Identification of a lactylation-related gene signature to characterize subtypes of hepatocellular carcinoma using bulk sequencing data
Highlight box
Key findings
• A close relationship exists between lactylation-related genes (LRGs) and tumor classification and immunity in hepatocellular carcinoma (HCC) patients. LRG signatures are good prognostic indicators for HCC.
What is known and what is new?
• The LRGs is essential for tumor classification and immunity of HCC.
• The LRGs-related biomarker candidates were identified.
What is the implication, and what should change now?
• The findings of our study may extend understandings of the diagnosis and treatment of HCC.
Introduction
Liver cancer is the sixth most common primary tumor site in humans globally and the fourth leading cause of cancer-related mortality (1). Approximately 90% of liver cancers are attributed to hepatocellular carcinoma (HCC) (2). The 5-year survival rate of HCC patients is low typically ranging from 18% to 30%, due to the high incidence of disease recurrence and metastasis (3). Approximately half of HCC patients receive systemic therapies, such as sorafenib or lenvatinib, as the first-line treatment (4).
The treatment of HCC has garnered significant attention in recent years, primarily due to the revolutionary impact of immune checkpoint inhibitors (ICIs) on cancer treatment (4,5). Despite significant progress in treatment of HCC, there remains a requirement for enhanced and efficacious novel biomarkers, given their pivotal role in the development and progression of HCC.
A key characteristic of cancer is the increase in extracellular acidity caused by the accumulation of lactate (6). Lactate was previously considered a mere metabolic waste product (6). However, emerging evidence indicates that lactate is a crucial metabolite in the tumor microenvironment (7). It serves as a fuel for mitochondrial metabolism, plays a vital role in modulating immune cell function, and thought to be carefully considered in cancer immunotherapy (6,7).
Lactate can regulate immune cell metabolism and suppress the activation and proliferation of immune cells (7). Lactate has been recognized as a biomarker in various diseases, including neoplastic, inflammatory, and autoimmune diseases (7). Recent evidence suggests that lactate, when updated, can function as an energy source, a signaling molecule, and an immunoregulatory molecule (7). Specifically, lactate plays vital roles in regulating metabolic pathways, tumor angiogenesis, immune response, and cell-to-cell communication within the tumor microenvironment (7). Also, it has been hypothesized that lactate regulates gene expression through a new post-translational modification of protein lactylation (8). Histone lysine lactylation can induce the polarization of macrophages toward a M2-like phenotype, resulting in the suppression of immune responses in the tumor microenvironment (9). The lactylation of proteins has not only opened up new research fields in post-translational modification, but has also led to new directions in the study of immunity, cancer, and other topics related to lactate (7-9). However, understandings of the interactions among protein lactylation and immunosuppression in HCC are limited. Therefore, these relationships need to be examined further to improve the treatment of HCC.
Tumor classifications of HCC based on genomic, epigenomic, or proteomic alterations have identified unique tumor subgroups linked to clinical outcomes (10-13). Chen et al. categorized patients into two groups based on mitophagy-related genes in HCC (12). Liang et al. identified novel ferroptosis-related gene signature for HCC (13). Numerous influential studies have shown that these classifications can greatly enhance patient outcomes by allowing personalized therapy based on specific cancer cell alterations (10,11).
The critical role of lactic acid in tumor development and anti-tumor processes is widely acknowledged (7-9). However, its specific function in HCC remains poorly understood. The aim of this study was to assess lactylation-related gene (LRG) expression levels in HCC, explore the correlation between lactate and the tumor immune microenvironment, and evaluate its prognostic significance. We present this article in accordance with the TRIPOD reporting checklist (available at https://jgo.amegroups.com/article/view/10.21037/jgo-24-405/rc).
Methods
Acquisition of HCC data from The Cancer Genome Atlas (TCGA)
The RNA sequencing data and relative clinical data of 371 patients with HCC were retrieved from the TCGA database (https://portal.gdc.cancer.gov/projects/TCGA-LIHC). A total of 325 LRGs were obtained from a previous study (14). TCGA-HCC patients were analyzed using a Cox univariate analysis to identify the prognostic genes and to discover their functions. The study was conducted in accordance with the Declaration of Helsinki (as revised in 2013).
