group had a higher level of KRAS pathway (NES =1.35, P=0.01) and transcriptional activity of the E2Fs family (NES=1.70, P=0.04; NES =1.64, P=0.04; NES =1.69, P=0.01), which could promote the development and progression of tumors. Several miRNAs repressing metabolic reprogramming were inactive in the low-risk score group (NES =1.63, P=0.04; NES=1.66, P=0.04) (Figure 7A-7F). Meantime, gene set variation analysis (GSVA) displayed that the PTM score was statistically correlated with the mitotic spindle and G2/M check point (Figure 7G). These results suggested that PTM signatures may play an essential role in regulating cancer-related processes.
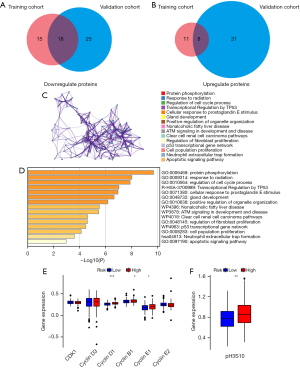
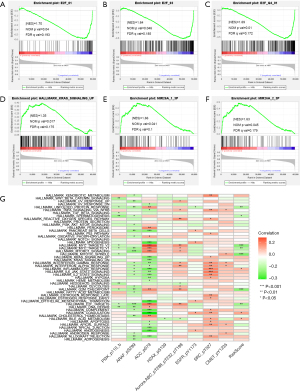
Correlation analysis between the risk model score and immune landscape
The dynamically changing immune microenvironment plays a vital role of tumor development. In order to figure out the correlation between calculated PTM risk score and immune microenvironments, TIMER, QUANTISEQ, and CIBERSORT algorithms were used to analyze immune cell infiltration. Correlation analysis showed that the activation of M2 macrophages was positively correlated with the risk model score (Figure 8A). These results were also verified in the Radar image (Figure 8B). However, no significant correlations were observed in relation to macrophage surface markers in varied risk groups (Figure 8C). Gratifyingly, CCR2, MARCO, CD40, CCL2, CSF1, and FCGR3A, tumor-associated macrophage (TAM) surface markers, presented a significantly positive correlation with the risk model score, indicating that screened PTM signatures promote tumor recruit macrophages (Figure 8D). Immune checkpoint blockade (ICB) has emerged as a promising approach to cancer treatment. To explore the association between immune checkpoints (ICs) and risk score, we compared the expression levels of ICs genes and found that the risk score was closely related to several ICs such as CD27, CD28, CD48, CD200 (Figure 9A). Moreover, even though there was no significance of immune therapy between risk score groups in The Cancer Immune Atlas (TCIA) (Figure 9B). According to the calculated TIDE scores, high-risk group patients possessed stronger immune escape capacity (P=0.001) (Figure 9C). Changes in microsatellite instability (MSI) could have an evident impact on the effectiveness of immunotherapy. As a result of our investigation, we found that microsatellite stability (MSS) was more likely to occur in the low-risk group, and the proportion of MSI-H was significantly higher in the high-risk group than in the low-risk group (24% vs. 18%) (Figure 9D). Hence, PTM risk score may allow the assessment of the tumor immune microenvironment and further ascertain whether immunotherapy can be generally applied in COAD patients.
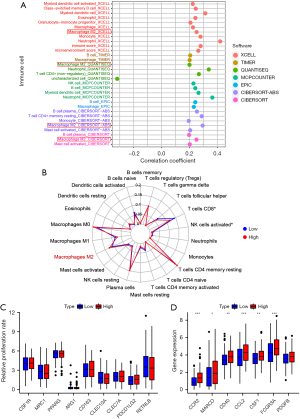
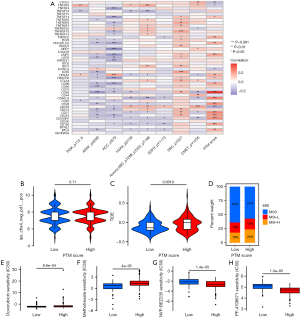
Sensitivity analysis of chemotherapy drugs and inhibitors
Next, predictive effect was observed with PTM risk score on the response to treatment through pRRophetic algorithm. Specifically, the low risk score indicated the decreased half-inhibitory concentration (IC50) of doxorubicin (P=9.6e−04) and methotrexate (P=4e−05) but elevated IC50 of NVP-BEZ235 (P=1.4e−05) and PF-4708671 (P=1.3e−05) (Figure 9E-9H). NVP-BEZ235, a selective and potent dual PI3K-mTOR inhibitor, could suppress the growth of carcinoma and has a synergistic effect with cisplatin. Consequently, this risk-adjusted model could be a feasible predictor of patient sensitivity to chemotherapy drugs and inhibitors.
