Analyzing the role of TM4SF1 expression in pancreatic adenocarcinoma: understanding prognostic implications and therapeutic opportunities
Highlight box
Key findings
• This study further revealing the molecular landscape of pancreatic adenocarcinoma (PAAD), emphasizing TM4SF1 as a key regulator and potential therapeutic target.
What is known and what is new?
• TM4SF1 was identified as significantly upregulated in PAAD.
• Lower TM4SF1 linked to enhanced anti-tumor immunity in PAAD. The least absolute shrinkage and selection operator regression analysis of the differentially expressed genes between the PAAD and normal tissues identified five genes that were closely related to PAAD prognosis (i.e., BPIFB4, PLEKHN1, CPTP, DVL1, and DDR1) and a prognostic model was constructed, which showed robust associations with patient survival outcomes, validated across diverse patient cohorts.
What is the implication, and what should change now?
• Prognostic model was validated in an independent cohort, showing clinical applicability, further research is warranted to elucidate the clinical utility of targeting TM4SF1 and other identified genes in PAAD management.
Introduction
Pancreatic adenocarcinoma (PAAD) is a particularly aggressive form of cancer, and ranks among the leading causes of global cancer-related deaths (1-3). Alarmingly, PAAD accounts for around 90% of all pancreatic cancer cases (4). Its 5-year survival rate is extremely low, among the lowest of all cancer types, which is one of the most distressing aspects of PAAD (5,6). This stark statistic underscores the urgency of addressing PAAD as a significant public health concern (7,8). The insidious nature of PAAD further complicates its prognosis and treatment (9,10). Its clinical manifestations are often ambiguous, overlapping with symptoms of other pancreatic disorders (11,12). Such non-specific presentations frequently result in delayed diagnoses, reducing the chances of timely and effective interventions (13,14). Despite considerable strides in oncology, PAAD remains a formidable challenge. The primary hurdles lie in the late-stage diagnosis common to many patients and the intricate mechanisms driving the progression of the disease (15-17). A more profound understanding of PAAD is crucial, not only to improve diagnostic techniques but also to pioneer more effective therapeutic strategies. Motivated by this pressing need, our study delved deep into the intricacies of PAAD to identify novel biomarkers and discover potentially transformative treatment pathways.
The development and progression of PAAD are often attributed to genetic mutations and dysregulated signaling pathways (18,19). Common mutations include KRAS, TP53, and CDKN2A mutations, but the complete landscape of genetic alterations and how they influence disease progression remains incompletely understood (20-22). These alterations lead to changes in cellular processes like cell proliferation, apoptosis, and DNA repair, contributing to tumor growth and metastasis (23,24). Presently, diagnosing PAAD primarily relies on imaging techniques and biopsy, which have limitations in terms of their sensitivity and specificity (25,26). The treatments include surgical resection, chemotherapy, and radiation therapy (25,27). However, due to the late-stage diagnosis in many cases, surgical options are often not viable, and current therapies often prove ineffective (28).
Recent advancements have shed light on the intricate relationship between the immune system and PAAD. Diverse immune cells play contrasting roles in tumor progression—some suppress it, while others promote it (29-31). Deepening our understanding of immune cell dynamics in PAAD could inform novel therapeutic strategies. However, PAAD treatment is currently challenged by the absence of reliable prognostic biomarkers, which limits personalized therapy and accurate risk assessment. Thus, the identification of molecular markers intimately linked to patient survival is pivotal in shaping treatment approaches.
Building upon the extensive body of research on TM4SF1 and its role in PAAD (32-34), this study ventured into uncharted territory. It sought to explore differential gene expression in PAAD tissues, focusing particularly on novel aspects of TM4SF1’s interaction with the disease. Our approach was distinct in its comprehensive analysis of genetic landscapes, immune cell infiltration patterns, and prognostic biomarkers, which was also coupled with an evaluation of drug sensitivities specific to PAAD. This study not only delved into the underexplored facets of TM4SF1 in the context of PAAD but also sought to bridge existing knowledge gaps. By integrating cutting-edge genomic and immunological analyses, we sought to provide unique insights that could revolutionize current understandings of PAAD and its management. Our findings might serve as a cornerstone for future therapeutic interventions, potentially altering the course of personalized treatment in PAAD. We present this article in accordance with the TRIPOD reporting checklist (available at https://jgo.amegroups.com/article/view/10.21037/jgo-24-564/rc).
Methods
Data collection and preprocessing
We sourced transcriptomic data for PAAD, along with pertinent clinical information, from the following two prominent databases: The Cancer Genome Atlas (TCGA; available at TCGA Portal) and the Gene Expression Omnibus (GEO), accessible at National Center for Biotechnology Information (NCBI) website. Our validation cohort comprised 80 PAAD samples from the GSE85916 series, while our training cohort comprised 177 PAAD samples from TCGA. In an effort to ensure data comparability, we addressed batch effects and performed normalization on the transcriptomic data from both TCGA and GEO using the “ComBat” algorithm, a feature of the sva package. This exclusion criteria extended to entries lacking adequate details on gender, age, stage, and grade, as well as those with unrecorded survival times, including instances of 0-day survival.
