Potential molecular metabolic mechanisms underlying the effects of cimifugin in gastric cancer through single-cell and bulk RNA sequencing combined with network pharmacology
Highlight box
Key findings
• Cimifugin potently suppresses MKN28 cell proliferation, invasion, and migration, highlighting its therapeutic potential against gastric cancer (GC).
• The compound modulates critical metabolic pathways, suggesting a role in cancer cell metabolism.
• Cimifugin’s interaction with AKR1C2, MAOB, and PDE2A indicates a possible multi-target therapeutic strategy.
What is known and what is new?
• This study links GC progression to metabolic heterogeneity within the tumor microenvironment, a recognized factor in cancer biology. Cimifugin has gained attention in recent years for its potential role in alleviating inflammation, neurological disorders, pain, cancer, and metabolic disorders.
• The manuscript employs single-cell RNA sequencing, bulkseq, and network pharmacology to provide an analysis of GC and cimifugin’s pharmacological effects, and further validates the therapeutic potential of cimifugin by demonstrating its inhibitory effects on cell proliferation and migration.
What is the implication, and what should change now?
• Cimifugin’s effects in GC offer preliminary evidence for clinical application, with its investigated targets potentially indicating strategies for herbal medicine development.
Introduction
Gastric cancer (GC), a common cancer of the digestive system, is the fifth most prevalent and fourth most fatal type of cancer worldwide (1). Currently, several GC treatment strategies focus on chemotherapy, targeted therapy, immunotherapy, and surgery (2). Considering that risk factors vary among GC cases, this observation is connected to the metabolic competition and reprogramming in the tumor microenvironment (TME), influencing treatment and clinical outcomes in patients with cancer. However, GC susceptibility demonstrates considerable heterogeneity across patients—a phenomenon closely related to cellular metabolic reprogramming in the TME—and has important implications for cancer treatment and clinical prognosis (3).
Traditional Chinese medicine (TCM), which has been used in China for centuries, has been associated with considerable cancer prevention and treatment effects. TCM remains an imperative choice in modern cancer adjuvant therapy because of its high efficacy, few toxic side effects, wide availability, and affordable price. Many natural herbal components, which possess antitumor activity, are often used as adjuvants to chemotherapeutic agents for enhancing patient sensitivity to treatment and mitigating side effects. Cimifugin, a crucial phytochemical constituent of Saposhnikovia divaricata and Cimicifuga racemosa coumarins, has gained attention in recent years for its potential role in alleviating inflammation, neurological disorders, pain, cancer (4), and metabolic disorders (5). Dongyuan Li, the founder of the Tonifying Spleen School and one of the four famous physicians of the Jin-Yuan Dynasty, contributed significantly to the development of Chinese medicine (6); he favored the addition of Cimicifuga for treating not only spleen and stomach disorders but also stomach cancer. Fever and low-grade fever are common symptoms in patients with advanced GC—which TCM practitioners attribute to the inhibition of yang qi of the spleen and stomach by the disease. Shengyang Sanhuo Decoction (SSD), which contains both Saposhnikovia and Cimicifuga, is a commonly used, classic formula derived from Dongyuan Li’s Lan-Shi-Mi-Cang (written in 1276 CE); it is also widely used today to treat throat cancer. Various Cimicifuga compounds, such as actein, cimiside E, and cimisterol A, may be valuable in GC treatment (7,8). However, the potential anti-GC activity of cimifugin and the underlying mechanisms remain underexplored; this issue is assessed in the current study.
Conventional bulk RNA sequencing (RNA-seq) provides information on average gene expression in tissues; however, it is associated with limitations when used for assessing intercellular functional differences in the TME. Single-cell RNA-seq (scRNA-seq), an emerging technology, can be a powerful approach for analyzing key biological issues such as cellular functional and metabolic heterogeneity of the GC TME (9). In recent years, network pharmacology has been widely used for identifying the active components of Chinese herbal medications and elucidating their pharmacological mechanisms (10). This approach may provide aid in understanding the pharmacological mechanisms underlying cimifugin activity.
In this study, we performed a comprehensive bioinformatics analysis, leveraging scRNA-seq data of early GC (EGC) and nonatrophic gastritis (NAG) cases from the Gene Expression Omnibus (GEO) database and combined them with bulk RNA-seq data from The Cancer Genome Atlas (TCGA) database. Moreover, the potential pharmacological mechanisms underlying the effects of cimifugin on GC were elucidated through the integration of network pharmacology and cell molecular biology experiments. Figure 1 depicts the current study’s workflow. We present this article in accordance with the MDAR reporting checklist (available at https://jgo.amegroups.com/article/view/10.21037/jgo-24-413/rc).
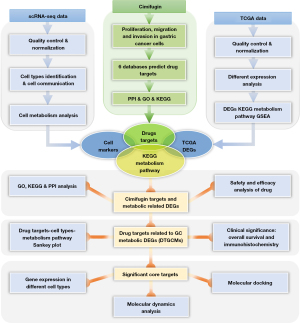
Methods
Cell culture and treatment
Human GC cells MKN28 (BNCC360329) were procured from BeNa Culture Collection (https://www.bncc.com/) and maintained in a complete Roswell Park Memorial Institute (RPMI)-1640 medium (KGM31800S; KeyGEN Bio, Nanjing, China) at 37 ℃ under 5% CO2. Cells were randomly assigned to treatment groups and control groups using a computer-generated randomization sequence. The sample size, calculated to provide power of 0.8 to detect a 20% effect in cell proliferation at a significance level of 0.05, necessitated three biological replicates per group. After treatment with cimifugin (B21156; Yuanye Bio, Shanghai, China) at 0, 40, 80, 160, 320, 640, or 1,280 µM for 24 and 72 hours, the cells’ functional responses were assessed. Biological replicates were conducted three times. Only cells at passages between 3 to 10 were used to ensure genetic stability and avoid potential senescence effects.
Cell counting kit-8 (CCK-8) assay and 5-ethynyl-2'-deoxyuridine (EdU) flow cytometry assay
Cimifugin-treated cells were added to a 96-well plate, and the medium was replaced with fresh culture medium at 100 µL per well. Next, 10 µL of CCK-8 reagent was added to each well, followed by incubation at 37 ℃ for 2 hours (11). Each well’s absorbance at 450 nm was detected under an enzyme-labeling instrument.
Next, cimifugin-treated cells were incubated with a prepared EdU working solution (C0071S; Beyotime Bio, Shanghai, China) and washed with a washing solution at the end of the incubation period (12). The cells were then suspended in 500 µL of phosphate-buffered saline (PBS) and assayed on a flow cytometer (NovoCyte 2060R; ACEA Biosciences, Hangzhou, China).
