Identification of a prognostic disulfidptosis-related gene signature in hepatocellular cancer
Highlight box
Key findings
• Disulfidptosis has been discovered to regulate various biological processes in hepatocellular carcinoma (HCC). Disulfidptosis-related genes are good prognostic indicators for HCC.
What is known and what is new?
• The disulfidptosis-related genes are essential for evaluation of HCC.
• The disulfidptosis-related subtypes and disulfidptosis-related biomarkers were identified for HCC.
What is the implication, and what should change now?
• The findings could provide insights into how HCC is diagnosed and treated in the future.
Introduction
Hepatocellular carcinoma (HCC) is common and one of the leading causes of cancer-related death (1-3). Multiple factors are involved in HCC development including an imbalance of inactivation and activation of tumor suppressor genes, disturbed molecular pathways, and upregulation of angiogenesis.
In 2020, there were 905,000 reported cases of cancer and 830,000 recorded deaths due to the disease globally (1). By 2024, it is anticipated that there will be a greater than 55% increase in the number of new cancer cases and deaths (1). Diagnosis, treatment, and prognosis hinge primarily on the degree of liver dysfunction and pathological examination (2). Bioinformatics has facilitated the utilization of diverse biomarkers as adjuncts in tumor detection and response to treatment (3,4). To improve outcome in these patients, it is essential to discover novel biomarkers that can be utilized as targets for diagnosing and treating HCC patients.
The concept of disulfidptosis has been proposed in a recent study (5). Disulfidptosis is triggered by an excess of cystine in cells, causing disulfide stress, in contrast to other cell death pathways such as apoptosis, ferroptosis, and necroptosis (5). As a result of glucose starvation, rapid cell death occurs. Cancer therapy can induce cell death by activating BAX and BAK in the cancer cells, or by inducing apoptosis (6). Cell death mechanisms are therefore of great importance in treating cancer patients. The development of new biomarkers for tumors has been the subject of numerous studies investigating different mechanisms of cell death, such as apoptosis, pyroptosis, ferroptosis, and cuproptosis (7,8). For example, scientists used ferroptosis-related regulatory factors to estimate survival of HCC patients, and distinguished various molecular subtypes of HCC by employing these genes (8).
Despite the significance of disulfidptosis in HCC, there is a scarcity of research on this subject. We performed a literature search for genes that are associated with disulfidptosis, analyzed them, and investigated the underlying mechanisms. We present this article in accordance with the TRIPOD reporting checklist (available at https://jgo.amegroups.com/article/view/10.21037/jgo-24-522/rc).
Methods
Data source of HCC
The Cancer Genome Atlas (TCGA)-HCC dataset, comprising RNA sequencing data and clinical information, was obtained from the TCGA database, which includes a total of 371 samples. The TCGA data were obtained from TCGA counts, converted to transcripts per million (TPM) formats, and then normalized to log2(TPM +1). The disulfidptosis-related genes were obtained from a previous study (9). The study was conducted in accordance with the Declaration of Helsinki (as revised in 2013).
Identification of prognostic disulfidptosis-related genes in HCC
Through univariate Cox analysis, the prognostic genes were discovered to exhibit a P value less than 0.05 in HCC patients. The Venn diagram was constructed using the online Venn tools (http://bioinformatics.psb.ugent.be/webtools/Venn/). The “clusterProfiler” package in R was utilized to perform enrichment analyses for Gene Ontology (GO) and Kyoto Encyclopedia of Genes and Genomes (KEGG) pathways, identifying the signaling pathways associated with prognostic genes in HCC.
HCC subtypes of disulfidptosis-related genes
Consensus clustering analysis was used to categorize TCGA-HCC patients according to their prognostic disulfide-related genes using “ConsensusClusterPlus” package (version 1.54.0). After defining the clustering variable (kappa) as 2, the TCGA-HCC patients were divided into two subtypes (high-risk and low-risk groups). The heatmap was created with the “Pheatmap” package (version 1.0.12). We used the “GGalluvial” R package to investigate the relationship between tumor types, overall survival (OS) status, and risk score.