Gene set enrichment analyses (GSEAs)
Our investigation into the functional roles of messenger RNAs (mRNAs) was informed by GSEAs, guided by the Kyoto Encyclopedia of Genes and Genomes (KEGG) and Gene Ontology (GO) standards. We implemented these analyses using a suite of R packages; that is, “clusterProfiler”, “enrichplot”, “limma”, “ggplot2”, and “org.Hs.eg.db”. “limma” is utilized to generate lists of differentially expressed genes as input data for GSEA, while “org.Hs.eg.db” facilitates the conversion of gene IDs. Subsequently, “clusterProfiler” is employed to conduct the GSEA analysis, and “enrichplot” alongside “ggplot2” are harnessed for the visualization of the analysis outcomes. Additionally, to gain deeper insights into the pathways and functions of differentially expressed genes (DEGs), we employed a GSEA using the same R packages. Reference gene sets “c2.cp.kegg.v7.4.symbols.gmt” and “h.all.v7.4.symbols.gmt” were used. Pathway enrichment was considered significant based on the following stringent criteria: a false discovery rate adjusted q value less than 0.25, a nominal P value less than 0.05, and a normalized enrichment score greater than 1.
Immune infiltration analysis
We leveraged the xCell algorithm, incorporating machine-learning techniques, to derive signatures from 64 immune and stromal cell types. The R package “estimate”, which includes the ESTIMATE algorithm, facilitated our analysis of variances in stromal, immune, and ESTIMATE scores. The single-sample gene set enrichment analysis (ssGSEA) in the “GSVA” R package was used to compute the enrichment scores. These scores represented the extent of immune cell infiltration into tumors and their functional roles. We visualized the results using R packages “limma”, “ggpubr”, and “reshape2”.
Construction and validation of a prognostic model
Recently, machine learning has emerged as a transformative tool in biomedical research (35), especially in the identification of biomarkers for disease prognosis. Among various machine-learning techniques, the least absolute shrinkage and selection operator (LASSO) method stands out for its efficacy in processing complex biological data (36). Our prognostic model was developed by selecting prognostically relevant DEGs using the LASSO-Cox regression method. The risk score for each patient was calculated as follows: Risk score = Σi Coefficient (mRNAi) × Expression (mRNAi). We employed the “surv_cutpoint” function of the “survminer” R package to determine the optimal cut-off value. This function computes metrics based on maximally selected rank statistics and identifies the most distinct cut-off value for survival rate differences after multiple simulations. Patients were then stratified into high- or low-risk groups based on this cut-off value. The Kaplan-Meier (K-M) method was used to compare overall survival (OS) between these groups. We further validated our findings in the GSE85916 cohort to ensure the robustness of our results.
Association among somatic mutation and risk score
For the single-nucleotide variant (SNV) data in multiple alignment format (MAF) from TCGA database, we used the “maftools” package in the R environment. This software also facilitated the visualization of genes exhibiting the highest mutation rates.
Drug sensitivity analysis
The half-maximal inhibitory concentration (IC50), which represents the concentration at which 50% inhibition is achieved, was calculated for 138 drugs using the R application “pRRophetic”. Correlation tests were conducted between each drug’s IC50 and the risk scores, yielding correlation coefficients. These correlations were visualized in a bar chart to highlight the differences between the drugs and risk scores.
Analysis of TM4SF1 expression using the Gene Expression Profiling Interactive Analysis (GEPIA) database
We used the GEPIA database (http://gepia.cancer-pku.cn/) (34) to clarify the clinical relevance and predictive importance of TM4SF1 expression in PAAD. This database compiles tumor and normal tissue data from TCGA database. Based on the median TM4SF1 expression level, the patients were allocated to high- and low-expression groups for comparisons. The prognostic impact was assessed using hazard ratios (HRs), 95% confidence intervals (CIs), and log-rank P values. The threshold for statistical significance regarding the prognostic value of TM4SF1 expression was set at a P value of less than 0.05.
Culturing and genetic manipulation of PAAD cell lines
The PAAD cell lines were obtained from the Chinese Academy of Sciences Cell Bank, Shanghai, China. These cell lines included hTERT-HPNE, AsPC-1, CFPAC-1, MIAPaCa-2, PANC-1, and PaTu8988t. Dulbecco’s Modified Eagle Medium was used to create these cell lines, along with 10% fetal bovine serum and 100-µg/mL penicillin-streptomycin supplements. The cultures were maintained at 37 ℃ in a moist environment with 5% carbon dioxide. For the genetic interference and overexpression studies, small interfering RNAs (siRNAs) targeting TM4SF1 and a plasmid encoding the TM4SF1 cDNA were obtained from GenePharma (Shanghai, China). In accordance with the manufacturer’s instructions, Lipo8000 (#C0533, Beyotime, Shanghai, China) was used to transfect these genetic constructs into the cell lines. The sequences for the siRNAs used were: negative control (5'-UUCUCCGAACGUGUCACGUTT-3'), si-TM4SF1#1 (5'-GCUAUGGGAAGUGUGCACGAUGCAU-3'), si-TM4SF1#2 (5'-AAGUAAAGCAAAAUAUUAGCC-3'), and si-TM4SF1#3 (5'-UUUAUUACUUGAAUAAGACAC-3').