Cell migration and invasion ability assay
Cells to be assessed were cultured in a 24-well plate, with adhesive chambers for invasion assay and without adhesive chambers for migration assay. In the upper chamber, the volume and cell number of cell suspension were 6×104 and 300 µL, respectively (13). Next, 500 µL of complete medium was added to the lower chamber, and the cells were fixed using 160 µM cimifugin for 72 hours (based on the preliminary proliferation results). The cells were stained using 0.1% crystal violet (G1061; Solarbio, Beijing, China). Excess stain was wiped off from the chamber by using a cotton swab, and the cells were observed under a microscope. After images were obtained, the staining solution was removed, and 33% acetic acid was added. Each well’s absorbance at 562 nm was measured with an enzyme labeler (WD-2012B; Liuyi Bio, Beijing, China).
Data download and processing
scRNA-seq data collection
We sourced scRNA-seq data of three NAG cases and one EGC case from the GEO (GSE134520) database (https://www.ncbi.nlm.nih.gov/geo/).
Bulk RNA-seq data collection
From the Xena platform (https://xenabrowser.net/datapages/) (14) and the stomach adenocarcinoma (STAD) dataset of TCGA, we obtained expression data of 407 samples, comprising 375 STAD and 32 noncancerous control samples. Since the study used publicly available data, no additional ethical approval was required. The study was conducted in accordance with the Declaration of Helsinki (as revised in 2013).
Single-cell data analysis
To delineate robust cell subpopulations, we preprocessed data by using the R (version 4.3.0) package Seurat (15). We set the following threshold for gene expression: gene expression in ≥3 cells and cells expressing ≥200 genes. Mitochondrial and ribosomal RNA percentages were calculated, ensuring mitochondrial content of <25% and number of unique molecular identifiers per cell of ≥100. Normalization of the Seurat objects for expression matrixes within groups was achieved using the SplitObject and SCTransform functions. Integration of normalized Seurat objects was facilitated using the FindIntegrationAnchors and IntegrateData functions. FindNeighbors (with k.param set to 20) and FindClusters functions (with Resolution set to 0.25) were used to identify cell subpopulations. Cell subpopulations were visualized with t-distributed stochastic neighbor embedding (tSNE).
Cell type identification
We used SingleR (version 2.2.0) (16) and the CellMarker database (17) to detect CellMarker genes and identify the cell types. Fisher’s test was used to compare NAG and EGC cells with a fold change (FC) value of >4 or <0.25 and P value of <0.05. The feature plot and dot plot functions were used to analyze feature gene expression across both cell types.
Cell-cell communication and cell metabolic activity analysis
We used CellChat (version 1.6.1) (18) to elucidate the intricate intercellular communication and the mechanisms of signaling molecules at the single-cell level across various cell types. We also used the R package scMetabolism (version 0.2.1) (19) to quantify metabolic activity with precision at the single-cell resolution.
Cimifugin structure profiling and target prediction
The detailed chemical structure of cimifugin was retrieved from the PubChem database (20). The MOL2 format of the compound was also downloaded for subsequent analysis. The potential biological targets of cimifugin were comprehensively predicted using 6 reputable public webtools and webservers: SwissTargetPrediction (21), SEA Search Server (22), Super-PRED (23), ChemMapper (24), GalaxyWEB (25), and PharmMapper (26). The gene symbol names corresponding to these targets were standardized using data from the UniProt database (27). The union of all targets identified using these databases was compiled, and a Venn diagram was generated using the BioLadder platform (https://www.bioladder.cn/) to visualize the intersection of these targets. Deep-PK database (28) was utilized to predict the toxicity and pharmacological properties of cimifugin. Additionally, based on PiscesCSM database (https://biosig.lab.uq.edu.au/piscescsm/), the drug’s potential effects in combination with various anticancer drugs was forecasted across a range of cancers.
Bulk RNA-seq differentially expressed genes (DEGs) analysis
DESeq2 (version 1.40.1) (29) was used to identify DEGs between STAD and normal samples in TCGA data, filtering with P<0.05 and |log2FC| >1.5.
Identification of drug targets related to GC metabolic DEGs (DTGCMs) and core targets of clinical significance
We used a meticulous screening process to identify DTGCMs in three subsets: drug targets, TCGA DEGs, and Kyoto Encyclopedia of Genes and Genomes (KEGG) metabolic genes. The core targets were further refined using four subsets: cell markers, TCGA DEGs, KEGG metabolic genes, and drug targets. The KEGG metabolic genes were sourced from scMetabolism. A Venn diagram was constructed using BioLadder to illustrate the overlap between these subsets. To elucidate the connections between cimifugin’s drug targets, cell types, and KEGG metabolic pathways, we created a Sankey plot by using Origin Pro (2021 v. 9.8).
Protein-protein interaction (PPI) network construction
To construct a PPI network, the identified targets of cimifugin and DTGCMs were integrated into the Search Tool for the Retrieval of Interacting Genes/Proteins (STRING) database (30) separately, with a confidence score threshold set to 0.4 and species specificity limited to Homo sapiens. Cytoscape (version 3.10.0) (31) was utilized for the topological analysis of the target network.
Functional enrichment and gene set enrichment analyses (GSEAs)
Gene Ontology (GO) and KEGG pathways (32) were analyzed using clusterProfiler (version 4.8.1) (33), with a focus on the metabolic pathway differences across various cell types in scRNA-seq and bulk RNA-seq data. KEGG metabolic pathway datasets were used for further enrichment analysis, with significance determined at Benjamini-Hochberg-adjusted P<0.05 and gene count ≥3. GSEA was used to characterize the biologically relevant DEGs within KEGG metabolic pathways in the TCGA STAD data.
Assessment of core targets expression and prognostic significance
To preliminarily validate the clinical relevance of the core targets identified from the STAD data, we examined the available data from public databases. The Human Protein Atlas (HPA) database (34) was queried to obtain representative immunohistochemical (IHC) images of the core target proteins in normal and STAD tissues, facilitating the evaluation of protein expression level differences. We used the Gene Expression Profiling Interactive Analysis 2 (GEPIA2) database (35) to assess the transcriptome-level expression differences of core targets between the STAD and normal cohorts. Overall survival (OS) was defined as the period from surgical resection to death. To investigate the impact of proteins on the prognosis of patients with STAD, the association between core targets expression and OS was analyzed by categorizing high and low expression levels into two groups (with the median as the cutoff) using the STAD and normal cohorts. Kaplan-Meier survival curves were derived from the GEPIA2 database. Significant core targets were identified by their P values for OS and log2-transcript per million (TPM). The cBioPortal database (36) was utilized to illustrate the mutational landscape of significant core targets through oncoprint visualizations.
Molecular docking and molecular dynamics (MD) simulation
The molecular structures of the significant core targets were retrieved from the RCSB Protein Data Bank (PDB) (37) and saved in the PDB format for subsequent use in AutoDock Vina (version 1.1.2) (38). Cimifugin structure was obtained from the PubChem database and imported into AutoDock Vina in the MOL2 format. To analyze protein-ligand interactions, we used the Protein-Ligand Interaction Profiler (PLIP) (39). PyMOL Molecular Graphics System (version 2.5; Schrödinger) (40) was utilized to modify the proteins—including original ligand and water molecule removal—and correct protein structures.
