Single-cell analysis of chemotherapy-resistant microenvironment identifies a chemo-response biomarker for pancreatic cancer
Highlight box
Key findings
• We found the potential important role of myeloid cells, natural killer cells, and erythroid cells in chemotherapy-resistant pancreatic ductal adenocarcinoma (PDAC).
• We developed a tumor microenvironment (TME)-based random forest model predicting chemotherapy response and patient prognosis.
What is known and what is new?
• TME plays a crucial role in chemoresistance. Genetic mutations in PDAC contribute to chemotherapy resistance.
• Comprehensive single-cell analysis identifies specific cell infiltrations linked to chemoresistance. Novel differentially expressed genes and signaling pathways involved in chemoresistance are characterized. A predictive model using TME-signatures offers a new tool for independently anticipating chemotherapy response and patient outcomes.
What is the implication, and what should change now?
• Future research should focus on validating TME-based predictive models in clinical trials and exploring therapeutic interventions aimed at modulating the TME.
Introduction
Pancreatic ductal adenocarcinoma (PDAC) is one of the most lethal types of cancer, with a low survival rate and high mortality rate (1). The treatment of PDAC typically involves a combination of therapies, including surgery and chemotherapy. However, due to late-stage diagnosis and metastases, many patients are not eligible for surgery. As a result, chemotherapy is widely used as a primary treatment option (2). Unfortunately, chemotherapy resistance is a critical problem in the treatment of PDAC, which hinders the cure of this disease.
Despite advances in cancer genomics, our understanding of the underlying mechanisms of chemotherapy resistance is limited. Previous research has revealed that the complex heterogeneity of PDAC contributes to chemotherapy resistance (3,4). For example, PDAC cells develop distinct genetic mutations that allow them to become resistant to chemotherapy drugs (5). Fiorini et al. proposed that TP53 mutations can drive the chemo-resistance to gemcitabine in PDAC cell lines (6). Patient-derived cell lines and xenografts tumors containing a mutation in STAG2 (stromal antigen 2) showed sensitivity to DNA cross-linking agents (7). Despite the advanced role of genetic mutations in drug resistance, the mutations are in the tumor, and tumor develops resistance.
The development of single-cell RNA sequencing (scRNA-seq) has revolutionized our understanding of cellular heterogeneity within the tumor microenvironment (TME) (8). Tumor genotype has been inefficient in predicting response to anticancer therapy, indicating that chemoresistance may be driven by TME. In gastric cancer, a high number of tumor-associated macrophages before treatment correlated with prolonged survival in patients who received 5-fluorouracil (FU)-based postoperative chemotherapy (9). A study on PDAC found that chemotherapy inhibited immunosuppressive TME reprogramming by downregulating TIGIT on CD8+ T cells and disrupting their interactions with cancer cells (10). Another study in the same context identified additional mediators of chemotherapy-induced immunostimulation, such as an enrichment of inflammatory cancer-associated fibroblasts and an increase in CD8+ T cells (11). Similarly, in esophageal adenocarcinoma, chemotherapy led to a higher ratio of effector CD8+ T cells to regulatory CD4+ T cells in the TME (12). Conversely, in other cancer types like stomach adenocarcinoma, chemotherapy had immunosuppressive effects, reducing CD4+ and CD8+ T cells, increasing endothelial cells and fibroblasts, and activating proangiogenic pathways in cancer cells (13). Despite the important role of TME cells in chemoresistance, there is still a lack of unbiased single-cell analysis to comprehensively reveal cell types which are involved in chemoresistance.
In this study, we hypothesized that the dynamic alteration of cancer TME is a crucial factor in chemoresistance. Therefore, we performed single-cell analysis for chemotherapy-treated PDAC. Through comparing differences at cellular and molecular levels, transcriptomic features of chemoresistant TME cells could be a promising signature for predicting chemotherapy response and survival of patients. We present this article in accordance with the REMARK reporting checklist (available at https://jgo.amegroups.com/article/view/10.21037/jgo-24-93/rc).