Clinical samples
Between January 2020 and December 2023, normal and pancreatic cancer tissue samples (n=10) were collected from patients undergoing surgery at Gaochun People’s Hospital. Among the 10 patients, 7 were males, aged 70±5.3 years; 3 were females, aged 69±1.0 years. The clinicopathologic findings were all pancreatic ductal adenocarcinoma. After the surgical specimen is separated from the body, the technician examines the surgical specimen according to the pathological standard process, cuts the normal pancreatic tissue and pancreatic cancer tissue according to the size of 5 mm × 5 mm × 5 mm, and then puts the surgical specimen into the cryopreservation tube, and then puts it into liquid nitrogen for preservation, this process must be completed in less than 20 minutes. The study was carried out in compliance with the 2013 revision of the Declaration of Helsinki. The study was approved by the Ethics Committee of Gaochun People’s Hospital (No. 2020002), and all patients signed written informed consent forms.
Western blot analysis
Cellular lysates were prepared by incubating the cells on ice with a radioimmunoprecipitation assay buffer (Thermo Fisher, Rockford, USA) supplemented with phenylmethylsulfonyl fluoride (#ST506, Beyotime), enabling total protein extraction from the samples. The concentration of proteins in the samples was determined using a bicinchoninic acid assay kit (Thermo Fisher). For protein separation, 40 µg of protein from each sample was resolved on sodium dodecyl-sulfate polyacrylamide gel electrophoresis gels, followed by electrophoretic transfer to 0.45-µm polyvinylidene fluoride membranes (#IPVH00010, Merck Millipore, Tullagreen, Ireland) at a steady 250-mA current. Subsequent to the electrophoretic transfer, the membranes were blocked for 2 hours using Tris-buffered saline with Tween (TBST), which contained 5% non-fat dry milk. Primary antibodies against β-actin (#AF0003, Beyotime) and TM4SF1 (#NBP1-76549, NOVUS, Colorado, USA) were incubated on the membranes overnight at 4 ℃. Following this incubation, the membranes were washed in TBST and then incubated with horseradish peroxidase-conjugated secondary antibodies for 2 hours at room temperature. The detection of protein bands was facilitated using an enhanced chemiluminescence detection kit (#P0018S, Beyotime). Band intensities were analyzed using ImageJ software (version 1.50b; ImageJ Software Inc., Maryland, MD, USA) from the National Institutes of Health.
Total RNA extraction and quantification of mRNA expression via quantitative reverse transcription polymerase chain reaction (qRT-PCR)
In this investigation, pancreatic cancer and normal tissues from a cohort of 10 patients were used to ascertain the mRNA expression differences of TM4SF1, BPIFB4, CPTP, DVL1, PLEKHN1, and DDR1 between pancreatic cancer and normal tissues. Each tissue sample, weighing 30 mg, was placed into a grinding tube along with two RNA-free grinding beads and 200 µL of Trizol reagent (#R0016, Beyotime). The samples were then subjected to low-temperature grinding for 60 seconds using a high-frequency grinding machine (Thermo Fisher) until tissue homogenization was achieved. Subsequently, 800 µL of Trizol reagent was added to facilitate further tissue lysis. Following the manufacturer’s guidelines, chloroform, isopropyl alcohol, and anhydrous ethanol were sequentially introduced into the grinding tube to precipitate RNA, followed by concentration determination and the subsequent procedural steps.
The total RNA from the cells was extracted using the Trizol reagent in accordance with the manufacturer’s guidelines. The quality and concentration of the extracted RNA were determined using a NanoDrop One C micro-spectrophotometer (#AZY2124606, Thermo Scientific, Waltham, USA). The integrity of the RNA was verified to ensure its suitability for downstream applications. The expression levels of TM4SF1 mRNA were measured using real-time qRT-PCR, with glyceraldehyde 3-phosphate dehydrogenase (GAPDH) serving as an internal reference for normalization. The following specific primer sequences were used for amplification: TM4SF1, forward primer (5'-CCTCTTGGCTCTTGGTGGAA-3') and reverse primer (5'-AGCAGTCATATTGCTGTTGGTG-3'); BPIFB4, forward primer (5'-AGACCAGCCTCAACCTCAGAAC-3') and reverse primer (5'-ACGCCAGAACCCAGCACAG-3'); CPTP, forward primer (5'-AAAGTCGTCCTGGTCAGTTTCAAG-3') and reverse primer (5'-GTCCTTGGAGATGAATGAGAAGATGG-3'); DVL1, forward primer (5'-CCATCGCCAATGCCGTCATC-3') and reverse primer (5'-ACCGTGTGCCGCAGGAAG-3'); PLEKHN1, forward primer (5'-TGGAGGAGAAGGAGAAGCAGATC-3') and reverse primer (5'-ACAGCAGCCAGTGACCGTAG-3'); DDR1, forward primer (5'-CATCTTTACTGCTGCTGCTCTTGG-3') and reverse primer (5'-GCACTTGGCAGGATCAAAATGTC-3'); GAPDH, forward primer (5'-GGAGCGAGATCCCTCCAAAAT-3') and reverse primer (5'-GGCTGTTGTCATACTTCTCATGG-3'). These primers were designed to amplify their respective targets efficiently to ensure the accuracy of the gene expression analysis.