MD simulations were performed using Gromacs (41) with the Amber14SB force field and TIP3P water model. Electrostatics were managed using Particle mesh Ewald. Simulations were conducted in canonical (NVT) and isothermal and isobaric system (NPT) ensembles for 100 ns; then, MD simulation results were calculated and stored every 10 ps. Root mean square deviation (RMSD), radius of gyration (Rg), root mean square fluctuation (RMSF), and solvent accessible surface area (SASA) were monitored, and binding free energy was calculated with gmx MMPBSA.
Statistical analysis
GraphPad Prism (version 9.0.0; GraphPad Software, San Diego, CA, USA) was used for statistical analysis and graphical representation. All experiments were repeated three times. Our quantitative results, expressed as mean ± standard deviation, were compared between multiple groups by using one-way analysis of variance (ANOVA) with a test level of α=0.05. A P value of <0.05 was considered to indicate significant differences.
Results
Construction of cell clusters and communication in EGC and NAG using scRNA-seq data
Single-cell analysis and cell clustering
First, after filtering ineligible cells, we used the remaining 13,490 cells for subsequent analysis. Single-cell filtration analysis yielded 3,010 and 6,283 cells in the EGC and NAG groups, respectively. The expression threshold of nFeature RNA, nCount RNA, percent.mt, percent.rb for scRNA-seq data were shown (Figure S1A,S1B). Our ANOVA for gene expression in the core cells revealed 3,000 highly variable genes (Figure S1C). Principal component analysis (PCA), uniform manifold approximation and projection (UMAP), and tSNE were then applied to different cell phases and groups (Figure S1D). Consequently, the cells were subsequently classified into 11 independent clusters, distributed between two groups using the tSNE algorithm (Figure 2A). The “FindAllMarkers” function was employed to identify marker genes in each of the 11 cell clusters. These clusters were annotated by identifying marker genes by using the singleR package and the CellMarker database. Consequently, we identified cell types such as B cells, basal cells, chief cells, common myeloid progenitors (CMPs), endocrine cells, isthmus cells, macrophages, neck cells, natural killer (NK) cells, pit mucous cells (PMCs), and T cells. The expression of key marker genes in each cell type was visualized using heatmaps and dot plots (Figure 2B,2C). Cell type distribution across different samples and groups was also analyzed (Figure 2D).
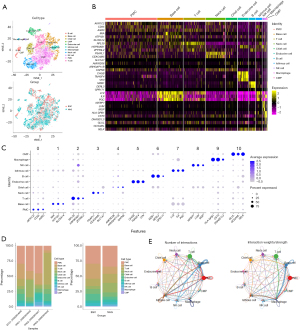
Cell-cell communication analysis results
We used CellChat to analyze the intercellular communication networks within the EGC cells derived from scRNA-seq data (Figure 2E). Consequently, we identified numerous significant ligand-receptor interactions across all 11 cell types. Notably, in the EGC group, the weight and strength of the interactions of macrophages with PMCs, basal cells, and isthmus cells were significantly enhanced. Stromal endocrine cells exhibited a communication pattern relatively uniform with other cell types. The crosstalk of macrophages with NK and T cells was found to facilitate the M1/M2 polarization.
Cell metabolism analysis
Next, we assessed the metabolic characteristics of 11 cells in human EGC. Gluconeogenesis, oxidative phosphorylation (OXPHOS), the tricarboxylic acid (TCA) cycle, amino sugar and nucleotide sugar metabolism, butanoate metabolism, and lipid metabolism demonstrated higher levels in epithelial cells than in other cell types. Fatty acid elongation, degradation, and biosynthesis were highly expressed in macrophages, similar to the biological function of tumor-associated macrophages (TAMs), suggesting their association with M2 activation in TAMs (Figure 3A). To explore the distribution of high-score metabolic pathways in all 11 cell types, we visualized the landscape of eight high-score pathways in the EGC group by using tSNE dimplots. OXPHOS and gluconeogenesis expression was noted to be higher in neck cells, PMCs, basal cells, and isthmus cells, whereas butanoate metabolism expression was higher in PMCs, basal cells, and T cells (Figure 3B).
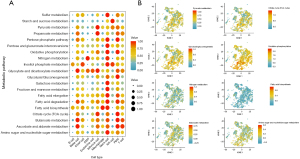
Data and GSEA of KEGG metabolic pathways
We obtained STAD tumor and normal sample data from the TCGA database and performed differential analysis by using DESeq2. In total, 2,596 genes were identified as DEGs, including 1,207 upregulated and 1,389 downregulated genes (Figure 4A); these DEGs are listed in table available at https://cdn.amegroups.cn/static/public/jgo-24-413-1.xlsx. These 2,596 DEGs were subjected to metabolism-related GSEA based on the KEGG metabolism pathway dataset in the scMetabolism package. The results demonstrated the presence of increased suppression of xenobiotic and drug metabolism pathway expression during tumorigenesis, along with inhibition of glycolysis, gluconeogenesis, pentose-glucuronate interconversion, and glutathione metabolism. In other words, during the progression of tumorigenesis, cells undergo metabolic reprogramming and xenobiotic and drug metabolism become suppressed (Figure 4B).
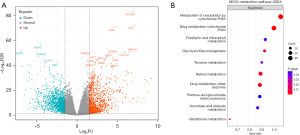
Effects of cimifugin on MKN28 cell proliferation, invasion, and migration
Our CCK-8 assay results indicated that cimifugin inhibits the GC cell line MKN28. In particular, compared with no treatment (control), treatment with 320 µM cimifugin significantly reduced MKN28 cell viability after 24 hours. Moreover, MKN28 cells treated with 40, 80, 160, 320, and 640 µM cimifugin for 72 hours demonstrated significant inhibition (Figure 5A).
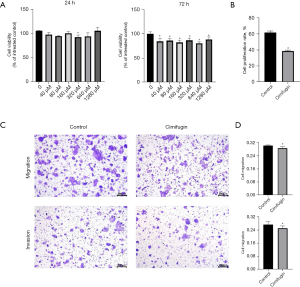
Our EdU flow cytometry assay results indicated that cimifugin also inhibited MKN28 cell proliferation. In particular, compared with control cells, MKN28 cells treated with 1,600 µM cimifugin for 72 hours demonstrated a significant decrease in proliferation (Figure 5B).
Cimifugin also inhibited MKN28 cell migration and invasion in the transwell assay. In particular, compared with control cells, MKN28 cells treated with 1,600 µM cimifugin for 72 hours demonstrated a significant reduction in migration and invasion (Figure 5C,5D).
Predictions of cimifugin’s drug targets, biological functions, safety, and efficacy
We downloaded the three-dimensional (3D) chemical structure of cimifugin from PubChem (Figure 6A). We also predicted cimifugin’s targets using six web resources: SwissTargetPrediction, SEA Search Server, Super-PRED, ChemMapper, GalaxyWEB, and PharmMapper. We collated the 6 sets of target results (Figure 6B), predicting 691 targets in total. After the exclusion of duplicate targets, 569 unique drug targets were included in the subsequent analyses. For instance, these 569 targets were entered into the STRING database for PPI analysis and to draw the PPI network diagram by using Cytoscape (Figure 6C).