Methods
PDAC patient cohorts and subject details
This study is a retrospective study including patients diagnosed with PDAC from January 1, 2010, to December 31, 2018. The transcriptome data were downloaded from publicly available databases, including The Cancer Genome Atlas (TCGA), International Cancer Genome Consortium (ICGC), and Gene Expression Omnibus (GEO) (Table 1). Survival data and chemotherapy information were obtained from corresponding database. Samples with chemotherapy response data were obtained. For samples with multiple rounds of gemcitabine treatments, the first response label was used to further analysis. According to Fig. 1 and Supplementary Tab. 2 from the original article by Werba et al., seven patients have drug response labels. This article conducted a single-cell analysis on this group of samples. The complete response (CR) and partial response (PR) labels were regarded as chemo-sensitivity, while stable disease (SD) and progressive disease (PD) labels were chemoresistance (10).
Table 1
Source | Dataset | Sample size | Link |
---|---|---|---|
TCGA | TCGA-PAAD | 146 | https://portal.gdc.cancer.gov/ |
GEO | GSE62452 | 65 | https://www.ncbi.nlm.nih.gov/geo/query/acc.cgi?acc=GSE62452 |
GEO | GSE57495 | 63 | https://www.ncbi.nlm.nih.gov/geo/query/acc.cgi?acc=GSE57495 |
GEO | GSE28735 | 45 | https://www.ncbi.nlm.nih.gov/geo/query/acc.cgi?acc=GSE28735 |
GEO | GSE71729 | 125 | https://www.ncbi.nlm.nih.gov/geo/query/acc.cgi?acc=GSE71729 |
GEO | GSE17891 | 19 | https://www.ncbi.nlm.nih.gov/geo/query/acc.cgi?acc=GSE17891 |
ICGC | PACA-AU | 242 | https://daco.icgc-argo.org/ |
ICGC | PACA-CA | 213 | https://daco.icgc-argo.org/ |
TCGA, The Cancer Genome Atlas; PAAD, pancreatic adenocarcinoma; GEO, Gene Expression Omnibus; ICGC, International Cancer Genome Consortium.
To ensure the independence of TME-signatures, stratification was employed based on the mutation or copy number variance of known driver genes (CDKN2A, KRAS, TTN, TP53). This study was approved by the Ethics Review Committee of the Second Affiliated Hospital of Harbin Medical University. The study was conducted in accordance with the Declaration of Helsinki (as revised in 2013).
Preprocessing of gene expression profile
mRNA from samples were quantified using RNA-sequencing or microarray. For microarray platform, the probe-level expression values were transformed into gene-level according to matched platform annotation. Multiple probe mapping to the same gene values were averaged to represent the gene expression. Probes were removed if multiple genes were mapped to the same probe.
Single cell analysis
We used the NormalizeData function to normalize raw counts and the FindVariableFeatures function to select highly variable genes in the R package ’seurat4’ (V4.3.0) to address the batch effect and tissue specificity of scRNA-seq data. Principal component analysis (PCA) was performed for dimensionality reduction based on the highly variable genes. We used established cell markers from previous studies to probe for cell types. For cell types with differential fraction, we performed cell-cell communication analysis using the R package ‘cellchat’.
Functional enrichment analysis
Functional enrichment analysis of differentially expressed genes (DEGs) was performed using the R package ‘clusterProfiler’ (version 4.0.5). Subsequently, the enrichment scores of the macrophage phenotype for each cell were calculated using single sample gene set enrichment analysis (ssGSEA). Genes that involved in two macrophage phenotypes were downloaded from He et al. (14).
Random forest model and validation
We applied the randomForest and varSelRF R packages to model random forest classification to predict chemotherapeutic response based on cell proportion and DEGs. The candidate features were composited of two parts: the fraction of cell types from myeloid cells, natural killer (NK) cells, and erythroid as well as DEGs. To enhance the robustness and avoid overfitting, we randomly split the TCGA PDAC dataset into two groups: a training dataset including 70% of samples and a validation dataset including 30%. Then we performed parameter tuning to train ‘ntree’ and ‘mtry’ for the random forest model. The two parameters were iterated from 10 to 800 and 1 to 50, respectively. Afterward, the model with the highest accuracy was returned as the optimal solution. Finally, the pROC package was used to plot receiver operating characteristic (ROC) and to calculate the area under the ROC curve (AUC). ROC curve is a performance measurement for the classification problems at various threshold settings, which is plotted with true-positive rates against the false-positive rates. The higher AUC indicates a better performance.