Alteration of CMET phosphorylated state affected the proliferation and migration of CRC cells in vitro
Cell experiments were performed to validate the function of the selected PTM sites. The CMET_pY1235 site was chosen for verification. We constructed plasmid DNA of CMETY1235E-HA, CMETY1235F-HA, and empty-plasmid transfected into CRC cells (HCT116 and DLD1), and CMET tyrosine residue Y1235 was replaced with either glutamic acid to mimic the phosphorylated state or phenylalanine to mimic the unphosphorylated state. Expression and phosphorylation status of HCT116 (P=0.01) and DLD1 (P=0.006) indicated proteins were monitored by western blotting (Figure 10A). Then, MTT (P=0.001 in HCT116, P=0.007 in DLD1) and colony formation assays (P=0.0007 in HCT116, P=0.0008 in DLD1) illustrated that phosphorylated CMET significantly inhibited the proliferation of CRC cells (Figure 10B,10C). Subsequently, the transwell experiment (P=0.002 in HCT116, P=0.001 in DLD1) and wound-healing assay (P=0.004 in HCT116, P=0.01 in DLD1) demonstrated that the migration of phosphorylated CMET cells was significantly inhibited compared with the control group (Figure 10D,10E). After that, identification of phosphorylated CMET function in cell cycle was conducted by a flow cytometry analysis and we found a cell-cycle arrest at the G0/G1 phase in CMETY1235E group (P=0.0031 in HCT116, P=0.0021 in DLD1) (Figure 11A). Apoptosis flow cytometry depicted that phosphorylated CMET was not involved in the apoptosis regulation process (Figure 11B). In summary, the 1235 site phosphorylation of CMET involved in our PTM prediction model could suppress proliferation, migration and induce cell-cycle arrest of CRC cells.
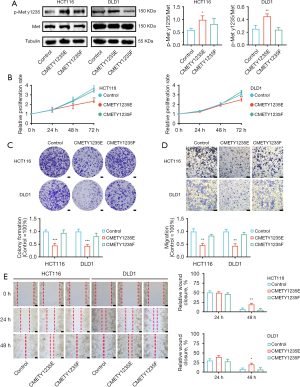
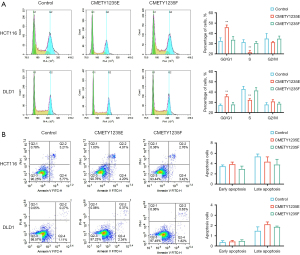
Discussion
By 2040, it is expected that there will be 3 million new cases of CRC and 1.6 million CRC related deaths, making it the leading cause of cancer-related mortality globally (1). There has been an alarming trend towards younger age-of-onset (<50 years old), despite the fact that the incidence and mortality rates have decreased overall (22,23). Therefore, more research into the growth and progression of CRC is necessary. Our understanding of the biology of cancer has improved owing to proteomics techniques, which have also revealed a number of biomarkers with predictive potential for early diagnosis, accurate prognosis, and therapy guidance (5). Increasing evidence points to PTM’s potential to modify particular amino acids to affect proteins’ conformation, activity, interaction, stability, and spatial distribution as a regulatory mechanism for the majority of eukaryotic proteins (24). Through the alteration of protein function, PTM is involved in a variety of intricate and dynamic cellular processes. The association between abnormal PTM events and CRC is being supported by more and more evidence, even though the precise molecular mechanisms and therapeutic targets of PTM in CRC are still unknown (24,25). PTMs come in more than 600 different varieties, including acetylation, glycosylation, ubiquitination, methylation, and citrullination (10). The frequently occurring PTM of phosphorylation, which typically occurs on serine, threonine, or tyrosine residues, acts as a molecular switch for a variety of protein functions and is crucial for signaling pathways (9). The cell cycle, the kinase family of proteins, apoptosis-related proteins, growth factor receptors, and other key biological processes are all closely correlated with abnormal phosphorylation in CRC (6-8). The study proposed that phosphoproteins might be predictive of CRC given these associations. Models for predicting clinical risks can help evaluate clinical decision-making (26,27). It is reasonable and urgent to build a PTM prognostic model in CRC considering the critical roles of PTM in normal physiology and the progression of cancer, as well as the lack of pertinent models.