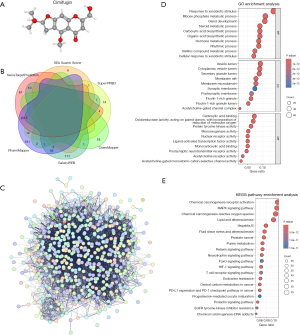
These 569 targets were also analyzed using clusterProfiler for GO enrichment and KEGG pathway analysis. In the biological process GO enrichment analysis, cimifugin’s targets were enriched in metabolic and biosystemic processes, such as ribose phosphate metabolic process, carboxylic acid biosystemic process, steroid metabolic process, hormone metabolic process, and process of response to oxygen levels. In other words, in the human body, cimifugin targets various cellular metabolic processes, including nucleotide metabolism, fat metabolism, sugar and acid metabolism, oxidation reaction, and oxidative stress processes. In cell component analysis, most of the cimifugin targets were enriched in the vesicle lumen, cell membrane, synaptic membrane, and other cell structures, indicating that when in large quantities, the compound acts on the surface structure of cells. In the molecular function analysis of cimifugin, nuclear receptor activity, carboxylic acid binding, and oxidoreductase activity were found to be highly enriched; therefore, the compound demonstrates some regulatory effect on cell energy metabolism, carbon cycle, and nuclear receptor activity. Taken together, these results indicated that some of the cimifugin targets are present in the TCA cycle, which was the core pathway for the metabolism of not only various nutrients but also the carbon chain degradation products of sugars, fats, proteins, and nucleic acids (Figure 6D, table available at https://cdn.amegroups.cn/static/public/jgo-24-413-2.xlsx).
Our KEGG enrichment analysis demonstrated that cimifugin targets were enriched in some chemical carcinogenesis pathways (including central carbon metabolic pathways underlying cancer, lipid, and atherosclerosis development) and the HIF-1 signaling pathway. Therefore, cimifugin may be involved in oxidative stress development, chemical factor production, and various energy metabolic pathways during tumor growth. Cimifugin targets were also highly enriched in programmed cell death ligand 1 (PD-L1) expression and programmed cell death protein 1 (PD-1) checkpoint pathways in cancer and the T-cell receiver pathway. Therefore, cimifugin may also regulate cancer immunity (Figure 6E, table available at https://cdn.amegroups.cn/static/public/jgo-24-413-3.xlsx).
We simultaneously calculated the pharmacokinetic and toxicity properties of cimifugin based on deep learning to assess the safety. The drug exhibited a logPaap value of −4.85 through the Caco-2 cell line in humans, demonstrating good absorption capacity, and its predicted oral bioavailability confidence was as high as 0.988, classified as “bioavailable”. This indicated that its absorption and distribution within the gastrointestinal tract were safe and effective, with most components being effectively utilized by the human body. Although it might potentially have caused respiratory diseases and underlying cardiac toxicity in some cases, the risk of hepatotoxicity and skin irritation was low, providing positive information on the utilization of cimifugin in the human body (Table S1).
Cimifugin has been predicted to interact with six chemotherapy drugs: capecitabine, cisplatin, 5-fluorouracil (5-FU), irinotecan, oxaliplatin, and paclitaxel. Cimifugin showed complex effects in interactions with a variety of chemotherapeutic agents. Overall, it was usually antagonistic in melanoma and ovarian cancer, but more synergistic in lung, breast, colon, and prostate cancers. Although we did not have direct data to evaluate the performance of cimifugin in GC, based on its performance in other cancer types, we speculated that it may also exhibit a similar interaction pattern in GC (Table S2).
Screening and biofunctional analysis of DTGCMs and cimifugin core targets
The intersections of 2,596 DEGs from TCGA, 569 cimifugin targets from GO, 1,667 KEGG metabolic genes, and 1,409 marker genes in the 11 cell types were visualized, as shown in Figure 7A. Next, we analyzed the eight core target intersection genes in the intersections of all four subsets, as well as 36 DTGCMs in the intersections of drug targets, TCGA DEGs, and KEGG metabolic genes. Based on the 36 DTGCMs, we performed PPI (Figure 7B) analyses. The results illustrated DTGCMs exhibited interactions among aldo-keto reductase (AKR) family proteins, monoamine oxidase (MAO) family proteins and cytochrome P450 (CYP) family proteins. KEGG pathway enrichment analysis was performed on 36 DTGCMs associated with gastric tumorigenesis and 26 pathways were obtained (P<0.05); only the first 15 pathways are listed in Figure 7C. Of them, steroid hormone biosynthesis, folate biosynthesis, chemical carcinogenesis pathways demonstrated higher enrichment scores. Expression of eight core targets in the 11 cell types was illustrated in Figure 7D; these targets demonstrated more significant expression in PMCs, neck cells, T cells, and basal cells. A Sankey plot was drawn to describe the relationship among cimifugin, eight core targets (AKR1B10, AKR1C2, AKR1C3, CA2, CBR1, GSTA1, MAOB, PDE2A), cell types, and metabolic pathways involved in GC treatment (Figure 7E, Table S3).
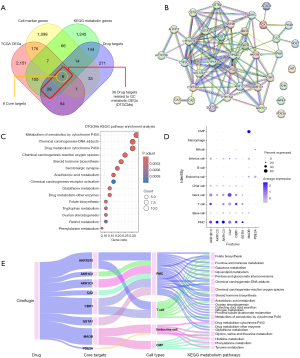
Clinical significance of core targets
To assess the therapeutic and prognostic significance of the core targets in STAD, we examined the expression data and OS of patients using data from the HPA and GEPIA2 databases. Our analysis showed three targets AKR1C2, MAOB, PDE2A significant impact on both OS and expression in GC. The expressions of AKR1C2, MAOB proteins and genes were considerably lower in STAD tissues than in normal tissues (Figure 8A,8B). However, no significant expression differences were observed across the four clinical stages of STAD (Figure 8C). Notably, although AKR1C2, MAOB, PDE2A were downregulated in cancer tissues, survival analysis indicated that patients with lower expression levels of these targets demonstrated longer OS (Figure 8D). The oncoprint plot revealed genetic alterations in the AKR1C2, MAOB, and PDE2A genes in GC and STAD, with 2.1%, 9%, and 6% of the samples exhibiting genetic changes, respectively, including deep deletions and amplifications (Figure 8E). This discrepancy may be attributable to the alterations in the expression of these targets during the initial stages of cancer development; therefore, these genes may not act independently, and they are potentially passively regulated by other factors within the TME.