Identification of prognostic HCC-related genes
Using a univariate Cox analysis, the prognostic genes were identified. The R package venn (v. 1.11; The R Foundation for Statistical Computing, Vienna, Austria) was used to construct the Venn diagram. The “clusterProfiler” package in R was used to conduct the Gene Ontology (GO) and Kyoto Encyclopedia of Genes and Genomes (KEGG) pathway enrichment analyses to identify the signaling pathways related to the prognostic genes in HCC. The Search Tool for the Retrieval of Interacting Genes/Proteins (STRING) website (https://string-db.org/) and the “clusterProfiler” package were used to build a protein-protein interaction (PPI) network using the LRGs of HCC. Cytoscape was used to visualize the PPI network. P values of <0.05 were considered statistically significant throughout the study.
Consensus clustering analysis of the LRGs in HCC
The correlations between the LRGs and HCC subtypes were examined. The “ConsensusClusterPlus” package (version 1.54.0) was used to perform the consistent cluster, cumulative distribution function (CDF), and consensus matrix analyses of the TCGA-HCC cohort. The value of the clustering variable (k) ranged from 2 to 6. A heatmap was generated using the “Pheatmap” package (version 1.0.12). The “GGalluvial” R package was used to investigate the correlation among types, overall survival (OS) status, and risk score.
Identifying lactylation-related differentially expressed genes (DEGs) in HCC
The “DEseq2” package in R was used to identify the DEGs between the HCC clusters. A P value <0.05 and |log2fold change| >1 indicated a statistically significant difference. A volcano plot was generated using the “ggplot2” R package to visualize the DEGs in HCC. The DEG heatmap was generated using the R package “pheatmap” (version 1.0.12). The “clusterProfiler” package in R was used to conduct the GO and KEGG pathway enrichment analyses.
Detection of immune activity in two lactylation-related clusters of HCC
“Immunoeconomics” was used to assess the immune function of LRGs. The immune activity of two lactylation-related clusters was compared by analyzing the expression of the eight immune checkpoint genes (i.e., CD274, PDCD1, PDCD1LG2, CTLA4, LAG3, HAVCR2, TIGIT, and SIGLEC15). Heatmaps and box plots were created individually using R packages “pheatmap” and “ggplot2”. The Wilcoxon test was used to measure the infiltration of immune cells and activated immune pathways between the two groups. P values of <0.05 were considered statistically significant.
Development of a prognostic model based on LRGs
The “glmnet” R package was used to perform a Cox regression analysis to evaluate the prognostic significance of the LRGs in the TCGA-HCC cohort. Initially, a multi-factor Cox regression was employed for the data analysis, after which iterative optimization was performed using the “step” function. Ultimately, the optimal model was chosen as the final model. The minimum criteria were used to determine the conditions for variables that had coefficients that were not zero. A risk score was calculated by adding up all the expression levels for each gene and multiplying them by the corresponding coefficients. Low-risk (G1) and high-risk (G2) subgroups were established based on the median risk scores of each TCGA-HCC patient. The “GGalluvial” R package was used to investigate the correlation among types, OS status, and risk score.
Statistical analysis
Survival analysis was conducted to identify independent prognostic factors for HCC, with a significance level set at P<0.05. Adjusted for multiple testing, bilateral P values <0.05 were deemed statistically significant.
Results
Identification of key prognostic LRGs for HCC
A total of 4,378 prognostic genes were identified; the top 20 prognostic genes, as determined by their P values, are displayed in a forest plot (Figure 1A,1B). Prognostic genes may be closely related to the occurrence of cancer, so we identified LRGs associated with prognosis. Using the Venn diagram was used to identify the prognostic LRGs, and ultimately 148 prognostic LGRs were discovered (Figure 1B). The results of the GO analysis of the biological processes revealed that the prognostic LRGs were associated with gene expression, nucleic acid metabolism, RNA metabolism, RNA splicing, and cellular macromolecule metabolism based on P value <0.05 (Figure 1C). The KEGG enrichment analysis revealed that the pathways of these key LRGs may significantly contribute to HCC through amino acid biosynthesis, carbon metabolism, the pentose phosphate pathway, glycolysis/gluconeogenesis, fructose and mannose metabolism, spliceosome, and the messenger RNA surveillance pathway (Figure 1D). Further, the selection of these prognostic LRGs was validated by a PPI network analysis (Figure 1D). Ultimately, the following 20 key LRGs: ACIN1, RAN, PPP1CB, ALDOB, SUMO2, THOC2, HDAC1, SF3A1, SF3B1, HNRNPM, PPP1CC, SRRM1, PRPF6, HDAC2, H2AFV, ALYREF, H2AFZ, H2AFX, HNRNPK, and MAGOH (Figure 1E) we identified.