In this study, we utilized 8 PTM sites to screen prognostic signatures, and constructed a new nomogram that took both the PTM signatures and clinical factors into account. In order to establish a strong correlation between the PTM risk score and the prognosis of CRC, as well as stable predictive performance in the validation dataset, we employed LASSO, univariate Cox regression analysis, and multivariate Cox regression analysis. We investigated various TME performances, evaluated the response of CRC patients to immunotherapy, and assessed the performance of our scoring system. While various machine learning techniques have been applied in previous studies, we selected the LASSO method due to its universality and wide usage across disciplines (28). Robert Tibshirani first introduced the LASSO method in 1996, and it has remained prevalent ever since (28). It is typical for many features with small coefficients that may not be significant to be gathered in high-dimensional datasets. The LASSO method reduces the dimensionality of the data while also achieving more accurate parameter estimation, whereas other algorithms, like ridge regression, may include these unimportant variables (28,29). Our study effectively identified PTM signatures and used a variety of ROC, AUC, DCA, and calibration curves to stratify patient outcomes in the COAD cohort. These analyses in both the training and validation cohorts showed how well our model predicted the outcomes for COAD patients.
Eight phosphorylation sites (ACC_pS79, ARAF_pS299, Aurora.ABC_p288/p232/p198, CMET_pY1235, EGFR_pY1173, SRC_pY527, H2AX_pS139, and PI3K_p110_b) that were most likely to have an impact on the outcomes of TCGA COAD patients were eliminated from further analysis. The biotin-containing enzyme acetyl-CoA carboxylase (ACC) is essential for the production of fatty acids (30). Apoptosis is triggered by the phosphorylation of ACC at Ser79, Ser1200, and Ser1215, which is primarily carried out by AMP-activated kinase (AMPK) (31). In agreement with our discovery that ACC phosphorylation is advantageous for cancer patients, Lally et al. showed that reduced ACC phosphorylation accelerates the development of human liver cancer cells and hepatocellular carcinoma in mice (32). Proto-oncogene A-raf serine/threonine kinase (ARAF) is thought to play a role in cell development and growth. In human breast tumors, Huang et al. discovered an aberrant ARAF pS299 phosphosite, which may serve as “proteomic drivers” of tumorigenesis and potential drug targets (33). CMET, as an oncogenic receptor tyrosine kinase (RTK) needs to be phosphorylated on Tyr1234/5 in order for the kinase to become active. A growing body of research suggests that CMET plays a role in the cellular control, development, and migration of various tumors (34). A crucial part of transcriptional control, DNA repair, DNA replication, and chromosomal stability is played by the DNA damage marker H2AX. According to Akcora-Yildiz et al., elevated H2AX phosphorylation is a sign of greater DNA damage and improved effectiveness (35). A significant portion of human cancers exhibit dysregulation of the RTK known as the epidermal growth factor receptor (EGFR). Numerous pieces of evidence, including various phosphorylated forms, demonstrated that aberrant EGFR activation is a known factor in the development and progression of cancer (36). A better prognosis is indicated by EGFR phosphorylation in our prediction model. Similar to this, Endoh et al. gathered 97 NSCLC patients with tumors that were positive for phospho-EGFR and showed prolonged survival (37). Numerous findings suggest that SRC plays a dual role in activating pro- and antiapoptotic pathways (38). The SRC protein is a tyrosine-protein kinase whose activity can be suppressed by c-SRC kinase phosphorylation. Additionally, Nishida et al. demonstrated that SRC drives cell proliferation and death in a coercively coupled manner via parallel MAPK pathways (39). The serine/threonine kinases Aurora-A, Aurora-B, and Aurora-C are members of the aurora kinase subfamily and are essential regulators of mitosis (40). PI3K_p110_b namely PIK3CB or PI3KB, this gene encodes an isoform of the catalytic subunit of phosphoinositide 3-kinase (PI3K). These kinases are important in signaling pathways involving receptors on the outer membrane of eukaryotic cells and are named for their catalytic subunit (41). Alterations in the PI3K pathway are the most common causes of tumor deterioration, disease progression, and development of treatment resistance (42). Dbouk et al. found PTEN-deficient tumors were found to be reliant on p110_b activity to sustain transformation (43). In several in vitro studies, PI3K_p110_b was shown to play a role in DNA synthesis or cell proliferation, and also promote oncogenic transformation (44,45). All these studies showed that PIK3CB is a pro-cancer gene that harms patient health, consistent with our analysis. The bioinformatics analysis results showed that CMET_pY1235 had the highest risk coefficient and contributed the most to the construction of the prediction model. Thus, we finally chose the CMET_pY1235 site for the wet experiment verification.