Cell type deconvolution with BayesPrism
The scRNA-seq data GSE205013 was used as the reference cell type-specific expression profile to deconvolution TCGA PDAC dataset. Ribosomal, sex chromosomal, and mitochondrial genes as well as lncRNA MALAT1, or genes which expressed in less than 1,000 cells were removed. Cell types having at least 1,000 cells remained. Furthermore, DEGs among cell types were chosen as marker genes for deconvolution. Bulk gene expression profiles at the count level of TCGA PDAC were download and loaded to the package ‘BayesPrism’.
Statistical analysis
The t-tests and Wilcoxon tests were used to assess group differences. Kaplan-Meier method was employed to estimate overall survival (OS) and progression-free survival (PFS) curves, which were compared between groups using the log-rank test. Hazard ratios (HRs) were calculated with the Cox proportional hazards model. A P value of less than 0.05 was considered statistically significant. All data analyses were conducted using R version 4.3.3.
Results
Increased infiltration of cells of innate immunity system in chemotherapy-resistant patients
In total, 274,070 cells were clustered and annotated using canonical lineage markers (Figure 1A,1B). Using the RECIST criteria, two treated patients were categorized as SD (P08 and P10), two partial response (P03 and P14), three PD (P06, P11, and P12). Interestingly, we found that myeloid cells, NK cells, and erythroid cells had higher fractions in chemotherapy-resistant patients (Figure 1C). In chemotherapy-treated patients, NK cells were further clustered into two subsets (Figure 1D). The first NK subset highly expressed cytotoxic factors (FGFBP2, GZMH, and PRF1) and FAGR3A, which is characteristic of the CD56dim NK cell subset. The second NK subset displayed upregulation of soluble factors (XCL1, XCL2, GZMK, and CCL3L1) associated with NK cell effector functions (Figure 1E). For myeloid cells, we identified six distinct myeloid clusters (Figure 1F). Macrophages representing the majority of myeloid cells, clustered into SPP1+ and C1QC+ subsets. Myeloid-derived suppressor cells (MDSCs), conventional dendritic cells (cDCs), and proliferating myeloid cells were also identified by canonical markers including S100A8, S100A9, FCER1A, CD1C, STMN1, and MKI67 (Figure 1E). For myeloid subsets, we found a significant increase in infiltration of MDSC in chemo-resistant patients.
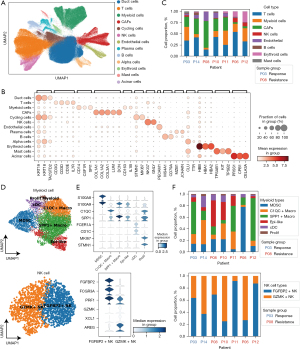
Activated signaling pathway of cells in chemotherapy-resistant TME
We then characterized the molecular response of enriched cell types in chemotherapy-resistance microenvironment. In total, 1,632, 1,459, and 554 genes were identified as DEGs for myeloid and NK cells. TGFB1 and FGFBP2 were significantly upregulated in chemo-resistant patients, indicating an expansion of FGFBP2+ NK subset (Figure 2A). These upregulated genes were enriched in TGF-β, IL-6, and TNF signaling functions, while the functions of Fc region receptor and Toll-like receptor were downregulated (Figure 2B). For chemo-resistant myeloid cells, VEGF1 and TGFB1 genes were upregulated, while MHC class II genes were downregulated (Figure 2C). Similarly, downregulated genes were also significantly involved in the function of antigens presentation, indicating a dysregulated status of macrophages in chemo-resistant patients (Figure 2D). The macrophages in chemoresistance showed significantly higher M2-like signatures (P<0.05, Figure 2E). Furthermore, we found that SPP1+ macrophages showed more interactions between tumor cells by inducing TNF-alpha and IL6-STAT3 signaling of tumor cells. NK cells upregulated IL10 and interacted tumor cells expressing IL10 receptor. The receptor-ligand interaction analysis indicated that macrophages upregulated IL6 expression and interacted tumor cells which express IL6RA (P<0.01, Figure 2F).