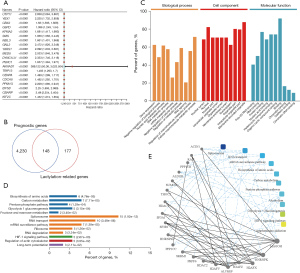
HCC subtypes based on the key LRGs
Lactylation is closely associated with tumor development (6-9); however, the specific role of LRGs in HCC has yet to be thoroughly investigated. Consistency clustering and a principal component analysis (PCA) indicated that TCGA-HCC patients were successfully categorized into two clusters using a clustering variable (k) of 2 (Figure 2A,2B). Through a consensus clustering analysis, we categorized TCGA-HCC patients into distinct subtypes using the 20 LRGs (Figure 2A). The heatmap of the LRGs in TCGA-HCC patients demonstrated a clear separation between the two groups (Figure 2C). As Figure 2D shows, the OS rate of the G1 group was statistically significantly higher than that of the G2 group [hazard ratio (HR): 2.025; 95% confidence interval (CI): 1.418–2.891; P=0.000103].
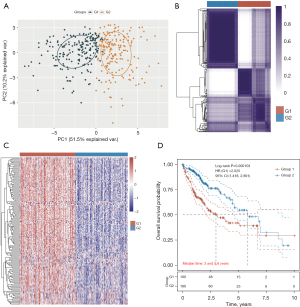
Mechanisms underlying the differences between the two types of HCC
The DEGs between the two groups were identified using a volcano plot (P value <0.05; |log2fold change| >1). Compared with the G2 group, 986 genes were upregulated in G1 and 123 genes were downregulated. In the heatmap, the top 50 DEGs were differently expressed in the two groups.
The biological functions of the 1,109 DEGs were elucidated through KEGG and GO enrichment analyses. The KEGG analysis revealed that the upregulated genes primarily participate in the p53 signaling pathway, regulation of actin cytoskeleton, phagosome, nucleocytoplasmic transport, focal adhesion, extracellular matrix (ECM)-receptor interaction, and cell cycle (Figure 3A), while the downregulated genes primarily participate in the PPAR signaling pathway, glycolysis/gluconeogenesis, carbon metabolism, and biosynthesis of amino acids (Figure 3B).
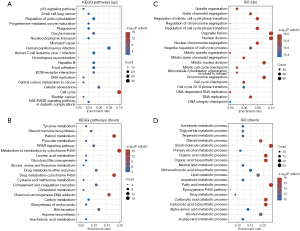
The GO analysis of the biological processes revealed that the upregulated genes primarily participate in spindle organization, mitotic cell cycle phase transition, nuclear division, and DNA replication (Figure 3C), while the downregulated genes primarily participate in the metabolic process, organic acid biosynthetic process, epoxygenase P450 pathway, and carboxylic acid catabolic process (Figure 3D). Previous research has demonstrated that the p53 signaling pathway, focal adhesion, ECM-receptor interaction, and cell cycle can serve as tumor markers (15).
Immune activity in the two lactylation-related groups in HCC
There is a strong correlation between lactylation and immune activity in many types of cancer (7-9). In this study, the immune response in two lactylation-related patient clusters with HCC was assessed. The box plots showed a significant difference in the immune cell populations, with a higher abundance of B cells, CD4+ T cells, CD8+ T cells, neutrophils, macrophages, and myeloid dendritic cells in the G1 HCC samples than the G2 HCC samples (Figure 4A). Further, the box plots showed higher expression levels of seven of the eight ICI-related genes (i.e., CD274, CTLA4, HAVCR2, LAG3, PDCD1, PDCD1LG2, and TIGIT) in the G1 HCC samples than the G2 samples (Figure 4B). According to the results, there is a close relationship between lactylation and immune function.