A novel model based on gene pairs related to ubiquitin was developed by Liang et al. in a prior study to forecast prognosis and treatment outcomes in CRC, and it demonstrated excellent predictive value (46). In the current study, we sought to assess the correlation between phosphorylation-related protein and COAD patient prognosis. We created a new nomogram that combined the clinical parameters and phosphorylated signatures. As the best tool for predicting outcomes in oncology, a nomogram incorporates potential risk factors and determines the contribution of each risk factor, offering individualized and visual prognostic capabilities for clinical outcomes (47,48). We used ROC curves and calibration curves, following Liang et al., to show that our nomogram had high prognostic accuracy (46). Additionally, DCA was used in the current study to further and thoroughly validate the model, which could determine whether the model is worthwhile to use and has clinical value (49). Overall, our study provided a thorough assessment of the prognostic significance of COAD phosphorylation-related proteins.
In addition, we divided the patients into high- and low-risk groups based on the median PTM score values. Enrichment analyses were carried out to clarify the survival differences between various groups. The KRAS signaling pathway was enriched in the high-risk group, according to GSEA. The high-risk group mainly enriched in cancer-related pathways, such as the cell cycle, TP53 signaling pathway, and higher transcriptional activity of the E2Fs family, in line with prior research (46,50), We also investigated differences in the immune microenvironment between high- and low-risk groups. In contrast to Liang et al.’s research (46), we employed multiple algorithms concurrently so as to ensure the accuracy of the analysis. The immune landscape showed notable differences between the high- and low-risk groups, including IC expression, immune cell activity, varying TIDE scores, and the high-risk group appearing to have an elevated immune escape capacity.
We randomly selected one signature from the model, CMET, and performed cell experiments on it in order to further improve the reliability of our nomogram. The cell viability of CRC cells was reduced and the cell cycle was arrested at the G0/G1 phase as a result of the phosphorylation of CMET at its 1235 site. Multiple cancer entities’ drug resistance, tumor metastasis, and cell proliferation have all been shown to be significantly associated with aberrant activation of CMET signaling (51). Matte et al. discovered, in contrast to our findings, that CMET phosphorylation was connected to Akt and EKR1/2 phosphorylation for promoting ascites-induced ovarian cancer migration (52). The experimental settings or the cancer cell model may be responsible for these variations.
Despite the clinical implications of our results, there are a few restrictions that must be taken into consideration as well. First and foremost, we only constructed training and validation cohorts in the TCGA-COAD database without an external database for the reason of the incompleteness and small scale of phosphorylation protein data in the open public database, which may introduce some biases in the analyzed profile. It is essential to evaluate it regarding other models. Second, the prognostic model developed throughout this study can only be further validated with independent prospective cohorts as our analysis was a retrospective analysis of publicly available datasets. Further research is also indispensable into the purpose and mechanism of those phosphorylation sites.
Conclusions
Based on the TCGA database, we looked into the prognostic value of PTM in COAD patients in this study. We developed a new nomogram that combined phosphorylated signatures and clinical parameters to predict individualized prognosis after opting for the 8 phosphorylation sites that had the strongest impact on patient outcomes. When high- and low-risk groups were divided based on PTM scores, enrichment analyses revealed differences in immune microenvironments and cancer-related pathways. Additionally, using one signature, CMET, we performed cell experiments to show the validity of our nomogram. Our research demonstrates the potential of proteins involved in phosphorylation as trustworthy prognostic markers and potential COAD therapeutic targets. As a retrospective analysis, there are some drawbacks in the present study such as lacking protein data, further analysis of the underlying molecular mechanisms of PTM in COAD is hence required in the future.