Differential gene analysis
DEGs between high- and low-risk groups in HCC were identified using R’s “DEseq2” package. DEGs were chosen using the P value <0.05 and |log2fold change| >1. The heatmap of DEGs was created using the R package “pheatmap” (version 1.0.12). The volcano plots of DEGs were created using the ggplot2. GO and KEGG analysis were employed to investigate the biological functions of DEGs using the “clusterProfiler” package.
Analysis on immune infiltration between the high- and low-risk groups
In this study, we used “immunoeconomics” to assess the immune function of HCC subtypes of disulfidptosis-related genes. The immune activity of two HCC subtypes was compared based on the expression of eight immune checkpoint genes, including CD274, CTLA4, HAVCR2, IGSF8, ITPRIPL1, LAG3, PDCD1, PDCD1LG2, SIGLEC15, and TIGIT. The heatmap of DEGs was created using the R package “pheatmap” (version 1.0.12). A volcano plot of DEGs was created using the ggplot2. The Wilcoxon test was applied to assess the infiltration of immune cells between the two groups using the P value <0.05.
Construction of a prognostic model based on disulfidptosis-related genes
We screened for genes associated with disulfideptosis using R’s glmnet package using least absolute shrinkage and selection operator (LASSO) regression analysis. Then, multivariate Cox regression was conducted using genes with non-zero penalty coefficients. To calculate the prognostic model regression coefficients, multivariate Cox regression analysis was performed. The risk score for TCGA-HCC patients was calculated, and the median risk score was used as the cutoff to classify TCGA-HCC patients into low- and high-risk groups. The “GGalluvial” R package was utilized to explore the correlation between different types, OS status, and risk scores.
Statistical analysis
A survival analysis was performed to ascertain independent prognostic factors for HCC, with statistical significance established at a P value of less than 0.05. After adjusting for multiple comparisons, bilateral P values less than 0.05 were considered statistically significant.
Results
Identification of prognostic disulfidptosis-related genes in HCC
A Cox univariate analysis was conducted on the TCGA-HCC patient data to identify prognostic genes. A total of 3,621 prognostic genes were identified exhibiting a P value less than 0.05 in HCC patients. The Venn diagram was further used to identify the prognostic disulfidptosis-related genes and ultimately identified 30 prognostic genes, including ANP32E, IPO4, BOP1, RPN1, SLC7A11, AAAS, HNRNPH1, PPIH, PRC1, SLC3A2, TARDBP, PCBP2, MYL6B, ME1, SQSTM1, PRDX1, FLNC, INF2, CD2AP, MYH11, LRPPRC, STRAP, HNRNPH3, DHX9, NCKAP1, CHCHD3, HNRNPM, FANCI, HNRNPA3, and SART3 (Figure 1A). To assess their functions of these 30 prognostic disulfidptosis-related genes, KEGG and GO enrichment analyses were conducted. The KEGG enrichment analysis indicated that the pathways associated with key disulfidptosis-related prognostic genes could significantly impact ferroptosis, spliceosome, and vascular smooth muscle contraction (Figure 1B). The GO analysis of the biological process revealed that disulfidptosis-related prognostic genes were linked to RNA splicing, cellular component assembly, cellular component biogenesis, biological processes involved in symbiotic interaction, and messenger RNA (mRNA) metabolic processes (Figure 1C).
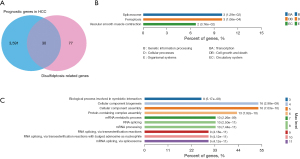
HCC subtypes of disulfidptosis-related genes
Although disulfidptosis is closely linked to tumor development (6-9), the specific function of disulfidptosis-related genes in HCC remains to be fully explored. Using consensus clustering analysis, we classified TCGA-HCC patients into distinct subtypes based on the expression of 30 disulfidptosis-related genes (Figure 2A,2B). The principal component analysis (PCA) showed that TCGA-HCC patients were effectively grouped into two clusters based on a clustering variable (kappa) of 2 (Figure 2A). The heatmap of TCGA-HCC patients showed a distinct separation between the two clusters (Figure 2C). The OS rate was significantly higher in low-risk cluster 2 (C2) compared to high-risk cluster 1 (C1) [hazard ratio (HR), 1.867; 95% confidence interval (CI): 1.315–2.651; P<0.001; Figure 2D].