Cell viability assessment via Cell Counting Kit-8 (CCK-8) assay
Cell viability was evaluated using the CCK-8 (#C0037, Beyotime) in accordance with the provided instructions. The cells were plated in 100 µL of culture media in 96-well plates (#11510, Labselect, Beijing, China) at a density of 5×103 per well and incubated for 24 hours. The plates were then incubated for a further 2 hours to allow for color development after 10 µL of CCK-8 solution had been added to each well. The experiments were conducted in triplicate to ensure consistency. A microplate reader (Bio-Rad, Hercules, CA, USA) measured absorbance at 450 nm. Blank wells were used as the baseline for normalization. Cell proliferation was quantified based on the absorbance readings. Additionally, the CCK-8 assay was employed to evaluate the sensitivity of the PAAD cells to gemcitabine by determining the IC50. 96-well plates were seeded with 1×104 cells per well of PANC-1, and the plates were incubated for 24 hours before being exposed to various concentrations of gemcitabine (0, 1, 5, 10, 20, and 40 µmol/L) provided by Qilu Pharmaceutical Co., Ltd. (Jinan, China) for 72 hours, after which the IC50 value was ascertained.
Colony formation proficiency analysis
The clonogenic capacity of the cells was evaluated by plating 800 cells per 6-cm dish and incubating them at 37 ℃. The culture medium was replenished periodically over 10–15 days, dependent on the growth kinetics of each cell line. Following incubation, the cells were rinsed twice with phosphate-buffered saline, stained with 0.1% crystal violet (#DZ0053, Leagene, Hefei, China), and fixed with 4% paraformaldehyde. Using ImageJ software (ImageJ Software Inc.), colonies with a minimum of 50 cells were counted to determine the cells’ capacity for cloning.
Assessment of cell invasion and migration
Using an 8-µm pore size Transwell insert (#PTEP24H48, Millipore, USA) and a Matrigel-coated (#0827045, ABW, Shanghai, China) invasion chamber test, the invasive and migratory potential of the PAAD cells was assessed. For this purpose, 20,000 PAAD cells were plated into the upper compartment of the Transwell setup in a 24-well plate (#11310, Labselect). The non-invading cells were gently scraped from the upper surface of the membrane after being incubated for 24 hours at 37 ℃. After the cells reached the bottom surface, they were fixed for 30 minutes using 4% paraformaldehyde and stained with crystal violet for 20 minutes. Using counting and microscope imaging (10×), the number of cells that moved through the holes to the lower side of the insert was determined.
Quantification of cellular wound healing
Confluent monolayers of cells in six-well plates were subjected to a scratch-wound assay to assess their wound closure capabilities. A sterile 1,000-µL pipette tip simulated a wound by making a linear scratch on the cell monolayer. The initial wound (at “0 hours”) and the extent of closure after 48 hours were documented using microscope imaging (5×) and ImageJ software. The following formula was used to determine the rate of wound healing: [(width of the scratch at ‘0 hours’ − width at ‘48 hours’)/width at ‘0 hours’] × 100%. This metric provided a quantitative measure of the cell’s ability to migrate and fill the wound area over the designated time period.
Statistical analysis
The R programming language was used for all the statistical analyses. We used survival and survminer R packages to conduct univariate and multivariate Cox regression analyses. A P value <0.05 was used to determine prognostic significance.
Results
Functional analysis of DEGs
Figure 1 shows the workflow diagram for our study. Figure 2A shows a volcano plot illustrating the distribution of the DEGs between the PAAD and normal tissues. Notably, a significant subset of genes exhibited upregulation in normal tissues, in contrast to the predominant upregulation observed in PAAD. TM4SF1 is prominently upregulated in tumor tissues and represents the most significant DEG between tumor and normal tissues. Our functional enrichment analysis of the DEGs based on GO and KEGG revealed that the upregulated DEGs were primarily linked to processes such as skin development, wound healing, tight junction formation, cell-cell junctions, and cytokine-cytokine receptor interactions. Conversely, the downregulated DEGs were predominantly involved in pathways such as trans-synaptic signaling regulation, presynapses, and neuroactive ligand-receptor interactions (Figure 2B). Further, the GSEA revealed significant upregulation in pathways, including hepatitis C, tight junction, pancreatic secretion, human papillomavirus infection, and retinol metabolism. Conversely, pathways like morphine addiction, circadian entrainment, and GABAergic synapses were notably downregulated (Figure 2C).
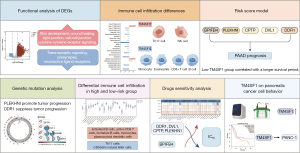
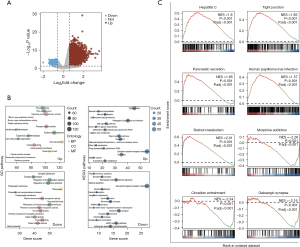
Immune cell infiltration differences between the high- and low-TM4SF1 expression groups
Patients with PAAD were allocated to high and low TM4SF1 expression groups based on the median TM4SF1 gene expression to analyze differences in immune cell infiltration. As Figure 3A shows, there was a significant correlation between TM4SF1 expression levels and immune cell infiltration, with a notable positive association observed with type 17 T helper cells and CD56 natural killer cells, and a negative correlation observed with monocytes, eosinophils, activated cluster of differentiation (CD)8 T cells, and activated B cells. This correlation was validated by a further analysis (Figure 3B). Additionally, as Figure 3C shows, the ssGSEA results indicated an elevated presence of immune cells, such as activated B cells, activated CD8 T cells, eosinophils, and monocytes, in the low TM4SF1 expression group, while CD56 natural killer cells and type 17 T helper cells were more prevalent in the high TM4SF1 group. The immunological, stromal, and ESTIMATE scores for the two risk groups revealed that the immunological score of the low-risk group was significantly higher than that of the high-risk group (Figure 3D; P<0.05), suggesting more pronounced immune cell infiltration.