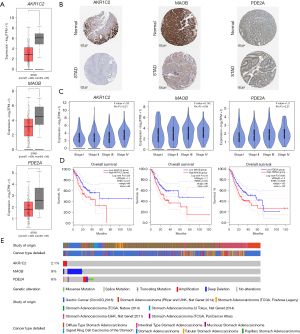
Expression of significant core targets in EGC and NAG
We analyzed AKR1C2, MAOB, and PDE2A expression in different cells and groups based on scRNA-seq data (Figure 9A,9B). The results indicated that the overall expression of AKR1C2, MAOB, and PDE2A was lower in the EGC group than in the NAG group (Figure 9B); these results are consistent with those based on bulk RNA-seq data. However, the expression distribution of these significant core targets in the cells was uneven. According to our results, AKR1C2 was upregulated in isthmus cell and PMC, MAOB was upregulated in EGC endocrine cells, and PDE2A was significantly upregulated in EGC endocrine cells, NK cells, and macrophages (Figure 9A).
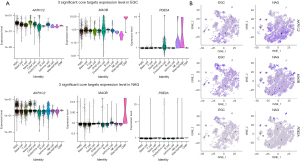
Molecular docking and MD simulation of significant core targets
We molecularly docked three significant core targets proteins with cimifugin and selected the best binding sites (Figure 10A-10C, Table 1). The highest binding energy calculated using AutoDock Vina was −7.7 kcal·mol−1, which was noted for PDE2A (3ITU), AKR1C2 (4JQA), and MAOB (6RKP) were noted to have the second and third highest binding energy, respectively.
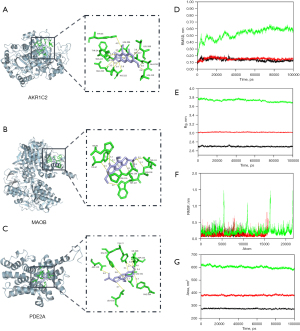
Table 1
Protein | PDB ID | AutoDock Vina score | Hydrophobic interactions | Hydrogen bonds | π-stacking |
---|---|---|---|---|---|
AKR1C2 | 4JQA | −6.75 | TYR24A, HIS222A, GLU224A, TRP227A, LEU268A, LEU306A | TRY55A, SER217A, ALA218A, LEU219A, HIS222A | – |
MAOB | 6RKP | −6.01 | TYR97A, PRO105A, VAL106A, ASN108A, THR111A | HIS90A, TRP107A, ASN108A | TYR97A |
PDE2A | 3ITU | −7.7 | PRO653A, THR703A, GLN833A, LEU836A | ASP652A, TYP680D, ARG701A, TYR788D | – |
PDB, protein data bank.
The stability of AKR1C2, MAOB, and PDE2A systems was assessed using RMSD, Rg, RMSF, and SASA metrics. The MAOB system reached stability after 18 ns with a low RMSD, whereas AKR1C2 maintained stability throughout the 100 ns simulation (Figure 10D). The Rg of results for the AKR1C2, MAOB, and PDE2A systems are presented in Figure 10E. The Rg of the PDE2A system decreased most significantly, indicating that PDE2A has the most compact structure among the three proteins. The RMSF results are presented in Figure 10F. PDE2A showed significant fluctuations in RMSF, suggesting conformational changes, which were confirmed by gmx MMPBSA analysis. SASA analysis revealed increasing surface area for all systems, with PDE2A having the largest, potentially affecting system stability (Figure 10G).
Discussion
Metabolic characteristics of EGC TME
In scRNA-seq analysis, we identified 11 cell types in the EGC TME, including B cells, basal cells, chief cells, CMPs, endocrine cells, isthmus cells, macrophages, neck cells, NK cells, PMCs, and T cells.
Glycolysis and gluconeogenesis were highly active in epithelial cells, influencing immune cell function through metabolites such as lactate and pyruvate. Glyoxylate and dicarboxylate metabolism, linked to p53 (42) and epithelial-mesenchymal transition (EMT) (43), was prominent in epithelial and endothelial cells. In NK cells, an increase in starch and sucrose metabolism, pyruvate metabolism, and inositol phosphate metabolism expression results from NK cell activation (44) and downstream recruitment (45). Pyruvate metabolism was noted to be enriched in epithelial and immune cells. Moreover, pentose and glucuronate interconversions are downregulated, and tumor and immune cells tend to convert pyruvate to lactate via lactic acid fermentation (i.e., glycolysis) to maintain adenosine triphosphate (ATP) production as a part of the Warburg effect (3). The OXPHOS pathway is enriched in EGC epithelial cells, with tumor cells potentially adapting through mitochondrial changes to meet metabolic needs, supporting cell proliferation and survival (46). Fatty acid biosynthesis was noted to be strongly expressed in TAMs with PMCs, basal cells, and T cells, suggesting that the presence of metabolic crosstalk and an increase in fatty acid contents can promote immune escape and metastasis of GC and affect TAMs’ M1/M2 polarization properties (47).
Potential value of cimifugin in GC metabolic reprogramming
We verified the proliferation inhibitory effects of cimifugin on GC cells and time-dependent cytotoxicity differences at 24 and 72 hours. Although a significant concentration dependence was not noted for MKN28 cells, cimifugin demonstrated an inhibitory effect on proliferation migration; however, further verification experiments are required. A literature review revealed that cimifugin has demonstrated anticancer effects in many cancer cell lines (4). A study revealed that cimifugin dose-dependently inhibited respiratory syncytial virus-induced in Hep-2 and A549 cells (P<0.0001); the antiviral activity of cimifugin was time-dependent (P<0.0001) (48). Cimifugin has also been noted to inhibit cell proliferation and induce apoptosis in human pharyngeal carcinoma cells (49).
Our enrichment analysis results revealed that cimifugin is involved in several metabolic pathways and targets are enriched in amino acids, lipids, glycerol, and sterols metabolism. A previous study found that cimifugin reduces intracellular lipid accumulation of 3T3-L1 adipocytes, ameliorates the effects of tumor necrosis factor α-induced insulin resistance and inflammation, and reduces P65 expression and MAPK pathway activation (5). The intersection of cimifugin-targeted metabolic pathways in EGC were shown to be related to folate biosynthesis, phenylalanine, histidine, galactose, glutathione, steroid hormone, and glycerolipid metabolism. Low folate levels correlate with GC severity (50), and altered cholesterol metabolism is a feature of transformed cells (51). Its association with these pathways suggested the potential of cimifugin to limit GC development.
Potential therapeutic targets of cimifugin in GC TME
Notably, three significant core targets underlying EGC regulation demonstrate clinical features in endocrine cells, CMPs, and PMCs; these targets may be potentially valuable for the prevention of EGC TME metabolic regulation in EMT progression.
AKR1C2, a member of the aldehyde/keto reductase superfamily, is involved in pathways including bile acid and bile salt syntheses, oxidoreductase activity, and carboxylic acid binding. We found that STAD tumor groups demonstrated a significant decrease in AKR1C2 expression. A recent study has reported that AKR1C2 silencing results in the downregulation of luminal androgen receptor (LAR) classification genes in triple-negative breast cancer cell lines (52). Although studies on the involvement of AKR1C2 in GC are limited, a recent study suggests that AKR1C2 downregulation in GC development and pathogenesis may be related to iron death regulation and immune responses (53). However, additional research is required to clarify the mechanisms underlying this association.