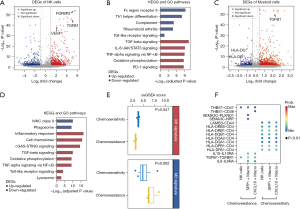
TME-signatures predicted chemotherapy response in bulk expression data
To predict the response of chemotherapy for patients, we conducted a random forest model to classify samples based on fractions and DEGs of cell subsets of myeloid, NK, and erythroid cells. According to the parameter training grid, the best parameters of ‘mtry’ and ‘ntree’ were chosen as 3 and 700, respectively (Figure 3A). Then we further performed five-fold cross-validation and Figure 3B showed the distribution of the mean error of cross-validation. Top 10 candidate features with minimum errors were obtained as final features. In the ROC curve analysis, using the best parameters and 10 features, the model reached a max AUC of 0.94 (Figure 3C). In the TCGA test dataset, 94% CR and 84% PR patients were predicted as chemosensitivity, while 96% SD and 98% PD were chemoresistance. The chemo-resistant samples predicted by the forest model had higher fractions of erythroid cell, NK cell, and macrophage (P<0.05, Wilcoxon rank-sum test, Figure 3D). Various types of CD4+ T-cells were also consistently highly infiltrated in chemoresistance, including memory and follicular CD4+ T-cells. Moreover, chemo-resistant samples displayed higher expression of TNF-alpha pathway genes, including TNFRSF4 and TNFSF18 (P<0.05, Wilcoxon rank-sum test, Figure 3E).
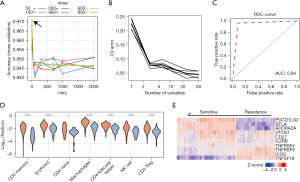
Validation of the prognosis value of TME-signature in independent datasets
Patients classified by TME-signature as chemo-resistant showed significantly poorer OS than chemo-sensitive patients in chemotherapy-treated TCGA group (P=2.5e−02, log-rank test, Figure 4A). Furthermore, in independent PDAC samples from GEO and ICGC, the Kaplan-Meier survival curve showed poorer OS in predicted chemo-resistant samples classified by the TME-signature (GSE71729: P=7.9e−03; GSE28735: P=5.6e−02; GSE57495: P=1.2e−02; GSE62452: P=7.5e−02; PACA-CA: P=2.3e−03; GSE17891: P=2.1e−02; PACA-AU: P=2.3e−05; log-rank test, Figure 4B-4H). The univariate Cox hazards analysis showed that elevated level of the TME-signature was significantly associated with reduced survival in pancreatic cancer patients [HR =2.35, 95% confidence interval (CI): 1.55–2.62, P<0.001]. The multivariate Cox analysis confirmed that elevated levels of the TME-signature are associated with poorer survival outcomes, independent of age and stage (HR =1.45, 95% CI: 1.25–1.62, P<0.001).
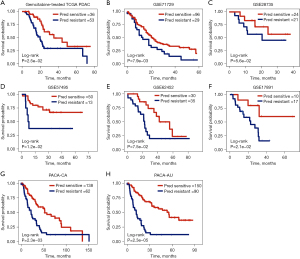
TME-signature predicted chemoresistance independently of PDAC genetic mutations
In order to confirm the independence of the TME-signature, we grouped TCGA PDAC patients by somatic mutations and copy number alterations of top five genes with highest frequencies, including KRAS, TP53, CDKN2A, and TTN. Patients with mutated KRAS and TP53 had worse prognosis than the wild-type group, respectively (P<0.05, Log-rank test). Similarly, amplified CDKN2A and TTN deletion showed positive correlation with patients’ survival (P<0.05, Log-rank test). TP53 and KRAS were frequently mutated in PD and SD patients and had a co-alteration relationship in PDAC patients (P<0.05, hypergeometric test, Figure 5A). For prognosis-related genomic alterations, we found that predicted chemo-sensitive patients had better prognosis than predicted chemo-sensitive in wild-type and mutated groups, respectively (P<0.05, Log-rank test, Figure 5B-5E).