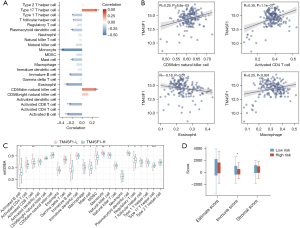
Construction and validation of the risk-score model
The LASSO regression analysis of the DEGs between high and low TM4SF1 expression groups identified five genes that were closely related to PAAD prognosis (i.e., BPIFB4, PLEKHN1, CPTP, DVL1, and DDR1) (Figure 4A,4B). Risk scores were calculated based on their expression levels and coefficients to categorize the PAAD patients into high- and low-risk groups. The median value of these risk scores was used as the threshold. As the K-M curve in Figure 4C shows, there was a significant survival difference between the two groups, with the low-risk group showing a notably better prognosis than the high-risk group. As Figure 4D shows, scatterplots further illustrated this trend, revealing a higher survival propensity in the low-risk group and a tendency towards mortality in the high-risk group. The robustness of these results was confirmed in the validation set (Figure 4E), which revealed a significant survival difference favoring the low-risk group. Survival status scatterplots for PAAD patients (Figure 4F) mirrored these findings, suggesting a survival advantage for low-risk patients and a higher mortality risk for high-risk patients.
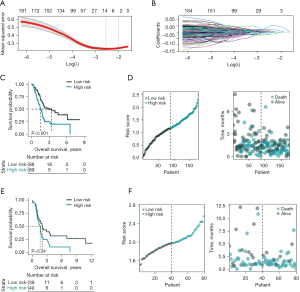
Genetic mutation analysis
The chromosomal positions of the five key genes in the model (i.e., BPIFB4, PLEKHN1, CPTP, DVL1, and DDR1) were examined (Figure 5A). The forest plot in Figure 5B elucidated the relationship between the expression of these genes and PAAD prognosis. DDR1 expression emerged as a significant protective factor, while PLEKHN1 expression was linked to poorer outcomes, which suggests that PLEKHN1 plays a tumor-promoting role, and exerts a suppressive function for. A correlation analysis between these key genes revealed significant positive correlations among certain gene pairs, while BPIFB4 exhibited a negative correlation with PLEKHN1 (Figure 5C). Figure 5D,5E showed the genetic mutation landscape of PAAD patients, highlighting the predominance of C>T transitions and the frequency of various mutation types. Additionally, we obtained cancer and normal tissue samples from 10 patients to examine the mRNA expression differences of TM4SF1, BPIFB4, CPTP, DVL1, PLEKHN1, and DDR1 between cancer and normal tissues. The mRNA expression levels of these six genes were significantly higher in the cancer tissues than the normal tissues (P<0.05, Figure 5F).
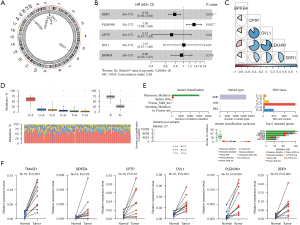
Differential immune cell infiltration in high- and low-risk groups
A ssGSEA was conducted to examine immune cell infiltration differences between the high- and low-risk groups (Figure 6A). The low-risk group exhibited significantly higher levels of several immune cells, while the high-risk group showed a marked presence of specific T helper cells and CD56dim natural killer cells (Figure 6B-6K). As the bubble chart in Figure 6L shows, we also examined the relationship between immune cell infiltration levels and the expression of the five key genes. As Figure 6M,6N show, the xCell algorithm revealed differences in immune cell infiltration between the risk groups, underscoring the association between enhanced anti-tumor immune activity and favorable prognosis in the low-risk group.
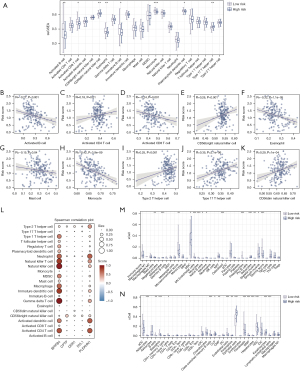
Drug sensitivity analysis
The study calculated the IC50 values for various drugs and examined their correlation with the genes included in the model (Figure 7A). The results indicated that, apart from BPIFB4, the other four genes (DDR1, DVL1, CPTP, PLEKHN1) showed a positive correlation with the drug IC50 values, suggesting their influence on tumor cell sensitivity to specific therapies. Figure 7B-7G displays the correlation coefficients between the risk scores and IC50 values of different drugs, revealing a moderate correlation and implying a potential relationship between high-risk status and increased sensitivity to certain treatments.