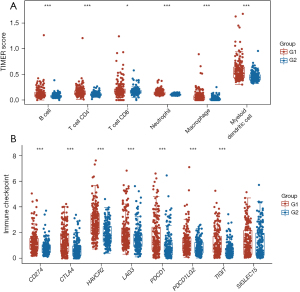
The tumor stemness in the two lactylation-related groups in HCC
Cancer stem cells (CSCs) are crucial for tumor initiation, recurrence, spread, and resistance to chemotherapy. There was a significant disparity in the CSC scores between the G1 and G2 TCGA-HCC patients (Figure 5A). Based on these findings, LRGs-related classification could serve as a reliable predictor of immune checkpoint blockade (ICB) therapy responses.
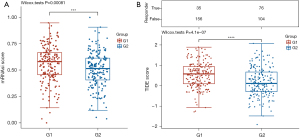
A low survival rate was observed following ICB treatment in cases in which the tumor immune dysfunction and exclusion (TIDE) score was elevated. The G1 TCGA-HCC patients had a higher TIDE score than the G2 TCGA-HCC patients (Figure 5B), indicating an insufficient response to ICB therapy and a bleak prognosis.
Development of a prognostic model based on LRGs
A total of 20 LRGs in HCC were identified through the STEP regression analyses (Figure 6A). A six-gene signature was developed using the optimal λ value (Figure 6A). Two risk groups, low and high, were identified in TCGA-HCC patients using this gene signature (Figure 6A). Patients with a G1 score exhibited significantly higher OS rates than those with a G2 score (HR: 2.539; 95% CI: 1.764–3.657; P=5.45e−07; Figure 6B). The prognostic models were evaluated by calculating the area under the receiver operating characteristic curve (AUC). As Figure 6C shows, the AUCs at 1, 3, and 5 years were 0.723, 0.731, and 0.750, which suggests that this model effectively predicted outcomes.
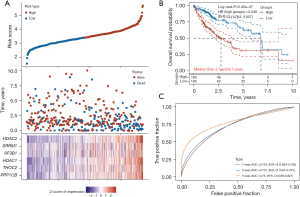
Discussion
In this study, 4,378 genes associated with prognosis were identified, and 20 LRGs (i.e., ACIN1, RAN, PPP1CB, ALDOB, SUMO2, THOC2, HDAC1, SF3A1, SF3B1, HNRNPM, PPP1CC, SRRM1, PRPF6, HDAC2, H2AFV, ALYREF, H2AFZ, H2AFX, HNRNPK, and MAGOH) were identified in HCC. The 20 LRGs were used to divide TCGA-HCC patients into G1 and G2 groups. The KEGG analysis revealed that the upregulated genes in the G1 patients primarily participate in the p53 signaling pathway, regulation of actin cytoskeleton, phagosome, nucleocytoplasmic transport, focal adhesion, ECM-receptor interaction, and cell cycle, while the downregulated genes primarily participate in the PPAR signaling pathway, glycolysis/gluconeogenesis, carbon metabolism, and biosynthesis of amino acids. A significant difference in the immune cell populations, with a higher abundance of B cells, CD4+ T cells, CD8+ T cells, neutrophils, macrophages, and myeloid dendritic cells, was observed between the G1 and G2 HCC samples. Further, seven of the eight ICI-related genes were more highly expressed in the G1 HCC samples than the G2 HCC samples. There was a significant difference in the CSCs scores between the G1 and G2 TCGA-HCC patients. The G1 TCGA-HCC patients had higher TIDE scores than the G2 TCGA-HCC patients. The prognosis of the HCC patients was predicted using a seven-LRG model, comprising CXCR4, AADAC, SLC2A3, CMTM3, RGS2, CD59, and ZEB2.
