Liquid-liquid phase separation-related features of PYGB/ACTR3/CCNA2/ITGB1/ATP8A1/RAP1GAP2 predict the prognosis of pancreatic cancer
Highlight box
Key findings
• This study established a novel prognostic risk model comprising six liquid-liquid phase separation-related genes (LRGs) in pancreatic cancer (PC) using transcriptomic data and clinical information from publicly accessible databases and a Mendelian randomization analysis.
What is known and what is new?
• LRGs can serve as significant prognostic indicators of cancers.
• PYGB, ACTR3, CCNA2, and ITGB1 are associated with an elevated risk of developing PC. Conversely, ATP8A1, and RAP1GAP2 offer protection against PC.
What is the implication, and what should change now?
• This study examined the relationship between these LRGs and immune infiltration, the efficacy of immunotherapy, associated pathways, and drug responsiveness in PC patients. Our findings provide novel insights into predicting the prognosis of PC patients.
Introduction
Pancreatic cancer (PC) is an exceedingly lethal digestive tract malignancy with a rising global incidence (1). Due to the lack of effective systemic treatment strategies, the current 5-year survival rate for PC is only 12% (2). Most PC are adenocarcinomas, which are extremely difficult to prevent and diagnose early at a curable stage (3).
Genetic alterations play a pivotal role in the pathogenesis and progression of PC (4). Currently available treatment options for PC include surgery, chemotherapy, radiotherapy, targeted therapy, and combination therapy; however, surgery remains the primary curative therapeutic modality for this disease (5). Unfortunately, almost 50% of patients present with distant metastasis, which reduces the opportunity for surgical intervention (6,7). Moreover, the enormous clinical cost, coupled with the medical burden associated with PC, poses a significant global public health issue. Thus, there is an urgent imperative to identify biomarkers or personalized therapeutic targets that facilitate the effective on biomarker during treatment of PC.
Previous studies have explored numerous potential PC biomarkers, such as carbohydrate antigen 19-9 (CA19-9), cell division cycle 25C (CDC25C), and BUB1 Mitotic Checkpoint Serine/Threonine Kinase (BUB1) (8-10). However, despite their role in PC prognosis, the sensitivity and specificity of many biomarkers remain subpar. Therefore, further in-depth investigations into PC biomarkers and the identification of novel, more effective biomarkers for early diagnosis and prognosis models are imperative.
It is generally agreed that cancer development is closely linked to genetic mutations and transcriptional changes; however, the precise mechanisms driving PC progression are not yet fully understood. Recent studies have shed light on the pivotal role of membrane-less organelles, like nucleoli, which display distinct liquid-like characteristics through a process known as liquid-liquid phase separation (LLPS) (11,12). With an advancing comprehension of the underlying role of LLPS in cancers, there has been increased understanding that LLPS plays a pivotal role in various cellular functional mechanisms, including stress response, gene expression regulation, signal transduction control, and other biological processes (13). An increasing number of studies have shown an association between LLPS and the onset and progression of tumors (14,15). LLPS influences cancer development through multiple mechanisms including the regulation of gene expression, promotion of cellular autophagy, modulation of tumor immunity, regulation of DNA damage repair, and cellular ferroptosis (16). It is important to note that abnormal LLPS not only impacts gene expression but also contributes to carcinogenesis by altering cellular autophagy, immune responses, DNA repair processes, and other signaling pathways (17). These findings reveal the intricate interplay between LLPS and various cellular processes in the context of cancer progression. LLPS-related genes (LRGs) have emerged as potential prognostic markers for malignancies such as low-grade glioma (18), ovarian cancer (19), and digestive system tumors (20). Offering new avenues for precision oncology. However, the specific roles of LRGs in shaping the prognosis and tumor immune microenvironment of PC remain to be elucidated.
Mendelian randomization (MR) is a type of instrumental variable (IV) analysis used in epidemiology to examine causal associations between risk factors and disease outcomes (21). This method uses publicly accessible data from an extensive integrative epidemiology unit (IEU) Open genome-wide association studies (GWAS) database (https://gwas.mrcieu.ac.uk/) to examine risk factor “exposures” and disease “outcomes”, which effectively addresses the restrictions typically posed by a observational study (22). Three fundamental assumptions underpin MR investigations: (I) a strong and statistically significant association exists between IVs and exposure; (II) IVs are independent of confounding factors; and (III) IVs independently influence outcomes through exposure, excluding other pathways.
Randomized controlled trials (RCTs), which are widely recognized as the gold standard epidemiological design for establishing direct causal effects of risk factors on disease development, may not always be feasible due to cost constraints, implementation challenges, and ethical considerations (23). MR, which mimics RCT designs, has become a popular alternative approach. There is a current lack of empirical evidence demonstrating a causal association between PC and LLPS.
This study leveraged PC-related transcriptome sequencing data and clinical information from publicly accessible databases, and used a MR analysis and bioinformatics tools to explore the LRGs causally associated with PC. The characterization of these genes facilitated the development of a novel model to evaluate patient prognosis. Our findings offer a novel perspective on the prognosis and treatment of PC. We present this article in accordance with the TRIPOD reporting checklist (available at https://jgo.amegroups.com/article/view/10.21037/jgo-24-426/rc).
Methods
Data source
The Cancer Genome Atlas (TCGA)—pancreatic adenocarcinoma transcriptomic data set and clinical information were obtained from TCGA database (http://cancergenome.nih.gov/). The data set comprised 178 PC samples and four control samples. Pancreatic normal tissue samples from the Genotype-Tissue Expression (GTEx) database (http://www.gtexportal.org/home%5B33) were also included in the study. The two data sets were merged to create a training cohort that comprised 178 PC samples and 171 control samples (24). Additionally, the GSE62452 data set, comprising 69 PC samples and 61 control samples, was acquired from Gene Expression Omnibus (GEO) database (https://www.ncbi.nlm.nih.gov/gds) for the acquisition of differential genes. A data set comprising 145 PC samples was acquired from the International Cancer Genome Consortium (ICGC) database (https://dcc.icgc.org) as the testing cohort. Moreover, 3,612 LRGs were obtained from the data resource of LLPS (DrLLPS) database (25,26) (https://llps.biocuckoo.cn). The study was conducted in accordance with the Declaration of Helsinki (as revised in 2013).
Identifying the DE-LRGs
In the GSE62452 data set, differentially expressed genes (DEGs) were obtained from the PC and control samples (based on the following criteria: |log2 fold change| >0.5 and P<0.05) using the DESeq2 package (27) (v. 3.4.1). Next, the differentially expressed-LRGs (DE-LRGs) were obtained by overlapping the DEGs and 3,612 LRGs using the VennDiagram package (v. 1.7.3) (28).
MR analysis
The Expression Quantitative Trait Loci (eQTL) data for the DE-LRGs of MR analysis were obtained from the IEU Open GWAS database for use as exposure factors. The PC data set (bbj-a-140) from the IEU Open GWAS database, comprising 8,885,075 single nucleotide polymorphisms (SNPs) from 196,187 samples (case =442 and control =195,745), was selected as the outcome variable. Next, MR analysis was conducted to identify the genes causally associated with PC. In the beginning, the extract_instruments function of the TwoSampleMR package was used to read the exposure factors and screen the SNPs (29) (using the following criterion: P<5×10−8), and the SNPs that were significantly correlated with the exposure factors were identified. When clump = TRUE, any SNPs with linkage disequilibrium were removed using the following settings: r2=0.001 and kb =10. We ensured that our analyses included only independent SNPs to minimize the potential for confounding effects. Next, the harmonise_data function was used to harmonize the effect alleles with effect sizes.
In this study, five diverse MR methods [i.e., the MR-Egger method (30), weighted median method (31), inverse variance weighted (IVW) method (32), simple mode (29), and weighted mode (33)] were used to explore the causality of the observed associations between the DE-LRGs and PC. This study focused on the P value and odds ratio (OR) value of the IVW method. A P value <0.05 indicated a significant causal association between the exposure factors and outcome, an OR value >1 indicated that the exposure factors were risk factors, and an OR value <1 indicated that exposure factors were protective factors.