Acknowledgments
Funding: This study was supported by
Footnote
Reporting Checklist: The authors have completed the TRIPOD and MDAR reporting checklists. Available at https://jgo.amegroups.com/article/view/10.21037/jgo-24-45/rc
Peer Review File: Available at https://jgo.amegroups.com/article/view/10.21037/jgo-24-45/prf
Conflicts of Interest: All authors have completed the ICMJE uniform disclosure form (available at https://jgo.amegroups.com/article/view/10.21037/jgo-24-45/coif). The authors have no conflicts of interest to declare.
Ethical Statement: The authors are accountable for all aspects of the work in ensuring that questions related to the accuracy or integrity of any part of the work are appropriately investigated and resolved. The study was conducted in accordance with the Declaration of Helsinki (as revised in 2013).
Open Access Statement: This is an Open Access article distributed in accordance with the Creative Commons Attribution-NonCommercial-NoDerivs 4.0 International License (CC BY-NC-ND 4.0), which permits the non-commercial replication and distribution of the article with the strict proviso that no changes or edits are made and the original work is properly cited (including links to both the formal publication through the relevant DOI and the license). See: https://creativecommons.org/licenses/by-nc-nd/4.0/.
References
- Keum N, Giovannucci E. Global burden of colorectal cancer: emerging trends, risk factors and prevention strategies. Nat Rev Gastroenterol Hepatol 2019;16:713-32. [Crossref] [PubMed]
- Liu PH, Wu K, Ng K, et al. Association of Obesity With Risk of Early-Onset Colorectal Cancer Among Women. JAMA Oncol 2019;5:37-44. [Crossref] [PubMed]
- Dienstmann R, Vermeulen L, Guinney J, et al. Consensus molecular subtypes and the evolution of precision medicine in colorectal cancer. Nat Rev Cancer 2017;17:79-92. [Crossref] [PubMed]
- Sirinukunwattana K, Domingo E, Richman SD, et al. Image-based consensus molecular subtype (imCMS) classification of colorectal cancer using deep learning. Gut 2021;70:544-54. [Crossref] [PubMed]
- Zhu G, Jin L, Sun W, et al. Proteomics of post-translational modifications in colorectal cancer: Discovery of new biomarkers. Biochim Biophys Acta Rev Cancer 2022;1877:188735. [Crossref] [PubMed]
- Ali NA, Molloy MP. Quantitative phosphoproteomics of transforming growth factor-β signaling in colon cancer cells. Proteomics 2011;11:3390-401. [Crossref] [PubMed]
- Kim JE, Tannenbaum SR, White FM. Global phosphoproteome of HT-29 human colon adenocarcinoma cells. J Proteome Res 2005;4:1339-46. [Crossref] [PubMed]
- Vlasova-St Louis I, Bohjanen PR. Post-transcriptional regulation of cytokine and growth factor signaling in cancer. Cytokine Growth Factor Rev 2017;33:83-93. [Crossref] [PubMed]
- Paulo JA, Schweppe DK. Advances in quantitative high-throughput phosphoproteomics with sample multiplexing. Proteomics 2021;21:e2000140. [Crossref] [PubMed]
- Wang H, Yang L, Liu M, et al. Protein post-translational modifications in the regulation of cancer hallmarks. Cancer Gene Ther 2023;30:529-47. [Crossref] [PubMed]
- Kim SY, Cho N, Choi Y, et al. Factors Affecting Pathologic Complete Response Following Neoadjuvant Chemotherapy in Breast Cancer: Development and Validation of a Predictive Nomogram. Radiology 2021;299:290-300. [Crossref] [PubMed]
- Balachandran VP, Gonen M, Smith JJ, et al. Nomograms in oncology: more than meets the eye. Lancet Oncol 2015;16:e173-80. [Crossref] [PubMed]
- Mortazavi A, Williams BA, McCue K, et al. Mapping and quantifying mammalian transcriptomes by RNA-Seq. Nat Methods 2008;5:621-8. [Crossref] [PubMed]