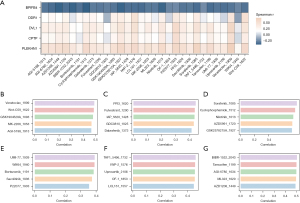
Functional effect of TM4SF1 on pancreatic cancer cell behavior
We searched GTEx and TCGA databases through GEPIA, revealed a considerable increase in TM4SF1 mRNA levels in the tumor tissues compared to the nearby normal tissues (Figure 8A), and high TM4SF1 expression was associated with decreased OS in patients (Figure 8B). As the Western blot results in Figure 8C,8D show, the TM4SF1 protein was increased in both the tumor samples (n=5) and cancer cell lines. Figure 8E shows TM4SF1 mRNA expression across various cell lines; among the cell lines with higher expression of TM4SF1 than hTERT-HPNE cell line, TM4SF1 was the highest expression in PANC-1 cell line and the lowest in CFPAC-1 cell line. Figure 8F,8G show a considerable decrease in PANC-1 cell mRNA and protein levels after TM4SF1 knockdown (siTM4SF1), siTM4SF1#3 was found to have the most significant knockdown effect on TM4SF1 mRNA and protein levels. The knockdown’s functional consequences are depicted in the subsequent panels: Figure 8H,8I shows that the cell proliferation and colony formation ability of the PANC-1 cells was suppressed post-siTM4SF1 treatment. Figure 8J reveals the enhanced sensitivity of these cells to the chemotherapeutic agent gemcitabine. The migration and invasion assay results in Figure 8K,8L show that siTM4SF1 treatment markedly reduced these capabilities in PANC-1 cells. Conversely, Figure 8M shows the overexpression of TM4SF1 (oeTM4SF1) in the CFPAC-1 cells and confirms that it was overexpressed at the protein level. Figure 8N,8O shows that oeTM4SF1 increased cell proliferation and colony formation. Lastly, Figure 8P,8Q shows that oeTM4SF1 increased the capacity of CFPAC-1 cells for migration and invasion, indicating that TM4SF1 is a key factor in promoting oncogenic tendencies in these pancreatic cancer models.
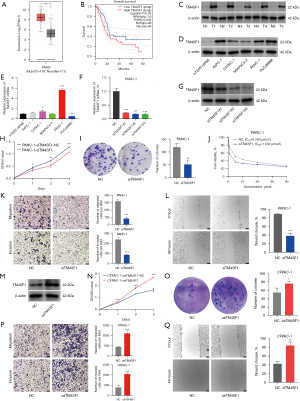
Discussion
The current study provided substantial insights into the genetic landscape, immune cell infiltration patterns, prognostic biomarkers, and drug sensitivities of PAAD. In the discussion section, these findings are contextualized with other existing research, allowing for a comprehensive understanding of their significance, potential implications, and areas for future study.
Our research identified specific genetic mutations and alterations distinctively associated with PAAD, expanding on previous research. Unlike a study that has primarily focused on common mutations like KRAS, TP53, and CDKN2A, this study delved deeper into lesser-known genetic factors (37). Identifying these additional mutations filled a critical gap in understanding the genetic heterogeneity of PAAD and could result in the development of targeted treatment strategies.
The study’s exploration of immune cell infiltration patterns in PAAD tissues builds on recent work in the field. Our findings indicate a more complex interaction between immune cells and tumor tissues than previously described (38). Unlike the generalized observation of immune suppression in PAAD, we identified specific immune cell subsets that might have contrasting roles. This nuanced understanding will contribute to the ongoing debate on immunotherapy’s potential efficacy in treating PAAD and may help more targeted approaches to be designed.
Our research significantly enhances personalized oncology medicine by identifying novel prognostic biomarkers for PAAD. Building on a previous study that has pinpointed specific proteins and genes as prognostic indicators (39), our study extended this knowledge base by introducing a more comprehensive set of biomarkers. This expansion not only reinforces existing findings but also aims to increase the precision in predicting patient outcomes, thereby facilitating more personalized treatment strategies and patient categorization. Notably, we found that TM4SF1 was upregulated in PAAD and linked to lower patient survival rates, thus we established it as a crucial prognostic marker for disease severity. Our study showed the significant role of TM4SF1 in promoting tumor growth and cellular proliferation. We confirmed that TM4SF1 was highly expressed in tumor tissues through the qRT-PCR analysis of cancer and normal tissues from patients. We observed a marked reduction in cell growth and colony formation following TM4SF1 suppression, indicating its key role in cancer cell survival and proliferation. Conversely, the oeTM4SF1 significantly enhanced these functions, underlining its importance in tumor development. Additionally, our research highlighted the effect of TM4SF1 on critical cancer cell behaviors, particularly migration and invasion, which are vital for metastasis. The inhibition of TM4SF1 led to a noticeable decrease in these capabilities, while its overexpression markedly increased them, which suggests that TM4SF1 may play a role in aiding cancer cells’ transition to a more aggressive phenotype.
In summary, our findings position TM4SF1 as a central player in PAAD progression. By establishing a direct correlation between TM4SF1 expression and malignant cell behaviors, our findings suggest that targeting TM4SF1 could disrupt essential cancer progression processes, such as proliferation, migration, and invasion. Thus, TM4SF1 could serve as a promising therapeutic target, and may have the potential to halt or slow the progression of PAAD. This aligns closely with the goals of personalized medicine and targeted therapy in oncological research.