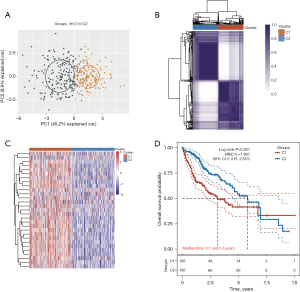
Differential gene analysis between the high- and low-risk clusters
The DEGs between the C1 and C2 clusters were identified through the use of a volcano plot. The biological functions of the DEGs were determined using GO and KEGG enrichment analyses.
GO analysis of the biological process revealed that the upregulated DEGs were linked to mitotic nuclear division, chromosome segregation, nuclear division, cell cycle checkpoint, and DNA replication (Figure 3A), whereas the downregulated DEGs were linked to beta-alanine metabolism, fatty acid metabolic process, and organic acid biosynthetic process (Figure 3B). The KEGG enrichment analysis indicated that the pathways associated with the upregulated DEGs could significantly impact cell cycle, DNA replication, extracellular matrix (ECM)-receptor interaction, cellular senescence, and the p53 signaling pathway (Figure 3C), whereas the pathways associated with the downregulated DEGs could significantly impact retinol metabolism, the PPAR signaling pathway, and tyrosine metabolism (Figure 3D). Some studies have shown that the cell cycle, DNA replication, ECM-receptor interaction, and the p53 signaling pathway act as tumor markers (10,11).
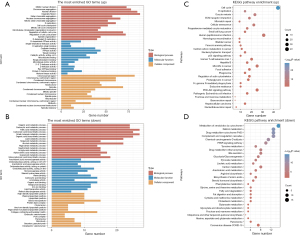
Analysis of immune infiltration between the high- and low-risk groups
We evaluated the immune response in two clusters related to disulfidptosis in HCC. The box plots revealed a significant variation in immune cell populations, specifically endothelial cells, CD4+ T cells, and macrophages between the C1 and C2 HCC samples (Figure 4A). The box plots revealed higher expression levels of nine out of ten immune checkpoint inhibitor (ICI)-related genes (CD274, CTLA4, HAVCR2, IGSF8, ITPRIPL1, LAG3, PDCD1, PDCD1LG2, and TIGIT) in the C1 HCC samples compared to the C2 samples (Figure 4B). Based on these results, there is a strong correlation between disulfidptosis and immune function in HCC.
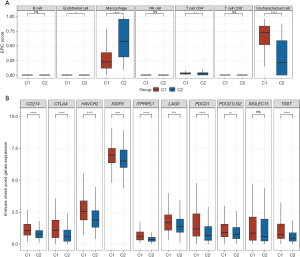
The tumor stemness in two disulfidptosis-related groups in HCC
Cancer stem cells (CSCs) play a critical role in tumor initiation, recurrence, metastasis, and resistance to chemotherapy. The gene expression profiling of CSCs, which included 11,774 CSC-related genes, revealed that G1 HCC patients exhibited higher CSC scores, indicating the enhanced tumorigenic capacity and tumor cell stemness of the G2 subtype (Figure 5).