Researchers have identified lactylatean important contributor to inflammation, fibrosis, and oncogenic processes (7-9). For instance, lactylate accumulates in the outer regions of tumor tissues, leading to the release of vascular endothelial growth factor, which in turn promotes angiogenesis and enhances the motility of cancer cells (16). Further, T-cell-mediated immunity is impaired by extracellular acidosis, and neutralizing tumor acidity may boost the anti-tumor effect of immunotherapy (17). The correlation between lactylation and HCC remains largely unexplored.
In this study, 20 LRGs were used to divide TCGA-HCC patients into two groups of G2 and G1 patients. The G1 subgroup had a higher abundance of B cells, CD4+ T cells, CD8+ T cells, neutrophils, macrophages, and myeloid dendritic cells than the G2 HCC subgroup. Recent evidence suggests that lactate can regulate immune cell metabolism and suppress the activation and proliferation of immune cells (7). Lactate has been recognized as a biomarker in various diseases, including neoplastic, inflammatory, and autoimmune diseases (7). The 20 LRGs might be closely related to the functions of immune cells obtained by functional enrichment analyses. The G1 TCGA-HCC patients had higher TIDE scores than the G2 TCGA-HCC patients. A classification of HCC tumors by LRGs could be established based on these results. LRG expression was positively correlated with the prognosis of HCC patients, and a prognostic model comprising six molecules (i.e., HDAC1, HDAC2, SRRM1, SF3B1, THOC2, and PPP1CB) was developed.
Inhibiting HDAC1 improved the efficacy of chemotherapy in ovarian cancer (18). HDAC2 has been recognized as a target for anti-cancer therapeutics (19). SRRM1 enhances the growth, movement, and spread of HCC cells through modulation of the JAK/STAT signaling pathway (20). SF3B1 is upregulated and plays a role in the aggressiveness and prognosis of HCC (21). A genetic variant in the PPP1CB gene is associated with the risk of hepatitis B virus-related HCC (22).
It should be noted that this study had some limitations. In vitro and in vivo examinations of these seven LRGs need to be conducted to confirm these findings. The related molecular mechanisms should also be examined in future research.
Conclusions
In conclusion, a close relationship exists between LRGs and tumor classification and immunity in patients with HCC. LRG signatures are good prognostic indicators for HCC. The findings of our study extend understandings of the diagnosis and treatment of HCC.
Acknowledgments
Funding: None.
Footnote
Reporting Checklist: The authors have completed the TRIPOD reporting checklist. Available at https://jgo.amegroups.com/article/view/10.21037/jgo-24-405/rc
Peer Review File: Available at https://jgo.amegroups.com/article/view/10.21037/jgo-24-405/prf
Conflicts of Interest: All authors have completed the ICMJE uniform disclosure form (available at https://jgo.amegroups.com/article/view/10.21037/jgo-24-405/coif). The authors have no conflicts of interest to declare.
Ethical Statement: The authors are accountable for all aspects of the work in ensuring that questions related to the accuracy or integrity of any part of the work are appropriately investigated and resolved. The study was conducted in accordance with the Declaration of Helsinki (as revised in 2013).
Open Access Statement: This is an Open Access article distributed in accordance with the Creative Commons Attribution-NonCommercial-NoDerivs 4.0 International License (CC BY-NC-ND 4.0), which permits the non-commercial replication and distribution of the article with the strict proviso that no changes or edits are made and the original work is properly cited (including links to both the formal publication through the relevant DOI and the license). See: https://creativecommons.org/licenses/by-nc-nd/4.0/.