The investigation into drug sensitivities in PAAD is an area that sets our study apart from many others. Our robust analysis identified potential drug targets that align with the genetic alterations observed in PAAD tissues. This aligns with recent precision medicine approaches but goes further by correlating specific genetic mutations with drug responsiveness. Our results pave the way for more personalized and effective treatment regimens that differ from conventional chemotherapy.
Our study provided important insights but also had some limitations. Despite being large, the sample size might not fully represent the intricacy of PAAD. The study also relied on specific methodologies that might benefit from further validation through different experimental designs. Future research should focus on validating these results in more extensive and diverse patient populations and via preclinical and clinical studies. Collaborative efforts with other research groups could lead to a more standardized approach to studying PAAD.
Conclusions
In conclusion, this study represents a critical advancement in understanding PAAD in terms of its genetic underpinnings and clinical applications. By contrasting our findings with existing research, we provided a more nuanced and comprehensive view of the disease, opening up new possibilities for targeted therapies and personalized treatment strategies. The novel insights into immune cell interactions, prognostic markers, and drug sensitivities contribute to an emerging paradigm shift in PAAD management and offer hope for improved patient outcomes. The ongoing efforts in this direction represent an exciting era of PAAD research that promises a deeper understanding and more effective intervention strategies.
Acknowledgments
Funding: This study was supported in part by grants from
Footnote
Reporting Checklist: The authors have completed the TRIPOD reporting checklist. Available at https://jgo.amegroups.com/article/view/10.21037/jgo-24-564/rc
Data Sharing Statement: Available at https://jgo.amegroups.com/article/view/10.21037/jgo-24-564/dss
Peer Review File: Available at https://jgo.amegroups.com/article/view/10.21037/jgo-24-564/prf
Conflicts of Interest: All authors have completed the ICMJE uniform disclosure form (available at https://jgo.amegroups.com/article/view/10.21037/jgo-24-564/coif). The authors have no conflicts of interest to declare.
Ethical Statement: The authors are accountable for all aspects of the work in ensuring that questions related to the accuracy or integrity of any part of the work are appropriately investigated and resolved. The study was carried out in compliance with the 2013 revision of the Declaration of Helsinki. The study was approved by the Ethics Committee of Gaochun People’s Hospital (No. 2020002), and all patients signed written informed consent forms.
Open Access Statement: This is an Open Access article distributed in accordance with the Creative Commons Attribution-NonCommercial-NoDerivs 4.0 International License (CC BY-NC-ND 4.0), which permits the non-commercial replication and distribution of the article with the strict proviso that no changes or edits are made and the original work is properly cited (including links to both the formal publication through the relevant DOI and the license). See: https://creativecommons.org/licenses/by-nc-nd/4.0/.
References
- Goral V. Pancreatic Cancer: Pathogenesis and Diagnosis. Asian Pac J Cancer Prev 2015;16:5619-24. [Crossref] [PubMed]
- Mizrahi JD, Surana R, Valle JW, et al. Pancreatic cancer. Lancet 2020;395:2008-20. [Crossref] [PubMed]
- Park W, Chawla A, O'Reilly EM. Pancreatic Cancer: A Review. JAMA 2021;326:851-62. [Crossref] [PubMed]
- Ryan DP, Hong TS, Bardeesy N. Pancreatic adenocarcinoma. N Engl J Med 2014;371:1039-49. [Crossref] [PubMed]
- Jiang Y, Sohal DPS. Pancreatic Adenocarcinoma Management. JCO Oncol Pract 2023;19:19-32. [Crossref] [PubMed]
- Vareedayah AA, Alkaade S, Taylor JR. Pancreatic Adenocarcinoma. Mo Med 2018;115:230-5. [PubMed]
- George B. Precision Medicine and Pancreatic Cancer. Surg Oncol Clin N Am 2021;30:693-708. [Crossref] [PubMed]
- Rawla P, Sunkara T, Gaduputi V. Epidemiology of Pancreatic Cancer: Global Trends, Etiology and Risk Factors. World J Oncol 2019;10:10-27. [Crossref] [PubMed]
- Narayanan G, Daye D, Wilson NM, et al. Ablation in Pancreatic Cancer: Past, Present and Future. Cancers (Basel) 2021;13:2511. [Crossref] [PubMed]
- Sun W, Ren Y, Lu Z, et al. The potential roles of exosomes in pancreatic cancer initiation and metastasis. Mol Cancer 2020;19:135. [Crossref] [PubMed]
- Chu LC, Goggins MG, Fishman EK. Diagnosis and Detection of Pancreatic Cancer. Cancer J 2017;23:333-42. [Crossref] [PubMed]
- Halbrook CJ, Lyssiotis CA, Pasca di Magliano M, et al. Pancreatic cancer: Advances and challenges. Cell 2023;186:1729-54. [Crossref] [PubMed]
- Vincent A, Herman J, Schulick R, et al. Pancreatic cancer. Lancet 2011;378:607-20. [Crossref] [PubMed]
- Zeng S, Pöttler M, Lan B, et al. Chemoresistance in Pancreatic Cancer. Int J Mol Sci 2019;20:4504. [Crossref] [PubMed]
- Ettrich TJ, Seufferlein T. Systemic Therapy for Metastatic Pancreatic Cancer. Curr Treat Options Oncol 2021;22:106. [Crossref] [PubMed]
- Kamisawa T, Wood LD, Itoi T, et al. Pancreatic cancer. Lancet 2016;388:73-85. [Crossref] [PubMed]
- Puckett Y, Garfield K. Pancreatic Cancer. StatPearls. Treasure Island (FL) ineligible companies. Disclosure: Karen Garfield declares no relevant financial relationships with ineligible companies. StatPearls Publishing Copyright © 2023, StatPearls Publishing LLC.; 2023.