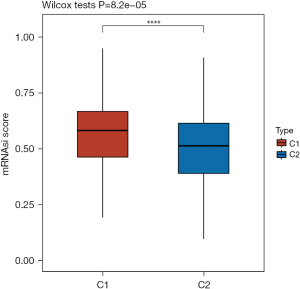
Construction of a prognostic model based on disulfidptosis-related genes
The 30 key disulfidptosis-related genes were screened using LASSO regression analysis. Then, multivariate Cox regression was conducted using genes with non-zero penalty coefficients. The risk score was calculated as follows: risk score = 0.0088 × ANP32E + 0.0191 × BOP1 + 0.0969 × RPN1 + 0.113 × SLC7A11 + 0.1884 × PPIH + 0.0408 × PCBP2 + 0.0244 × ME1 + 0.1434 × PRDX1 + 0.0274 × FLNC + 0.2259 × INF2 − 0.1111 × MYH11 + 0.1154 × LRPPRC + 0.0109 × HNRNPM. The risk score for TCGA-HCC patients was calculated, and the median risk score was used as the cutoff to classify TCGA-HCC patients into low- and high-risk groups (Figure 6A). Low-risk patients had a significantly higher OS rate than high-risk patients (HR, 2.773; 95% CI: 1.914–4.019; P<0.001; Figure 6B). The area under the curve (AUC) at 1, 3, and 5 years was 0.758, 0.711, and 0.718, respectively, indicating an effective prediction of outcomes by the model (Figure 6C).
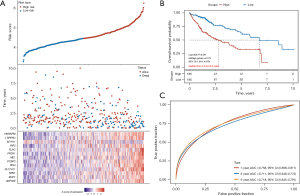
Discussion
In TCGA-HCC patients, a total of 3,621 prognostic genes and 30 key prognostic disulfidptosis-related genes (ANP32E, IPO4, BOP1, RPN1, SLC7A11, AAAS, HNRNPH1, PPIH, PRC1, SLC3A2, TARDBP, PCBP2, MYL6B, ME1, SQSTM1, PRDX1, FLNC, INF2, CD2AP, MYH11, LRPPRC, STRAP, HNRNPH3, DHX9, NCKAP1, CHCHD3, HNRNPM, FANCI, HNRNPA3, and SART3) were identified. Using key prognostic disulfidptosis-related genes, TCGA-HCC patients were categorized into low- and high-risk clusters. The upregulated DEGs in high-risk C1 could significantly impact cell cycle, DNA replication, ECM-receptor interaction, mismatch repair, cellular senescence, and the p53 signaling pathway, whereas the pathways associated with the downregulated DEGs in high-risk C1 could significantly impact metabolism of xenobiotics by cytochrome P450, retinol metabolism, complement and coagulation cascades, the PPAR signaling pathway, and tyrosine metabolism. Furthermore, the immune activity of the high-risk C1 group was different with low-risk C2 group. The 13 disulfidptosis-related genes were finally screened using LASSO regression analysis, including ANP32E, BOP1, RPN1, SLC7A11, PPIH, PCBP2, ME1, PRDX1, FLNC, INF2, MYH11, LRPPRC, and HNRNPM.
It is difficult to find relevant articles on disulfideptosis or its related genes (5,12). In this study, based on key prognostic disulfidptosis-related genes, TCGA-HCC patients were categorized into low- and high-risk clusters. The upregulated DEGs in high-risk C1 could significantly impact cell cycle, DNA replication, ECM-receptor interaction, mismatch repair, cellular senescence, and the p53 signaling pathway, which acted as tumor markers. Furthermore, the immune activity of the high-risk C1 group was different with the low-risk C2 group. These findings indicate that HCC tumors can be divided into two subgroups based on disulfidptosis-related genes.
Disulfidptosis, a recently identified form of cell death distinct from necroptosis and apoptosis, underscores the significance of disulfide and glucose metabolism in the demise of tumor cells (5). Disulfidptosis-targeted therapies based on molecular landmarks of tumor disulfidptosis across cancer types (13). In lung adenocarcinoma, Huang et al. identified a sulfidptosis-related gene signature for prognostic implications (9). The extracellular cystine transporter SLC7A11 facilitates cysteine synthesis, thereby sustaining intracellular glutathione levels to avert cell death induced by aberrant disulfide bonds and the accumulation of reactive oxygen species (ROS) (14). The accumulation of ROS is a characteristic feature shared by both disulfidptosis and cuproptosis, and it plays a pivotal role in inflammation-related diseases such as cancer (5). ROS exhibit a dual function in tumors: at elevated levels, they promote cell death, whereas at lower levels, they can stimulate tumor growth (5,6).