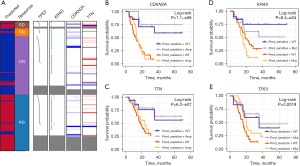
Discussion
Chemoresistance is a leading cause of morbidity and mortality in PDAC and it continues to be a challenge in cancer treatment. The dynamic alteration of TME is a key role in chemoresistance. In this regard, we analyzed comprehensively TME and DEGs among treated patients by scRNA-seq data. We found that myeloid cells, NK cells, and erythroid cells were highly infiltrated in chemo-resistant patients. Signaling pathways including TGF-β and IFN in myeloid and NK cells were upregulated in chemo-resistant patients. By producing IFN-γ, macrophages and NK cells may induce tumor cells to express STAT3 and induce epithelia-mesenchymal transition, which promote the chemoresistance of tumor cells. We developed a TME-signature for therapeutic response of chemotherapy predicted accurately survival and response based on bulk transcriptome. The TME-based signature for chemoresistance may have value in improving the clinical survival of PDAC patients.
Chemoresistance and chemotherapy-induced immunosuppression can result in the relapse of tumors and is critical for survival in cancer patients. We found that myeloid cells, particularly macrophages, generally accumulate in chemo-resistant tumors. The increased infiltration of myeloid cells and erythroblasts in chemo-resistant patients is particularly interesting. Erythroblasts were removed at single-cell analysis in many studies, which may limit the discovery of potential role in cancer progression (10,15). However, the evaluated erythroblasts fraction in chemoresistance implicated their potential importance by our study. Furthermore, the macrophages had higher M2 signature, indicating a M2 phenotype which has been reported in tumor progression (16).
We found that M2 macrophages downregulated the MHC-II function and highly expressed genes involved in TGF-β and IFN-related pathways. The M2 macrophages also showed higher IL-6 and STAT3 signaling pathways. It is well-known that IFNγ typically activates the transcription factor STAT1, while high expression of IL-6 activates the STAT3 signaling pathway. However, Yoyen-Ermis et al. found that in acute myeloid leukemia, IFNγ can inhibit the expression of immune checkpoint genes such as PD-L1 through the activation of a non-classical STAT3 signaling pathway (17). Additionally, Qing and Stark observed prolonged activation of STAT3 signaling in embryonic fibroblasts from Stat1-Null mice compared to wild-type mice (18). Our results suggest the presence of aberrant non-classical regulatory pathways in macrophages within the TME.
The activated signaling pathways in the TME of chemo-resistant patients may also be contributing to chemotherapy resistance. TGF-β, IL-6, and TNF are all cytokines that can promote tumor growth and metastasis. The activation of these pathways in the TME of chemo-resistant patients may be providing the tumor with the growth factors and signaling molecules it needs to resist chemotherapy.
In addition to the findings discussed above, the study also found that the TME-signature predicted chemotherapy response in bulk expression data and in independent datasets. This suggests that the TME-signature may be a useful tool for predicting chemotherapy response in PDAC patients. When patients were classified with different genetic alterations, the TME-signature still showed a good performance in survival prediction. In all wild-type PDAC patients, predicted chemo-sensitive patients showed better survival than chemo-resistant patients. These results confirmed the independence of our TME-signature, which could predict the response regardless of the genetic status of cancer cells. It is worth noted that patients with KRAS mutation still had worse survival in both predicted sensitive and resistant groups, which implicated a better performance when combining the KRAS mutation and TME-signature. However, further research is needed to validate the use of the TME-signature in clinical trials.
Conclusions
Overall, the findings of this study provide new insights into the role of the TME in chemotherapy resistance in PDAC. These findings suggest that the TME may be a promising target for therapeutic intervention in PDAC patients.
Acknowledgments
Funding: None.
Footnote
Reporting Checklist: The authors have completed the REMARK reporting checklist. Available at https://jgo.amegroups.com/article/view/10.21037/jgo-24-93/rc
Peer Review File: Available at https://jgo.amegroups.com/article/view/10.21037/jgo-24-93/prf
Conflicts of Interest: All authors have completed the ICMJE uniform disclosure form (available at https://jgo.amegroups.com/article/view/10.21037/jgo-24-93/coif). The authors have no conflicts of interest to declare.
Ethical Statement: The authors are accountable for all aspects of the work in ensuring that questions related to the accuracy or integrity of any part of the work are appropriately investigated and resolved. This study was approved by the Ethics Review Committee of the Second Affiliated Hospital of Harbin Medical University. The study was conducted in accordance with the Declaration of Helsinki (as revised in 2013).