References
- Li X, Ramadori P, Pfister D, et al. The immunological and metabolic landscape in primary and metastatic liver cancer. Nat Rev Cancer 2021;21:541-57. [Crossref] [PubMed]
- Galle PR. Treating Hepatobiliary Cancers: The Oncology Way. Dig Dis 2017;35:384-6. [Crossref] [PubMed]
- Chidambaranathan-Reghupaty S, Fisher PB, Sarkar D. Hepatocellular carcinoma (HCC): Epidemiology, etiology and molecular classification. Adv Cancer Res 2021;149:1-61. [Crossref] [PubMed]
- Llovet JM, Castet F, Heikenwalder M, et al. Immunotherapies for hepatocellular carcinoma. Nat Rev Clin Oncol 2022;19:151-72. [Crossref] [PubMed]
- Harkus U, Wankell M, Palamuthusingam P, et al. Immune checkpoint inhibitors in HCC: Cellular, molecular and systemic data. Semin Cancer Biol 2022;86:799-815. [Crossref] [PubMed]
- Lv X, Lv Y, Dai X. Lactate, histone lactylation and cancer hallmarks. Expert Rev Mol Med 2023;25:e7. [Crossref] [PubMed]
- Liu X, Zhang Y, Li W, et al. Lactylation, an emerging hallmark of metabolic reprogramming: Current progress and open challenges. Front Cell Dev Biol 2022;10:972020. [Crossref] [PubMed]
- Chen L, Huang L, Gu Y, et al. Lactate-Lactylation Hands between Metabolic Reprogramming and Immunosuppression. Int J Mol Sci 2022;23:11943. [Crossref] [PubMed]
- Liberti MV, Locasale JW. Histone Lactylation: A New Role for Glucose Metabolism. Trends Biochem Sci 2020;45:179-82. [Crossref] [PubMed]
- Moldogazieva NT, Zavadskiy SP, Terentiev AA. Genomic Landscape of Liquid Biopsy for Hepatocellular Carcinoma Personalized Medicine. Cancer Genomics Proteomics 2021;18:369-83. [Crossref] [PubMed]
- Yim SY, Lee JS. An Overview of the Genomic Characterization of Hepatocellular Carcinoma. J Hepatocell Carcinoma 2021;8:1077-88. [Crossref] [PubMed]
- Chen H, Wang J, Zeng R, et al. Development and Validation of a Novel Mitophagy-Related Gene Prognostic Signature for Hepatocellular Carcinoma Based on Immunoscore Classification of Tumor. J Oncol 2021;2021:5070099. [Crossref] [PubMed]
- Liang JY, Wang DS, Lin HC, et al. A Novel Ferroptosis-related Gene Signature for Overall Survival Prediction in Patients with Hepatocellular Carcinoma. Int J Biol Sci 2020;16:2430-41. [Crossref] [PubMed]
- Cheng Z, Huang H, Li M, et al. Lactylation-Related Gene Signature Effectively Predicts Prognosis and Treatment Responsiveness in Hepatocellular Carcinoma. Pharmaceuticals (Basel) 2023;16:644. [Crossref] [PubMed]
- Senga SS, Grose RP. Hallmarks of cancer-the new testament. Open Biol 2021;11:200358. [Crossref] [PubMed]
- Chen AN, Luo Y, Yang YH, et al. Lactylation, a Novel Metabolic Reprogramming Code: Current Status and Prospects. Front Immunol 2021;12:688910. [Crossref] [PubMed]
- Erra Díaz F, Dantas E, Geffner J. Unravelling the Interplay between Extracellular Acidosis and Immune Cells. Mediators Inflamm 2018;2018:1218297. [Crossref] [PubMed]
- Liu X, Yu Y, Zhang J, et al. HDAC1 Silencing in Ovarian Cancer Enhances the Chemotherapy Response. Cell Physiol Biochem 2018;48:1505-18. [Crossref] [PubMed]
- Jo H, Shim K, Kim HU, et al. HDAC2 as a target for developing anti-cancer drugs. Comput Struct Biotechnol J 2023;21:2048-57. [Crossref] [PubMed]
- Song X, Ma J. SRRM1 promotes the proliferation, migration, and invasion of hepatocellular carcinoma cells by regulating the JAK/STAT signaling pathway. Tissue Cell 2022;79:101954. [Crossref] [PubMed]
- López-Cánovas JL, Del Rio-Moreno M, García-Fernandez H, et al. Splicing factor SF3B1 is overexpressed and implicated in the aggressiveness and survival of hepatocellular carcinoma. Cancer Lett 2021;496:72-83. [Crossref] [PubMed]
- Mai H, Xie H, Hou J, et al. A Genetic Variant of PPP1CB Influences Risk of Hepatitis B Virus-Related Hepatocellular Carcinoma in Han Chinese: A Pathway Based Analysis. J Hepatocell Carcinoma 2021;8:1055-64. [Crossref] [PubMed]