- Collisson EA, Bailey P, Chang DK, et al. Molecular subtypes of pancreatic cancer. Nat Rev Gastroenterol Hepatol 2019;16:207-20. [Crossref] [PubMed]
- Kleeff J, Korc M, Apte M, et al. Pancreatic cancer. Nat Rev Dis Primers 2016;2:16022. [Crossref] [PubMed]
- Abe K, Kitago M, Kitagawa Y, et al. Hereditary pancreatic cancer. Int J Clin Oncol 2021;26:1784-92. [Crossref] [PubMed]
- Grant TJ, Hua K, Singh A. Molecular Pathogenesis of Pancreatic Cancer. Prog Mol Biol Transl Sci 2016;144:241-75. [Crossref] [PubMed]
- Luo J. KRAS mutation in pancreatic cancer. Semin Oncol 2021;48:10-8. [Crossref] [PubMed]
- Bannoura SF, Uddin MH, Nagasaka M, et al. Targeting KRAS in pancreatic cancer: new drugs on the horizon. Cancer Metastasis Rev 2021;40:819-35. [Crossref] [PubMed]
- Li J, Chen X, Kang R, et al. Regulation and function of autophagy in pancreatic cancer. Autophagy 2021;17:3275-96. [Crossref] [PubMed]
- Tempero MA. NCCN Guidelines Updates: Pancreatic Cancer. J Natl Compr Canc Netw 2019;17:603-5. [PubMed]
- Zhang L, Sanagapalli S, Stoita A. Challenges in diagnosis of pancreatic cancer. World J Gastroenterol 2018;24:2047-60. [Crossref] [PubMed]
- Morrison AH, Byrne KT, Vonderheide RH. Immunotherapy and Prevention of Pancreatic Cancer. Trends Cancer 2018;4:418-28. [Crossref] [PubMed]
- Karunakaran M, Barreto SG. Surgery for pancreatic cancer: current controversies and challenges. Future Oncol 2021;17:5135-62. [Crossref] [PubMed]
- Padoan A, Plebani M, Basso D. Inflammation and Pancreatic Cancer: Focus on Metabolism, Cytokines, and Immunity. Int J Mol Sci 2019;20:676. [Crossref] [PubMed]
- Timmer FEF, Geboers B, Nieuwenhuizen S, et al. Pancreatic Cancer and Immunotherapy: A Clinical Overview. Cancers (Basel) 2021;13:4138. [Crossref] [PubMed]
- Bear AS, Vonderheide RH, O'Hara MH. Challenges and Opportunities for Pancreatic Cancer Immunotherapy. Cancer Cell 2020;38:788-802. [Crossref] [PubMed]
- Xu L, Li Q, Xu D, et al. hsa-miR-141 downregulates TM4SF1 to inhibit pancreatic cancer cell invasion and migration. Int J Oncol 2014;44:459-66. [Crossref] [PubMed]
- Zheng B, Ohuchida K, Cui L, et al. TM4SF1 as a prognostic marker of pancreatic ductal adenocarcinoma is involved in migration and invasion of cancer cells. Int J Oncol 2015;47:490-8. [Crossref] [PubMed]
- Tang Z, Li C, Kang B, et al. GEPIA: a web server for cancer and normal gene expression profiling and interactive analyses. Nucleic Acids Res 2017;45:W98-W102. [Crossref] [PubMed]
- Liu J, Chen P, Zhou J, et al. Prognostic impact of lactylation-associated gene modifications in clear cell renal cell carcinoma: Insights into molecular landscape and therapeutic opportunities. Environ Toxicol 2024;39:1360-73. [Crossref] [PubMed]
- Li H, Zhou L, Zhou W, et al. Decoding the mitochondrial connection: development and validation of biomarkers for classifying and treating systemic lupus erythematosus through bioinformatics and machine learning. BMC Rheumatol 2023;7:44. [Crossref] [PubMed]
- Smith JD, Birkeland AC, Rosko AJ, et al. Mutational profiles of persistent/recurrent laryngeal squamous cell carcinoma. Head Neck 2019;41:423-8. [Crossref] [PubMed]
- Jones RG, Pearce EJ. MenTORing Immunity: mTOR Signaling in the Development and Function of Tissue-Resident Immune Cells. Immunity 2017;46:730-42. [Crossref] [PubMed]
- Taylor E, Yeung I, Keller H, et al. Quantifying hypoxia in human cancers using static PET imaging. Phys Med Biol 2016;61:7957-74. [Crossref] [PubMed]
(English Language Editor: L. Huleatt)