Open Access Statement: This is an Open Access article distributed in accordance with the Creative Commons Attribution-NonCommercial-NoDerivs 4.0 International License (CC BY-NC-ND 4.0), which permits the non-commercial replication and distribution of the article with the strict proviso that no changes or edits are made and the original work is properly cited (including links to both the formal publication through the relevant DOI and the license). See: https://creativecommons.org/licenses/by-nc-nd/4.0/.
References
- Siegel RL, Miller KD, Fuchs HE, et al. Cancer Statistics, 2021. CA Cancer J Clin 2021;71:7-33. [PubMed]
- Traub B, Link KH, Kornmann M. Curing pancreatic cancer. Semin Cancer Biol 2021;76:232-46. [PubMed]
- Bailey P, Chang DK, Nones K, et al. Genomic analyses identify molecular subtypes of pancreatic cancer. Nature 2016;531:47-52. [PubMed]
- Hu HF, Ye Z, Qin Y, et al. Mutations in key driver genes of pancreatic cancer: molecularly targeted therapies and other clinical implications. Acta Pharmacol Sin 2021;42:1725-41. [PubMed]
- Gao C, Wisniewski L, Liu Y, et al. Detection of Chemotherapy-resistant Pancreatic Cancer Using a Glycan Biomarker, sTRA. Clin Cancer Res 2021;27:226-36. [PubMed]
- Fiorini C, Cordani M, Padroni C, et al. Mutant p53 stimulates chemoresistance of pancreatic adenocarcinoma cells to gemcitabine. Biochim Biophys Acta 2015;1853:89-100. [PubMed]
- Witkiewicz AK, Balaji U, Eslinger C, et al. Integrated Patient-Derived Models Delineate Individualized Therapeutic Vulnerabilities of Pancreatic Cancer. Cell Rep 2016;16:2017-31. [PubMed]
- Fomitcheva-Khartchenko A, Kashyap A, Geiger T, et al. Space in cancer biology: its role and implications. Trends Cancer 2022;8:1019-32. [PubMed]
- Dong X, Fan J, Xie W, et al. Efficacy evaluation of chimeric antigen receptor-modified human peritoneal macrophages in the treatment of gastric cancer. Br J Cancer 2023;129:551-62. [PubMed]
- Werba G, Weissinger D, Kawaler EA, et al. Single-cell RNA sequencing reveals the effects of chemotherapy on human pancreatic adenocarcinoma and its tumor microenvironment. Nat Commun 2023;14:797. [PubMed]
- Cui Zhou D, Jayasinghe RG, Chen S, et al. Spatially restricted drivers and transitional cell populations cooperate with the microenvironment in untreated and chemo-resistant pancreatic cancer. Nat Genet 2022;54:1390-405. [PubMed]
- Cidre-Aranaz F, Li J, Hölting TLB, et al. Integrative gene network and functional analyses identify a prognostically relevant key regulator of metastasis in Ewing sarcoma. Mol Cancer 2022;21:1. [PubMed]
- Wen G, Yao L, Hao Y, et al. Bilirubin ameliorates murine atherosclerosis through inhibiting cholesterol synthesis and reshaping the immune system. J Transl Med 2022;20:1. [PubMed]
- He L, Jhong JH, Chen Q, et al. Global characterization of macrophage polarization mechanisms and identification of M2-type polarization inhibitors. Cell Rep 2021;37:109955. [PubMed]
- Wang Y, Liang Y, Xu H, et al. Single-cell analysis of pancreatic ductal adenocarcinoma identifies a novel fibroblast subtype associated with poor prognosis but better immunotherapy response. Cell Discov 2021;7:36. [PubMed]
- Boutilier AJ, Elsawa SF. Macrophage Polarization States in the Tumor Microenvironment. Int J Mol Sci 2021;22:6995. [PubMed]
- Yoyen-Ermis D, Tunali G, Tavukcuoglu E, Horzum U, Ozkazanc D, Sutlu T, et al. Myeloid maturation potentiates STAT3-mediated atypical IFN-gamma signaling and upregulation of PD-1 ligands in AML and MDS. Sci Rep. 2019;9:11697. [PubMed]
- Wang W, Lopez McDonald MC, Kim C, et al. The complementary roles of STAT3 and STAT1 in cancer biology: insights into tumor pathogenesis and therapeutic strategies. Front Immunol 2023;14:1265818. [PubMed]