Moreover, a sensitivity analysis was conducted to evaluate the reliability of the MR results. First, a heterogeneity test (34) was conducted using Cochran’s Q test via the MR_heterogeneity function, and a P value >0.05 indicated no heterogeneity. Second, a horizontal pleiotropy test (35) was performed to determine the presence of confounding factors via the MR_pleiotropy_test function, and a P value >0.05 indicated the absence of confounding factors in the MR analysis. Finally, a leave-one-out (LOO) sensitivity test (36) was conducted by sequentially removing each SNP to evaluate the influence of excluding an individual SNP on the overall results. The genes that underwent the MR analysis and the sensitivity analysis were defined as the candidate genes for this study.
Functional enrichment analysis
Gene Ontology (GO) and Kyoto Encyclopedia of Genes and Genomes (KEGG) enrichment analyses of the candidate genes were conducted using the ClusterProfiler package (v. 4.7.1.3) (37) and org.Hs.eg.db package (v. 3.13.0) (38) (P<0.05). Subsequently, a protein-protein interaction (PPI) network was constructed of the candidate genes using the Search Tool for the Retrieval of Interacting Genes (STRING) database (interaction scores >0.4).
Construction and validation of a risk model
First, a univariate Cox regression analysis of the candidate genes was conducted using the survival package (v. 0.4.9) (39) to initially identify the potential prognostic-related genes. Subsequently, the glmnet package (v. 4.1.4) (40) was used for the least absolute selection and shrinkage operator (LASSO) regression analysis of the potential prognostic-related genes to further screen for any biomarkers. Using the biomarkers, a risk model was constructed in the training cohort. The risk score of each PC patient was calculated using the following formula:
where χi is the relative expression of biomarker i, and coefi is the LASSO coefficient of biomarker i.
PC patients were then categorized into high- and low-risk groups according to the median value of the risk score. The survminer package (v. 0.4.9) (39) was used for the Kaplan-Meier (K-M) survival analysis of the different risk groups. The model’s 1-, 3- and 5-year receiver operating characteristic (ROC) curves were plotted using survival ROC package (v. 1.18.0) (41). In addition, the risk model was validated using the testing cohort.
Independent prognostic analysis
The risk scores and clinical characteristics [i.e., age, gender, alcohol history, examined lymph nodes, diabetes, pathologic TNM staging (pathologic N stage), family history of cancer, and grade] of PC patients from the training cohort were first combined to perform a univariate Cox regression analysis. Next, independent prognostic factors were identified by a multivariate Cox analysis of the variables that passed the proportional hazards (PH) assumption test. Finally, the rms package (v. 6.5.0) (42) was used to construct a nomogram of the independent prognostic factors. The predictive survival performance of the nomogram was evaluated using calibration curves and ROC curves.
Disease correlation and enrichment analysis
To determine the relationship between the biomarkers and PC, we analyzed the correlations between the two using the Comparative Toxicogenomics Database (CTD) (https://ctdbase.org/). Next, the samples in the training cohort were classified into high- and low-expression groups. A differential expression analysis was then conducted using the DESeq2 package (v. 3.4.1) (27), and biomarkers ranked according to log2 fold change. A Gene Set Enrichment Analysis (GSEA) was conducted using the ClusterProfiler package (P<0.05). The Molecular Signatures DataBase (MSigDB) c2: KEGG gene set was used as the background gene set.
Immunoassays associated with PC
The ssGESA and Tumor Immune Dysfunction and Exclusion (TIDE) analysis of immune cells in PC
To assess the composition of the immune cells in the immune microenvironment of the PC patients, the single-sample Gene Set Enrichment Analysis (ssGESA) algorithm in the GSVA package (v. 1.46.0) (43) was used to compute 28 cell enrichment scores for the different risk groups and to compare differences between the groups. Correlation analyses between the biomarkers and differential immune cells were performed using the Spearman method. Moreover, to further assess the effectiveness of the immunotherapeutic response in the training cohort, TIDE scores, dysfunction scores, exclusion scores and microsatellite instability (MSI) scores were compared between the different risk groups. The TIDE algorithm was also used to assess the response rates of the different groups to immunotherapy.
Immune checkpoint inhibitor therapy
The immune checkpoint is a type of immunosuppressive molecule expressed on immune cells, which can modulate the level of immune activation. These molecules play a crucial role in preventing the development of autoimmunity. To put it simply, they are small protein molecules produced by immune cells to regulate autoimmune function. A Spearman correlation analysis was conducted between these six prognostic genes and 19 immune checkpoints (44). In the training set samples, with a significance threshold of P<0.05 and a correlation coefficient of R>0.3 as the screening conditions. Through this analysis, we identified significant associations between the prognostic genes and immune checkpoints, which could provide insights into the mechanisms underlying PC progression and potential therapeutic targets.
Construction of a regulatory network
To identify other genes connected with biomarker function, a Gene-Gene Interaction (GGI) network was constructed based on the biomarkers using the GeneMANIA database (http://genemania.org). Additionally, in an effort to investigate the regulation of the biomarkers by transcription factors (TFs), the Jaspar database (http://jaspar.genereg.net) was used to predict the TFs responsible for regulating these biomarkers. To further elucidate the mechanisms underlying the role of the biomarkers in PC, we predicted the associated micro RNAs (miRNAs) using the miRTarBase (http://mirtarbase.cuhk.edu.cn/) and miRDB (http://www.mirdb.org/) databases, and determined the corresponding long non-coding RNAs (lncRNAs) using the starBase database (https://rnasysu.com/encori/). Eventually, a competitive endogenous RNA (ceRNA) network was established, and visualized using Cytoscape software (v. 3.7.2) (45).
Drug sensitivity analysis
To further explore the potential drugs associated with PC, Gene Set Cancer Analysis (GSCA) Lite was used to assess the correlations and false discovery rates (FDRs) among the biomarker expression levels and drug sensitivity in the Cancer Therapeutics Response Portal (CTRP) (http://portals.broadinstitute.org/ctrp/) and Genomics of Drug Sensitivity in Cancer (GDSC) databases (http://cancerrxgene.org) (using the following criteria: |cor| >0.1 and FDR <0.05).
Statistical analysis
All the statistical analyses were executed in R software (v. 4.2.3). Differences between the groups were analyzed by the Wilcoxon test. A P value <0.05 was considered statistically significant.
Results
A total of 297 DE-LRGs were identified
A total of 1,524 DEGs, of which 1,069 were upregulated and 455 were downregulated, were identified between the PC and control samples from the GSE62452 data set (Figure 1A,1B). Subsequently, 297 DE-LRGs were identified by overlapping the 1,524 DEGs and 3,612 LRGs (Figure 1C).
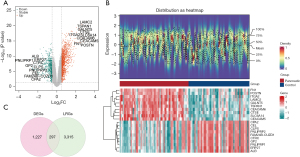
A total of 39 candidate genes were identified by the MR analysis
Upon identifying the 297 DE-LRGs, we conducted a MR analysis to examine any potential causal associations with PC. From the analysis, 39 genes emerged as candidate exposure factors, of which 20 appeared to decrease the risk of PC (OR <1), and 19 appeared to increase the risk of PC (OR >1) (Table 1). The results for ACTR3, ATP8A1, CCNA2, ITGB1, PYGB, and RAP1GAP2 were significant, and PYGB [OR =1.1117; 95% confidence interval (CI): 1.0797–1.1447; P<0.001], ACTR3 (OR =17.3032; 95% CI: 2.2063–135.7010; P=0.007), CCNA2 (OR =1.5399; 95% CI: 1.1263–2.1055; P=0.007), and ITGB1 (OR =1.8330; 95% CI: 1.1335–2.9643; P=0.01) were identified as risk factors for PC, while ATP8A1 (OR =0.8833; 95% CI: 0.8061–0.9680; P=0.008), and RAP1GAP2 (OR =0.7692; 95% CI: 0.5997–0.9866; P=0.04) were found to be protective against PC. The degree of correlation between the 39 potential exposure factors and PC were visualized in a scatter plot (Figure S1), the forest plots substantiated the significant effect of the IVW model (Figure S2), and the funnel plots showed that MR conformed to Mendel’s second law of random grouping (Figure S3).