MAOA and MAOB are considered therapeutic targets for neurological disorders (54) because of their ability to inactivate a wide range of catecholamines (e.g., dopamine, epinephrine, and serotonin). The biological function of MAOB is closely related to the metabolism of glycine, serine, and threonine, and also to the metabolism of histidine, phenylalanine, and tyrosine (Table S3). MAOB demonstrates heterogeneous expression in many cancers, as a chemotherapeutic target, MAOs have value in immunological and anticancer therapies (55). Therefore, MAOB may hold informative significance in the pathogenesis and prognosis of GC (Figure 8). The analysis of scRNA-seq data demonstrated that MAOB expression is increased in EGC endothelial cells. Moreover, the gastrointestinal tract contains many neurotransmitters, and MAOB is a major metabolizing enzyme of these neurotransmitters and a great immunotherapy target (56). Some TCM drugs can also be used for cancer treatment targeting MAOB; for instance, danshensu can reduce MAOB activity and attenuate NF-κB signaling significantly, thus causing radiosensitization in non-small cell lung cancer (57).
PDE2A demonstrates low expression in various cancers (58). In the current study, PDE2A gene expression was significantly lower in the STAD tumor samples than in the normal samples (Figure 8A), was implicated in the metabolic pathways of glycine, serine, and threonine (Table S3). Its role in cancer progression may depend on its dual specificity for cAMP and cGMP, which regulate cell differentiation and DNA replication (59). In our findings, PDE2A was strongly expressed in endothelial cells; this result may be related to EMT progression in EGC (60). In addition, during T-cell activation, PDE2A activation may act as a feed-forward mechanism to the feedback NP/cGMP signaling, thereby reducing cAMP levels and maintaining T-cell activation, proliferation, and chemotactic effects (61).
Although AKR1C2, MAOB, and PDE2A exhibit mutation rates of 2.1%, 9%, and 6% in GC, respectively, these were comparatively lower than those of TP53 and Ras. Nonetheless, the differential expression of these genes in GC, as well as their association with OS, holed significant implications for the prediction and progression of GC.
Our research identified cimifugin as exhibiting moderate binding affinities (62) to three significant core targets—AKR1C2, MAOB, and PDE2A—with binding energies that ranged from −6.01 to −7.7 kcal·mol−1. Comparable binding energies were reported in similar studies, such as MAOB-crinamine at −8.20 kcal·mol−1, MAOB-haemanthidine at −7.7 kcal·mol−1, and MAOB-haemanthamine at −6.9 kcal·mol−1 (63). In the past, certain compounds associated with GC inflammation and carcinogenesis were demonstrated to have targeted and high-affinity binding. For instance, ellagic acid (EA) was known to target molecules such as TP53, JUN, and CASP3 in GC. MD simulations indicated that EA could effectively bind to key targets, inhibiting cancer promotion (64). Other compounds, such as quercetin, matrine, and ursolic acid, which targeted AKT1, TP53, epidermal growth factor, and CASP3 in GC, had shown promising binding and apoptosis-inducing capabilities as revealed by Autodock Vina and MD simulations (65). These studies demonstrate the binding profiles of various compounds with important molecules and three significant core targets in GC, providing a reference for the results of cimifugin and laying the groundwork for further validation. Our study revealed the metabolic profile of the EGC TME and identified key cimifugin pathways and targets influencing epithelial, immune, and stromal cell functions related to EGC cell growth and movement. We also confirmed cimifugin’s anti-cancer effects in GC cells and predicted its metabolic reprogramming role using network pharmacology, with plans for future studies to experimentally validate these targets.
Conclusions
Our results indicated that cimifugin inhibits GC cell proliferation, invasion, and migration. It may also regulate glutathione, histidine, phenylalanine, steroid hormone, and glycerolipid metabolism, folate biosynthesis, and 15 other EGC-related metabolic reprogramming processes. Target-regulated AKR1C2, MAOB, and PDE2A are strongly expressed in endocrine cells, CMPs, and PMCs, respectively; they might have potential diagnostic and prognostic clinical significance and provide insight into the pharmacological and clinical use of cimifugin. This study provides a novel basis for the development of metabolic reprogramming targets in EGC in TME. However, the current results warrant further validation.
Acknowledgments
We would like to thank the native English-speaking scientists of Elixigen Company (Huntington Beach, CA, USA) for editing our manuscript.
Funding: This work was supported by
Footnote
Reporting Checklist: The authors have completed the MDAR reporting checklist. Available at https://jgo.amegroups.com/article/view/10.21037/jgo-24-413/rc
Data Sharing Statement: Available at https://jgo.amegroups.com/article/view/10.21037/jgo-24-413/dss
Peer Review File: Available at https://jgo.amegroups.com/article/view/10.21037/jgo-24-413/prf
Conflicts of Interest: All authors have completed the ICMJE uniform disclosure form (available at https://jgo.amegroups.com/article/view/10.21037/jgo-24-413/coif). All authors report that this work was funded by the Innovation Project of Guangxi Graduate Education of GXUCM (No. YCBXJ2023018), the Education Department of Guangxi Zhuang Autonomous Region, China (No. 2020KY07012), the Administration of Traditional Chinese Medicine of Guangxi Zhuang Autonomous Region, China (No. GZZC2020094), the Top Talents Program of Guangxi University of Chinese Medicine, China (No. 2022C023), and the Natural Science Foundation of Guangxi Zhuang Autonomous Region (CN) (No. AD19245168). The authors have no other conflicts of interest to declare.
Ethical Statement: The authors are accountable for all aspects of the work in ensuring that questions related to the accuracy or integrity of any part of the work are appropriately investigated and resolved. The study was conducted in accordance with the Declaration of Helsinki (as revised in 2013).
Open Access Statement: This is an Open Access article distributed in accordance with the Creative Commons Attribution-NonCommercial-NoDerivs 4.0 International License (CC BY-NC-ND 4.0), which permits the non-commercial replication and distribution of the article with the strict proviso that no changes or edits are made and the original work is properly cited (including links to both the formal publication through the relevant DOI and the license). See: https://creativecommons.org/licenses/by-nc-nd/4.0/.