- Fan Y, Luo C, Wang Y, et al. A nomogram based on cuproptosis-related genes predicts 7-year relapse-free survival in patients with estrogen receptor-positive early breast cancer. Front Oncol 2023;13:1111480. [Crossref] [PubMed]
- Estimating excess mortality due to the COVID-19 pandemic: a systematic analysis of COVID-19-related mortality, 2020-21. Lancet 2022;399:1513-36. [Crossref] [PubMed]
- Subramanian A, Tamayo P, Mootha VK, et al. Gene set enrichment analysis: a knowledge-based approach for interpreting genome-wide expression profiles. Proc Natl Acad Sci U S A 2005;102:15545-50. [Crossref] [PubMed]
- Smyth GK, Michaud J, Scott HS. Use of within-array replicate spots for assessing differential expression in microarray experiments. Bioinformatics 2005;21:2067-75. [Crossref] [PubMed]
- Chen L, Zhang YH, Lu G, et al. Analysis of cancer-related lncRNAs using gene ontology and KEGG pathways. Artif Intell Med 2017;76:27-36. [Crossref] [PubMed]
- Xu Q, Xu H, Chen S, et al. Immunological Value of Prognostic Signature Based on Cancer Stem Cell Characteristics in Hepatocellular Carcinoma. Front Cell Dev Biol 2021;9:710207. [Crossref] [PubMed]
- Jiang P, Gu S, Pan D, et al. Signatures of T cell dysfunction and exclusion predict cancer immunotherapy response. Nat Med 2018;24:1550-8. [Crossref] [PubMed]
- Geeleher P, Cox N, Huang RS. pRRophetic: an R package for prediction of clinical chemotherapeutic response from tumor gene expression levels. PLoS One 2014;9:e107468. [Crossref] [PubMed]
- Bray F, Ferlay J, Soerjomataram I, et al. Global cancer statistics 2018: GLOBOCAN estimates of incidence and mortality worldwide for 36 cancers in 185 countries. CA Cancer J Clin 2018;68:394-424. [Crossref] [PubMed]
- Gunter MJ, Alhomoud S, Arnold M, et al. Meeting report from the joint IARC-NCI international cancer seminar series: a focus on colorectal cancer. Ann Oncol 2019;30:510-9. [Crossref] [PubMed]
- Hsu CY, Fu SH, Chien MW, et al. Post-Translational Modifications of Transcription Factors Harnessing the Etiology and Pathophysiology in Colonic Diseases. Int J Mol Sci 2020;21:3207. [Crossref] [PubMed]
- Lindhorst PH, Hummon AB. Proteomics of Colorectal Cancer: Tumors, Organoids, and Cell Cultures-A Minireview. Front Mol Biosci 2020;7:604492. [Crossref] [PubMed]
- Usher-Smith JA, Walter FM, Emery JD, et al. Risk Prediction Models for Colorectal Cancer: A Systematic Review. Cancer Prev Res (Phila) 2016;9:13-26. [Crossref] [PubMed]
- Xu W, He Y, Wang Y, et al. Risk factors and risk prediction models for colorectal cancer metastasis and recurrence: an umbrella review of systematic reviews and meta-analyses of observational studies. BMC Med 2020;18:172. [Crossref] [PubMed]
- Tibshirani R. The lasso method for variable selection in the Cox model. Stat Med 1997;16:385-95. [Crossref] [PubMed]
- Wang W, Liu W. PCLasso: a protein complex-based, group lasso-Cox model for accurate prognosis and risk protein complex discovery. Brief Bioinform 2021;22:bbab212. [Crossref] [PubMed]
- Xiang X, Saha AK, Wen R, et al. AMP-activated protein kinase activators can inhibit the growth of prostate cancer cells by multiple mechanisms. Biochem Biophys Res Commun 2004;321:161-7. [Crossref] [PubMed]
- Park SH, Gammon SR, Knippers JD, et al. Phosphorylation-activity relationships of AMPK and acetyl-CoA carboxylase in muscle. J Appl Physiol (1985) 2002;92:2475-82. [PubMed]
- Lally JSV, Ghoshal S, DePeralta DK, et al. Inhibition of Acetyl-CoA Carboxylase by Phosphorylation or the Inhibitor ND-654 Suppresses Lipogenesis and Hepatocellular Carcinoma. Cell Metab 2019;29:174-182.e5. [Crossref] [PubMed]
- Huang KL, Li S, Mertins P, et al. Proteogenomic integration reveals therapeutic targets in breast cancer xenografts. Nat Commun 2017;8:14864. [Crossref] [PubMed]