Table 1
Outcome | Genes | NSNP | P value | OR | OR_lci95 | OR_uci95 |
---|---|---|---|---|---|---|
Pancreatic cancer (bbj-a-140) | RECQL | 20 | 0.009 | 1.5144 | 1.1095 | 2.0670 |
AP2B1 | 16 | 0.001 | 1.4897 | 1.1847 | 1.8732 | |
MVP | 24 | 0.02 | 1.3982 | 1.0605 | 1.8435 | |
EIF2AK2 | 33 | 0.02 | 0.7418 | 0.5814 | 0.9464 | |
BCAS1 | 14 | <0.001 | 1.8245 | 1.3389 | 2.4863 | |
ENO1 | 45 | <0.001 | 0.6452 | 0.5368 | 0.7755 | |
MCM6 | 115 | 0.004 | 0.9578 | 0.9301 | 0.9863 | |
PSEN1 | 62 | <0.001 | 1.1518 | 1.0768 | 1.2321 | |
XPO1 | 10 | 0.001 | 2.3704 | 1.4225 | 3.9499 | |
LYZ | 71 | 0.005 | 1.0609 | 1.0185 | 1.1051 | |
SEC14L2 | 16 | <0.001 | 1.4994 | 1.2257 | 1.8341 | |
PYGB | 109 | <0.001 | 1.1117 | 1.0797 | 1.1447 | |
CORO1C | 36 | 0.008 | 1.0886 | 1.0226 | 1.1589 | |
ACTR3 | 3 | 0.007 | 17.3032 | 2.2063 | 135.7010 | |
STAT1 | 48 | <0.001 | 0.5549 | 0.4305 | 0.7151 | |
SLC25A12 | 35 | 0.03 | 0.8766 | 0.7809 | 0.9840 | |
ATP8A1 | 39 | 0.008 | 0.8833 | 0.8061 | 0.9680 | |
PALLD | 17 | 0.003 | 0.5054 | 0.3249 | 0.7861 | |
ACTN4 | 38 | <0.001 | 1.3653 | 1.1514 | 1.6190 | |
CAP1 | 27 | 0.003 | 1.3167 | 1.0957 | 1.5822 | |
RAP1GAP2 | 28 | 0.04 | 0.7692 | 0.5997 | 0.9866 | |
MYH11 | 31 | <0.001 | 0.8892 | 0.8392 | 0.9421 | |
LCP1 | 15 | 0.001 | 1.5035 | 1.1794 | 1.9167 | |
KIF23 | 11 | 0.007 | 1.4953 | 1.1183 | 1.9994 | |
TMOD3 | 48 | <0.001 | 0.6364 | 0.5865 | 0.6905 | |
BRCA2 | 8 | 0.02 | 0.6823 | 0.4925 | 0.9451 | |
PML | 21 | <0.001 | 1.4616 | 1.2171 | 1.7552 | |
CDC42BPA | 37 | 0.004 | 0.6964 | 0.5447 | 0.8904 | |
RALB | 67 | <0.001 | 0.8847 | 0.8389 | 0.9331 | |
SCD5 | 31 | <0.001 | 0.9138 | 0.8796 | 0.9492 | |
CCNA2 | 14 | 0.007 | 1.5399 | 1.1263 | 2.1055 | |
ITGB1 | 13 | 0.01 | 1.8330 | 1.1335 | 2.9643 | |
ALB | 7 | 0.008 | 2.6246 | 1.2855 | 5.3587 | |
PSMD1 | 26 | 0.04 | 0.8901 | 0.7951 | 0.9965 | |
SPATS2L | 60 | 0.002 | 0.8394 | 0.7509 | 0.9382 | |
KIF13B | 21 | <0.001 | 0.7696 | 0.6837 | 0.8663 | |
ECI2 | 18 | 0.04 | 0.8075 | 0.6578 | 0.9913 | |
INF2 | 11 | 0.002 | 0.7014 | 0.5621 | 0.8752 | |
HSPA1B | 129 | 0.04 | 0.9047 | 0.8253 | 0.9918 |
MR, Mendelian randomization; NSNP, number of single nucleotide polymorphism; OR, odds ratio; lci95, 95% lower confidence interval; uci95, 95% upper confidence interval.
To assess the validity of the MR analysis, a sensitivity analysis was performed. The results from Cochran’s Q test indicated no heterogeneity (P>0.05) within the samples (Table 2). However, according to the horizontal pleiotropy test, LYZ, STAT1, PALLD, KIF23, TMOD3, and SCD5 were found to have confounding factors (P<0.05), which necessitated their removal from the analysis (Table 2). On sequentially eliminating each SNP, the effect of the remaining SNPs on PC remained stable, indicating the reliability of the MR analysis results (Figure S4). Consequently, 39 candidate genes were determined to have potential causal associations with PC and were thus retained for further investigation.
Table 2
Outcome | Genes | MR_pleiotropy_test Q_P value | MR_heterogeneity P value |
---|---|---|---|
Pancreatic cancer (bbj-a-140) | RECQL | 0.89 | 0.49 |
AP2B1 | 0.04 | 0.06 | |
MVP | 0.70 | 0.33 | |
EIF2AK2 | 0.84 | 0.78 | |
BCAS1 | 0.83 | 0.31 | |
ENO1 | >0.99 | 0.36 | |
MCM6 | 0.32 | 0.78 | |
PSEN1 | >0.99 | 0.75 | |
XPO1 | >0.99 | 0.98 | |
LYZ | 0.27 | 0.006 | |
SEC14L2 | >0.99 | 0.70 | |
PYGB | 0.18 | 0.06 | |
CORO1C | >0.99 | 0.93 | |
ACTR3 | 0.91 | 0.74 | |
STAT1 | 0.92 | 0.04 | |
SLC25A12 | >0.99 | 0.94 | |
ATP8A1 | 0.99 | 0.43 | |
PALLD | 0.56 | 0.005 | |
ACTN4 | 0.98 | 0.81 | |
CAP1 | >0.99 | 0.86 | |
RAP1GAP2 | 0.64 | 0.46 | |
MYH11 | >0.99 | 0.86 | |
LCP1 | 0.92 | 0.07 | |
KIF23 | 0.72 | 0.04 | |
TMOD3 | 0.38 | 0.001 | |
BRCA2 | 0.99 | 0.96 | |
PML | 0.95 | 0.12 | |
CDC42BPA | 0.99 | 0.18 | |
RALB | 0.99 | 0.52 | |
SCD5 | 0.47 | 0.009 | |
CCNA2 | 0.97 | 0.75 | |
ITGB1 | 0.90 | 0.36 | |
ALB | >0.99 | 0.79 | |
PSMD1 | >0.99 | 0.50 | |
SPATS2L | 0.16 | 0.05 | |
KIF13B | 0.81 | 0.47 | |
ECI2 | 0.27 | 0.31 | |
INF2 | 0.39 | 0.09 | |
HSPA1B | 0.60 | <0.001 |
MR, Mendelian randomization.
Candidate genes were mainly involved in functions such as the regulation of ATP metabolic process and pathways such as tight junction
The functional enrichment analysis revealed that the candidate genes were associated with the regulation of the ATP metabolic process, cell-substrate junction, 3'-5' DNA helicase activity, and other GO entries (Figure 2A), and were enriched in KEGG pathways such as tight junction, the endocytosis, the regulation of actin cytoskeleton, and other pathways (Figure 2B). The PPI network demonstrated 27 interactions for 19 proteins. For example, ALB was associated with ACTR3, BRCA2, ENO1, HSPA1B, PSEN1, and EIF2AK2 (Figure 2C).