The expression levels of disulfidptosis-related genes showed a positive correlation with the prognosis of HCC patients. A prognostic model comprising 13 disulfidptosis-related genes (ANP32E, BOP1, RPN1, SLC7A11, PPIH, PCBP2, ME1, PRDX1, FLNC, INF2, MYH11, LRPPRC, and HNRNPM) was established for HCC patients.
The expression of ANP32E is associated with immune cell infiltration and immune status in the tumor microenvironment of HCC (15). Furthermore, patients with high ANP32 family expression exhibited poor sensitivity to immunotherapy (15). The protein, BOP1, contributes to the development of HCC by inducing epithelial-to-mesenchymal transition (16). Moreover, inhibition of circ-SNX27 suppressed the invasion and proliferation of HCC cells and facilitated their apoptosis via the RPN1 signaling pathway (17). Enhanced SOCS2-mediated ubiquitination of SLC7A11 promotes ferroptosis and enhances radiosensitization in HCC (18). PCBP2 plays a crucial role in regulating post-transcriptional and translational processes of various signaling molecules by directly binding to single-stranded poly(C) motifs (19). The overexpression of PCBP2 is associated with a poor prognosis and increased cell growth in human HCC (19). Increased PRDX1 expression in HCC patients is associated with improved responsiveness to immunotherapy agents including sorafenib, IGF-1R inhibitor, and JAK inhibitor (20).
This study has some limitations. Our research findings are based on retrospective analysis, and thus should be validated in prospective studies. Secondly, future research should conduct functional experimental studies on these genes to gradually clarify the role of these genes in HCC.
Conclusions
Genes related to disulfidptosis are closely associated with tumor classification and immunity in patients with HCC. The gene signature related to disulfidptosis demonstrated a strong association with the HCC prognosis. These findings could provide new perspectives for the diagnosis and treatment of HCC.
Acknowledgments
Funding: This work was supported by
Footnote
Reporting Checklist: The authors have completed the TRIPOD reporting checklist. Available at https://jgo.amegroups.com/article/view/10.21037/jgo-24-522/rc
Peer Review File: Available at https://jgo.amegroups.com/article/view/10.21037/jgo-24-522/prf
Conflicts of Interest: All authors have completed the ICMJE uniform disclosure form (available at https://jgo.amegroups.com/article/view/10.21037/jgo-24-522/coif). T.B.d.C. receives honoraria from Bristol-Myers Squibb, Merck Sharp & Dohme Corp, Astra-Zeneca, MD Health Brasil, Remedy Health Group; support for attending meetings and travel from Ipsen and A2Bio; and payment for participation in Advisory Board from Ipsen and Moderna. The other authors have no conflicts of interest to declare.
Ethical Statement: The authors are accountable for all aspects of the work in ensuring that questions related to the accuracy or integrity of any part of the work are appropriately investigated and resolved. The study was conducted in accordance with the Declaration of Helsinki (as revised in 2013).
Open Access Statement: This is an Open Access article distributed in accordance with the Creative Commons Attribution-NonCommercial-NoDerivs 4.0 International License (CC BY-NC-ND 4.0), which permits the non-commercial replication and distribution of the article with the strict proviso that no changes or edits are made and the original work is properly cited (including links to both the formal publication through the relevant DOI and the license). See: https://creativecommons.org/licenses/by-nc-nd/4.0/.