- Li D, Yang J, Xu Z, et al. c-Met-Targeting (19)F MRI Nanoparticles with Ultralong Tumor Retention for Precisely Detecting Small or Ill-Defined Colorectal Liver Metastases. Int J Nanomedicine 2023;18:2181-96. [Crossref] [PubMed]
- Akcora-Yildiz D, Gonulkirmaz N, Ozkan T, et al. HIV-1 integrase inhibitor raltegravir promotes DNA damage-induced apoptosis in multiple myeloma. Chem Biol Drug Des 2023;102:262-70. [Crossref] [PubMed]
- Kumagai S, Koyama S, Nishikawa H. Antitumour immunity regulated by aberrant ERBB family signalling. Nat Rev Cancer 2021;21:181-97. [Crossref] [PubMed]
- Endoh H, Ishibashi Y, Yamaki E, et al. Immunohistochemical analysis of phosphorylated epidermal growth factor receptor might provide a surrogate marker of EGFR mutation. Lung Cancer 2009;63:241-6. [Crossref] [PubMed]
- Pedraza LG, Stewart RA, Li DM, et al. Drosophila Src-family kinases function with Csk to regulate cell proliferation and apoptosis. Oncogene 2004;23:4754-62. [Crossref] [PubMed]
- Nishida H, Okada M, Yang L, et al. Methionine restriction breaks obligatory coupling of cell proliferation and death by an oncogene Src in Drosophila. Elife 2021;10:e59809. [Crossref] [PubMed]
- Fatma H, Siddique HR. AURORA KINASE A and related downstream molecules: A potential network for cancer therapy. Adv Protein Chem Struct Biol 2023;134:115-45. [Crossref] [PubMed]
- Fan K, Hu Q, Yu S, et al. SP1 Mediated PIK3CB Upregulation Promotes Gastric Carcinogenesis. J Cancer 2024;15:1355-65. [Crossref] [PubMed]
- Li A, Schleicher SM, Andre F, et al. Genomic Alteration in Metastatic Breast Cancer and Its Treatment. Am Soc Clin Oncol Educ Book 2020;40:1-14. [Crossref] [PubMed]
- Dbouk HA, Vadas O, Shymanets A, et al. G protein-coupled receptor-mediated activation of p110β by Gβγ is required for cellular transformation and invasiveness. Sci Signal 2012;5:ra89. [Crossref] [PubMed]
- Karlsson T, Krakstad C, Tangen IL, et al. Endometrial cancer cells exhibit high expression of p110β and its selective inhibition induces variable responses on PI3K signaling, cell survival and proliferation. Oncotarget 2017;8:3881-94. [Crossref] [PubMed]
- Suh KJ, Ryu MH, Zang DY, et al. AZD8186 in Combination With Paclitaxel in Patients With Advanced Gastric Cancer: Results From a Phase Ib/II Study (KCSG ST18-20). Oncologist 2023;28:e823-34. [Crossref] [PubMed]
- Liang L, Liu L, Mai S, et al. A novel machine learning model based on ubiquitin-related gene pairs and clinical features to predict prognosis and treatment effect in colon adenocarcinoma. Eur J Med Res 2023;28:41. [Crossref] [PubMed]
- Liu Y, Wu L, Ao H, et al. Prognostic implications of autophagy-associated gene signatures in non-small cell lung cancer. Aging (Albany NY) 2019;11:11440-62. [Crossref] [PubMed]
- Iasonos A, Schrag D, Raj GV, et al. How to build and interpret a nomogram for cancer prognosis. J Clin Oncol 2008;26:1364-70. [Crossref] [PubMed]
- Zhang B, Liu Q, Zhang X, et al. Clinical Utility of a Nomogram for Predicting 30-Days Poor Outcome in Hospitalized Patients With COVID-19: Multicenter External Validation and Decision Curve Analysis. Front Med (Lausanne) 2020;7:590460. [Crossref] [PubMed]
- Zhang D, Wang T, Zhou Y, et al. Comprehensive analyses of cuproptosis-related gene CDKN2A on prognosis and immunologic therapy in human tumors. Medicine (Baltimore) 2023;102:e33468. [Crossref] [PubMed]
- Bradley CA, Salto-Tellez M, Laurent-Puig P, et al. Targeting c-MET in gastrointestinal tumours: rationale, opportunities and challenges. Nat Rev Clin Oncol 2017;14:562-76. [Crossref] [PubMed]
- Matte I, Lane D, Laplante C, et al. Ovarian cancer ascites enhance the migration of patient-derived peritoneal mesothelial cells via cMet pathway through HGF-dependent and -independent mechanisms. Int J Cancer 2015;137:289-98. [Crossref] [PubMed]