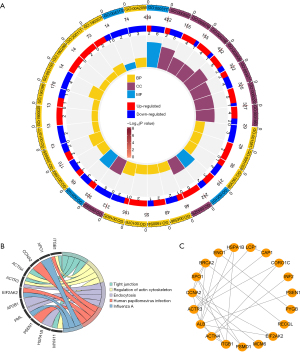
The risk model demonstrated robust evaluation capabilities
A total of 21 potential prognostic-related genes were identified by the univariate Cox regression analysis (P<0.05) (Figure 3A). In total, 14 of these genes were consistent with the risk and protective factors of MR. Therefore, we selected these 14 genes for the next stage of the analysis. Ultimately, we identified six biomarkers; that is, ACTR3, ATP8A1, CCNA2, ITGB1, PYGB, and RAP1GAP2 (Figure 3B,3C). A risk model was constructed using these biomarkers, with the risk score calculated as follows: = ACTR3 × (−0.130110904) + ATP8A1 × (−0.040437132) + CCNA2 × 0.290313262 + ITGB1 × 0.187252514 + PYGB × 0.187252514 + RAP1GAP2 × (−0.302985606). The risk scores, survival status, and biomarker expression were calculated for different groups (Figure 3D). In the training cohort, patients with a low risk showed a longer survival span than those with a high risk (P<0.05) (Figure 3E). The areas under the curve (AUCs) were reported as 0.741, 0.687, and 0.715 for 1, 3, and 5 years, respectively (Figure 3F), indicating the potential prediction capacity of this model. Additionally, consistent results were observed when verification was performed in the testing cohort. The risk scores, survival status, and expression of the biomarkers were displayed for the diverse groups in the testing cohort (Figure 3G). The low-risk patients survived longer than the high-risk patients (Figure 3H), while AUC scores of 0.73, 0.73, and 0.67 were obtained for 1, 3 and 5 years, respectively (Figure 3I). These findings supported the predictive accuracy of the risk model.
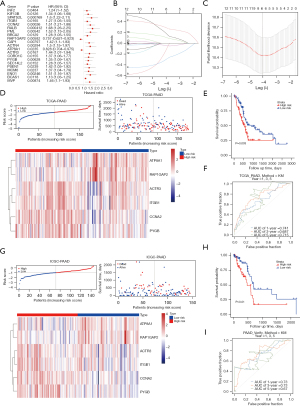
Only risk score, age, and pathologic N stage were independent factors of prognosis in PC patients
The risk score, age, pathological N, and grade were selected for the subsequent univariate Cox regression analysis (P<0.05) (Figure 4A). The conformity of all four clinical attributes to the PH assumption was affirmed by the PH assumption test (P>0.05). This led to the application of a multivariate Cox regression analysis, taking into account the risk score, age, pathologic N stage, and grade. Based on the results, only risk score, age, and pathologic N stage emerged as independent predictors of the prognosis of PC patients (P<0.05) (Figure 4B).
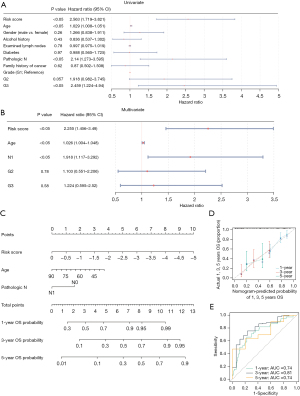
Consequently, a nomogram was constructed to forecast the 1-, 3-, and 5-year survival rates of the PC patients (Figure 4C). Both the calibration curves (which showed a high degree of overlap with the reference line) and the ROC curves (with an AUC >0.6) indicated that the nomogram yielded promising predictions (Figure 4D,4E). This lends credence to the potential clinical applicability of our prognostic model.
CCNA2 gene was highly correlated with PC and enriched in pathways, such as maturity-onset diabetes of the young (MODY)
The reasoning scores for ACTR3, ATP8A1, CCNA2, ITGB1, PYGB, and RAP1GAP2 were 50.15, 32.73, 142.29, 93.19, 44.95, and 35.43, respectively. CCNA2 had the highest reasoning score, which indicated that CCNA2 was highly correlated with PC (Figure 5A). The GSEA results showed that ATP8A1, CCNA2, PYGB, and RAP1GAP2 were co-enriched in the MODY in the KEGG terms. ACTR3 and ITGB1 were co-enriched in olfactory transduction and graft versus host disease in the KEGG terms (Figure 5B-5G).
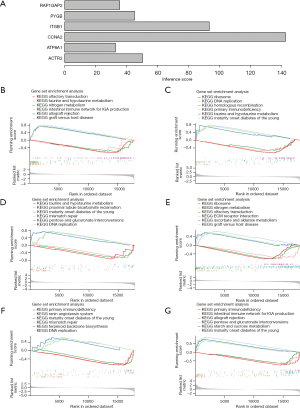
Immune analysis of PC patients
Based on the training cohort, an extensive series of immune analyses were performed on the PC patients. We found significant differences in 10 cell types, including eosinophils and gamma delta T cells, across the different groups (P<0.05) (Figure 6A,6B). These varying types of immune cells may influence the prognostic risk of PC patients, and thus could play a potential role in patient prognosis.
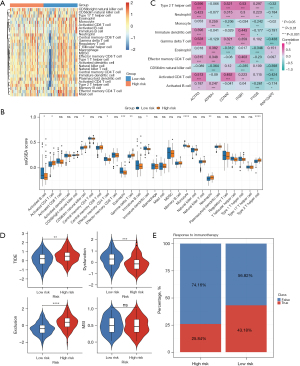
Additionally, a correlation analysis of the immune-infiltrating cells revealed that ACTR3, CCNA2, and ITGB1 were primarily associated with type 2 T helper cells, and ATP8A1 and PYGB were mainly linked to eosinophils, while RAP1GAP2 was strongly associated with gamma delta T cells. These findings suggest that these biomarkers may have influence PC prognosis through immune signatures (Figure 6C).
Higher TIDE and exclusion scores were observed in the high-risk patients, while significantly lower dysfunction scores were observed in the low-risk patients. These findings indicate that high-risk patients demonstrated an increased potential for immune escape, which could potentially diminish the efficacy of immune checkpoint inhibition (ICI) therapy (Figure 6D). Conversely, the low-risk patients exhibited a higher immunotherapy response rate of 43.18% (Figure 6E). And the results of immunological examination showed that most of the 6 biomarkers were correlated with 19 immunological examination points. Among them, RAP1GAP2 was negatively correlated with CD70 (R=−0.31), and ACTR3 was positively correlated with CD47 (R=0.73) (Figure S5).
Regulatory networks of biomarkers
Through the analysis of the GGI network, we identified the main functions associated with six biomarkers, such as actin nucleation and actin binding (Figure 7A). The TF for ATP8A1 was not retrieved in database. Therefore, five biomarkers were used to construct a regulatory network of TFs and biomarkers. The results showed that the regulatory network comprised a total of 95 TFs and 117 interaction pairs, such as CCNA2-RFXANK-PYGB-ZNF341-ITGB1-SIRT6-ACTR3-BCOR-RAP1GAP2 (Figure 7B). The ceRNA network showed that 10 lncRNA (e.g., MIR29B2CHG, AC020916.1, and AC084082.1) regulated six messenger RNAs (mRNAs) through 28 miRNAs, forming a total of 50 interaction pairs, such as CCNA2-hsa-miR-29a-3p-AC145207.5 (Figure 7C).
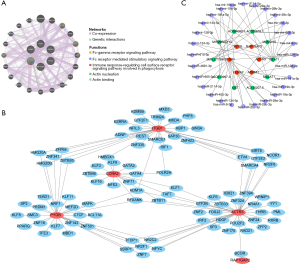
The six biomarkers were correlated with potential drugs
The results showed that the six biomarkers were co-correlated with potential drugs, such as PHA-793887. Specifically, ACTR3 was correlated with PIK-93, BIX02189, and other drugs, ATP8A1 was correlated with PD-0325901, THZ-2-102-1, and other drugs, CCNA2 was correlated with TG101345, Y-39983, and other drugs, IGTB1 was correlated with PI-103, GSK690693, and other drugs, PYGB was correlated with ispinesib mesylate, navitoclax, and other drugs, and RAP1GAP2 was correlated with AZD6482, NPK76-II-72-1, and other drugs (Figure 8A-8F).
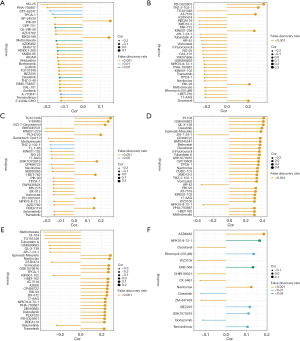
Discussion
PC is a highly malignant neoplasm with an unfavorable prognosis, and its incidence and mortality rates are nearly equivalent (46). Owing to the absence of evident symptoms in the early stages of PC as well as the lack of biomarkers for its early detection, approximately 80% of diagnosed patients have advanced-stage disease at the time of diagnosis, which reduces their eligibility for surgical intervention (47). Moreover, PC exhibits low sensitivity to chemotherapy and immunotherapy regimens, even when combination therapeutic approaches are employed. Thus, it remains challenging to achieve favorable clinical outcomes for PC patients (48). Consequently, it is imperative to identify suitable biomarkers for the early detection and prognostic monitoring of PC.