References
- Conti CB, Agnesi S, Scaravaglio M, et al. Early Gastric Cancer: Update on Prevention, Diagnosis and Treatment. Int J Environ Res Public Health 2023;20:2149. [Crossref] [PubMed]
- Guan WL, He Y, Xu RH. Gastric cancer treatment: recent progress and future perspectives. J Hematol Oncol 2023;16:57. [Crossref] [PubMed]
- Zhao L, Liu Y, Zhang S, et al. Impacts and mechanisms of metabolic reprogramming of tumor microenvironment for immunotherapy in gastric cancer. Cell Death Dis 2022;13:378. [Crossref] [PubMed]
- Patel DK. Biological importance of bioactive phytochemical ‘Cimifugin’as potential active pharmaceutical ingredients against human disorders: A natural phytochemical for new therapeutic alternatives. Pharmacological Research-Modern Chinese Medicine 2023;7:100232. [Crossref]
- Deng X, Liu Z, Han S. Cimifugin inhibits adipogenesis and TNF-α-induced insulin resistance in 3T3-L1 cells. Open Med (Wars) 2023;18:20230855. [Crossref] [PubMed]
- Chen KJ, Xie YH, Liu Y. Profiles of traditional Chinese medicine schools. Chin J Integr Med 2012;18:534-8. [Crossref] [PubMed]
- Yang ZC, Ma J. Actein enhances TRAIL effects on suppressing gastric cancer progression by activating p53/Caspase-3 signaling. Biochem Biophys Res Commun 2018;497:1177-83. [Crossref] [PubMed]
- Fatima S, Verma M, Ansari IA. Phytochemistry and ethnopharmacological studies of genus Cimicifuga: A systematic and comprehensive review. Fitoterapia 2024;172:105767. [Crossref] [PubMed]
- Zhang Y, Wang D, Peng M, et al. Single-cell RNA sequencing in cancer research. J Exp Clin Cancer Res 2021;40:81. [Crossref] [PubMed]
- Zhou Z, Chen B, Chen S, et al. Applications of Network Pharmacology in Traditional Chinese Medicine Research. Evid Based Complement Alternat Med 2020;2020:1646905. [Crossref] [PubMed]
- Zhang WY, Liu YJ, He Y, et al. Suppression of long noncoding RNA NCK1-AS1 increases chemosensitivity to cisplatin in cervical cancer. J Cell Physiol 2019;234:4302-13. [Crossref] [PubMed]
- Zhou M, Xiao L, Jin J, et al. Role of p53/circRNA0085439/Ku70 axis in DNA damage response in lung cells exposed to ZnO nanoparticles: Involvement of epigenetic regulation. Cancer Nanotechnology 2023;14:42. [Crossref]
- Hieda M, Matsuura N, Kimura H. Histone modifications associated with cancer cell migration and invasion. Methods Mol Biol 2015;1238:301-17. [Crossref] [PubMed]
- Goldman MJ, Craft B, Hastie M, et al. Visualizing and interpreting cancer genomics data via the Xena platform. Nat Biotechnol 2020;38:675-8. [Crossref] [PubMed]
- Hao Y, Hao S, Andersen-Nissen E, et al. Integrated analysis of multimodal single-cell data. Cell 2021;184:3573-3587.e29. [Crossref] [PubMed]
- Aran D, Looney AP, Liu L, et al. Reference-based analysis of lung single-cell sequencing reveals a transitional profibrotic macrophage. Nat Immunol 2019;20:163-72. [Crossref] [PubMed]
- Zhang X, Lan Y, Xu J, et al. CellMarker: a manually curated resource of cell markers in human and mouse. Nucleic Acids Res 2019;47:D721-8. [Crossref] [PubMed]
- Jin S, Guerrero-Juarez CF, Zhang L, et al. Inference and analysis of cell-cell communication using CellChat. Nat Commun 2021;12:1088. [Crossref] [PubMed]
- Wu Y, Yang S, Ma J, et al. Spatiotemporal Immune Landscape of Colorectal Cancer Liver Metastasis at Single-Cell Level. Cancer Discov 2022;12:134-53. [Crossref] [PubMed]
- Kim S, Chen J, Cheng T, et al. PubChem 2023 update. Nucleic Acids Res 2023;51:D1373-80. [Crossref] [PubMed]
- Daina A, Michielin O, Zoete V. SwissTargetPrediction: updated data and new features for efficient prediction of protein targets of small molecules. Nucleic Acids Res 2019;47:W357-64. [Crossref] [PubMed]
- Keiser MJ, Roth BL, Armbruster BN, et al. Relating protein pharmacology by ligand chemistry. Nat Biotechnol 2007;25:197-206. [Crossref] [PubMed]
- Nickel J, Gohlke BO, Erehman J, et al. SuperPred: update on drug classification and target prediction. Nucleic Acids Res 2014;42:W26-31. [Crossref] [PubMed]
- Gong J, Cai C, Liu X, et al. ChemMapper: a versatile web server for exploring pharmacology and chemical structure association based on molecular 3D similarity method. Bioinformatics 2013;29:1827-9. [Crossref] [PubMed]
- Ko J, Park H, Heo L, et al. GalaxyWEB server for protein structure prediction and refinement. Nucleic Acids Res 2012;40:W294-7. [Crossref] [PubMed]
- Wang X, Shen Y, Wang S, et al. PharmMapper 2017 update: a web server for potential drug target identification with a comprehensive target pharmacophore database. Nucleic Acids Res 2017;45:W356-60. [Crossref] [PubMed]
- UniProt: the Universal Protein Knowledgebase in 2023. Nucleic Acids Res 2023;51:D523-31. [Crossref] [PubMed]
- Myung Y, de Sá AGC, Ascher DB. Deep-PK: deep learning for small molecule pharmacokinetic and toxicity prediction. Nucleic Acids Res 2024;52:W469-75. [Crossref] [PubMed]
- Love MI, Huber W, Anders S. Moderated estimation of fold change and dispersion for RNA-seq data with DESeq2. Genome Biol 2014;15:550. [Crossref] [PubMed]
- Szklarczyk D, Morris JH, Cook H, et al. The STRING database in 2017: quality-controlled protein-protein association networks, made broadly accessible. Nucleic Acids Res 2017;45:D362-8. [Crossref] [PubMed]
- Shannon P, Markiel A, Ozier O, et al. Cytoscape: a software environment for integrated models of biomolecular interaction networks. Genome Res 2003;13:2498-504. [Crossref] [PubMed]
- Ogata H, Goto S, Sato K, et al. KEGG: Kyoto Encyclopedia of Genes and Genomes. Nucleic Acids Res 1999;27:29-34. [Crossref] [PubMed]
- Yu G, Wang LG, Han Y, et al. clusterProfiler: an R package for comparing biological themes among gene clusters. OMICS 2012;16:284-7. [Crossref] [PubMed]
- Sjöstedt E, Zhong W, Fagerberg L, et al. An atlas of the protein-coding genes in the human, pig, and mouse brain. Science 2020;367:eaay5947. [Crossref] [PubMed]
- Tang Z, Kang B, Li C, et al. GEPIA2: an enhanced web server for large-scale expression profiling and interactive analysis. Nucleic Acids Res 2019;47:W556-60. [Crossref] [PubMed]
- Gao J, Aksoy BA, Dogrusoz U, et al. Integrative analysis of complex cancer genomics and clinical profiles using the cBioPortal. Sci Signal 2013;6:pl1. [Crossref] [PubMed]
- Burley SK, Bhikadiya C, Bi C, et al. RCSB Protein Data Bank (RCSB.org): delivery of experimentally-determined PDB structures alongside one million computed structure models of proteins from artificial intelligence/machine learning. Nucleic Acids Res 2023;51:D488-508. [Crossref] [PubMed]
- Eberhardt J, Santos-Martins D, Tillack AF, et al. AutoDock Vina 1.2.0: New Docking Methods, Expanded Force Field, and Python Bindings. J Chem Inf Model 2021;61:3891-8. [Crossref] [PubMed]
- Adasme MF, Linnemann KL, Bolz SN, et al. PLIP 2021: expanding the scope of the protein-ligand interaction profiler to DNA and RNA. Nucleic Acids Res 2021;49:W530-4. [Crossref] [PubMed]
- Schrödinger, LLC. The PyMOL Molecular Graphics System. Version 2.5.6.