References
- Rumgay H, Arnold M, Ferlay J, et al. Global burden of primary liver cancer in 2020 and predictions to 2040. J Hepatol 2022;77:1598-606. [Crossref] [PubMed]
- Qi X, Li Q, Che X, et al. Application of Regulatory Cell Death in Cancer: Based on Targeted Therapy and Immunotherapy. Front Immunol 2022;13:837293. [Crossref] [PubMed]
- Newton K, Strasser A, Kayagaki N, et al. Cell death. Cell 2024;187:235-56. [Crossref] [PubMed]
- Xu Y, Li Q, Lin H. Bioinformatics analysis of CMTM family in pan-cancer and preliminary exploration of CMTM6 in bladder cancer. Cell Signal 2024;115:111012. [Crossref] [PubMed]
- Zhang L, Chou CP, Moo-Young M. Disulfide bond formation and its impact on the biological activity and stability of recombinant therapeutic proteins produced by Escherichia coli expression system. Biotechnol Adv 2011;29:923-9. [Crossref] [PubMed]
- Strasser A, Vaux DL. Cell Death in the Origin and Treatment of Cancer. Mol Cell 2020;78:1045-54. [Crossref] [PubMed]
- Shao W, Yang Z, Fu Y, et al. The Pyroptosis-Related Signature Predicts Prognosis and Indicates Immune Microenvironment Infiltration in Gastric Cancer. Front Cell Dev Biol 2021;9:676485. [Crossref] [PubMed]
- Zhang W, Yao S, Huang H, et al. Molecular subtypes based on ferroptosis-related genes and tumor microenvironment infiltration characterization in lung adenocarcinoma. Oncoimmunology 2021;10:1959977. [Crossref] [PubMed]
- Huang J, Zhang J, Zhang F, et al. Identification of a disulfidptosis-related genes signature for prognostic implication in lung adenocarcinoma. Comput Biol Med 2023;165:107402. [Crossref] [PubMed]
- Senga SS, Grose RP. Hallmarks of cancer-the new testament. Open Biol 2021;11:200358. [Crossref] [PubMed]
- Pickup MW, Mouw JK, Weaver VM. The extracellular matrix modulates the hallmarks of cancer. EMBO Rep 2014;15:1243-53. [Crossref] [PubMed]
- Liu X, Nie L, Zhang Y, et al. Actin cytoskeleton vulnerability to disulfide stress mediates disulfidptosis. Nat Cell Biol 2023;25:404-14. [Crossref] [PubMed]
- Zhao D, Meng Y, Dian Y, et al. Molecular landmarks of tumor disulfidptosis across cancer types to promote disulfidptosis-target therapy. Redox Biol 2023;68:102966. [Crossref] [PubMed]
- Xia Y, Liu S, Li C, et al. Discovery of a novel ferroptosis inducer-talaroconvolutin A-killing colorectal cancer cells in vitro and in vivo. Cell Death Dis 2020;11:988. [Crossref] [PubMed]
- Liu X, He Y, Wang P, et al. ANP32 Family as Diagnostic, Prognostic, and Therapeutic Biomarker Related to Immune Infiltrates in Hepatocellular Carcinoma. Dis Markers 2022;2022:5791471. [Crossref] [PubMed]
- Chung KY, Cheng IK, Ching AK, et al. Block of proliferation 1 (BOP1) plays an oncogenic role in hepatocellular carcinoma by promoting epithelial-to-mesenchymal transition. Hepatology 2011;54:307-18. [Crossref] [PubMed]
- Zheng C, Liang J, Yu S, et al. Circ-SNX27 sponging miR-375/RPN1 axis contributes to hepatocellular carcinoma progression. Korean J Physiol Pharmacol 2023;27:333-44. [Crossref] [PubMed]
- Chen Q, Zheng W, Guan J, et al. SOCS2-enhanced ubiquitination of SLC7A11 promotes ferroptosis and radiosensitization in hepatocellular carcinoma. Cell Death Differ 2023;30:137-51. [Crossref] [PubMed]
- Zhang X, Hua L, Yan D, et al. Overexpression of PCBP2 contributes to poor prognosis and enhanced cell growth in human hepatocellular carcinoma. Oncol Rep 2016;36:3456-64. [Crossref] [PubMed]
- Zhang S, Li X, Zheng Y, et al. Single cell and bulk transcriptome analysis identified oxidative stress response-related features of Hepatocellular Carcinoma. Front Cell Dev Biol 2023;11:1191074. [Crossref] [PubMed]
(English Language Editor: J. Jones)