Tumorigenesis is intricately linked to gene mutations and transcriptional dysregulation. However, despite considerable progress in cancer pathogenesis research, a comprehensive understanding of the mechanisms driving cancer growth remains elusive (19,49). Aberrant LLPS of biomolecules plays an important role in epigenetic dysregulation, significantly affecting transcriptional and translational regulation, which in turn promotes tumorigenesis and tumor progression (50-52). For example, LLPS drives cancer progression by influencing gene fusions and mutations through multivalent interactions forming SPOP-DAXX vesicles (53,54), PML vesicles (51), and FET protein condensates (55). Abnormalities in LLPS might impact biomolecules involved in DNA damage repair, thus affecting the DNA repair capacity of tumor cells. This effect can lead to genomic instability in tumor cells and promote tumorigenesis (17). Takayama’s study reveals that TF activation through LLPS-based transcription collaborates to contribute to tumor aggressiveness and drug resistance (56). Deeply exploring the molecular mechanisms of LLPS and its synergistic effects with RNA modifications can develop new therapeutic strategies and drug targets to treat cancer patients more effectively.
The merits of a MR analysis lie in its capacity to elucidate causal associations, mitigate confounding effects, and enhance analytical reliability. To the best of our knowledge, there is currently no established causal association between PC and LLPS based on MR. Thus, our research explored the potential causality between DE-LRGs and PC using the MR approach. This methodology astutely mitigates the confounding factors and reverse causality typically experienced in standard observational studies. We selected the IVW method as our primary approach, as it offers the improved detection of causal associations. The results revealed 19 risk factors and 20 protective factors among the exposure variables. By employing a MR analysis and a sensitivity analysis, we identified a total of 39 genes with no heterogeneity or horizontal pleiotropy. This indicates the reliability of our MR results.
Our investigation identified six LLPS-related biomarkers that were associated with the prognosis of PC; that is, ACTR3, ATP8A1, CCNA2, ITGB1, PYGB, and RAP1GAP2. Our MR analysis revealed that PYGB (OR =1.1117), CCN2 (OR =1.5399), ITGB1 (OR =1.1117), and ACTR3 (OR =17.3032) were risk factors for PC, while ATP8A1 (OR =0.8833) and RAP1GAP2 (OR =0.7692) were protective factors for PC. This led us to advance our inquiry into the modes of action pertaining to underlying the six biomarkers identified in PC.
Importantly, these genes have been extensively studied for their involvement in tumorigenesis and tumor proliferation. Notably, ACTR3 expression is significantly upregulated in PC tissues, and high levels of ACTR3 are indicative of a poor prognosis in patients with PC. Further, ACTR3 promotes the migration and invasion of PC cells by inducing epithelial-mesenchymal transition (57).
Phospholipid phosphatidylserine is the predominant constituent of eukaryotic cell membranes, and exhibits an asymmetric distribution within biofilms. ATP8A1 is a member of the P4-ATPase family, which consists of phospholipid turnover enzymes that intricately regulate the precise transport of phosphatidylserine. In hematopoietic stem cells, deficiency in Atp8a1 indirectly activates the PI3K-AKT-mTORC1 signaling pathway, resulting in an increase in JNK/AP-1 signaling activity and YAP1 phosphorylation. This ultimately leads to an asymmetric loss of phosphatidylserine and disruption in intracellular signaling (58). ATP8A1 has been found to be upregulated in non-small cell lung cancer (NSCLC) tissues, and to enhance the invasive and migratory capabilities of NSCLC cells by upregulating the expression of MMP-9 and Vimentin, while downregulating the expression of E-cadherin (59).
CCNA2, a member of the cyclin family, has been identified as an oncogene in various solid tumors, and exerts a significant influence on the prognosis of diverse tumor types (60). Moreover, CCNA2 has been shown to be significantly correlated with the infiltration of immune cells and the expression of immune checkpoint inhibitory genes. Additionally, CCNA2 is closely associated with drug resistance. The overexpression of CCNA2 has been observed in endometrial cancer tissues, promoting cell migration and invasion, which can be targeted by miR-524-5p (61).
Under hypoxia, the lncRNA NNT-AS1/METTL3-HuR complex mediates the m(6)A modification of ITGB1 expression, thereby promoting immune evasion in PC cells (62). Iwatate et al. found a significant correlation between the ITGB1 expression levels and a poor prognosis, as well as a high recurrence rate in the group with high ITGB1 expression, which suggests that targeting ITGB1 could be a potential therapeutic strategy for PC (63).
PYGB exhibits high expression levels in PC, and it exerts a regulatory influence on the malignant behavior of PC cells via the modulation of the NF-kappa B signaling pathway. These findings suggest that targeting PYGB could serve as a potential therapeutic strategy for managing PC (64).
To date, no studies have reported on the association between RAP1GAP2 and tumor development. However, previous research has highlighted the crucial role played by Rap1gap2 in orchestrating axonal growth dynamics within olfactory sensory neurons during early postnatal development (65). Further, Rap1GAP2 has been shown to regulate dense granule secretion in platelets via its interaction with synaptotagmin-like protein 1 Slp1 (Slp1) (66).
Despite the extensive literature, the prognostic correlation between these six LRGs and PC patients remains unclear. Future research endeavors will include the continued observation of these genes with subsequent investigations into their mechanisms of action. This approach is anticipated to provide further understanding and clarification of their roles and implications in relation to PC.
Multiple signaling pathways are implicated in the tumorigenesis and malignant progression of PC. The present study found a significant association between CCNA2 and PC, particularly in individuals with MODY. Further, the GSEA analysis revealed the co-enrichment of ATP8A1, CCNA2, PYGB, and RAP1GAP2 in the AID-juvenile diabetes pathway of the KEGG data set, but further experimental validation is required. Diabetes is not only a risk factor for PC, but is also one of the secondary diseases resulting from it. Type 2 diabetes mellitus serves as a predisposing factor for PC, particularly in cases of new-onset diabetes mellitus (67). These biomarkers are implicated in multiple signaling pathways. For instance, PYGB exerts an oncogenic role in ovarian cystadenocarcinoma (68), NSCLC (69), and gastric cancer (70) via the modulation of the Wnt/β-catenin signaling pathway. The expression of PYGB is significantly upregulated in PC, and it exerts regulatory control over the malignant behavior of PC cells by modulating the NF-kappa B signaling pathway (64). Biomarkers can exert their effects through diverse signaling pathways, and tumorigenesis represents a complex interplay among multiple signaling cascades.
The immune system exerts an anti-tumor effect by effectively targeting and eliminating tumor cells. Conversely, it can also contribute to the development of tumors by creating a conducive immunosuppressive microenvironment (71). PC represents one of the most immune-resistant tumor types characterized by its “cold” nature and limited response to immune-related therapies (72). This implies impaired immune cell function and resistance to checkpoint blockade, indicating that the mechanisms of immunotherapy resistance in the treatment of PC are diverse (73). Given the personalized genetic and phenotypic heterogeneity observed in individual patients with PC, immunotherapy strategies may need to be tailored accordingly.
To elucidate the underlying immune characteristics, we conducted an analysis of the high- and low-risk groups in the training cohort. Our findings revealed that patients in the high-risk group showed a heightened potential for immune evasion and a diminished response to ICI therapy, while those in the low-risk group demonstrated a favorable immunotherapeutic response rate. No significant disparities were observed in the immune microenvironment between the samples from the different risk groups, but notable distinctions were identified in 10 specific immune cell populations between these two groups. Notably, ACTR3, CCNA2, and ITGB1 displayed predominant associations with type 2 helper T cells, and ATP8A1 and PYGB showed primary associations with eosinophils, while RAP1GAP2 showed primary associations with gamma delta T cells. These observations suggest that these biomarkers may exert their influence on patient outcomes via the modulation of these specific immune cell subsets. A low eosinophil count and a low eosinophil/lymphocyte ratio have been identified as independent risk factors for the poor prognosis of PC. T helper cell type 2 infiltration promotes tumor lymph node hyperplasia and influences the anti-tumor immune response (74). However, to date, there is no definitive conclusion regarding which subtype of PC patients would be more suitable candidates for immunotherapy.