- Páll S, Zhmurov A, Bauer P, et al. Heterogeneous parallelization and acceleration of molecular dynamics simulations in GROMACS. J Chem Phys 2020;153:134110. [Crossref] [PubMed]
- Ju Z, Shao J, Zhou M, et al. Transcriptomic and metabolomic profiling reveal the p53-dependent benzeneacetic acid attenuation of silica-induced epithelial-mesenchymal transition in human bronchial epithelial cells. Cell Biosci 2021;11:30. [Crossref] [PubMed]
- Huang M, Wu Y, Cheng L, et al. Multi-omics analyses of glucose metabolic reprogramming in colorectal cancer. Front Immunol 2023;14:1179699. [Crossref] [PubMed]
- He L, Zhao J, Li H, et al. Metabolic Reprogramming of NK Cells by Black Phosphorus Quantum Dots Potentiates Cancer Immunotherapy. Adv Sci (Weinh) 2023;10:e2202519. [Crossref] [PubMed]
- Duwaerts CC, Amin AM, Siao K, et al. Specific Macronutrients Exert Unique Influences on the Adipose-Liver Axis to Promote Hepatic Steatosis in Mice. Cell Mol Gastroenterol Hepatol 2017;4:223-36. [Crossref] [PubMed]
- Lu X, Wu N, Yang W, et al. OGDH promotes the progression of gastric cancer by regulating mitochondrial bioenergetics and Wnt/β-catenin signal pathway. Onco Targets Ther 2019;12:7489-500. [Crossref] [PubMed]
- Su P, Wang Q, Bi E, et al. Enhanced Lipid Accumulation and Metabolism Are Required for the Differentiation and Activation of Tumor-Associated Macrophages. Cancer Res 2020;80:1438-50. [Crossref] [PubMed]
- Wang KC, Chang JS, Lin LT, et al. Antiviral effect of cimicifugin from Cimicifuga foetida against human respiratory syncytial virus. Am J Chin Med 2012;40:1033-45. [Crossref] [PubMed]
- Park J, Kim DK. Effects of cimifugin on cell growth inhibition and cell apoptosis induction in fadu human pharyngeal squamous cell carcinoma. Oral Biol Res 2022;46:165-70. [Crossref]
- Lee TY, Chiang EP, Shih YT, et al. Lower serum folate is associated with development and invasiveness of gastric cancer. World J Gastroenterol 2014;20:11313-20. [Crossref] [PubMed]
- Giacomini I, Gianfanti F, Desbats MA, et al. Cholesterol Metabolic Reprogramming in Cancer and Its Pharmacological Modulation as Therapeutic Strategy. Front Oncol 2021;11:682911. [Crossref] [PubMed]
- Li S, Lee W, Heo W, et al. AKR1C2 Promotes Metastasis and Regulates the Molecular Features of Luminal Androgen Receptor Subtype in Triple Negative Breast Cancer Cells. J Breast Cancer 2023;26:60-76. [Crossref] [PubMed]
- Liu W, Zhang F, Yang K, et al. Comprehensive analysis regarding the prognostic significance of downregulated ferroptosis-related gene AKR1C2 in gastric cancer and its underlying roles in immune response. PLoS One 2023;18:e0280989. [Crossref] [PubMed]
- Park JH, Ju YH, Choi JW, et al. Newly developed reversible MAO-B inhibitor circumvents the shortcomings of irreversible inhibitors in Alzheimer's disease. Sci Adv 2019;5:eaav0316. [Crossref] [PubMed]
- Aljanabi R, Alsous L, Sabbah DA, et al. Monoamine Oxidase (MAO) as a Potential Target for Anticancer Drug Design and Development. Molecules 2021;26:6019. [Crossref] [PubMed]
- Wang Y, Wang S, Yang Q, et al. Norepinephrine Enhances Aerobic Glycolysis and May Act as a Predictive Factor for Immunotherapy in Gastric Cancer. J Immunol Res 2021;2021:5580672. [Crossref] [PubMed]
- Son B, Jun SY, Seo H, et al. Inhibitory effect of traditional oriental medicine-derived monoamine oxidase B inhibitor on radioresistance of non-small cell lung cancer. Sci Rep 2016;6:21986. [Crossref] [PubMed]
- Li SZ, Ren KX, Zhao J, et al. miR-139/PDE2A-Notch1 feedback circuit represses stemness of gliomas by inhibiting Wnt/β-catenin signaling. Int J Biol Sci 2021;17:3508-21. [Crossref] [PubMed]
- Monterisi S, Lobo MJ, Livie C, et al. PDE2A2 regulates mitochondria morphology and apoptotic cell death via local modulation of cAMP/PKA signalling. Elife 2017;6:e21374. [Crossref] [PubMed]
- Sadeghi M, Karimi MR, Karimi AH, et al. Network-Based and Machine-Learning Approaches Identify Diagnostic and Prognostic Models for EMT-Type Gastric Tumors. Genes (Basel) 2023;14:750. [Crossref] [PubMed]
- Kurelic R, Krieg PF, Sonner JK, et al. Upregulation of Phosphodiesterase 2A Augments T Cell Activation by Changing cGMP/cAMP Cross-Talk. Front Pharmacol 2021;12:748798. [Crossref] [PubMed]
- Saini N, Grewal AS, Lather V, et al. Natural alkaloids targeting EGFR in non-small cell lung cancer: Molecular docking and ADMET predictions. Chem Biol Interact 2022;358:109901. [Crossref] [PubMed]
- Naidoo D, Roy A, Slavětínská LP, et al. New role for crinamine as a potent, safe and selective inhibitor of human monoamine oxidase B: In vitro and in silico pharmacology and modeling. J Ethnopharmacol 2020;248:112305. [Crossref] [PubMed]
- Liu Z, Huang H, Yu Y, et al. Exploring the mechanism of ellagic acid against gastric cancer based on bioinformatics analysis and network pharmacology. J Cell Mol Med 2023;27:3878-96. [Crossref] [PubMed]
- Wang Y, Wang H, Xu S. Natural Bioactive Compounds Promote Cell Apoptosis in Gastric Cancer Treatment: Evidence from Network Pharmacological Study and Experimental Analysis. Journal of Chemistry 2023;2023:6316589. [Crossref]
(English Language Editor: J. Jones)