In this study, a MR analysis was employed to identify six genes associated with LLPS, which play a role in immune cell infiltration. Compared to the low-risk patients, the high-risk patients exhibited significantly elevated TIDE and exclusion scores, along with notably reduced dysfunction scores. These findings suggest an increased probability of immune escape and diminished response to ICI therapy in high-risk patients, while low-risk patients demonstrated higher rates of immunotherapy response. Therefore, our model could be used to potential the efficacy of immunotherapy for PC patients.
Our analysis of the GGI network revealed that the primary functions associated with the six identified biomarkers in PC were the Fc-gamma receptor signaling pathway, Fc receptor mediated stimulatory signaling pathway, and immune response-regulating cell surface receptor signaling pathway. These pathways are implicated in processes such as phagocytosis, actin nucleation, and actin binding. Notably, these pathways are closely linked to tumor immunity and metastasis.
The Fc-gamma receptor signaling pathway is a crucial mechanism by which various immune cell-mediated biological responses, including cellular phagocytosis, oxidative burst, and cytokine production, can be elicited. It is predominantly expressed on the surface of key immune cells, such as macrophages, dendritic cells, and natural killer (NK) cells. The activation of these receptors instigates phagocytic action in macrophages, dendritic cells, and NK cells, thereby enabling the efficient engulfment and degradation of pathogens, cellular debris, and tumor cells.
The mechanism of action of Fc-gamma receptor in anti-tumor immunotherapy is increasingly being acknowledged (75). Actin proteins are vital components of the cytoskeleton that are essential in maintaining cell shape and enabling functions such as cell movement. The nuclear localization and interaction of actin proteins are complexly involved in various biological processes, including cell signaling, migration, and division. Cancer cells exploit rearrangements of the actin cytoskeleton for malignant activities, such as activation, proliferation, migration, and invasion (76). Therefore, nuclear formation and the ligation of action proteins may also be involved in PC.
In our study, no TFs associated with ATP8A1 were identified. However, the other five biomarkers’ regulatory networks encompassed 95 TFs and 117 interaction pairs. Our analysis indicated that the TF RFXANK plays a pivotal role in the transcriptional regulation of MHC II genes, influencing the behavior of CCNA2, PYGB, and ACTR3. However, the exact mechanism of this regulation has yet to be elucidated. Additionally, TF ZNF341, which is part of the C2H2-type zinc finger protein family, is implicated in DNA binding and the modulation of gene transcription. Nonetheless, additional research is required to clarify the molecular mechanisms underlying this regulatory activity in PC.
The literature has reported that ZNF341 may exert a regulatory effect on the STAT3 gene (77). Our analysis revealed that ITGB1 and PYGB are under the regulation of ZNF341; thus, further research needs to be conducted into the underlying mechanism. The hsa-miR-29a-3p is a human miRNA that belongs to the class of small, non-coding RNA molecules that play a pivotal role in post-transcriptional gene expression regulation. Studies have demonstrated its potential effect on cancer development via the modulation of DNA methylation patterns (78,79). The lncRNA AC145207.5 has emerged as a potential prognostic biomarker for PC, based on available data.
This study identified six biomarkers, which led to the prediction that several compounds (i.e., PHA-793887, PIK-93, BIX02189, PD-0325901, and THZ-2-102-1) may hold therapeutic potential. Further, TG101343, Y-39983, PI-103, GSK690693, ispinesib mesylate, navitoclax, and AZD6482 emerged as promising agents. These compounds are linked to signaling pathways that inhibit cellular growth, proliferation, and differentiation, suggesting their possible therapeutic application in PC. For example, PHA-793887 is a cyclin-dependent kinase inhibitor that could be considered for clinical trial inclusion due to its potential to suppress tumor cell growth. PIK-93, which inhibits both PI3K and mTOR, targets important molecular pathways involved in tumor initiation and progression, including in PC. BIX02189, a selective inhibitor of MEK5, disrupts tumor cell signaling. Nevertheless, the precise relationships and effects of these agents require further empirical validation through experimental and clinical studies.
Prognostic risk models have demonstrated significant strengths in exploring the relationship between pancreatic adenocarcinoma and LLPS. First, high accuracy and personalized prediction. The prognostic risk model integrates key genes related to pancreatic adenocarcinoma, improving prediction accuracy and enabling personalized treatment plans. Additionally, consideration of immune microenvironment. The model incorporates traditional prognostic factors and genes from the immune microenvironment, providing a more comprehensive risk assessment by reflecting inter-patient immune variability. Finally, utility and potential for clinical application. The prognostic risk model has shown good stability and applicability across diverse patient groups, indicating its strong potential for widespread clinical use. However, there are some limitations. All the clinical data on PC were derived from public databases, which may have introduced some bias into the data. Second, while our findings suggest a causal association between the LRGs and PC, the underlying mechanism remains unclear. Additionally, the validity of the risk scoring model needs to be confirmed using a larger sample size. Finally, while our research was grounded in bioinformatics analyses, comprehensive in-vitro and clinical studies need to be conducted to substantiate our findings. Future work will thus seek to elucidate the functional implications of these genes in PC.
Conclusions
This study integrated a transcriptome analysis and a MR analysis to identify biomarkers associated with LLPS in PC. It also investigated the genetic causality between LRGs and PC, and developed a risk prognosis model. Our findings provide novel insights for the clinical diagnosis and treatment of PC.
Acknowledgments
The authors would like to express their appreciation for the efforts of the GWAS consortium, TCGA, ICGC, GTEx, GEO, and DrLLPS databases. The authors also appreciate the great support from Dr. Damiano Caputo (Università Campus Bio-Medico, Italy) in improving the quality of this paper.
Funding: None.
Footnote
Reporting Checklist: The authors have completed the TRIPOD reporting checklist. Available at https://jgo.amegroups.com/article/view/10.21037/jgo-24-426/rc
Peer Review File: Available at https://jgo.amegroups.com/article/view/10.21037/jgo-24-426/prf
Conflicts of Interest: All authors have completed the ICMJE uniform disclosure form (available at https://jgo.amegroups.com/article/view/10.21037/jgo-24-426/coif). The authors have no conflicts of interest to declare.
Ethical Statement: The authors are accountable for all aspects of the work in ensuring that questions related to the accuracy or integrity of any part of the work are appropriately investigated and resolved. The study was conducted in accordance with the Declaration of Helsinki (as revised in 2013).
Open Access Statement: This is an Open Access article distributed in accordance with the Creative Commons Attribution-NonCommercial-NoDerivs 4.0 International License (CC BY-NC-ND 4.0), which permits the non-commercial replication and distribution of the article with the strict proviso that no changes or edits are made and the original work is properly cited (including links to both the formal publication through the relevant DOI and the license). See: https://creativecommons.org/licenses/by-nc-nd/4.0/.
References
- Klein AP. Pancreatic cancer epidemiology: understanding the role of lifestyle and inherited risk factors. Nat Rev Gastroenterol Hepatol 2021;18:493-502. [Crossref] [PubMed]
- Halbrook CJ, Lyssiotis CA, Pasca di Magliano M, et al. Pancreatic cancer: Advances and challenges. Cell 2023;186:1729-54. [Crossref] [PubMed]
- Kleeff J, Korc M, Apte M, et al. Pancreatic cancer. Nat Rev Dis Primers 2016;2:16022. [Crossref] [PubMed]
- Aslanian HR, Lee JH, Canto MI. AGA Clinical Practice Update on Pancreas Cancer Screening in High-Risk Individuals: Expert Review. Gastroenterology 2020;159:358-62. [Crossref] [PubMed]
- Duan H, Li L, He S. Advances and Prospects in the Treatment of Pancreatic Cancer. Int J Nanomedicine 2023;18:3973-88. [Crossref] [PubMed]
- Siegel RL, Miller KD, Fuchs HE, et al. Cancer Statistics, 2021. CA Cancer J Clin 2021;71:7-33. [Crossref] [PubMed]
- Kommalapati A, Tella SH, Goyal G, et al. Contemporary Management of Localized Resectable Pancreatic Cancer. Cancers (Basel) 2018;10:24. [Crossref] [PubMed]
- Bauer TM, El-Rayes BF, Li X, et al. Carbohydrate antigen 19-9 is a prognostic and predictive biomarker in patients with advanced pancreatic cancer who receive gemcitabine-containing chemotherapy: a pooled analysis of 6 prospective trials. Cancer 2013;119:285-92. [Crossref] [PubMed]
- Li M, Duan X, Xiao Y, et al. BUB1 Is Identified as a Potential Therapeutic Target for Pancreatic Cancer Treatment. Front Public Health 2022;10:900853. [Crossref] [PubMed]
- Zhou C, Wang L, Hu W, et al. CDC25C is a prognostic biomarker and correlated with mitochondrial homeostasis in pancreatic adenocarcinoma. Bioengineered 2022;13:13089-107. [Crossref] [PubMed]
- Lu J, Qian J, Xu Z, et al. Emerging Roles of Liquid-Liquid Phase Separation in Cancer: From Protein Aggregation to Immune-Associated Signaling. Front Cell Dev Biol 2021;9:631486. [Crossref] [PubMed]
- Liu Q, Li J, Zhang W, et al. Glycogen accumulation and phase separation drives liver tumor initiation. Cell 2021;184:5559-5576.e19. [Crossref] [PubMed]
- Boeynaems S, Alberti S, Fawzi NL, et al. Protein Phase Separation: A New Phase in Cell Biology. Trends Cell Biol 2018;28:420-35. [Crossref] [PubMed]
- Taniue K, Akimitsu N. Aberrant phase separation and cancer. FEBS J 2022;289:17-39. [Crossref] [PubMed]
- Cai D, Liu Z, Lippincott-Schwartz J. Biomolecular Condensates and Their Links to Cancer Progression. Trends Biochem Sci 2021;46:535-49. [Crossref] [PubMed]
- Tao R, Zhang S, Guo W, et al. Research Progress in the Role of Liquid-Liquid Phase Separation in Human Cancer. Sichuan Da Xue Xue Bao Yi Xue Ban 2024;55:24-30. [PubMed]
- Zhang X, Yuan L, Zhang W, et al. Liquid-liquid phase separation in diseases. MedComm (2020) 2024;5:e640.
- Zheng J, Wu Z, Qiu Y, et al. An integrative multi-omics analysis based on liquid-liquid phase separation delineates distinct subtypes of lower-grade glioma and identifies a prognostic signature. J Transl Med 2022;20:55. [Crossref] [PubMed]
- Qiu Y, Pan M, Chen X. A Liquid-Liquid Phase Separation-Related Gene Signature as Prognostic Biomarker for Epithelial Ovarian Cancer. Front Oncol 2021;11:671892. [Crossref] [PubMed]
- Zhang Y, Li J, Feng D, et al. Systematic Analysis of Molecular Characterization and Clinical Relevance of Liquid-Liquid Phase Separation Regulators in Digestive System Neoplasms. Front Cell Dev Biol 2022;9:820174. [Crossref] [PubMed]
- Lawlor DA, Harbord RM, Sterne JA, et al. Mendelian randomization: using genes as instruments for making causal inferences in epidemiology. Stat Med 2008;27:1133-63. [Crossref] [PubMed]
- Wu F, Huang Y, Hu J, et al. Mendelian randomization study of inflammatory bowel disease and bone mineral density. BMC Med 2020;18:312. [Crossref] [PubMed]
- Mao X, Mao S, Sun H, et al. Causal associations between modifiable risk factors and pancreatitis: A comprehensive Mendelian randomization study. Front Immunol 2023;14:1091780. [Crossref] [PubMed]
- Yan C, Niu Y, Li F, et al. System analysis based on the pyroptosis-related genes identifies GSDMC as a novel therapy target for pancreatic adenocarcinoma. J Transl Med 2022;20:455. [Crossref] [PubMed]
- Wang J, Meng F, Mao F. Single cell sequencing analysis and transcriptome analysis constructed the liquid-liquid phase separation(LLPS)-related prognostic model for endometrial cancer. Front Oncol 2022;12:1005472. [Crossref] [PubMed]
- Tang Q, Mao X, Chen Z, et al. Liquid-liquid phase separation-related gene in gliomas: FABP5 is a potential prognostic marker. J Gene Med 2023;25:e3517. [Crossref] [PubMed]
- Love MI, Huber W, Anders S. Moderated estimation of fold change and dispersion for RNA-seq data with DESeq2. Genome Biol 2014;15:550. [Crossref] [PubMed]
- Chen H, Boutros PC. VennDiagram: a package for the generation of highly-customizable Venn and Euler diagrams in R. BMC Bioinformatics 2011;12:35. [Crossref] [PubMed]
- Hemani G, Zheng J, Elsworth B, et al. The MR-Base platform supports systematic causal inference across the human phenome. Elife 2018;7:e34408. [Crossref] [PubMed]
- Bowden J, Davey Smith G, Burgess S. Mendelian randomization with invalid instruments: effect estimation and bias detection through Egger regression. Int J Epidemiol 2015;44:512-25. [Crossref] [PubMed]
- Bowden J, Davey Smith G, Haycock PC, et al. Consistent Estimation in Mendelian Randomization with Some Invalid Instruments Using a Weighted Median Estimator. Genet Epidemiol 2016;40:304-14. [Crossref] [PubMed]
- Burgess S, Scott RA, Timpson NJ, et al. Using published data in Mendelian randomization: a blueprint for efficient identification of causal risk factors. Eur J Epidemiol 2015;30:543-52. [Crossref] [PubMed]
- Hartwig FP, Davey Smith G, Bowden J. Robust inference in summary data Mendelian randomization via the zero modal pleiotropy assumption. Int J Epidemiol 2017;46:1985-98. [Crossref] [PubMed]
- Qin Q, Zhao L, Ren A, et al. Systemic lupus erythematosus is causally associated with hypothyroidism, but not hyperthyroidism: A Mendelian randomization study. Front Immunol 2023;14:1125415. [Crossref] [PubMed]
- Davey Smith G, Hemani G. Mendelian randomization: genetic anchors for causal inference in epidemiological studies. Hum Mol Genet 2014;23:R89-98. [Crossref] [PubMed]
- Cui Z, Feng H, He B, et al. Relationship Between Serum Amino Acid Levels and Bone Mineral Density: A Mendelian Randomization Study. Front Endocrinol (Lausanne) 2021;12:763538. [Crossref] [PubMed]
- Yu G, Wang LG, Han Y, et al. clusterProfiler: an R package for comparing biological themes among gene clusters. OMICS 2012;16:284-7. [Crossref] [PubMed]
- Qing J, Li C, Hu X, et al. Differentiation of T Helper 17 Cells May Mediate the Abnormal Humoral Immunity in IgA Nephropathy and Inflammatory Bowel Disease Based on Shared Genetic Effects. Front Immunol 2022;13:916934. [Crossref] [PubMed]
- Ramsay IS, Ma S, Fisher M, et al. Model selection and prediction of outcomes in recent onset schizophrenia patients who undergo cognitive training. Schizophr Res Cogn 2017;11:1-5. [Crossref] [PubMed]
- Li Y, Lu F, Yin Y. Applying logistic LASSO regression for the diagnosis of atypical Crohn's disease. Sci Rep 2022;12:11340. [Crossref] [PubMed]
- Heagerty PJ, Lumley T, Pepe MS. Time-dependent ROC curves for censored survival data and a diagnostic marker. Biometrics 2000;56:337-44. [Crossref] [PubMed]
- Sachs MC. plotROC: A Tool for Plotting ROC Curves. J Stat Softw 2017;79:2. [Crossref] [PubMed]
- Hänzelmann S, Castelo R, Guinney J. GSVA: gene set variation analysis for microarray and RNA-seq data. BMC Bioinformatics 2013;14:7. [Crossref] [PubMed]
- Davoudi F, Moradi A, Sadeghirad H, et al. Tissue biomarkers of immune checkpoint inhibitor therapy. Immunol Cell Biol 2024;102:179-93. [Crossref] [PubMed]
- Liu P, Xu H, Shi Y, et al. Potential Molecular Mechanisms of Plantain in the Treatment of Gout and Hyperuricemia Based on Network Pharmacology. Evid Based Complement Alternat Med 2020;2020:3023127. [Crossref] [PubMed]
- Wood LD, Canto MI, Jaffee EM, et al. Pancreatic Cancer: Pathogenesis, Screening, Diagnosis, and Treatment. Gastroenterology 2022;163:386-402.e1. [Crossref] [PubMed]
- Conroy T, Ducreux M. Adjuvant treatment of pancreatic cancer. Curr Opin Oncol 2019;31:346-53. [Crossref] [PubMed]
- Bear AS, Vonderheide RH, O'Hara MH. Challenges and Opportunities for Pancreatic Cancer Immunotherapy. Cancer Cell 2020;38:788-802. [Crossref] [PubMed]
- Kakiuchi N, Ogawa S. Clonal expansion in non-cancer tissues. Nat Rev Cancer 2021;21:239-56. [Crossref] [PubMed]
- Ahn JH, Davis ES, Daugird TA, et al. Phase separation drives aberrant chromatin looping and cancer development. Nature 2021;595:591-5. [Crossref] [PubMed]
- Nozawa RS, Yamamoto T, Takahashi M, et al. Nuclear microenvironment in cancer: Control through liquid-liquid phase separation. Cancer Sci 2020;111:3155-63. [Crossref] [PubMed]
- Wang B, Zhang L, Dai T, et al. Liquid-liquid phase separation in human health and diseases. Signal Transduct Target Ther 2021;6:290. [Crossref] [PubMed]
- An J, Ren S, Murphy SJ, et al. Truncated ERG Oncoproteins from TMPRSS2-ERG Fusions Are Resistant to SPOP-Mediated Proteasome Degradation. Mol Cell 2015;59:904-16. [Crossref] [PubMed]
- Bouchard JJ, Otero JH, Scott DC, et al. Cancer Mutations of the Tumor Suppressor SPOP Disrupt the Formation of Active, Phase-Separated Compartments. Mol Cell 2018;72:19-36.e8. [Crossref] [PubMed]
- Zuo L, Ding J, Qi Z. Visualizing carboxyl-terminal domain of RNA polymerase II recruitment by FET fusion protein condensates with DNA curtains. Biophys Rep 2022;8:80-9. [Crossref] [PubMed]
- Takayama KI, Inoue S. Targeting phase separation on enhancers induced by transcription factor complex formations as a new strategy for treating drug-resistant cancers. Front Oncol 2022;12:1024600. [Crossref] [PubMed]
- Doerr ME, Jones JI. The roles of integrins and extracellular matrix proteins in the insulin-like growth factor I-stimulated chemotaxis of human breast cancer cells. J Biol Chem 1996;271:2443-7. [Crossref] [PubMed]
- Zheng L, Pan C, Tian W, et al. Atp8a1 deletion increases the proliferative activity of hematopoietic stem cells by impairing PTEN function. Cell Oncol (Dordr) 2023;46:1069-83. [Crossref] [PubMed]
- Yount WJ, Fuller CR, Simmons JG. Distribution of IgG subclasses in human B lymphocytes: evidence for dual expression of subclasses in surface and cytoplasmic IgG in minor B lymphocyte subpopulations. J Immunol 1980;124:431-6. [Crossref] [PubMed]
- Jiang A, Zhou Y, Gong W, et al. CCNA2 as an Immunological Biomarker Encompassing Tumor Microenvironment and Therapeutic Response in Multiple Cancer Types. Oxid Med Cell Longev 2022;2022:5910575. [Crossref] [PubMed]
- Mahoney CW. High-throughput nonradioisotopic determination of binding of platelet-derived growth factor to platelet-derived growth factor receptor beta-extracellular domain using biotinylated ligand with enzyme-linked immunosorbent assay. Anal Biochem 1999;276:106-8. [Crossref] [PubMed]
- Lu Y, Chen Q, Zhu S, et al. Hypoxia promotes immune escape of pancreatic cancer cells by lncRNA NNT-AS1/METTL3-HuR-mediated ITGB1 m(6)A modification. Exp Cell Res 2023;432:113764. [Crossref] [PubMed]
- Iwatate Y, Yokota H, Hoshino I, et al. Transcriptomic analysis reveals high ITGB1 expression as a predictor for poor prognosis of pancreatic cancer. PLoS One 2022;17:e0268630. [Crossref] [PubMed]
- Wang G, Ni X, Wang J, et al. METTL3-mediated m6A methylation of PYGB facilitates pancreatic ductal adenocarcinoma progression through the activation of NF-κB signaling. Pathol Res Pract 2023;248:154645. [Crossref] [PubMed]
- Sadrian B, Cheng TW, Shull O, et al. Rap1gap2 regulates axon outgrowth in olfactory sensory neurons. Mol Cell Neurosci 2012;50:272-82. [Crossref] [PubMed]
- Neumüller O, Hoffmeister M, Babica J, et al. Synaptotagmin-like protein 1 interacts with the GTPase-activating protein Rap1GAP2 and regulates dense granule secretion in platelets. Blood 2009;114:1396-404. [Crossref] [PubMed]
- Gallo M, Adinolfi V, Morviducci L, et al. Early prediction of pancreatic cancer from new-onset diabetes: an Associazione Italiana Oncologia Medica (AIOM)/Associazione Medici Diabetologi (AMD)/Società Italiana Endocrinologia (SIE)/Società Italiana Farmacologia (SIF) multidisciplinary consensus position paper. ESMO Open 2021;6:100155. [Crossref] [PubMed]
- Zhou Y, Jin Z, Wang C. Glycogen phosphorylase B promotes ovarian cancer progression via Wnt/β-catenin signaling and is regulated by miR-133a-3p. Biomed Pharmacother 2019;120:109449. [Crossref] [PubMed]
- Xiao L, Wang W, Huangfu Q, et al. PYGB facilitates cell proliferation and invasiveness in non-small cell lung cancer by activating the Wnt-β-catenin signaling pathway. Biochem Cell Biol 2020;98:565-74. [Crossref] [PubMed]
- Xia B, Zhang K, Liu C. PYGB Promoted Tumor Progression by Regulating Wnt/β-Catenin Pathway in Gastric Cancer. Technol Cancer Res Treat 2020;19:1533033820926592. [Crossref] [PubMed]
- Allen BM, Hiam KJ, Burnett CE, et al. Systemic dysfunction and plasticity of the immune macroenvironment in cancer models. Nat Med 2020;26:1125-34. [Crossref] [PubMed]
- Wang S, Li Y, Xing C, et al. Tumor microenvironment in chemoresistance, metastasis and immunotherapy of pancreatic cancer. Am J Cancer Res 2020;10:1937-53. [PubMed]
- Binnewies M, Roberts EW, Kersten K, et al. Understanding the tumor immune microenvironment (TIME) for effective therapy. Nat Med 2018;24:541-50. [Crossref] [PubMed]
- Nizri E, Bar-David S, Aizic A, et al. Desmoplasia in Lymph Node Metastasis of Pancreatic Adenocarcinoma Reveals Activation of Cancer-Associated Fibroblasts Pattern and T-helper 2 Immune Cell Infiltration. Pancreas 2019;48:367-73. [Crossref] [PubMed]
- Galvez-Cancino F, Simpson AP, Costoya C, et al. Fcγ receptors and immunomodulatory antibodies in cancer. Nat Rev Cancer 2024;24:51-71. [Crossref] [PubMed]
- Biber G, Ben-Shmuel A, Noy E, et al. Targeting the actin nucleation promoting factor WASp provides a therapeutic approach for hematopoietic malignancies. Nat Commun 2021;12:5581. [Crossref] [PubMed]
- Cekic S, Huriyet H, Hortoglu M, et al. Increased radiosensitivity and impaired DNA repair in patients with STAT3-LOF and ZNF341 deficiency, potentially contributing to malignant transformations. Clin Exp Immunol 2022;209:83-9. [Crossref] [PubMed]
- Ali A, Mahla SB, Reza V, et al. Predicting the possible effect of miR-203a-3p and miR-29a-3p on DNMT3B and GAS7 genes expression. J Integr Bioinform 2021;19:20210016. [Crossref] [PubMed]
- Mo WY, Cao SQ. MiR-29a-3p: a potential biomarker and therapeutic target in colorectal cancer. Clin Transl Oncol 2023;25:563-77. [Crossref] [PubMed]
(English Language Editor: L. Huleatt)